Don't wanna be here? Send us removal request.
Text
Unveiling the Magic: Decoding Data Science and Machine Learning
In today's data-driven world, information is king. But raw data is like an undiscovered treasure trove - its true value lies in its insights. This is where data science and machine learning come in, the dynamic duo transforming data into actionable knowledge.
Data Science:Data Science: The Art of Exploration
Imagine a vast library filled with countless books. Data science is like being a skilled librarian, able to not only find the information you need but also uncover hidden patterns and connections. Here's what data scientists do:
Data Collection and Cleaning: They gather data from various sources and ensure it's accurate and usable for analysis.
Exploratory Data Analysis (EDA): They explore the data to understand its characteristics, patterns, and trends.
Data Visualization: They create compelling charts and graphs to communicate insights effectively.
Statistical Modeling: They build models to predict future outcomes or classify data points.
Machine Learning: The Algorithmic Powerhouse
Machine learning algorithms are like intelligent students who can learn from data without explicit programming. They can identify complex patterns and make predictions based on those patterns. Here are some common types of machine learning:
Supervised Learning: Algorithms learn from labeled data to make predictions for new, unseen data (e.g., spam filtering).
Unsupervised Learning: Algorithms uncover hidden patterns in unlabeled data (e.g., customer segmentation).
Reinforcement Learning: Algorithms learn through trial and error to maximize rewards in a specific environment (e.g., self-driving cars).
The Power of Together: Data Science and Machine Learning
Data science and machine learning are a perfect blend. Data science provides the foundation for understanding and preparing data, while machine learning algorithms leverage this data to make predictions and uncover hidden insights. Together, they revolutionize various fields:
Finance: Fraud detection, risk assessment, personalized financial products.
Healthcare: Disease prediction, personalized medicine, drug discovery.
Retail: Recommendation engines, targeted marketing, customer churn prediction.
Become a Data Science Mastermind
The demand for data science skills is skyrocketing. If you're fascinated by the potential of data and want to unlock its secrets, consider enrolling in a comprehensive data science course like the one offered by Dicecamp.
Dicecamp Data Science Course:Data Science Course: This course can equip you with the essential skills in Python, data wrangling, visualization, and machine learning algorithms. Whether you dream of becoming a data scientist, business analyst, or anyone who wants to leverage data for better decision-making, this course can be your launchpad.
The future belongs to those who can harness the power of data. Are you ready to join the data science revolution?
To get more information , Kindly Visit:
Data Science & Machine Learning Course
Bridge the Gap Between Data and Decisions: Master Data Science Course using Machine Learning Empower yourself with the in-demand skills of data science and machine learning through our dynamic Applied Hybrid Training program! This innovative data science course seamlessly blends classroom instruction with online learning, providing a well-rounded foundation for your data science journey. Learn to unlock the power of data and leverage machine learning algorithms to solve real-world challenges. Uncover the Magic Behind the Data: Machine Learning Fundamentals: Demystify the concepts of machine learning algorithms and explore their practical applications across various industries. Python Programming Prowess: Gain hands-on experience with Python, the language of choice for data science. Learn how to leverage its libraries and tools to implement machine learning models effectively. Data Wrangling Expertise: Master techniques for handling and manipulating datasets from diverse fields. Understand how to prepare data for optimal use with machine learning algorithms. Actionable Insights from Algorithms: Discover how to interpret machine learning outputs and translate them into actionable insights that drive real-world results. Data Communication Mastery: Learn to communicate your data science findings with clarity and impact, effectively presenting the results of your machine learning models. By the end of this Data Science Course using Machine Learning , you will have enough knowledge and hands-on expertise in Python to use and apply them in the real world around you. Also, you will be able to get prepared for certifications of Data Camp and Cognitive AI.
0 notes
Text
Generative AI Course - Dicecamp
Master the future of content creation! Learn how Generative AI can generate unique text, images, and music in our comprehensive Generative AI course.
Link to get Registered: https://dicecamp.com/generative-ai-course
0 notes
Text
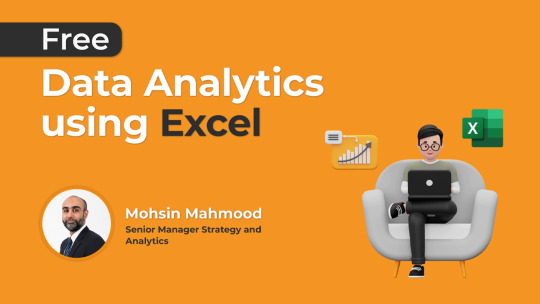
Data Analysis Using Excel Free Course
In this training you will explore how to get started with a non-technical background with Micosoft most used tool Excel. This complete training will help you master all the basic elements of Excel – Statistical use, and Business Knowledge.
Enroll Now and earn a Certificate :
https://dicecamp.com/data-analytics-using-excel-crash-course
#datanalysis
#excel
#freeexcelcourse
#excelcourse
#sql
#sqlcourse
0 notes
Text
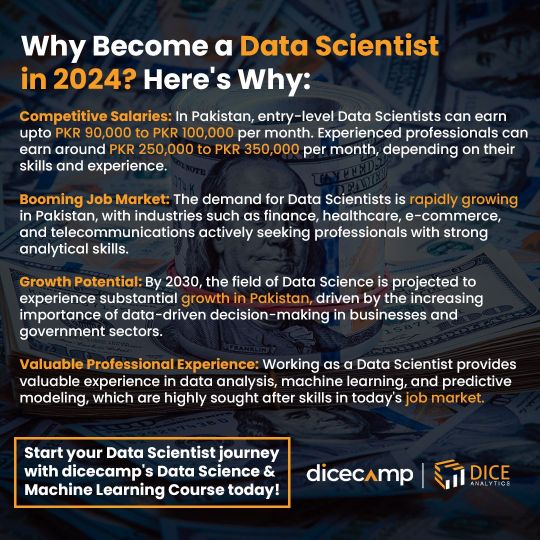
Why become a Data Scientist in 2024?
Why become a Data Scientist in 2024? The demand for Data Scientists is rapidly growing in Pakistan. Enroll in our Data Science & Machine Learning course today.
Link to get registered : https://dicecamp.com/data-science-machine-learning-course
0 notes
Text
SQL Server is quickest way to your Data Analytics journey, says expert
SQL server tools get you through the whole, end-to-end ‘data ecosystem’; where you learn data engineering, data warehousing, and business intelligence all in single platform
Microsoft SQL Server is currently leading the RDBMS market with its tremendously diverse tools and services for data analytics. Whether it’s data management, analysis or reporting, you get all in one package, that too for free.
Given that SQL server provides an end-to-end exposure to the whole data ecosystem, learning SQL server is the quickest path to your data analytics journey..
Note: This career advice is for newbies just starting their analytics journey, as well as for technical geeks who wish to opt for SQL server job roles.
Table of contents
Data Ecosystem and SQL Server Tools
How can SQL Server help me begin a Career in Data Analytics?
Career Tracks to target a job role
Learn SQL Server Tools
Watch Webinar!
Data Ecosystem and SQL Server tools
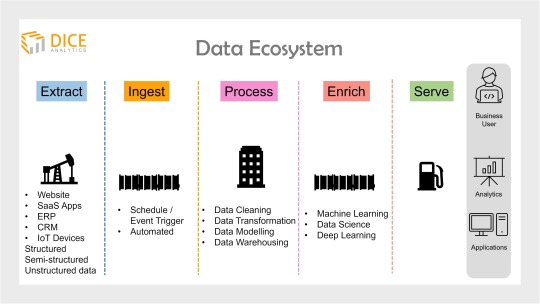
Data ecosystem is the backbone of any organization’s data analytics project.
Simply put, a data ecosystem documents and presents infrastructure and applications for data storage and processing.
Any data ecosystem portrays four to five stages of data, depending on the organization’s objectives.
Starting off, a data expert always needs to collect data from the vast sources of the organization. This includes, website data, SaaS applications, IoT devices, CRM, ERP etc.
Next, all of the data from diverse sources is gathered over a common place through a process called ingestion.
Integrated on a single database, this data needs to be cleaned, transformed and organized into a universal format (data harmonization) to avoid misalignment across the ecosystem.
This process is called data warehousing (aka data engineering).
Optional is to further enrich data using machine learning technology. One of the main data science job roles is to apply predictive analytics at this stage.
Finally, at the last stage, data is analyzed and presented to business users for value driving and decision making. A BI developer or engineer is specialized to handle data visualization at this stage.
SQL Server tools and services offer a low code environment to all of the above steps and therefore quickly and easily helps to build an end-to-end data ecosystem for an organization.
The tools and services can be broadly classified as data management and business intelligence (BI) functionalities.
For data management, SQL Server provides SQL Server Integration Services (SSIS), SQL Server Data Quality Services, and SQL Server Master Data Services.
SQL Server provides SQL Server Data tools for building a database. And for management, deployment, and monitoring the platform has SQL Server Management Studio (SSMS).
SQL Server Analysis Services (SSAS) handle data analysis.
SQL Server Reporting Services (SSRS) are used for reporting and visualization of data.
Earlier known as the R services, the Machine Learning Services came as part of SQL Server suite in 2016 and renamed afterwards.
How can SQL Server help me begin a Career in Data Analytics?
When you learn SQL Server, it exposes you to the complete data ecosystem. This helps you in your career advancement in two ways.
Access to the vast SQL Server jobs
Microsoft SQL Server currently stands at 3rd rank (after Oracle and MySQL) in the world’s most used commercial relational databases. This is because Microsoft offers an intensely feature-rich version of SQL Server for free.
This makes SQL server skills one of the most in-demand across the data analytics ecosystem.
Tip: For newbies, and those with career transitions, if you want to land on an analytics job quickly, learning SQL server tools is a smart idea since the job market is lucrative.
Further, once in, as you move along in your career we recommend growing your skill set and ascending towards more specific job roles, for example, data engineer and BI developer.
Career Tracks to target a job role
Once you get a grab of the end-to-end data analytics ecosystem, now it’s a step to move forward in your analytics journey.
But why?
Data analytics is a broad field, and carries lucrative job opportunities in the form of various job roles available in the market.
Moreover, given a myriad of job roles, you can opt for a career in the field of your interest.
What are the career tracks when I work with SQL server?
Become a Data Engineer
Once getting to know what data engineering holds, you can now opt for a vendor specific data engineering skills.
For example, Teradata is a market leader in on-premise data warehousing solutions. Learning data engineering on Teradata will offer bright career prospects in the data analytics field.
While SQL Server and Teradata RDBMS have a data architecture built for small scale data, when it comes to data volumes up to petabytes size, these solutions don’t work.
Thus a data engineer can move to learning big data technology that holds even brighter career prospects (read about the blooming big data market forecast).
Become a BI Developer/BI Engineer
This job role narrows to the data visualization, and reporting only. A BI developer is expert in BI tools such as Power BI and Tableau.
As a next step, a BI Developer can also opt for no code/low code Data Science using Knime.
Become an ML Engineer
A Machine Learning engineer uses ML technology to employ predictive analytics and finds out future trends and patterns within data.
The requirement for an ML engineer is to understand how databases and data warehousing works, and needs to build a strong foundation in that.
Next, you can opt for Deep Learning in your career journey for better positions in large enterprises.
Become a Data Analyst
After getting to work with SQL server tools, you can also opt data analyst as your career choice. This requires you to build expertise in BI tools such as Power BI and Tableau.
The next step for career advancement is to learn Business Analytics that deals with business data and marketing analytics.
You might want to view Business Analytics career prospects and salary in Pakistan.
Interested in Learning SQL Server Tools?
Dicecamp offers an 8 weeks course* on Learning SQL Server Tools.
The course covers four tools; SQL Server Integrated Services (SSIS), SQL Server Management Services (SSMS), Azure Cloud, and Power BI.
You will learn:
SQL hands-on
DWH building using SSIS
Data Management using SSMS
AZURE SQL CONFIG, DTU basics
DAX implementation in Power BI
Data visualization in Power BI
Visit complete course outline and registration details here.
*We offer flexible pricing and valuable concessions.
Straight from the Horse’s Mouth!
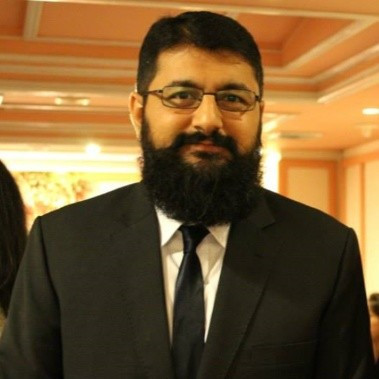
The instructor of this course is Mr. Abu Bakar Nisar Alvi who’s Pakistan’s celebrated engineer awarded Tamgha e Imtiaz (fourth highest civil rank) for his excellent engineering performance back in 2005.
Mr. Alvi serves as a senior IT consultant at the World Bank with key experience in enabling digital transformation as part of the village service delivery in Indonesia.
Taking two decades of experience and vast work diversity, Mr. Alvi is now associated with Dicecamp as a lead trainer Data Analytics and Visualization.
Webinar: Watch him speaking on ‘Why to Learn SQL Server Tools’ in the latest webinar (LinkedIn Webinar Link).
#dicecamp#datascience#careertest#devops#dataengineercourse#datawarehouse#devopscourse#datawarehousecourse#sqlserver#sql#mysql
1 note
·
View note
Text
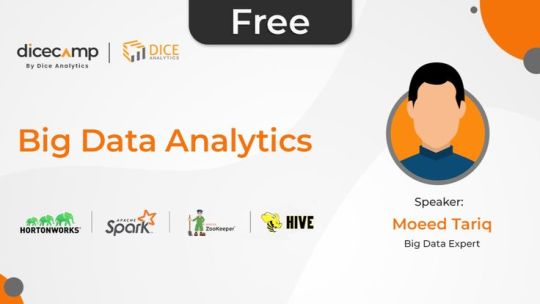
Big Data Analytics Free Course
During this interactive training , you will learn about the different ingredients of Big Data such as Hadoop, Spark, Pig, Hive & Sqoop. Further, you will have hands-on experience on different pillars of the Big Data Ecosystem starting from parallel processing frameworks like Map Reduce & Spark, Distributed Storage techniques like HDFS, Big Data Administration Ambari etc. At the end of the training, you will have an in-depth understanding & hands-on related to Big Data solutions like Cloudera & HortonWorks.
Link to get you registered : https://dicecamp.com/big-data-analytics-crash-course
#dicecamp#bigdatacourse#bigdatafreecourse#hadoopfreecourse#cloudera#clouderafreecourse#hortonworks#hortonworksfreecourse#freecourse
0 notes
Text
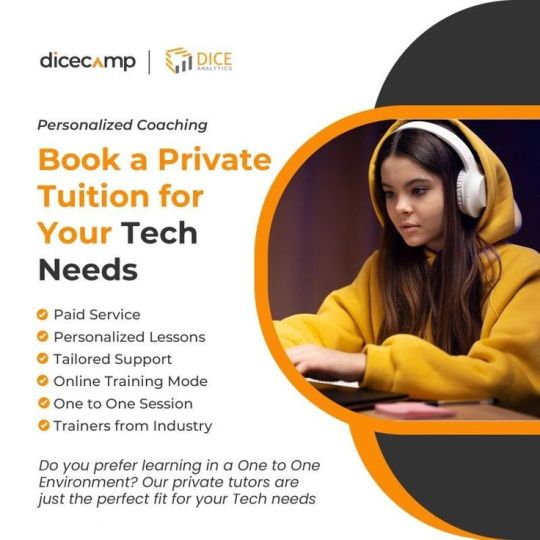
Book a Private Tuition for Your Tech Needs
Decide yourself what, where and when you learn!
Do you prefer learning in a 1 to 1 environment? Our private tutors are just the perfect fit for your Tech needs. Get a tailored curriculum and exclusive support that suits your learning approach.
Link to get you registered : https://dicecamp.com/acquire-skill/private-tution
0 notes
Text
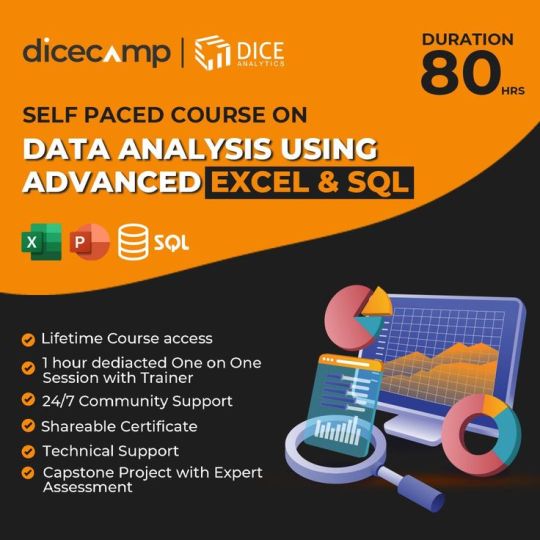
Data Analysis Course using Advanced Excel & SQL
Do you want to gain expertise in Excel and SQL, starting from the very basics and advancing to the next level for top-tier data analysis? Secure your spot now in our Data Analysis using Advance Excel and SQL 80 hours Self-paced training by Dicecamp and transform your analytics game with practical insights and real-world applications.
Reserve your seat Now : https://dicecamp.com/data-analysis-using-advance-excel-and-sql-course
0 notes
Text
8 winning qualities of a Quality Assurance Lead | Become a QA expert
Quality assurance and software testing has become important more than ever.
In the fast paced environment of today’s software development industry, the high speed delivery of any software requires a roundly efficient and effective quality assurance workflow. Thus the role of a QA engineer has now evolved to some thing that works hand-in-hand with all stakeholders and develops new processes that support quality testing, in parallel to software development.
Maintaining such a graceful process is a challenge. A QA lead is that remarkable personality who uses a set of core skills to make it possible.
These skills are presented as 8 qualities of great QA leads which makes them create a reliable quality assurance mechanism in today’s agile development environment.
Table of contents
QA Engineer vs. Software Tester
QA engineer roles and responsibilities
8 Qualities of great QA lead
How to become a QA engineer?
Career paths for QA engineer
Career transition to quality assurance
Who is a QA Engineer? And how do they differ from a Software Tester?
A QA engineer is a vibrant personality in the quality management department who plays an active role across the whole testing life cycle– from requirement analysis to forming testing strategies and managing change. As a core requirement of this process, a QA specialist is responsible for improving a company’s current testing life cycle through new process establishment, and evaluation.
Software testers, on the other hand, play just part of the whole quality assurance role. These personalities mainly work at the end of the testing life cycle– perform test case execution and report findings, although not always necessary. What differentiates quality testers from QA engineers is that a quality tester implements a process while a QA specialist establishes and improves thereof.
Job Description of a QA Engineer
A software company requires QA engineers to establish and maintain a quality and reliable testing mechanism for its products/projects.
A QA engineer uses their skills to enable a cost friendly quality control mechanism for a company. These skills include sound knowledge and hands-on ability in the software testing life cycle– STLC, agile testing methodology, and project management.
Generally, a software company seeking an expert QA engineer envisions fulfillment of the following job responsibilities.
Gain first-hand knowledge via meetings with all business and nonbusiness teams starting at the earliest stage of the SDLC.
Evaluate existing processes and establish agile testing practices to improve test productivity.
Set quality standards and metrics that reflect the company’s values and fulfill client’s requirements.
Inform and inspire the development team to fulfill the minimum quality standards during software development.
Cover an extra mile by collaborating with clients and ask for critical details on user and software requirements.
Manage and take on change requests at any stage during the software development.
Evaluate the bug severity and urgency and create simplicity in communication to the development team.
Involve and encourage all QA engineers and software testers in quality assurance workflows through distribution of work based on talent.
8 Qualities of a Great QA Lead
A great QA lead has a set of core skills that help them establish an adaptive quality assurance mechanism that guarantees cost and time saving for the company.
Following are 8 winning qualities of a great QA lead:
1. To them, Quality Assurance is nothing without 3 things
SDLC & STLC
Testing Methodology
Project Management Phases
To briefly state the above, a QA lead applies knowledge from these three core areas to manage the software testing life cycle. Here’s how it helps them:
STLC + Project Management: To enable a reliable testing life cycle, a QA lead builds an STLC workflow based on project management principles. This is achieved using the 5 commonly known project management phases:
Understanding: A QA lead interacts with the teams dedicated at every phase of the development cycle (SDLC) to truly understand business requirements
Planning: A quality assurance plan is developed as per business requirements.
Execution: The QA plan is executed at this stage.
Feedback: Feedback from client is collected and incorporated in another round of testing.
Windup: The QA efforts are considered done after the delivery of quality assured software to the client.
However, to comply with the fast paced development environment we have these days, project management driven STLC is still not enough.
… + Testing Methodology: Testing methodologies guide a QA lead to adopt an adaptive project management approach (one that welcomes changes to effectively meet the requirements of the business stakeholders). For clarity, an example of this could be a QA lead regularly reflecting on the current practices to identify improvement needs.
About Testing Methodology: A testing methodology refers to either the waterfall or agile (also termed as scrum) method of software development. At its core, it defines a set of behaviors for SDLC teams to effectively meet development goals. For this, the testing methodology reflects if the phases in a software development life cycle run in series or in parallel.
In the waterfall model, for example, a phase once completed is bound for no revisits. This is just like the manner of how water falls in a spring. But, as customer requirements started to change frequently in the 1990s, developers found the waterfall approach ineffective in managing change. Thus, as a result of combined efforts from developers, the Agile concept was established.
Briefly stating, the Agile concept focuses on customer and cross-team collaboration on a daily basis. Alongside, it establishes a mechanism to adjust the resulting feedback by breaking big tasks into smaller ones and enabling continuous delivery of changes in the software.
2. Prioritizes bugs on severity
A great QA lead evaluates and prioritizes bugs on the basis of severity with the aim of taking the development and testing to an ultimate level of productivity.
The QA lead uses a system called a bug triage to identify and evaluate bugs. A bug triage takes a proactive approach and considers shortlisting bugs based on severity. At its minimum, a bug triage takes three subsequent stages. It starts with identifying how problematic the bugs are. Each bug is then prioritized for fixing along with finding the processes that fix them.
The frequency of bug occurrence is also taken into account so that the team knows how the discovered processes should be used to avoid recurrence of bugs.
3. Won’t skip Unit & Integration testing before System testing
Ideally, any software testing starts with the smallest feature or a ‘unit’ and gradually moves to the system level features. Finding and fixing bugs at the micro level of a software eases the testing pressure on QA team at the later stages.
A great QA lead thus prioritizes unit and integration testing before jumping to the system level testing. They also understand the impact of unit features on customer experience and dedicate resources for unit and integration testing.
A wrong approach for QA teams is to jump straight to the system testing and taking on the pressure of untouched features at the unit and integration levels.
4. Places collaboration at the heart of QA
A great QA lead autonomously gets involved with all stakeholders (including business teams and customers).
As per the Agile testing philosophy, the QA specialists are to engage with business and development teams starting right at the beginning of the software development life cycle.
The QA lead thus builds an autonomous team, one that independently collaborates with all other teams and takes a first hand knowledge of the quality requirements. The QA specialist knows the fruits which are brought as a result of software testers being aware not only of the client and business expectations but also the thought process of the intended users.
In other words, It’s only through an extensive collaboration that a QA lead becomes able to deliver remarkable quality standards as perceived by the business, the client and the users.
With the idea of autonomous quality assurance teams, the QA lead is also encouraged to independently divide work among resources and plan and estimate time for the test life cycle.
5. Distributes work based on talent
A great QA lead understands every QA resource has to play a pivotal role across the whole testing lifecycle. They have the eye that sees what each member is best capable of and divide responsibilities accordingly.
For example, a testing engineer well-versed in written communication could be assigned the documentation work. Another member who’s excellent in strategy formation might be assigned with formulating the test cases. Similarly, a testing engineer with strong analytical ability might be dedicated to perform user requirement analysis.
6. Understands that Testing is not a phase (Agile testing principles)
Software testing has become an integral part of the complete software development process instead of being an isolated phase. This new paradigm of quality assurance is called agile testing and is part of the agile development methodology.
So, working as part of the agile testing environment a QA lead changes conventional testing principles to adopt the following agile principles:
Testing is not just for designated testers: A QA lead understands that testing is no more confined to software testers only. They involve all teams across the development lifecycle to adhere to the minimum quality standards.
Test driven development: Testing runs in parallel with each development sprint instead of in the last.
Test early: The QA lead establishes efficient testing processes that ensure bug detection and resolution before the sprint is completed.
Further, a QA lead nourishes the testing environment with automation testing; an agile practice. They utilize the capability of automation to shorten a sprint period and accomplish maximum testing within that sprint. At the minimum, a QA lead automates unit and integration tests.
7. Knows the art of Change Management
A great QA lead knows the art of effective change management and successfully adapts their testing strategy to adjust for new software changes.
While working in an agile environment, there’s always the possibility of change requests even at a later stage in development. The sources of change could include for example, detected bugs and errors, changes in market dynamics or customer interests, or business reorganization.
As change requests occur, the QA lead gears-up the testing team and uses change management principles to establish processes that perform testing under time and cost constraints. Once the new changes have been tested, the QA lead communicates these changes to developers and the cycle continues.
8. Avoids failure cost of Quality
A great QA uses effective test planning and execution to avoid the failure cost of quality. They save the company’s spending on expensive quality assurance that might occur after the production stage.
To minimize failure cost of quality, the QA lead ensures adequate spend before arrival of the production stage and believes to bring greater good to the company
First cost which the QA lead puts focus on is the prevention cost. It’s the cost that is incurred during the planning stage of the software testing life cycle. The amount is spent on establishing quality assurance processes such as user and software requirement analysis, prioritizing bug fixes, and developing testing processes. This can also include hiring of specialists and team upskilling.
Second is the appraisal cost that is incurred on the software before it is shipped to the customer. It’s the amount a company spends to ensure an effective test execution and reporting.
Setting a quality testing cycle like such, the QA lead is able to save the company from failure costs and the reputational damages that it brings with it.
Become an expert Quality Assurance lead: Online Course
Dicecamp brings a complete course on quality assurance and software testing led by a leading industry expert. Find more about the course.
Why take this course: Individuals can learn the ability to become a qualified Quality Assurance lead who confidently takes forward an organization’s quality assurance initiatives.
What will you learn: This course covers all the core theory, tools and techniques used in the area of software testing and quality assurance. You will learn: software testing techniques, software testing planning & strategies, software testing management, agile testing, and software testing automation.
View the detailed course outline.
What are possible Career Paths for a QA lead?
As a QA engineer you are open to more charming opportunities such as QA developer in test, QA in DevOps and DevOps engineer.
DevOps is the glorious role in current technology scape that offers handsome salary along with remarkable tributes. The demand for DevOps skills has severely increased worldwide with supply remaining at dire levels.
Taking this opportunity, a QA specialist can easily build on top of their quality assurance profile and learn DevOps skills.
Here’s how to do this step by step.
Learn and practice automation testing: The first step for you is to learn and practice automation testing. You would need following skills:
Knowledge of Python or Javascript for writing automation tests.
API automation testing
SQL query writing and Shell Scripting
CI/CD pipeline testing/Integration testing using Jenkins and/or Gitlab
Development (Fix a group of bugs): Companies leverage the proximity between software testing and development by offering the job role of a ‘QA Software Developer in Test’. Testers at this job role manage testing and development in consecutive phases. You would need following skills:
Programming knowledge and hands-on skills on high level languages.
By this stage, an individual has 3 core skills of a DevOps engineer: quality testing skills, automation testing hands-on and programming language proficiency.
With little more effort, a software quality testing specialist can adopt a full DevOps engineer role.
Learn configuration management:
CLI of Unix/Linux or Windows OS.
Configuration automation tools (Puppet, Chef, Ansible)
Container run time (Docker, rkt, cri-o)
Container orchestration tools (Kubernetics)
Knowledge of any one cloud platform: AWS, Azure etc
Start your QA journey today! Watch the webinar.
youtube
#dicecamp#datascience#sqacourse#softwaretestingcourse#qualityassurancecourse#data scientist#data science course#selfpacedcourse#Youtube
0 notes
Text
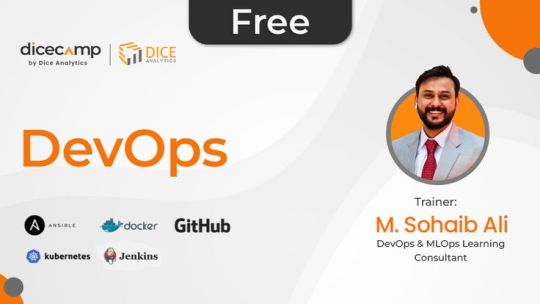
DevOps Free Course - Dicecamp
DevOps Free Course
Launch your DevOps journey with Dicecamp's free course! Learn core DevOps principles and tools to streamline software delivery. Get introduced to Git version control, CI/CD pipelines with Jenkins, and containerization with Docker. This course equips you for a successful transition into the exciting world of DevOps.
Link to get Registered : https://dicecamp.com/devops-crash-course
0 notes
Text
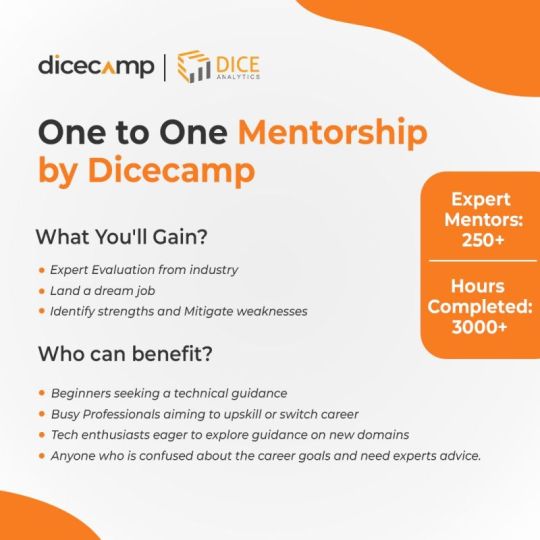
Mentorship Session - Dicecamp
Feeling stuck in your career? Get expert guidance to propel your journey forward! Join our personalized 1-on-1 sessions with seasoned professionals. Gain invaluable insights, goal clarity, and enhance your tech skills. Don't miss out!
Link to get Registered ; https://dicecamp.com/mentorship
0 notes
Text
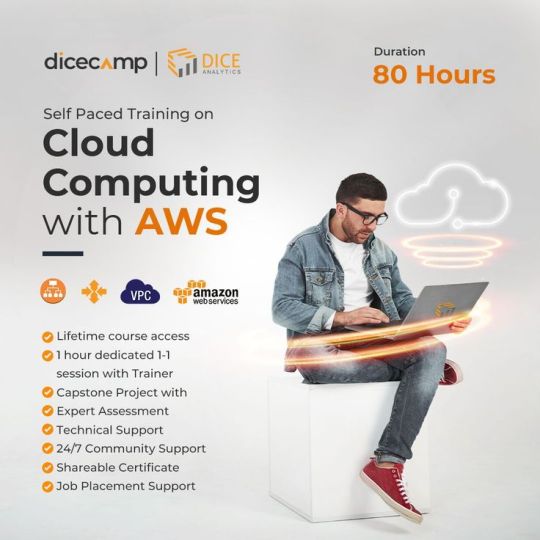
Cloud Computing Course With AWS
Elevate your career with our Cloud Computing with AWS self-paced course! Over 80 hours, you'll gain in-depth knowledge and hands-on experience with AWS, the leading cloud platform. 💻 Equip yourself with the skills needed to thrive in the rapidly evolving tech landscape.
Link to get Registered : https://dicecamp.com/cloud-computing-course-with-aws-
#dicecamp#datascience#cloudcomputingonlinecourse#cloudcomputing#cloudcomputingcourse#selfpacedcourse#careertest
0 notes
Text
Data Science is for everyone! (Build AI in minutes with no-code)
Data Science is now possible without Python. As per Gartner, majority of analytics workloads in future would be on no-code data science tools, making it a no-sweat skill.
While it’s an irrefutable fact that data science brings immense benefits for businesses in the form of remarkable efficiency and accuracy in decision making, it’s also true that it brings with itself the toil of crafting a recondite pipeline. And to build a working analytics system, data scientists master the inter-disciplinary knowledge that extracts the crux of otherwise out-of-context data.
Now, as we’ll make it clear to you, organizations want to leverage a simple and intuitive way towards data science that ideally empowers anyone to harness its powers. For this, technology has created a non-technical paradigm of data science: the no-code Data Science.
With no-code Data Science, it literally becomes possible for anyone to apply data science principles and process data into legitimate insights as accurately as with code. Combined with data literacy, users can drag and drop data nodes on the project screen and build an AI within minutes. Just that’s how simple it is.
This article disseminates everything about no-code Data Science- covering the context of no-code technology and its working, best no-code AI tools, citizen data scientists and how to become one, and a note on the future of Data Science with no-code platforms.
Stick by to discover how aspiring professionals can learn no-code Data Science at the comfort of their homes with live-sessions by certified experts.
Jump to your Intended Topic in no-code Data Science
1- What exactly is Data Science?, Skills, and Supply Gap.
2- The no-code Movement and Data Science.
3- List of best no-code AI platforms, and details.
4- Can I become a citizen data scientist? Pretty Easily!
5- How no-code data science tools help organizations?
6- No-code Data Science a threat to the job security of Data Scientists?
What’s Data Science?
Data Science is the application of machine intelligence to make business decisions in the shortest time and with greater wisdom. This machine intelligence is created by Data Scientists who blend statistics, artificial intelligence, and domain knowledge together over vast volumes of data.
In simple terms, a data scientist rounds up relevant business data, cleanses and structures the data, and builds statistical models to look for unseen patterns. These patterns (as validated through historical data) act as knowledge for business managers and help them make business decisions.
What are the Skills of a Data Scientist?
Primarily, a data scientist requires hands-on expertise in: mathematics and statistics, programming languages (Python, R and SQL), and application domain knowledge (visit our dedicated career guide if you wish to understand more about skills of data scientist).
Scarcity in Supply of Data Scientists
The toil aspiring individuals take to become professional data scientists, as well as the effortful work in constructing an analytics pipeline, results in scarcity of Data Scientists. On the other hand, the demand for data scientists is more than ever, with companies intrigued to leverage the competitive advantage data science provides.
This supply-demand gap becomes an inspiration for engineers to create tools that offer intuitive data science capabilities. They envision easier accessibility to data and analytics with non-specialists generating and creating complex statistical models with as little effort as possible. This gives rise to non-traditional data science roles that require abstract knowledge of data and analytics compared to the profound expertise in statistics and programming required by professional data scientists.
Gartner termed these emerging data science roles as Citizen data scientists and extrapolated them as dominant players who can maximize an organization’s D&A strategy. Building on Gartner’s view, no-code tools and automation are two key drivers that can empower citizen data scientists in successfully conducting analytics operations.
Read further as we deep-dive into what’s no-code Data Science.
What’s no-code Data Science? It all started with the no-code Movement
The no-code movement emphasizes technology usage for everyone, offering non-technical persons to build things such as websites and data analytics applications. The movement solely exists to empower every individual to implement their unique ideas and use the power of technology in easiest ways. Note: to gain first-hand account, visit the no-code platforms such as MarkerPad and Nocode.tech.
As the no-code movement expanded across technology disciplines, data science gurus also saw greater enablement, a platform idea that makes it possible for anyone to build data science models without having to code. Using these platforms, implementing data science workflows has become extremely easy and quick thanks to the drag-and-drop layout. There’s literally no coding involved that was earlier required to write and execute each step along the data science hierarchy (including feature engineering and model building).
So how does no-code Data Science work?
No-code data science platforms leverage the power of programming (at the backend) to create intuitive drag-and-drop functionalities at the user interface. The four core characteristics of a no-code data science platform are:
Visual programming that provides graphical layout with drag-and-drop capabilities. Users pick a component (or node) and drop it on the project window before building their logical data hierarchy.
Additional flexibility of embedded code editor as in Data Query, for customization of processes.
Provision of APIs that lets users import their no-code prediction data to their applications, or visualization tools such as Power BI and Looker etc.,
Hundreds of pre-built analytics components such as data readers, data joiners, and complex statistical models etc., save hours of time and effort for data science teams.
AI is now possible without coding! The Best no-code AI platforms for all business users
Following is a list of top no-code AI platforms that cover all applications of artificial intelligence including Data Science (Learn Data Science vs. AI). As you’ll witness below, with these tools, data science is possible without python.
Microsoft Lobe
Microsoft takes the no-code machine learning experience for non-specialists to an ultimate level with its Lobe application. It comes as a downloadable, free application that has an incredibly creative yet super intuitive graphical layout that makes sense of ML to any layman.
Users start with uploading their data onto Lobe. This is example data (also known as historical data) using which users make an ML model learn and discriminate between a variety of examples. Once imported, Lobe automatically trains a ML model (also selected by Lobe) and displays a model performance report. This is where users can see and verify if the ML model actually predicts in the way they expect it to. For example, users can upload new data if the model displays wrong predictions. Once fixed, use Lobe to export the model onto any application.
Download and train your ML model on the go using Lobe. Watch how Lobe trains an ML model in minutes time with its super intuitive layout .
Akkio
Akkio markets itself as one of the best no-code data science tools in that it offers fastest model training in a work environment that’s highly intuitive. It’s with these features that professionals from sales, marketing, and finance find Akkio as a promising no-code platform for predictions such as customer churn, subscription score and customer acquisition.
Visit Akkio to get a free trial of Akkio Application.
Google Auto ML
Another no-code platform in the list of best no-code AL tools is Google Auto ML. Auto ML covers the whole gamut of ML (ranging from data science to pure ML tasks), providing a simple, no-code solution for custom business needs.
Google AutoML is a paid application.
Amazon SageMaker
Amazon provides the best no-code machine learning experience for developers and data scientists alike with its incredible no-code AI tool called SageMaker. With its intensely rich machine learning capabilities, non-specialists, data scientists and developers save time and effort in data preparation, building, training, and deployment of models.
Starting with feature engineering, SageMaker Data Wrangler automatically builds features on users’ selected data. Users can save different versions of features in the SageMaker Feature Store and select and use them for model training. Now, trusting an ML is another problem that SageMaker gracefully handles with its SageMaker Clarify. It suggests a balanced feature set by checking for issues such as: biases towards a single feature. An individual feature’s role can also be inspected using Clarify. Once a model is trained, the SageMaker Debugger identifies improvements in an ML model by measuring for example CPU memory, and number of violations on training data. Finally, SageMager pipelines helps data scientists and developers to automate the whole ML development process in a single click.
See how Amazon SageMaker expedites a data science workflow within minutes.
Users can try Amazon SageMaker for free for 2-months after they sign-up to AWS Cloud.
KNIME
Competing with top no-code AI platforms, KNIME Analytics platform successfully delivers the simplicity and ease of building machine learning algorithms for data science applications (check out KNIME Reviews at Gartner Peer Reviews). It’s a visual, no code analytics platform that lets all business users create powerful machine learning algorithms by simply dragging data nodes on KNIME workbench.
KNIME Analytics Software is absolutely free to download and use.
As you’ve studied the above best no-code data science tools, we don’t want to miss yet another top no-code platform that’s loved by its users. Obviously AI is a complete data science team and offers capabilities that merge and clean data, and perform statistical work.
Become a citizen data scientist or simply save time and effort in the tedious building of ML algorithms.
At Dicecamp, KNIME L2 certified instructors teach complete KNIME analytics program, taking you through basics of Data Science to advanced use cases such as anomaly detection, fraud detection, and social media clustering.
Visit detailed course outline for no-code Data Science at Dicecamp.
How no-code Data Science serves organizations?
No-code Data Science primarily serves those organizations who lack key resources such as data science professionals, technology infrastructure, and time in developing a mature data analytics ecosystem. Further, no-code data science helps business executives gain an intuitive view of Data Science, leading to quality data governance.
Learn more about the right way of leveraging Data Science in business.
The idea of no-code data science is also backed by credible market analysis. According to Gartner, citizen data scientists can ‘accelerate’ organizations into AI and ML without spending huge costs and efforts in complex implementation. Equipped with the right tools, non-specialists as well as professional data scientists can perform intricate diagnostic analysis as well as create models that leverage predictive or prescriptive analytics, using simplified technology platforms (view Data Science tasks in 2020).
Another Gartner Trend Insight report on low code technologies highlights an interesting fact on the utility of no-code data science. According to the report, within an organization, the majority of employees constitute business technologists who have an average technology grasp. This chunk of the audience (avg. 41% among all employees) is already using low-code and no-code technology solutions for data and analytics.
Does no-code Data Science pose a threat to the job security of Data Scientists?
Although no-code Data Science empowers non-technical persons to gracefully take out advanced analytics, it doesn’t mean it’s the end for professional Data Scientists.
As we’ve stated in section 2, how the no-code platforms come with advanced functionalities such as code editors that let’s data scientists write code to customize workflows. This brings more flexibility for professional data scientists who can use their coding skills and data science expertise in building competing data science solutions.
Hence, no-code platforms truly facilitate a data scientist who can use their programming knowledge to craft immensely valuable and innovative data models that bring a powerful difference in their work.
Apart from the above, no-code platforms salvage data scientists from repetitive coding; building or revamping code for every new project. With no-code tools, data scientists can focus on the design of analytics pipeline making their work more meaningful and interesting.
Another exclusive benefit of no-code platforms for professional data scientists is the use of subject expertise to create breakthrough data analytics environments for no-coders; such as with the open source no-code data science platform KNIME. Data scientists can opt for developing their commercial proprietary tools, and supply service layers and support at KNIME.
Concluding: Data Science is for everyone with no-code platforms
From credible market research and subjective analysis presented above, it’s certain that the reins of data science is safe at the hands of no-code platforms. With no-code data science it becomes extremely simple to create data models without writing a single line of code. This not only empowers citizen data scientists, but brings a lot more opportunities for professional data scientists as well. It’s with the simplicity of technology that organizations now perform intricate diagnostic analysis as well as predictive or prescriptive analytics without investing plentiful resources.
Visit Dicecamp no-code Data Science remote, self paced training.
Watch the insightful demo-session on no-code Data Science using KNIME at Dicecamp
youtube
#dicecamp#datascience#data scientist#data science course#knime#knimecourse#nocodedatascience#nocodedatasciencecourse#machinelearning#Youtube
0 notes
Text
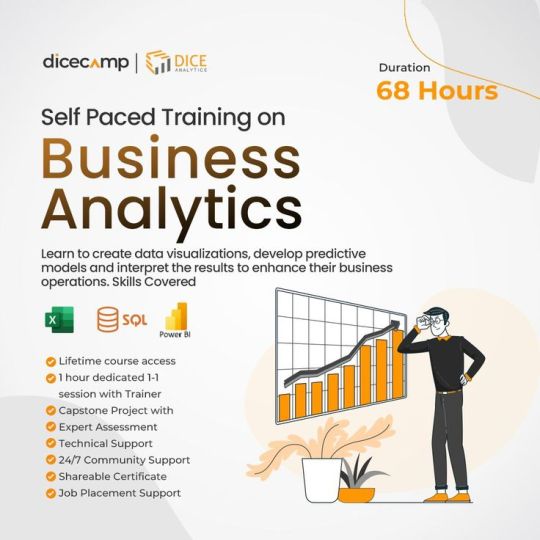
Business Analytics Course
Master Excel, SQL, and Power BI seamlessly in one comprehensive program, propelling yourself into the world of business analysis. Gain invaluable insights with self-paced modules and enhance your skills with a 1-hour live session led by industry expert. Embrace data-driven decision-making, and become a proficient Business Analyst with our dynamic training approach.
Enroll Now : https://dicecamp.com/business-analytics-course
#dicecamp#businessanalyticsapplications#businessanalytics#businessanalyst#businessanalyticscourse#businessanalyticsselfpacedcourse#businessanalyticsonlinecourse#selfpacedcourse
0 notes
Text
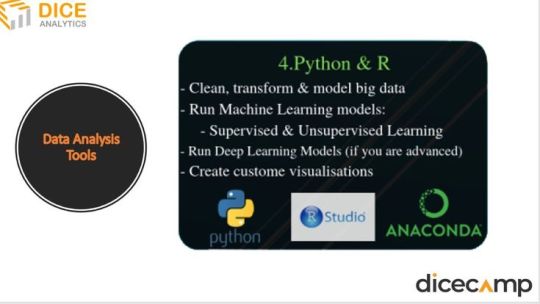
Data Analysis Tools : Python & R - Dicecamp
Python & R reign supreme in data analysis. Python (general-purpose) excels in data manipulation and integration with other tools, while R (statistics-focused) boasts powerful statistical functions and visualizations. Choose Python for versatility, R for deep statistics. Explore Dicecamp's Python for Data Science path to launch your journey!
Link : https://dicecamp.com/python-course
#dicecamp#pythoncourse#python#pythononlinecourse#pythonselfpacedcourse#datascience#data scientist#data science course#selfpacedcourse
0 notes
Text
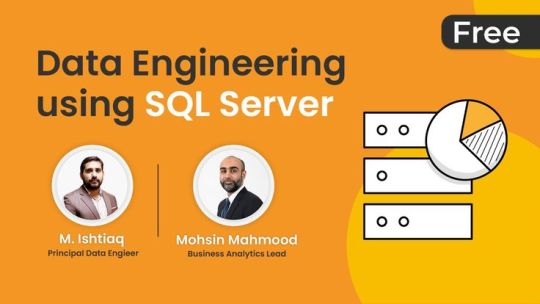
Data Engineering Using SQL Server Free Course
Learn SSIS, SSAS, SSRS for data modeling, visualization, and advanced analytics in this Course and Get Certificate.
Link : https://dicecamp.com/data-engineering-using-sql-server-
#dicecamp#datascience#data scientist#dataengineercourse#dataengineering#dataengineer#dataengineeringfreecourse#freecourse
0 notes
Text
Creative AI: Transform Your Ideas Into Reality with Zero Talents
Ideas are no further bound by talents and skills. You can easily see them realized with 'Creative AI’ that can make any digital product– music, art, web apps or anything you name it.
Unleashing Creativity: How Generative AI is Revolutionizing Idea Realization
Ideas, once confined to the minds of their creators, are now breaking free. Thanks to the advent of generative AI, turning imagination into reality is no longer a privilege reserved for the skilled few. This transformative technology empowers anyone to bring their concepts to life, regardless of artistic or technical prowess.
The Power of Generative AI
Generative AI, often referred to as creative AI, is a game-changer. By providing a bridge between abstract thought and tangible output, it democratizes the creative process. With simple text prompts, users can guide AI models to generate music, art, code, and more. This unprecedented ability to rapidly iterate and experiment has opened up a world of possibilities for innovators and dreamers alike.
Imagine:
A musician without instrumental skills composing a full symphony.
A writer struggling with writer's block generating compelling stories.
A designer crafting stunning visuals without graphic design experience.
Generative AI makes these scenarios a reality.
From Concept to Creation
The journey from idea to product has been significantly shortened. No longer do brilliant ideas languish due to a lack of resources or expertise. With generative AI, individuals can quickly prototype and refine their concepts. It's like having a team of talented collaborators at your fingertips.
Key benefits of using generative AI:
Rapid prototyping: Quickly visualize and test ideas.
Overcoming skill barriers: Create without specialized training.
Enhanced creativity: Explore new possibilities and push boundaries.
Increased efficiency: Automate repetitive tasks.
A New Era of Innovation
Generative AI has the potential to spark a new wave of innovation. As the technology continues to evolve, we can expect even more groundbreaking applications. From revolutionizing industries to solving global challenges, the possibilities are endless.
Conclusion
Generative AI is undeniably a powerful tool for turning dreams into reality. By breaking down barriers and accelerating the creative process, it empowers individuals to bring their visions to life. As this technology matures, we can anticipate a future where innovation is driven by a diverse range of creators, unconstrained by traditional limitations.
Are you ready to unlock your creative potential with generative AI?
Dicecamp Generative AI Course
Weekend, 8-week course
Live on Zoom
Skills Covered: Chatbots, RAGs, Vector Databases, Agents
Tools Covered: Python, Langchain, OpenAI, Ollama
Link : https://dicecamp.com/generative-ai-course
0 notes