#3D image
Explore tagged Tumblr posts
Text
well, if I don't do much drawing anymore it's simply because I'm !
T-I-R-E-D
so suddenly I do 3d, because it reassures me and I don't have any problems with my "drawing style"
for those who have the image references ...
78 notes
·
View notes
Text
First ever creation on POVRay, its ORBSMAN >:)))
(The lighting was a pain in the ass and so was the experience of positioning the cones)
#povray#3d illustration#3d image#orbsman#tadc orbsman#tadc#the amazing digital circus#the amazing digital circus fan art#fanart#fan art#tadc fanart#the amazing digital circus orbsman#tadc ep 4
24 notes
·
View notes
Text
How to resize image without losing quality
Supervised Learning: KNN is a supervised learning algorithm, meaning it learns from labeled data to make predictions. Instance-Based Learning: KNN is also considered an instance-based or lazy learning algorithm because it stores the entire training dataset and performs computations only when making predictions.
Use Java Maven Project For Image Resize
youtube
media stands out as the best tool for resizing pictures. With its user-friendly interface and advanced resizing capabilities, Shrink. media allows you to adjust your pictures' dimensions and file sizes while maintaining excellent visual quality.
Boof CV for Java Image Processing
Java CV for Java Image Processing
ImageJ for Java Image Processing
Boof CV is a comprehensive library designed for real-time computer vision and image processing applications. It focuses on providing simple and efficient algorithms for tasks such as image enhancement, feature detection, and object tracking.
#animation#academia#animals#adobe#image comics#3d printing#image description in alt#image archive#image described#3d image producing#3d image process#3d image processing#3d image#machine learning#artificial image#100 days of productivity#Youtube
9 notes
·
View notes
Text
Improved Graphics
A little like watching a remastered movie or video game, as technology improves, scientists get the chance to revisit intricate natural structures and marvel at new details. Here we see a 'cinematic rendering' of a healthy human placenta, based on new data from cutting-edge imaging techniques. Using a form of phase-contrast X-ray tomography, high-powered particles, generated by a synchrotron, deflect off the surface of the living tissues revealing its contours to graphics specialists who reassemble the organ in 3D. Zooming in on different regions of the placenta like a panning shot across the surface of an alien planet, we see the twisting form of the umbilical cord and its network of blood vessels. While it’s exciting to see these dramatic details, these high-resolution techniques also help researchers spot clues to help both mother and child during pregnancy.
Written by John Ankers
Video from work by Jakob Reichmann and colleagues
Institute for X-ray Physics, University of Göttingen, Göttingen, Germany
Video originally published with a Creative Commons Attribution 4.0 International (CC BY 4.0)
Published in PNAS NEXUS, January 2025
You can also follow BPoD on Instagram, Twitter, Facebook and Bluesky
5 notes
·
View notes
Text
silly little wizard thing
2 notes
·
View notes
Text
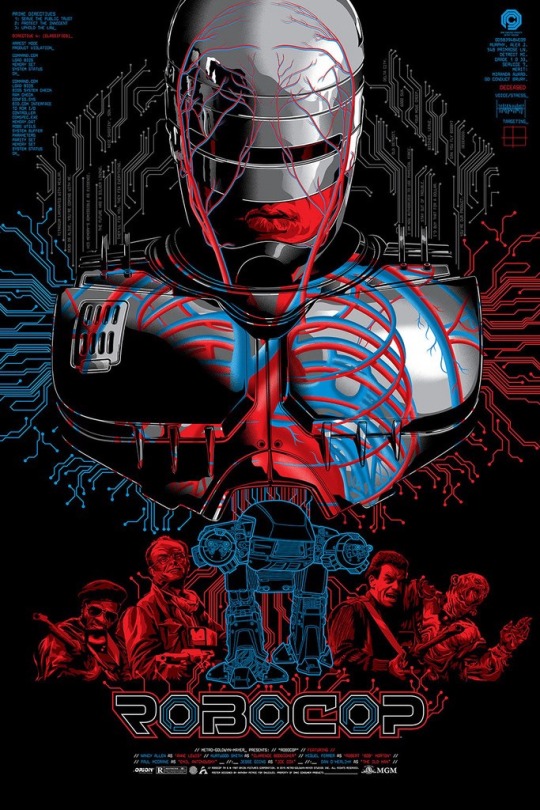
“Can you fly Bobby?”
9 notes
·
View notes
Text
Metaverse Design: The Future of Immersive Experiences
The rise of immersive experiences in the production of both in-person and virtual events – such as art shows, live concerts and interactive museum exhibits – is a well-documented phenomenon. However, the term can be punctuated by a certain vagueness, encompassing many different production practices from audiovisual enhancements to methods of interaction between performers and spectators.
Get Free Banner Designing Tutorial with Leonardo AI 👈

This brief overview aims to demystify immersive experiences and make a case for their incorporation into both virtual and in-person events – be it interactive art exhibits, immersive theater shows, or interactive online experiences.
#learn graphic design#pixel graphics#glitter graphics#web graphics#graphic design#3d banner design#3d image design#3d vector design#3d painting#3d image processing#3d image#ai generated picture#ai generated images#ai image editing#ai generated art#ai generated
5 notes
·
View notes
Text
Contrast and Emphasis: Creating Visual Impact
The use of contrasting colors in ancient Indian art: How bright hues like red and yellow are juxtaposed with cooler tones for emphasis. The role of negative space in Indian sculptures and paintings: How empty areas create a sense of depth and enhance the impact of the subject. Understanding the use of scale and size to create visual hierarchy: How central figures and objects are emphasized through their size.

Visual Communication
Purpose: To convey information visually rather than through text alone.
Application: Logos, posters, websites, packaging, ads.
Working Principle: Use of imagery, typography, and layout to quickly attract and inform the viewer.
FIRST PRACTICAL KNOWLEDGE 👈.
Process Workflow
Brief/Objective – Understand the goal.
Research – Study the target audience, competitors, and theme.
Concept Development – Sketch ideas and layouts.
Design Execution – Create digital or physical artwork.
Feedback & Revision – Refine based on input.
Final Output – Deliver in proper formats (print, web, etc.).
Second Practical Knowledge 👈.
Get more video lesson on @technolandexpart
#ai image generator#ai image editing#ai artwork#ai image#ai generated picture#ai generated images#ai generated art#ai generated content#stable diffusion#image fusion#3d animation services#3d model#3d artwork#3d image#3d image processing#3d animation company#3d animation#3d animator#3d animated story 2025#ai image creation#ai generated image
4 notes
·
View notes
Text
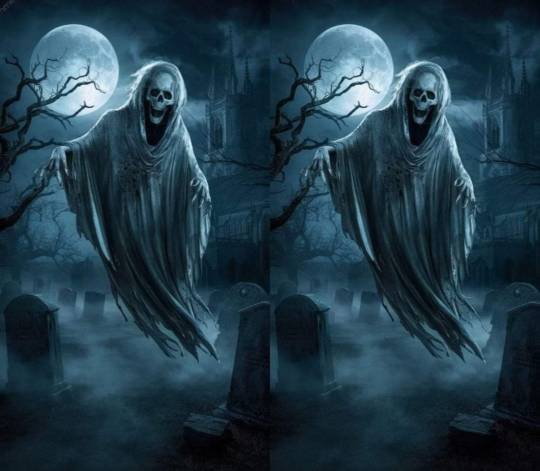
23 notes
·
View notes
Text
Creative marketing with the help of 3D Template Design
What Is 3D Template Design?
A 3D template is a pre-designed 3D model or layout that can be customized for branding, product presentation, or promotional content. These templates can be used in videos, interactive web elements, social media, AR.

GET FREE DESIGNING TOOLS (FOR STUDENT ONLY) 👈.
This is the process of creating the 3D geometry of your object, character, or environment. Polygonal Modeling: Creating objects using polygons (vertices, edges, and faces). This is the most common method for hard surface objects and characters. Spline Modeling: Creating shapes based on curves and then defining their thickness or depth. NURBS (Non-Uniform Rational B-Splines): For smoother surfaces, often used in automotive and industrial design. Sculpting: Involves pushing and pulling digital clay, commonly used in organic models like characters, creatures, or detailed objects.
IMPORTANT TOOLS AND SOFTWARE FOR DESIGN
Blender: Free, open-source software known for its versatility in modeling, animation, and rendering.
Autodesk Maya: Used widely in animation and visual effects. It excels at polygonal modeling, rigging, and animation.
3ds Max: Similar to Maya but often preferred for architectural visualization and game asset creation.
ZBrush: Specialized in digital sculpting, ideal for creating highly detailed organic models like characters.
Cinema 4D: Great for motion graphics and 3D modeling with a user-friendly interface.
SketchUp: Simple tool used mainly for architectural modeling.
#light particles#quantum jumping#quantum mechanics#quantum physics#subatomic particles#3d image#3d printing#3d image design#banner design#image archive#3d object#3d object creation#3d ai image creation#ai image editing#ai artwork#ai generated#ai art#ai image#ai model#artificial intelligence#3d banner design#3d model#animation#3d animation#ai image generator#3d ai image#ai image creation#ai video
1 note
·
View note
Text
I took a 3D image with the Gameboy Camera
Get your 3D glasses out (or look crosseyed at the bottom pic, your choice!)
Still amazed how well this turned out on the first attempt
#game boy camera#game boy#photography#2bitphotography#stereoscopic#3d#3d image#Pocket Camera#90s tech#I'm becoming way too powerful
10 notes
·
View notes
Text
Machine Learning Image Processing
Supervised Learning: KNN is a supervised learning algorithm, meaning it learns from labeled data to make predictions.
Instance-Based Learning: KNN is also considered an instance-based or lazy learning algorithm because it stores the entire training dataset and performs computations only when making predictions.
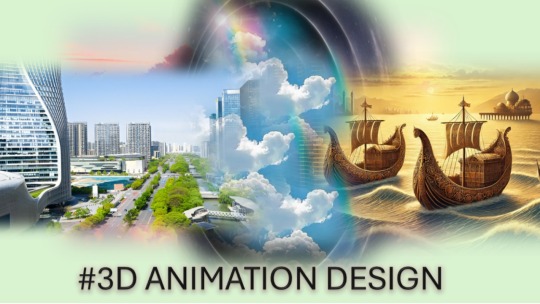
⬇⬇⬇⬇⬇ ⬇⬇⬇⬇⬇ ⬇⬇⬇⬇⬇ ⬇⬇⬇⬇⬇ ⬇⬇⬇⬇⬇ ⬇⬇⬇⬇⬇ ⬇⬇⬇⬇⬇ ⬇⬇⬇⬇⬇ ⬇⬇⬇⬇⬇ ⬇⬇⬇⬇⬇
youtube
Photography and Artistic Effects: In photography, image fusion can be used for artistic purposes, such as blending two images together to create surreal or blended effects. For example, blending a portrait with a landscape or combining different lighting conditions.
Techniques Used in Image Fusion: Pyramid Decomposition: This technique involves breaking down images into multiple levels using methods like wavelet or Laplacian pyramids, and then combining these levels to preserve important features from each image.
#animation#3d printing#academia#animals#2d animation#accounting#adobe#animation meme#digital animation#3d image process#3d image#3d image producing#image described#image description in alt#image archive#image processing#Youtube
6 notes
·
View notes
Text
Click & Detect
Click3D: a method using click chemistry (a class of reactions that make molecules observable) that achieves high-resolution 3D fluorescence imaging of whole organs at unprecedented depth – for example, imaging hypoxia (inadequate tissue oxygen) in a tumour (as shown here) or in the mouse whole brain
Read the published research article here
Video from work by Iori Tamura and colleagues
Department of Chemistry and Biotechnology, Graduate School of Engineering, The University of Tokyo, Bunkyo-ku, Tokyo, Japan
Video originally published with a Creative Commons Attribution 4.0 International (CC BY-NC 4.0)
Published in Science Advances, July 2024
You can also follow BPoD on Instagram, Twitter and Facebook
#science#biomedicine#immunofluorescence#cancer#biology#3d image#imaging#hypoxia#brain#neuroscience#click chemistry#click#chemistry
12 notes
·
View notes
Text
This is how Roy got Siegfried
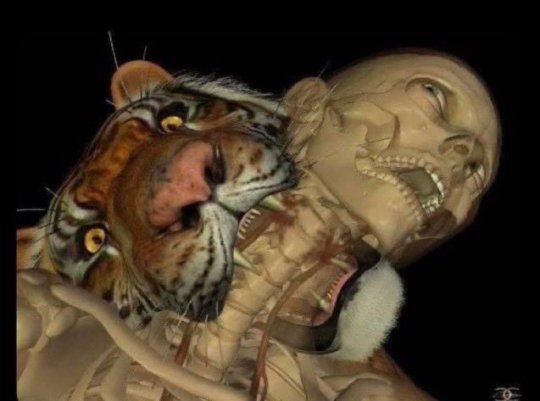
honestly for me this situation isnt drawing a single bead of sweat first i grab the tiger's jaw with both hands and pry it open, quickly bandaging the wounds i received (didn't hit anything important) and pushing the tiger away while simultaneously falling into my grizzly bear stance and watching as the tiger scurries away with its tail betweens its legs at the sight of my massive shoulder humps. i roar defiantly and catch three dozen salmon at the river
20K notes
·
View notes