#quantitative data collection
Explore tagged Tumblr posts
Text
The Power of Precision: A Deep Dive into Quantitative Data Research
In the dynamic world of research and decision-making, data is the bedrock upon which sound strategies are built. Among the various research methodologies, quantitative data research stands tall as a powerful and precise tool. At Philomath Research, we understand the significance of quantitative research and its unparalleled ability to uncover insights, drive informed decisions, and shape the future. In this deep-dive exploration, we'll delve into the world of quantitative data research, understanding its methodologies, applications, and the transformative impact it has on businesses and industries.
Chapter 1: Understanding Quantitative Data Research
Quantitative data research is a systematic approach to collecting, analyzing, and interpreting numerical data. It involves structured data collection methods such as surveys, questionnaires, experiments, and observations. This methodology ensures that data is precise, measurable, and statistically significant. The quantitative approach allows researchers to answer questions with numbers, providing a foundation for data-driven decision-making.
Chapter 2: The Methodologies of Quantitative Research
Quantitative research methodologies come in various forms, each tailored to the specific research objectives. We'll explore the most common methods:
Surveys and Questionnaires: Surveys are a staple of quantitative research, enabling researchers to collect data from a large sample size. We'll delve into best practices for designing effective surveys and questionnaires.
Experiments: Controlled experiments allow researchers to isolate variables and establish causal relationships. We'll discuss how experiments are designed and executed.
Observational Research: Observational studies involve systematic observations of subjects in their natural settings. We'll explore the advantages and challenges of this method.
Chapter 3: The Power of Precision in Data Collection
The heart of quantitative research lies in its precision in data collection. We'll explore:
Structured Data Collection: Quantitative research employs structured data collection tools that ensure uniformity and consistency in responses.
Large Sample Sizes: The ability to collect data from large sample sizes provides statistical power and reliability.
Quantifiable Data: Numerical data is highly quantifiable and lends itself to statistical analysis.
Statistical Significance: Quantitative research allows researchers to determine the statistical significance of findings, providing confidence in the results.
Chapter 4: Data Analysis in Quantitative Research
In this chapter, we'll dive into the data analysis phase of quantitative research:
Descriptive Statistics: Descriptive statistics provide an overview of the data, including measures of central tendency and dispersion.
Inferential Statistics: Inferential statistics allow researchers to draw conclusions about a population based on a sample. We'll explore hypothesis testing, confidence intervals, and more.
Statistical Software: The role of statistical software in data analysis, including popular tools like SPSS, SAS, and R.
Chapter 5: The Real-World Applications of Quantitative Research
Quantitative research finds application in a wide range of fields and industries. We'll showcase some real-world examples:
Market Research: How businesses use quantitative research to understand consumer behavior, preferences, and market trends.
Healthcare: The role of quantitative research in clinical trials, patient outcomes analysis, and healthcare policy.
Education: How educational institutions leverage quantitative research to improve teaching methods and student performance.
Finance: Quantitative research in financial modeling, risk assessment, and investment strategies.
Chapter 6: The Transformative Impact of Quantitative Research
Quantitative research has the power to transform industries and shape strategies. We'll discuss:
Evidence-Based Decision-Making: How quantitative research provides the evidence needed for informed decisions.
Competitive Advantage: How businesses gain a competitive edge by using quantitative insights to optimize operations and customer experiences.
Innovation: The role of quantitative research in driving innovation, product development, and process improvements.
Chapter 7: Challenges and Ethical Considerations
No research methodology is without its challenges and ethical considerations. We'll explore:
Sampling Bias: The potential for bias in sampling methods and how researchers mitigate it.
Privacy and Data Security: The importance of safeguarding participant data and ensuring compliance with data protection regulations.
Ethical Conduct: The ethical responsibilities of researchers in quantitative studies, including informed consent and transparency.
Chapter 8: The Future of Quantitative Data Research
As technology advances and the world becomes more data-centric, we'll look ahead to the future of quantitative research:
Big Data and AI: The convergence of quantitative research with big data analytics and artificial intelligence.
Multimodal Research: The potential for combining quantitative and qualitative research methods for deeper insights.
Globalization: How quantitative research is adapting to the challenges and opportunities of a globalized world.
Conclusion: Harnessing the Power of Precision
Quantitative data research is not merely a methodology; it's a strategic imperative in today's data-driven world. At Philomath Research, we embrace the power of precision in data collection and analysis. We understand that the insights derived from quantitative research have the potential to transform businesses, industries, and societies.
In this deep dive into quantitative data research, we've explored its methodologies, applications, and transformative impact. It's a journey that leads to a deeper understanding of the world around us, enabling us to make informed decisions and shape a brighter future.
To learn more about how Philomath Research leverages the power of precision in quantitative data research, please visit www.philomathresearch.com. Together, we can uncover insights that drive success and innovation.
#quantitative data collection#quantitative surveys#quantitative market research services#quantitative market research companies#quantitative survey research#research services#quantitative data collection methods
0 notes
Text
Sometimes when I'm bored I respond to survey requests that I got from like online retailers and such.
Here I am filling out the super standard demographics section and then it asks what my highest level of education is and the options are either primary or secondary, no option to select any level of higher education... so, I see your survey will have realistic results, company. No incorrect demographic information at all, everyone who took the survey is only educated to secondary level... clearly...
6 notes
·
View notes
Text
Precision Insights: Expert Quantitative Market Research Services
Our Quantitative Market Research Services help you quickly gather insights from our panellists and understand the changing consumer behaviour. Using our comprehensive services, we find the answers to the most of your questions! Follow this link to know more https://insighttellers.com/services/quantitative-research-market
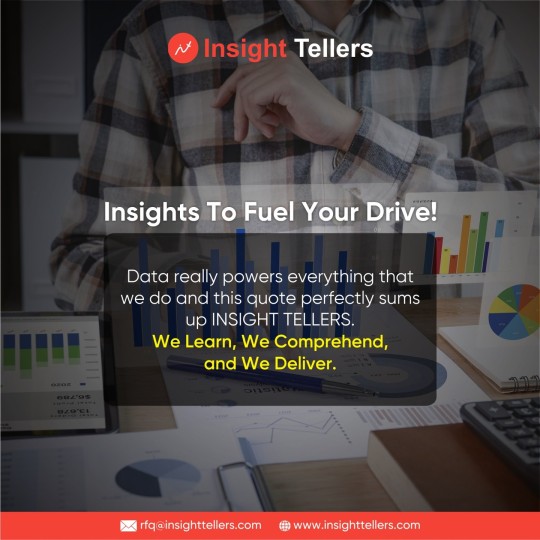
#Quantitative Market Research Services#Qualitative Research#Translation#Survey Programming#Data Collection & Analysis#Secondary Research#Panel Aggregation#Contracted Work
2 notes
·
View notes
Text
Boost Customer Loyalty with Data-Driven Personalization
Data can help businesses understand their customers, personalize experiences, and build lasting relationships, which can lead to increased customer loyalty. Invest in your customers. Invest in Data.
#quantitative research#data analysis#marketresearch#businessgrowth#insightsopinion#data collection solutions#data processing services
0 notes
Text
5 Methods of Data Collection for Quantitative Research
Discover five powerful techniques for gathering quantitative data in research, essential for uncovering trends, patterns, and correlations. Explore proven methodologies that empower researchers to collect and analyze data effectively.
#Quantitative research methods#Data collection techniques#Survey design#Statistical analysis#Quantitative data analysis#Research methodology#Data gathering strategies#Quantitative research tools#Sampling methods#Statistical sampling#Questionnaire design#Data collection process#Quantitative data interpretation#Research survey techniques#Data analysis software#Experimental design#Descriptive statistics#Inferential statistics#Population sampling#Data validation methods#Structured interviews#Online surveys#Observation techniques#Quantitative data reliability#Research instrument design#Data visualization techniques#Statistical significance#Data coding procedures#Cross-sectional studies#Longitudinal studies
1 note
·
View note
Text
However, UV/visible spectroscopy is used widely in detectors for the analysis of liquid and gas samples as it can detect very low concentrations of analyte and provides quantitative data.
"Chemistry" 2e - Blackman, A., Bottle, S., Schmid, S., Mocerino, M., Wille, U.
#book quote#chemistry#nonfiction#textbook#spectroscopy#uv#ultraviolet#visible spectroscopy#analysis#quantitative#quantitative analysis#data collection
0 notes
Text
#Top 5 Market Research Companies in Malaysia#Market Research Companies in Malaysia#qualitative and quantitative research#Data Collection Services in Malaysia
0 notes
Text
transphobes & conservatives throw around the "80% of trans children desist" statistic, and it comes from the average of a few quantitative studies that did produce those numbers
and they're relying on people to not have the knowledge to actually dig into these things, analyze the statistics, and question the methodologies in a scientific way
if you're curious at all how to debate these claims when people bring it up, i've summarized a meta-review below:
the biggest, most glaring issue of these studies is sample size and participant selection -- Baer Karrington, MD opened up these papers and found that one of the 4 studies (yes, the 80% number comes from the average of 4 studies, as of 2022) had identified nearly 300 eligible participants and then selected 132 of them, and we don't know why.
it's normal to screen out participants for psych & medical studies for any number of reasons, but you have to be completely transparent about why you're excluding them - and the researchers never told us. we can't know if you deliberately or subconsciously chose people who would confirm your hypothesis, which calls into question the data you collected.
another study was unable to contact 24 of the original participants out of 77, and then automatically classified the uncontactable participants as desisters. this isn't good science. i'm hesitant to even call it science.
the third study didn't list how many participants were included, but the assumption is that it was 10 people.
the fourth study had 25.
and across all of this research into desistance, including dozens of non-quantitative studies, case studies, and interviews, the researchers give us different definitions of desistance
(wouldn't the most important part of a cohesive argument against childhood gender affirming care be a mutually understood definition of desistance)
included under the definitions of desisters are:
adults who decide to stop HRT for any reason. this includes children who underwent the puberty of their choice and then ceased medical intervention in adulthood, without ascertaining if the reason was that they were satisfied with their body post-treatment, or if they had or intended to de-transition back to a body reflecting their birth sex
people who may have initially begun as binary transgender but settled on a non-binary or gender expansive identity. one former trans woman is identified in the research as now going by they/them and ceased HRT, and the studies do not reach a consensus on whether non-binary identities are included in the transgender non-desisting category, or if it is considered desistance
children for whom medical transitioning is stopped at any point - with obvious difficulties around determining whether was initiated by parents or truly the child's wishes
people who never received medical intervention and no longer experience gender dysphoria in adulthood, regardless of whether they have socially transitioned, changed their name, or engaged in non-medical affirmations of identity
children who decide not to medically transition. yes, that's it. they have desisted by virtue of not seeking medical intervention for the way that they conceive of their identity
and the issue with qualitative studies is that doctors are specifically reporting on people who have come to them seeking their help, and while their stories are important, and desistance does happen, you cannot draw actionable statistics from a self-selecting pool of people
the bottom line is that we have a double digit number of subjects across four questionable quantitative studies that gives us the repeatedly quoted 80%, and this is supposed to predict how several million children around the world will operate - and that's not how science works
663 notes
·
View notes
Photo
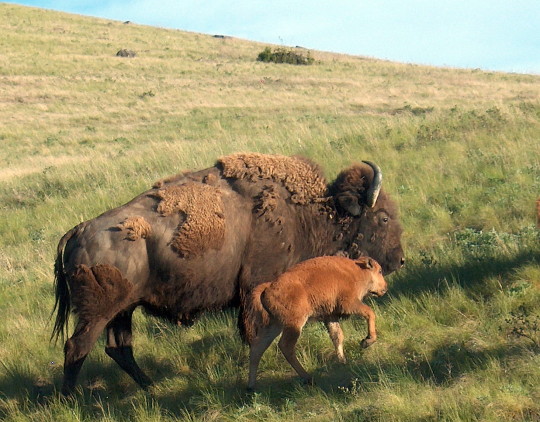
Kansas Research Shows Reintroducing Bison on Tallgrass Prairie Doubles Plant Diversity
Findings from decades of data also point to resistance to extreme drought.
Decades of research led by scientists at Kansas State University offered evidence reintroducing bison to roam the tallgrass prairie gradually doubled plant diversity and improved resilience to extreme drought.
Gains documented in the journal Proceedings of the National Academy of Science were among the largest recorded globally in terms of species richness on grazing grasslands. The research involved more than 30 years of data collected at the Konza Prairie Biological Station near Manhattan.
Zak Ratajczak, lead researcher and assistant professor of biology at Kansas State, said removal of nearly all bison from the prairie occurred before establishment of quantitative records. That meant effects of removing the dominant grazer were largely unknown, he said.
“Bison were an integral part of North American grasslands before they were abruptly removed from over 99% of the Great Plains,” Ratajczak said...
Read more: https://www.agriculture.com/news/business/kansas-research-shows-reintroducing-bison-on-tallgrass-prairie-doubles-plant-diversity
#bison#prairie#ecology#prairie ecology#grasslands#nature#science#ungulate#grazing#animals#north america#biodiversity#environment#conservation#restoration ecology#range ecology
1K notes
·
View notes
Text





the biophysics students
examining life on all possible scales
reading a dozen papers on just one molecule
marveling at the work of early x-ray crystallographers
untangling a complex NMR spectrum as if it's a logic puzzle
deducing a chemical structure one piece at a time
hours spent perfecting a computational model
grounding your work in quantitative theory
a disciplined approach to problem-solving
the satisfaction of clean mass spec data
becoming comfortable with complicated equations
chilly air in the temperature-controlled equipment room
understanding the physics that grounds all larger phenomena
pages of instrument printouts cluttering your desk
seeing the contributions of a single atom to a wider system
safety goggles sized perfectly to fit your face
diagraming electron spins and magnetic fields
loyalty to your favorite graphing software
savoring a cup of coffee while you wait to collect your data
dreaming of the contributions your work will make one day
late nights at the lab computer
doodling protein ribbon diagrams on your homework
#this post is dedicated to my time in a protein nmr lab#student aesthetic#studying aesthetic#studyblr#studyspo#dark academia#dark acadamia aesthetic#academia#academia aesthetic#light academia#light academia aesthetic
55 notes
·
View notes
Note
*waves shyly* Hello!! First off, I absolutely adore all of your stats and get excited when you post new ones -- thank you so much for all that you do!
Secondly, a friend and I have been discussing fandom longevity lately, and I wondered if you have thoughts? Subjectively, it seems to us that new fandoms tend to have more quick bursts of fandom activity when a new season/movie/book/etc comes out that fades quickly with time, whereas older established fandoms have more staying power. I'm curious if you have any insight about whether this is objectively true in most cases or not, and as to whether or not the type of canon source material matters (eg show-based fandoms vs book-based fandoms). I hope you're having a great day <3
Hi there, and thanks! :D This is a great question, and one I have been having a bunch of conversations about lately.
I share this subjective experience -- it sure seems like the attention span of fans and lifespan of fandoms is shorter than it used to be, when I think of how quickly people stop talking about a bunch of newer movies and TV shows these days. And then I see some of the older fandoms like Harry Potter still producing a ton of new fanworks, and I think, "Wow, maybe new fandoms just don't have the staying power of older ones." At the same time, I also question how objectively true/simple that story is for a few reasons, including:
Memory bias: When we look back on the past, the fandoms we remember most are usually the ones that lasted a long time. So our estimates of past fandom longevity may be overly generous.
Changes to fandom size: Maybe any changes to fandom lifespan are mostly due to some other change, like fandom size... Attention is more splintered these days than it used to be across more streaming services/etc, and I think there are more, smaller fandoms than there used to be. Maybe if a fandom doesn't get really huge, it's just not likely to last that long.
For TV fandoms -- changes to canon release schedule: most TV shows used to have seasons that lasted most of the year, so they had a lot more reason to stay in the public mind longer. Now many seasons are shorter and sometimes drop all at once. Perhaps if we compared popular TV procedurals with 22 episodes/season from now vs. ~a decade ago, we'd see similar patterns of fandom activity?
I've been thinking about ways to try to gather quantitative data about the changes, and testing out a few methods. A few ideas I've had:
Look at the Tumblr official lists of top fandoms and see whether the top fandoms tend to leave the top 20 rankings faster now than they used to. (The Tumblr rankings go all the way back to 2013 on a yearly basis, at least -- I'm not sure how long they've been releasing the weekly lists; those may have started later.)
Look at AO3 fandom activity after new canon infusions - how quickly does activity drop off after a new movie/book/video game release, or after a TV season ends? How has the rate of activity dropoff changed over the years? (And how much of that seems to be explained by other factors, like fandom size?)
See how quickly AO3 authors/creators tend to migrate to new fandoms, and how that's changed over time - many authors tend to be active in multiple fandoms, so we'd have to define what it means to migrate to a new fandom, but I think we could do so in a way that would allow us to look for changes.
Look at Tumblr, Twitter/X, and/or Reddit activlty after new canon infusions - same as AO3, but on a platform where people are posting shorter content and there's more of a discussion. (This data would be harder to collect, though.)
I'd love to also hear other ideas. I think I'm going to need some volunteers to help gather data if I do any of the above, though... Readers, if you'd be interested in helping to gather data for an hour or more to help investigate this question, please reply/DM and let me know! And/or join the new fandom-data-projects community.
Also if any readers know of anyone else who has looked into this/similar questions, I'd love to hear about it!
#fandom lifespan#call for volunteers#I'll also post more details later#but it would involve doing AO3 searches or other searches and copying numbers into a spreadsheet#questions for the tumblmind#asks#toasty replies#fandom stats#toastystats#50
58 notes
·
View notes
Text
Understanding Regression Analysis in Quantitative Research: Unravelling the Complex Threads of Data
Quantitative research forms the backbone of modern scientific inquiry and business decision-making. In the vast landscape of statistical techniques, regression analysis stands out as a powerful tool that allows researchers to model complex relationships among variables. In this comprehensive guide, we will unravel the intricacies of regression analysis in quantitative research, shedding light on its principles, applications, and significance in shaping data-driven decisions.
The Foundation of Regression Analysis
Regression analysis, at its core, aims to understand the relationship between a dependent variable and one or more independent variables. It goes beyond simple correlations, delving into the nuances of cause-and-effect relationships within a dataset. Whether in the realms of economics, healthcare, marketing, or social sciences, regression analysis provides a systematic framework to analyze and interpret data.
Types of Regression Models
1. Linear Regression:
Linear regression is the most basic form, exploring linear relationships between variables. It assumes a straight-line relationship between the variables and is widely used for prediction and forecasting.
2. Multiple Regression:
Multiple regression extends linear regression by considering multiple independent variables. It enables researchers to analyze the impact of several predictors on the dependent variable simultaneously, providing a more comprehensive understanding of the relationships.
3. Logistic Regression:
Logistic regression is employed when the dependent variable is categorical (binary or ordinal) rather than continuous. It's frequently used in predicting outcomes like yes/no responses or probabilities of an event occurring.
4. Polynomial Regression:
Polynomial regression deals with nonlinear relationships between variables. It fits a polynomial equation to the data, capturing more complex patterns that cannot be addressed by linear regression models.
Steps in Regression Analysis
1. Defining the Research Question:
The first step involves clearly defining the research question and identifying the variables of interest. Understanding the context is crucial for selecting the appropriate regression model.
2. Data Collection and Preparation:
Data collection involves gathering relevant data for the variables identified. The dataset must be cleaned, outliers removed, and missing values addressed to ensure the accuracy and reliability of the analysis.
3. Model Specification:
Choosing the right regression model is essential. Based on the type of variables and the research question, researchers select between linear, multiple, logistic, or other specialized regression models.
4. Parameter Estimation:
Regression analysis calculates the coefficients of the model, indicating the strength and direction of the relationships between variables. Various techniques, like the method of least squares, are employed to estimate these parameters.
5. Model Evaluation:
Evaluating the regression model involves assessing its goodness of fit, checking assumptions, and validating its predictive power. Techniques such as R-squared, residuals analysis, and cross-validation play a crucial role in this step.
6. Interpretation and Inference:
Interpreting the results is the final step. Researchers analyze the coefficients to understand the relationships and draw meaningful conclusions. Inferential statistics help in making broader generalizations about the population based on the sample data.
Applications of Regression Analysis
1. Economics and Finance:
Regression models are used to analyze economic indicators, stock prices, and market trends, enabling investors and policymakers to make informed decisions.
2. Healthcare and Medicine:
Regression analysis helps in predicting disease outcomes, analyzing patient data, and understanding the effectiveness of treatments, aiding healthcare professionals in optimizing patient care.
3. Marketing and Consumer Behavior:
Marketers use regression analysis to study consumer preferences, buying patterns, and the impact of advertising, enabling companies to tailor their strategies for maximum impact.
4. Social Sciences:
Sociologists and psychologists employ regression analysis to study various social phenomena, including crime rates, education outcomes, and behavioral patterns, leading to valuable societal insights.
The Significance of Regression Analysis
Regression analysis holds immense significance in the realm of quantitative research for several reasons:
1. Causality Inference:
Regression analysis enables researchers to infer causal relationships between variables, providing deeper insights into the underlying mechanisms at play.
2. Predictive Power:
Regression models can make accurate predictions based on historical data, allowing businesses and researchers to forecast trends and plan accordingly.
3. Data-driven Decision Making:
In an era where data-driven decisions are paramount, regression analysis provides a robust framework for businesses and policymakers to base their strategies on empirical evidence.
4. Continuous Improvement:
By analyzing regression results, businesses can identify areas for improvement, optimize processes, and enhance the quality of products and services, leading to sustainable growth.
Conclusion
In the intricate tapestry of quantitative research, regression analysis stands tall as a beacon of insight and understanding. Its ability to unravel complex relationships within datasets empowers researchers, businesses, and policymakers to make informed decisions that shape our world.
At Philomath Research, we recognize the transformative power of regression analysis. Our dedicated team of experts utilizes advanced statistical techniques, including regression analysis, to deliver unparalleled insights to our clients. By embracing the nuances of quantitative research, we pave the way for innovation, growth, and progress.
Embrace the power of regression analysis, and unlock the secrets hidden within your data. Visit Philomath Research to explore our comprehensive suite of quantitative research services and embark on a journey towards data-driven excellence. Together, let's illuminate the path to a future shaped by knowledge, precision, and informed decision-making.
#quantitative data collection#Quantitative Research Solutions#Global Research Solutions#Quantitative Solutions and Services#Quantitative market research companies#quantitative research services#Best quantitative research firm usa
0 notes
Text
Fine.
MY Naruto OC is a genjutsu master femme fatale missing-nin whose raison d'etre is to investigate the inner workings of the grass-roots, organised criminal networks that support missing-nin.
She is 52 and she has no name, no bank account, and six different illusory personas she uses as she deems necessary (some of them are real people who already exist) (some of them are real ninja who already exist).
She considers herself a criminologist and collects quantitative data about client-nin interactions, including negotiations, prices for services, murders and double-crosses, and the like. She publishes them and sometimes missing-nin just... discover they are in her case studies.
She has been stalking Kakuzu, her greatest outlier, for 35 years. He is an outlier in several ways, usually because he is a legacy object in the whole missing-nin network. She is unwell about him. Accordingly, 13 year old Itachi joining the Akatsuki—with his genjutsu proof eyes—almost ended her whole career.
#tozette.txt#naruto oc#let's call her red. but she really has no name. you can go find her paperwork at her old village but it's mostly illusory.
239 notes
·
View notes
Text
Qualitative Research for Comprehensive Data Insights
Numerous academic fields, including social science, market research, and psychology, heavily rely on qualitative analysis. Qualitative analysis primary purpose is to describe the subject in detail so that researchers can better understand its intricacies and nuances. When context and subjective information are essential for undertaking social, cultural, or psychological research, this method is especially helpful. Follow this link to know more https://insighttellers.com/blog/qualitative-research-for-comprehensive-data-insights
#Market Research agency#top market research company#qualitative market research company#quantitative research company#data collection company#top market research companies#quantitative marketing research#desk research#secondary research
0 notes
Text
Poor people pay higher time tax

Doubtless you’ve heard that “we all get the same 24 hours in the day.” Of course it’s not true: rich people and poor people experience very different demands on their time. The richer you are, the more your time is your own — not only are many systems arranged with your convenience in mind, but you also command the social power to do something about systems that abuse your time.
If you’d like an essay-formatted version of this post to read or share, here’s a link to it on pluralistic.net, my surveillance-free, ad-free, tracker-free blog:
https://pluralistic.net/2023/02/10/my-time/#like-water-down-the-drain
For example: if you live in most American cities, public transit is slow, infrequent and overcrowded. Without a car, you lose hours every day to a commute spent standing on a lurching bus. And while a private car can substantially shorted that commute, people who can afford taxis or Ubers get even more time every day.
There’s a thick anthropological literature on the ways that cash-poverty translates into #TimePoverty. In David Graeber’s must-read essay “The Utopia of Rules,” he nails the way that capitalist societies generate Soviet-style bureaucracies, especially for poor people. Means-testing for benefits means that poor people spend endless hours filling in forms, waiting on hold, and lining up to see caseworkers to prove that they are among the “deserving poor” — not “mooches” who are defrauding the system:
https://memex.craphound.com/2015/02/02/david-graebers-the-utopia-of-rules-on-technology-stupidity-and-the-secret-joys-of-bureaucracy/
The social privilege gradient is also a time gradient: if you can afford a plane ticket, you can travel quickly across the country rather than losing days to the Greyhound or a road-trip. But if you’re even richer, you can pay for TSA Precheck and cut your airport security time from an hour to minutes. Go further up the privilege gradient and you’ll acquire airline status, shaving another hour off the check-in process.
This qualitative account of time poverty is well-developed, but it’s lacked a good, detailed quantitative counterpart, and our society often discounts qualitative work as mere anecdote and insists on having every story converted to numbers before it is taken seriously.
In “Examining inequality in the time cost of waiting,” published this month in Nature Human Behavior, public affairs researchers Steve Holt (SUNY) and Katie Vinopal (Ohio State) analyze data from the American Time Use Survey (AUTS) to produce a detailed, vibrant quantitative backstop to the qualitative narrative about time poverty:
https://www.nature.com/articles/s41562-023-01524-w
(The paper is paywalled, but the authors made a mostly final preprint available)
https://osf.io/preprints/socarxiv/jbk3x/download
The AUTS “collects retrospective time diary data from a nationally representative subsample drawn from respondents to the Census Bureau’s Community Population Survey (CPS) each year.” These time-diary entries are sliced up in 15-minute chunks.
Here’s what they found: first, there are categories of basic services where high-income people avoid waiting altogether, and where low-income people experience substantial waits. A person from a low-income household “an hour more waiting for the same set of services than people from high-income household.” That’s 73 hours/year.
Some of that gap (5%) is attributable to proximity. Richer people don’t have to go as far to access the same services as poorer people. Travel itself accounts for 2% more — poorer people wait longer for buses and have otherwise worse travel options.
A larger determinant of the gap (25%) is working flexibility. Poor people work jobs where they have less freedom to take time off to receive services, so they are forced to take appointments during peak hours.
Specific categories show more stark difference. If a poor person and a wealthy person go to the doctor’s on the same day, the poor person waits 46.28m to receive care, while the wealthy person waits 28.75m. The underlying dynamic here isn’t hard to understand. Medical practices that serve rich people have more staff.
The same dynamic plays out in grocery stores: poor people wait an average of 24m waiting every time they go shopping. For rich people, it’s 15m. Poor people don’t just wait in longer lines — they also have to wait for understaffed stores to unlock the cases that basic necessities are locked behind (poor people also travel longer to get to the grocery store — and they travel by slower means).
A member of a poor household with a chronic condition that requires two clinic visits per month loses an additional five hours/year to waiting rooms when compared to a wealthy person. As the authors point out, this also translates to delayed care, missed appointments, and exacerbated health conditions. Time poverty leads to health poverty.
All of this is worse for people of color: “Low-income White and Black Americans are both more likely to wait when seeking services than their wealthier same-race peer” but “wealthier White people face an average wait time of 28 minutes while wealthier Black people face a 54 minute average wait time…wealthier Black people do not receive the same time-saving attention from service providers that wealthier non-Black people receive” (there’s a smaller gap for Latino people, and no observed gap for Asian Americans.)
The gender gap is more complicated: “Low-income women are 3 percentage points more likely than low-income men and high-income women are 6 percentage points more likely than high-income men to use common services” — it gets even worse for low-income mothers, who take on the time-burdens associated with their kids’ need to access services.
Surprisingly, men actually end up waiting longer than women to access services: “low-income men spend about 6 more minutes than low-income women waiting for service…high-income men spend about 12 more minutes waiting for services than high-income women.”
Given the important role that scheduling flexibility plays in the time gap, the authors propose that interventions like subsidized day-care and afterschool programming could help parents access services at off-peak hours. They also echo Graeber’s call for reduced paperwork burdens for receiving benefits and accessing public services.
They recommend changes to labor law to protect the right of low-waged workers to receive services during off-peak hours, in the manner of their high-earning peers (they reference research that shows that this also improves worker productivity and is thus a benefit to employers as well as workers).
Finally, they come to the obvious point: making people less cash-poor will alleviate their time-poverty. Higher minimum wages, larger earned income tax credits, investments in low-income neighborhoods and better public transit will all give poor people more time and more money with which to command better services.
This week (Feb 13–17), I’ll be in Australia, touring my book Chokepoint Capitalism with my co-author, Rebecca Giblin. We’re doing a remote event for NZ on Feb 13. Next are Melbourne (Feb 14), Sydney (Feb 15) and Canberra (Feb 16/17). More tickets just released for Sydney!
[Image ID: A waiting room, draped with cobwebs. A skeleton sits in one of the chairs. A digital display board reads 'Now serving 53332.' An ogrish, top-hatted figure standing at a podium, yanking a dollar-sign shaped lever looms into the frame from the right. He holds a clock aloft disdainfully, pinched between the thumb and fingers of one white-gloved hand.]
#pluralistic#scholarship#auts#american time use survey#time use#jenny odell#race#graeber#david graeber#how to do nothing#utopia of rules#inequality#gender#time poverty
803 notes
·
View notes
Text
What Is Market Research: Methods, Types & Examples
Learn about the fundamentals of market research, including various methods, types, and real-life examples. Discover how market research can benefit your business and gain insights into consumer behavior, trends, and preferences.
#Market research#Methods#Types#Examples#Data collection#Surveys#Interviews#Focus groups#Observation#Experimentation#Quantitative research#Qualitative research#Primary research#Secondary research#Online research#Offline research#Demographic analysis#Psychographic analysis#Geographic analysis#Market segmentation#Target market#Consumer behavior#Trends analysis#Competitor analysis#SWOT analysis#PESTLE analysis#Customer satisfaction#Brand perception#Product testing#Concept testing
0 notes