#link leads to the full film available until 26/10!
Explore tagged Tumblr posts
Text
Good Girls Club: A Virginity Odyssey (2023) dir. DImitris Tsakaleas & Lida Vartzioti
#queer short film#queer greek cinema#good girls club: a virginity odyssey#link leads to the full film available until 26/10!
46 notes
·
View notes
Text
GJ and ZZH Updates — September 25-October 1
<<< previous week || all posts || following week >>>
This is part of a weekly series collecting updates from and relating to Gong Jun and Zhang Zhehan.
This post is not wholly comprehensive and is intended as an overview, links provided lead to further details. Dates are in accordance with China Standard Time, the organization is chronological. My own biases on some things are reflected here. Anything I include that is not concretely known is indicated as such, and you’re welcome to do your own research and draw your own conclusions as you see fit. Please let me know if you have any questions, comments, concerns, or additions. :)
[Glossary of names and terms] [Masterlist of my posts about the situation with Zhang Zhehan]
09-25 → Gong Jun posted a douyin of himself. Caption: “Shock! It turned out to be...”
09-26 → Kangshifu posted a photo ad featuring Gong Jun.
→ ELLE magazine posted a trailer for the short film Guardian of Alpine Plants. The caption included, “Gong Jun said: ‘I also feel that the things I love will not be consumed in the process of working hard for them. There will always be problems and even burnout in the process of climbing, but I always feel that there are more areas worth exploring.’ This day is different from every day in the city, Gong Jun described it as feeling like entering another world.”
→ Zhang Zhehan requested to dissolve a company he established in 2019. In the paperwork, it is listed that the company is not under investigation, owed any debts, involved in any lawsuits, or listed as a bad creditor, and it is up to date on its taxes. This is the first news we’ve had about him himself since January!

This directly contradicts Xie Yihua and co.’s claims that Zhang Zhehan’s name is not in the brand’s paperwork because his assets have been frozen, that he is not psychologically able to handle his own business affairs, and/or that he has signed over power of attorney to Xie Yihua. Instagram believers responded by saying that this legal document publicly available on a government website with a signature that actually matches his is fake.
09-27 → ELLE magazine posted nine photos of Gong Jun from Guardian of Alpine Plants.
→ Kuaikan, ELLE magazine (English subbed), and the Shangri-La Alpine Botanical Garden all posted the full Guardian of Alpine Plants short film. Gong Jun’s studio reposted it from Kuaikan with the added caption: “Follow boss @ Gong Jun Simon into Shangri-La, listen to the stories of the guardians of alpine plants, and feel the charm of nature.” Gong Jun reposted it from the Botanical Garden’s account, with the added caption: “Walking in Shangri-La, we are amazed at the majesty of the mountains and the tenacity of the flowers and plants. On this beautiful planet, every tiny life deserves to be respected and guarded.”
This was filmed in early to mid-June in Shangri-La, northeastern Yunnan. It was publicly displayed on a large 3D screen outside the Wuhan Sanlian bookstore, and is scheduled to continue to do so until 10-07.
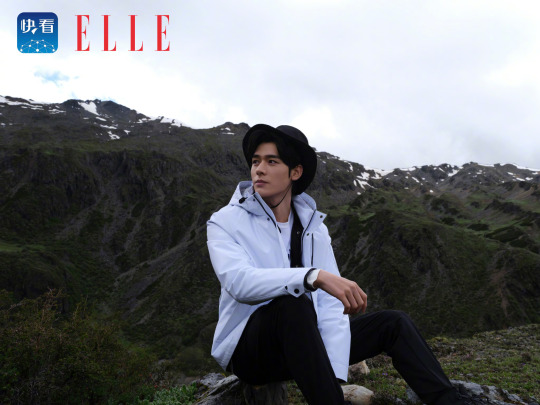
→ ELLE posted an article to their WeChat about the short film. [translation]
09-28 → The Shangri-La Alpine Botanical Garden made a post expressing surprise and thanks for the surge in public support garnered from the short film.
→ Kangshifu posted an illustrated ad featuring Gong Jun.
→ Gong Jun posted a promotional video for Downy to his Xiao Hong Shu.
09-29 → Colgate posted a photo ad featuring Gong Jun.
→ The Instagram posted 10 wildly different photos of “Zhang Zhehan”, several of which include a kitten that we can only hope will be treated better than Kele.
→ The NRTA posted a list of movies and shows that showcare Chinese core values and the “China dream”, which included Gong Jun’s drama The Flaming Heart.
→ Kangshifu posted an illustrated ad featuring Gong Jun.
→ Gong Jun’s studio posted his schedule for October: only drama filming, no public schedule. Caption: “📣Boss @ Gong Jun Simon's October schedule is here! The small studio wishes everyone a happy National Day in advance and a happy holiday~” BGM is eight by IU (feat. Suga) (check the lyrics). [background about the song] Fan Observation: 18:00 timestamp; 10-18 was the date of Zhang Zhehan’s 2020 I Met Me mini-concert, a name that referenced the origin of soulmates from Plato’s Symposium. On 2020-09-29, Gong Jun posted a douyin of his reflection in a mirror with Making a Lover by SS501 as the BGM. [full candy breakdown]
→ Gong Jun’s studio posted the two photos used in the schedule. Caption: “It’s here it’s here”
09-30 → Gong Jun reposted a post by CCTV for Martyrs’ Day with the added caption, “Deeply remembered! Salute to the people's heroes!” His studio did the same, caption: “Don't forget the legacy of the martyrs and pay tribute to the people's heroes!”
→ Kangshifu posted three illustrated ads featuring Gong Jun.
→ 361° posted two photo ads featuring Gong Jun.
10-01 (China National Day, start of Golden Week) → Just after midnight, Gong Jun reposted a post by CCTV for National Day with the added caption, “I wish my dear motherland prosperity ♥” His studio did the same, caption, “The country is peaceful and the people are safe, China is splendid! Celebrate the 73rd anniversary of the founding of New China!“
→ Also just after midnight, the Instagram posted two photos of “Zhang Zhehan” with white hair and a photo of a windmill. Fan Observations: - The white hair is possibly meant to shade the 3D artist YDS, who has art of Zhang Zhehan with white hair as her pfp and has done other such art. Back in the spring, YDS was approached by Sophie and co. who tried to coerce her into helping them, and she has subsequently been repeatedly attacked by them for refusing. The day before this, she posted two new pictures inspired by Zhang Zhehan’s song When The Glaciers Disappear and admonished Xie Yihua and co. for capitalizing off the song without his permission. Update 10-04: YDS was accused by solos masquerading as CPFs to be involved in the making of the Instagram photos. - The windmill looks very similar to the one in the pfp used by 不想匿名, a Zhihu account that some CPFs think might be Zhang Zhehan and/or Gong Jun (it’s the account that posted the frog picture at the end of July). The presumption is that the Instagram is trying to discredit this account.
→ 361° posted a photo ad featuring Gong Jun.
→ Hsu Fu Chi posted a photo ad featuring Gong Jun.
→ Kangshifu posted a photo ad featuring Gong Jun.
→ The brand’s Weibo supertopic was deleted and its “ootd” tag was wiped citing “relevant laws, regulations and policies”. [screenshots] Update 10-04: The supertopic returned but is unavailable.
Additional Reading: → Flora’s daily fan news thread → Douyin recently added a reporting option for video fraud specifically aimed at combatting deepfakes, as they are illegal in China under image use laws. Zhang Sanjian videos, including the 07-17 “fanmeet” videos, have been removed without fail when they’re reported for this.
<<< previous week || all posts || following week >>>
This post was last edited 2022-10-04.
#Slow week but with a couple quite notable things#Zhang Zhehan#Gong Jun#Word of Honor#woh cast#lld timeline
33 notes
·
View notes
Text
Creative Journal
26/10/20- Week 5
Session Themes: We began this weeks session recapping Edward De Bono’s 6 Thinking Hats idea from last week and there were some important points i thought i should note down as a reminder for myself:
“Thinking as a skill”
A “tools approach”
“Software for thinking”
Parallel thinking- Everyone thinking the same thing at the same time
we covered whether originality is important in terms of creativity. Looking back at our discussion in previous lessons regarding the definition of creativity, we saw how overall originality is a key aspect of a lot of peoples interpretation of creativity. We looked at Disney’s Steamboat Willie as a case study. The iconic 1928 short film known by many as the opening animation for numerous Disney films, is actually an idea taken from Buster Keaton’s Steamboat Bill Jr., a silent picture from the same year. Using characters like Mickey mouse, changing the story and the addition of music makes Disney’s take entirely different from the “original”. It’s not that the idea was stolen and copied, but instead used as a point of influence for the animation. Many people today know the name “Steamboat Willie” but would not recognise “Steamboat Bill Jr.”.
We also learned about “Permission Culture” or “Read-Only Culture”, “ a culture in a culture in which creators get to create only with the permission of the powerful, or of creators from the past. ” (Lessig, Free Culture, 2004). I found this idea super interesting as someone that utilises “outsourced” mixed media in my own graphics work, the idea that so many people can produce things with the intent of it being theirs only, so that nobody else can work on it, add to it, or use it in their work. This has lead to the rise in popularity of concepts such as “Creative Commons”, a creative copywrite license that allows free public distribution and usage of materials that may have otherwise been copywrited in the past. Concepts such as these are so important to the industry especially in times like these when going out and capturing images for yourself is less feasible.
We discussed whether utilising somebody else’s work was necessarily considered “stealing”; the difference between outright theft and copying. The examples we looked at were parody and pastiche.
Pastiche
Imitative work with the purpose of replicating artistic methods/stylisation
To celebrate/admire
To pay homage (publicly displayed special honour or respect)
Parody
Imitative work with satiric/ironic intent
To mock
Parody and Pastiche link to the ideas we’ve been learning about in terms of “reverse brainstorming”. In order to replicate something for pastiche/parody you need to truly understand your source materials and similarly “reverse engineer” it.
DSD Activity: This week I’ve started trying to listen to “sleep meditation” podcasts. I’m on sleep medication but still struggle to fall asleep before 3am on a regular evening, usually i listen to fan noises or rain noises but haven’t been able to recently since i broke my phone. So far I’ve been struggling to get into it, it’s a pretty foreign feeling to me trying to tune out of a conversation in order to sleep. They keep talking about how “some people find it difficult to tune out but keep at it” but I’m too interested in whatever they’re speaking about. Sometimes they tell stories, other times they’re more of a guided meditation to help you become more relaxed and think about physical sensations and distractions that may be preventing you from getting to sleep. I find the latter much better for sleeping, but i do enjoy listening to the stories although they keep me awake.
Inspiration: Some interesting media I’ve been looking into this week are old “big tobacco” advertisements. A friend and I were discussing the depopularisation of smoking in recent years, how we always thought it looked “cool” when we were younger but now my 13 year old sister pretends to gag when i come in from a cigarette at my mums place. We were talking about how old “big tobacco propaganda” has been replaced by vape/ecigarette/shisa advertisements. It’s not quite the same in terms of product, obviously cigarettes are much worse than their substitutes, however in terms of corporate propaganda its exactly the same. In years time when we have more research into the long term effects that come with these substitutes we may even laugh in disbelief that these were once being marketed as a safer and healthier alternative. Looking at some of these advertisements, i also really enjoyed the retro art style and shocking nature of some of the messaging, so i’ve also held onto this as inspiration for my own graphics work.

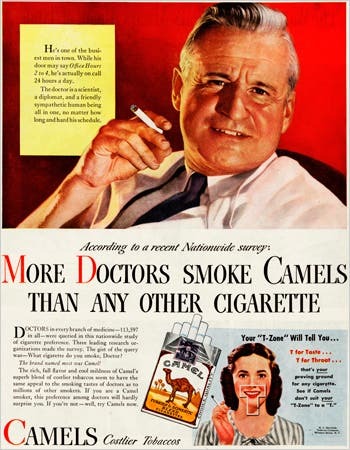
“Make Something Rubbish” Project: For this mini-project, I decided to pitch a film about paint drying. I thought about doing grass/plant growth but in order to actually see any growth on film i think I’d need to do some kind of time lapse which may end up being actually interesting, the only interesting thing that occurs as paint dry's is perhaps a slight change in depth of colour, which is MUCH LESS interesting than plant growth. I started by thinking about traditionally enjoyed aspects of film and how i could counter-cater to the masses. Popular film usually has a range of exciting characteristics whilst this does not. No exciting cast, no music, no interesting camerawork or lighting. This film couldn’t even be enjoyed by people that are into “interpretive art“.
I really thought i had done something clever with this idea, until i did more research and found out that such a film already exists! However, i think my idea is unique to this in terms of intent. Charlie Lyne’s Paint Drying was made with the purpose of making a statement against the BBFC for making film production in this country less accessible to aspiring creators. He made it with the intent of making the BBFC sit and watch an entire 10 hour film of only paint drying, which, whilst making a statement on censorship and economic privilege, was a conversation heavily publicised due to it’s message and comedic nature. This research inspired me in some ways, instead of making a time-lapse, I too had decided to pitch a 10 hour paint drying film. However, the key difference as I’ve stated is intent. Whilst the exact same concept, my film is not trying to make any kind of statement, cause any kind of conversation. It’s simply a film about paint drying. It doesn’t mean anything, doesn’t have any kind of purpose. Just boring paint drying. I can’t find the full film anywhere online but judging from the video on the kickstarter page and the trailer made available on youtube, i believe the actual piece may even contain some music and/or somebody painting the actual wall initially. This would be much too exciting in my film.
youtube
0 notes
Text
Facebook
Transparent Film Festival Home
Posts
MINIATURE CHESS Phil Cheney New York, NY Reviewed Mon. Aug. 31
Prisoners pass the hours playing chess in order to cope with their inevitable fate.
Aug 31, 2020, 9:44 AM
INFATUATION Nace DeSanders New City, NY Reviewed Sun. Aug. 30
A man is obsessed with white women... until...
Aug 30, 2020, 6:58 PM Click for video:
Clips from Phosphene, In The Zebra Pavillion, Knives and Peaches, Buzzing 2C, The Artist Formerly Known As Lucifer, We're At War Clips from Phosphene, In The Zebra Pavillion, Knives and Peaches, Buzzing 2C, The Artist Formerly Known As Lucifer, We're At War
Aug 29, 2020, 12:11 AM
SUMMER ATTIC Wenting Gao Beijing, CH Reviewed Fri. Aug. 28
Moody tale about Love and Loss.
Aug 28, 2020, 1:59 PM
THE SCREEN WHISPERER Abram Hammer Glenside, PA Reviewed Thurs. Aug. 27
A writer has to deal with a script consultant.
Aug 28, 2020, 12:23 AM
APORIA (THE EVANGENITALS) Juli Crockett Enterprise, AL Reviewed Thurs. Aug. 27
The one question that is on everybody’s mind right now is...
Aug 27, 2020, 8:33 PM Go here now!
At 12:30 am - my cable show.
https://www.mnn.org/watch/channels/community-channel Updated Aug 22, 2020, 12:29 AM
Aug 22, 2020, 12:29 AM
SPARKY THE SPARK DOG Cameron Carr University For The Creative Arts, UK Reviewed Thurs. Aug. 20 A dog in a cup has talent. Aug 20, 2020, 6:51 AM https://youtu.be/WXh81JTt0ng Updated Aug 18, 2020, 7:18 PM
Aug 18, 2020, 7:18 PM
THE TOUR John Gigrich Minneapolis, MN Reviewed Sun. Aug. 16 A woman makes a video postcard for her yet to be born baby. Aug 16, 2020, 9:54 PM
NO TURNING BACK Violeta Rodrigues Rio De Janeiro, Brazil Reviewed Sat. Aug. 15
The Covid-19 lockdown motivates a “successful” woman to rethink her life.
Aug 15, 2020, 6:24 PM
New Episode This Friday Night Of Transparent Film Festival Presents with clips from: Phosphene, In The Zebra Pavillion, Knives and Peaches, Buzzing 2C, The Artist Formerly Known As Lucifer, We’re At War. Watch LIVE from NYC online at: https://www.mnn.org/watch/channels/community-channel 12:30 am EST In Manhattan on FIOS (Ch. 33) Spectrum Cable (Ch. 34) RCN (Ch. 82) New Episode This Friday Night Of Transparent Film Festival Presents with clips from:
Phosphene, In The Zebra Pavillion, Knives and Peaches, Buzzing 2C, The Artist Formerly Known As Lucifer, We’re At War.
Watch LIVE from NYC online at: https://www.mnn.org/watch/channels/community-channel 12:30 am EST
In Manhattan on FIOS (Ch. 33) Spectrum Cable (Ch. 34) RCN (Ch. 82)
Aug 11, 2020, 4:53 PM
KANARTA Akimi Ota Manchester, UK Reviewed Sat. Aug. 8
The life of a medicinal botanist and his family in the Amazon rain forest.
Aug 8, 2020, 8:16 PM
PIECES OF DAVID Lawrence Lee Wallace Chicago, IL Reviewed Sat. Aug. 8
Man cheats on several girlfriends at the same time.
Aug 8, 2020, 7:51 PM
LOVE THY NEIGHBOR Brandon Smith Santa Fe, TX Reviewed Sat. Aug. 8
Greater love has no one than this, than to lay down one’s life for his friends.
Aug 8, 2020, 2:03 PM
BUZZING 2C Jayson Wesley Jersey City, NJ Reviewed Sat. Aug. 8
Two neighbors, each struggling with depression, get a temporary reprieve from their individual torment.
Aug 8, 2020, 12:41 PM
LOVE YOU LATER Charles W. Bush Dallas, TX Reviewed Sat. Aug. 8
The importance of music in a relationship.
Aug 8, 2020, 12:10 PM
EXTRANEOUS MATTER Kenichi Ugana Tokyo, Japan Reviewed Fri. Aug. 7
A hideous squid like monster becomes the lover of a group of neglected sugar babies.
Aug 7, 2020, 11:06 PM
THE ARTIST FORMERLY KNOWN AS LUCIFER Tom Carroll Armstrong, Jeffrey Frost Collegeville, PA Reviewed Thurs. Aug. 6
After showing up on Earth to rampage, kill and destroy, the devil gets sidetracked by a painting class.
Aug 6, 2020, 2:35 PM
Updated Aug 6, 2020, 2:53 AM
Aug 6, 2020, 2:53 AM
OUTSIDE Prospero Pensa Italy Reviewed Wed. Aug. 5
The elements are all around us, even in our homes.
Aug 5, 2020, 1:33 PM
WE ARE AT WAR! Felizitas Steiner, Christophe Berthier Villeneuve-Le-Roi, France Reviewed Wed. Aug. 5
A couple can’t survive the lockdown, even in the bucolic French countryside.
Aug 5, 2020, 1:37 AM
POLITICAL PRIDE Vitalijs Osipenko Los Angeles, CA Reviewed Tues. Aug. 4
Pride in the era of protest.
Aug 4, 2020, 7:22 PM
ENOUGH Jesse Hutchins Pittsburgh, PA Reviewed Tues. Jul. 28
A couple tries to put their relationship back together though substance abuse threatens to derail their reconciliation.
Jul 28, 2020, 11:42 PM
KAREN Lex Hurson New York, NY Reviewed Mon. Jul. 27
This Karen, doesn’t see it coming...
Jul 27, 2020, 11:33 PM
THE GRAND SHOWROOM James Freeman Murphy’s California Reviewed Mon. Jul. 27
A drifter wanders into an abandoned showroom which has a ghostly resident.
Jul 27, 2020, 11:17 PM
DYSTOPIAN SNOW GLOBE Charlotte Wincott Richmond, VA Screened Mon. Jul. 27 The host of a popular podcast has a fan who is not your ordinary listener. Jul 27, 2020, 8:01 PM
THE ROAD TO SUCCESS Fernando García-Pliego Madrid, Spain Reviewed Mon. Jul. 27
A mother is pressured to allow her son to take performance-enhancing drugs.
Jul 27, 2020, 6:11 PM
PHOSPHENE Bryan Ribeiro Mount Vernon, NY Reviewed Sun. Jul. 26 (Resubmission)
Two young adults struggle to overcome their respective addictions until they meet.
Jul 26, 2020, 3:44 PM
FRANKIE AND JUDE STAR WARS - The Search for Jude Amy Baklini, Lauren Myers Sherman Oaks, California Reviewed Tues. Jul. 21
A woman is panicked by the disappearance of her boyfriend.
Jul 21, 2020, 8:46 PM
Koko Ni Inai (Japanese) Emel Saat New York, NY Reviewed Mon. Jul. 20
A schoolgirl stumbles into a middle-aged woman's parallel universe.
Jul 20, 2020, 2:01 PM
THE CAROUSEL MAN Anton Evangelista Bronx, NY Reviewed Sun. Jul. 19
A different kind of Bronx Tale about one friend who supports the other in his quest to build a mini-carousel.
Jul 19, 2020, 4:14 PM
HOW TO OBTAIN BREATHING MASKS Rodja Tröscher Düsseldorf Germany Reviewed Sun. Jul. 19
A boss orders his secretary to get a hold of Gas Masks
Jul 19, 2020, 3:47 PM
SPLIT SECOND Kristen Cubbage, Phillip Lehn Hickory, NC Reviewed Fri. Jul. 17
Did you know that the second leading cause of death in children is....
Jul 17, 2020, 12:49 PM
FROM CULIACAN Carolina Herzlt TLALNEPANTLA DE BAZ, MÉXICO Reviewed Thurs. Jul. 16
A woman is separated from her loved ones during lockdown.
Jul 16, 2020, 10:42 PM Click for video:
Transparent Film Festival Presents Episode 29 - Smoky Clips from The First Responder, Recluse, Saudade, Out of Thin Air, Dog Report, Riot in the Meadow, Kaminda, Coronalone. Special Musical Guest Tim Russ (Star Trek) Transparent Film Festival Presents Episode 29 - Smoky
Clips from The First Responder, Recluse, Saudade, Out of Thin Air, Dog Report, Riot in the Meadow, Kaminda, Coronalone. Special Musical Guest Tim Russ (Star Trek)
Jul 14, 2020, 9:56 PM
COME CLOSER Frank Meyer, Maged Mohamed Woerthsee, Germany Reviewed Tues. Jul. 14
Two lovers overcome the barriers of social distancing.
Jul 14, 2020, 5:15 PM
THE FIRST RESPONDER Rajan Gangahar Brooklyn, NY Reviewed Mon. Jul. 13
A look at the emotional toll a first responder’s job takes on his personal life.
Jul 13, 2020, 11:57 PM
RECLUSE Franklin Livingston New York, NY Reviewed Fri. Jul. 10
A man with bigoted viewpoints changes when a new immigrant family moves in upstairs.
Jul 11, 2020, 1:29 AM Transparent Film Festival hosted a watch party. Transparent Film Festival's Watch Party
Jul 8, 2020, 11:52 AM
THE ICE SKATER Bryan And I London, England Reviewed Mon. Jul. 6
A doll comes back to life.
Jul 6, 2020, 10:55 AM
COVID CABIN FEVER: (Working from home with mum) Susie Sparkes Blackburn South, Australia Reviewed Mon. Jul. 6
A mother gets on her daughter’s nerves during the pandemic.
Jul 6, 2020, 10:36 AM
THE HITCHHIKER’S GUIDE TO GROWING UP Brett Chapman Sheffield, England Reviewed Thurs. July 2
Time Capsule of a trip from long ago that could present a remedy for current societal ills.
Jul 3, 2020, 8:22 PM instagram.comInstagram Post by Brian McLane • July 2, 2020 at 08:51AM EDThttps://www.instagram.com/p/CCI-1T1FClP/?igshid=v6kza8u363ps As you know our sponsors are very important to us, and to our filmmakers.Click the link to find out what’s happening at 7pm tonight from Atlanta!
https://www.instagram.com/p/CCI-1T1FClP/?igshid=v6kza8u363ps Updated Jul 2, 2020, 8:52 AM
Jul 2, 2020, 8:52 AM
OR SO THE STORY GOES: KNOCK KNOCK Theresa Labreglio Weehawken, NJ Reviewed Wed. Jul. 1
Things go missing in a small town full of off beat characters.
Jul 1, 2020, 11:17 PM
DOG REPORT Porcelain Bomb Parts Unknown Reviewed Wed. Jul. 1
A dog is disgusted with the human race (and who can blame him).
Jul 1, 2020, 10:44 PM
SAUDADE Kieron Anthony New York, NY Reviewed Wed. Jul. 1
A young man comes to the realization that his world has suddenly changed.
Jul 1, 2020, 10:29 PM
OUT OF THIN AIR Alexandra Guillossou St. Louis, MO Reviewed Wed. Jul. 1
An artist’s creations are inspired by the available resources at hand.
Jul 1, 2020, 9:58 PM
KNIVES AND PEACHES Adrian Daniel Botnariu Berlin, Germany Reviewed Wed. Jul. 1
Former friends settle a score over a past infidelity.
Jul 1, 2020, 2:09 PM
RIOT IN THE MEADOW Thomas Harman London, UK Reviewed Tues. Jun. 30
Touching portrait of the British artist Vicky Hawkins.
Jun 30, 2020, 11:54 PM
THE MUSIC TEAM (Herman Faustus Chatman Pt. 2) Jonathan Croft Ontario, CA Reviewed Sat. Jun. 27
A musician tries his best to be creative without instruments.
Jun 27, 2020, 1:46 PM
TITIEN 1538 Jérôme Possoz Angrie, FR Reviewed Sat. Jun. 27 Voyeurism or truth? Jun 27, 2020, 1:35 PM
NO SAFE HARBOR Noah Schwartz New York, NY Reviewed Friday Jun. 26
The concept of what it means to be “safe and secure” is challenged.
Jun 26, 2020, 4:02 PM
LAYOVER Olivier Frigon Montreal, CA Reviewed Thurs. June 25
A flight attendant finds herself in over her head, after doing a favor for a friend.
Jun 25, 2020, 11:45 PM
THE FINAL PERFORMANCE David J. Phillips Canada Reviewed Wednesday June 24 A would be clown gives a performance to a captive audience. Jun 24, 2020, 9:00 PM Updated Jun 24, 2020, 11:41 AM
Jun 24, 2020, 11:41 AM
THE ZEBRA PAVILLION Melanie Futorian New York, NY Reviewed Tues. Jun. 23
A visual collage duplicating a world where Black and White don’t stand alone.
Jun 23, 2020, 11:03 PM
KAMINDA David van Delden The Netherlands Reviewed Mon. Jun. 22
A fearless little boy explores the world around him.
Jun 22, 2020, 4:21 PM
ONE EARTH, ONE CHANCE Mansha Totla India Reviewed Sun. June 21
Plastics are choking the planet. Along with asshole politicians. So you have less time to do twice as much.
Jun 21, 2020, 2:04 PM Transparent Film Festival posted in Independent Horror Filmmakers. https://www.facebook.com/watch/?v=3255005944563273 Jun 20, 2020, 8:39 AM Transparent Film Festival posted in Asian Actors & Filmmakers Community. Transparent Film Festival has been a champion of all filmmakers including our 2020 Horror Winner "Hello Baby" directed by Lee Siu Lung.
Please take a look at some of the best Black Filmmakers.
https://www.facebook.com/watch/?v=3255005944563273 Jun 19, 2020, 10:32 PM Click for video:
Transparent Film Festival Presents Episode 27 Black Filmmakers Special - Sponsored By Joseph Victori Wines Clips from MLK JR BLVD, REYNA, THE BEEZ & THE CHICKADEEZ Music Videos from Daniel Laurent & J Key. Transparent Film Festival Presents Episode 27 Black Filmmakers Special - Sponsored By Joseph Victori Wines
Clips from MLK JR BLVD, REYNA, THE BEEZ & THE CHICKADEEZ Music Videos from Daniel Laurent & J Key.
Jun 19, 2020, 8:45 PM Click for video:
Transparent Film Festival Presents Episode 27 - Black Filmmakers Special Brought To You By SweetBitch Wines Transparent Film Festival Presents Episode 27 - Black Filmmakers Special Brought To You By SweetBitch Wines
Jun 19, 2020, 8:33 PM
CORONALONE Nigel Gould-Davies London, England Reviewed Thursday Jun. 18
A man comes face to face with his true self in lockdown.
Jun 18, 2020, 9:58 PM
TELEAPATIC (Minova Music Video) Sito Ruiz, Esteve Puig Spain Reviewed Tues. Jun. 17
It’s back to the future with a video that’s like New Order meets Tron.
Jun 17, 2020, 10:09 AM
DRIFTWOOD Rebecka Ray Los Angeles, CA Reviewed Mon. Jun. 15 A woman believes in Aliens and UFOs. Jun 15, 2020, 10:06 PM
CFA Presents "WAXWING SLAIN (1968)" by John Boggs (commentary by Charles Minnimatsu) Michael Natale Massapequa, NY
Reviewed Sun. Jun. 14
A student defends his mentor’s filmmaking legacy.
Jun 14, 2020, 11:23 PM
WHITE WALLS Carol Rotella New York, NY Reviewed Sat. Jun. 13 A young woman does her best to keep occupied during quarantine. Jun 13, 2020, 10:33 AM
THE CLOWN Laura Lewis-Barr Elmwood Park, IL Reviewed Fri. Jun. 12 Barbiemation about a businesswoman who encounters a clown in a park. Jun 12, 2020, 10:53 PM
SCRAMBLED Bret Lada New York, NY Reviewed Wednesday Jun. 10
A womanizer is surprised by his girlfriend.
Jun 10, 2020, 11:47 PM
COWBOY BOOTS Donny Walker Chicago, IL Reviewed Tues. Jun. 9
Well orchestrated country song by a talented new music artist from Chicago.
Jun 9, 2020, 8:45 PM
Piss Off Henry Baker Washington, D.C. Reviewed Mon. June 8
A man gets off on urinating in public.
Jun 8, 2020, 11:17 PM https://youtu.be/Sff7Kc77QAY Updated Jun 8, 2020, 2:20 AM
Jun 8, 2020, 2:20 AM
WREATHLESS Georgios Sefer Greece Reviewed Sun. Jun. 7 A variety of people wake up in a void and encounter a strange foe...
Jun 7, 2020, 10:27 PM
REBECCA Niladree Bhattacharya West Bengal, IN Reviewed Sat. Jun. 6
A traumatized woman can’t get along with her therapist(s).
Jun 6, 2020, 2:41 PM
CHUTZPAH Carter Bowden Little Rock, AR Reviewed Fri. June 5
A teenager has a hard time accepting his father’s new love.
Jun 5, 2020, 11:52 PM
Like You’re Dying Kelsey Ferrell Nashville, TN Reviewed Thurs. June 4
A young woman struggles to find meaning In her life despite coping with a life threatening illness.
Jun 4, 2020, 10:16 PM
Sunder Edward Heredia Norwich, UK Reviewed Wed. June 3
A woman's night of relaxing at home is disrupted by a mysterious visitor.
Jun 3, 2020, 5:29 PM
Mixed Movie Vasco Diogo Lisbon, PT Reviewed Wed. June 3
A moving collage creating on the iPhone using apps.
Jun 3, 2020, 5:23 PM
Crossroads Elijah Rodriguez Brooklyn, NY Reviewed Wed. June 3
Two couples go away for a weekend but only one makes it home.
Jun 3, 2020, 5:02 PM
Player 2 Jonathan Lau Roseville, MI Reviewed Wed. June 3
The bond of two brothers as seen through the lens of their shared love of video games.
Jun 3, 2020, 4:30 PM
Clear Creek Raymond Hill Portland, OR Reviewed Tues. June 2
Desperate men battle it out in the Oregon forest circa 1905
Jun 3, 2020, 1:01 AM Transparent 3 is now officially open. 2020 winners will be featured. New films, new categories, new mission.
filmfreeway.com/transparentfilmfestival Updated Jun 1, 2020, 9:10 AM
Jun 1, 2020, 9:10 AM
As we get ready for Transparent 3 (which will officially open June 1st), we just thought it would be a nice reminder to look at all the great selections from Transparent 2.
So these next few nights we'll run the selections, finalists and winners clips every other night.
Barring another pandemic, global power outage or alien invasion, Transparent 3 will take place in NYC at The Producer's Club Thursday April 8th through 10th. One of the programs will be The Winners From 2020.
Here's how it unfolded. Thanks again for you support.
https://www.facebook.com/transparentfilmfestival/videos/203177567659783/ Updated May 27, 2020, 8:06 PM
May 27, 2020, 8:06 PM
If Devo were formed in Ibiza today it might sound like this.... https://m.youtube.com/watch?v=INn8c_n-FW0 May 20, 2020, 2:38 AM Transparent Film Festival updated their status. THE WINNERS - AND WRAP UP
The judges have made their decisions. I will acknowledge my own favorites in another post.
There are 2 or 3 films across all categories that are worthy of "Winner" status. This is also true in all three Best Director, Best Actor and Best Actress categories.
One fact is indisputable. Features carry more weight than shorts in the decision making process because it's harder to maintain excellence for a longer period of time.
We would love to see more features, as well as follow up episodes in the TV Pilot Category. We will be providing a waiver/discount code for filmmakers who submit a second episode to Transparent 3 (2021).
Over this next year, we will continue to build out our streaming channel (TransparentFilmFestival.com). It's a great time to join if you haven't already. So support us as we promote the best in True Indie.
Our weekly show, Transparent Film Festival Presents will be expanding to new television markets.
Thank you all for the kind reviews. It was an amazing year despite the inherent challenges we all faced.
Brian McLane Director
May 5, 2020, 12:08 PM Click for video:
Transparent Film Festival 2020 Winners Special THE WINNERS - AND WRAP UP The judges have made their decisions. I will acknowledge my own favorites in another post. There are 2 or 3 films across all categories that are worthy of "Winner" status. This is also true in all three Best Director, Best Actor and Best Actress categories. One fact is indisputable. Features carry more weight than shorts in the decision making process because it's harder to maintain excellence for a longer period of time. We would love to see more features, as well as follow up episodes in the TV Pilot Category. We will be providing a waiver/discount code for filmmakers who submit a second episode to Transparent 3 (2021). Over this next year, we will continue to build out our streaming channel (TransparentFilmFestival.com). It's a great time to join if you haven't already. So support us as we promote the best in True Indie. Our weekly show, Transparent Film Festival Presents will be expanding to new television markets. Thank you all for the kind reviews. It was an amazing year despite the inherent challenges we all faced. Brian McLane Director Transparent Film Festival 2020 Winners Special
THE WINNERS - AND WRAP UP
The judges have made their decisions. I will acknowledge my own favorites in another post.
There are 2 or 3 films across all categories that are worthy of "Winner" status. This is also true in all three Best Director, Best Actor and Best Actress categories.
One fact is indisputable. Features carry more weight than shorts in the decision making process because it's harder to maintain excellence for a longer period of time.
We would love to see more features, as well as follow up episodes in the TV Pilot Category. We will be providing a waiver/discount code for filmmakers who submit a second episode to Transparent 3 (2021).
Over this next year, we will continue to build out our streaming channel (TransparentFilmFestival.com). It's a great time to join if you haven't already. So support us as we promote the best in True Indie.
Our weekly show, Transparent Film Festival Presents will be expanding to new television markets.
Thank you all for the kind reviews. It was an amazing year despite the inherent challenges we all faced.
Brian McLane Director
May 5, 2020, 12:01 PM Transparent Film Festival updated their status. IMPORTANT UPDATE:
We have just had confirmation that New York City will not be reopening for "Phase 2" (meaning bars and nightclubs), before at best, June 15. Accordingly, that precludes us from putting on a live screening this year.
The winning films as well as Best Director, Best Actor and Best Actress will be announced on the next episode of Transparent Film Festival Presents (Friday night May 8th) at 9pm EST.
A program will be added to Transparent 3 (April 2021) that will include the winners from Transparent 2 (this year's festival). All winners from Transparent 2 will be included at the Transparent 3 Awards Ceremony.
Let's hope these assholes don't find a way to kill us all before then. Keep making art... or die trying.
May 1, 2020, 12:23 PM While we’re waiting, from our friend Charles Roth...
https://mobile.twitter.com/rexchapman/status/1253016359455383552?s=21&fbclid=IwAR3u5cBIi7CxL9HAlf2sUJx-KvD7tIT7LjN1SxcSQrDClkGjGCZPbkX1xLY Updated Apr 23, 2020, 11:49 AM
Apr 23, 2020, 11:49 AM Transparent Film Festival updated their status. Just to give you an idea of what we’re dealing with on almost a daily basis.... An email from someone who hasn’t been following since October...
"...Have any films dropped out by any chance? We would love to screen. Has the festival been canceled? I’d still love to know why the movie wasn’t accepted. Between the 20 best picture awards we’ve already won and (they name drop a TV show) coming to an end and our lead actor being the star of (they name drop the same TV show) (and all over the media right now), I’m trying to understand the logic..."
Our response is this... Just so there’s no confusion...
We don’t care who you know, how many awards you won, how many people could “pack our screenings”, etc. We don’t need to explain our logic to you. We screen every film from beginning to end, let you know when we watched it and post screen grabs. The films make it to a second round and then go to our judges (who have Emmys and Grammys - one had an Oscar but he died), and they make the decisions not the festival organizers.
As far as when the finalists will be screened, that’s up to Governor Andrew Cuomo. If that doesn’t happen in May, we will do a show similar to the last one (The Finalists Episode), of Transparent Film Festival Presents the last weekend of May where we will announce the winners.
One final thought. Network Television sucks and all 5 of the TV Pilots in that category are just as good (if not better) than the show you referenced.
Nuff Said. Have a nice day.
Apr 16, 2020, 3:18 PM So you know how we're all sitting around with some extra time to be creative....
https://vimeo.com/406912285 Updated Apr 12, 2020, 8:01 PM
Apr 12, 2020, 8:01 PM Click for video:
We started The Transparent Film Festival because, as filmmakers, we were tired of getting ripped off. Ripped off by “film festivals” collecting submissions fees and sending us a form letter. In many cases, we weren’t even told whether we were accepted or rejected. Sundance (we’ll never bother with that again), had 13,000 submissions in 2018 and maybe a dozen people “to screen them”. Do the math. And factor in features. So our concept was, start a festival, get qualified judges, watch every frame and prove it by showing on Facebook that we watched your film and when, let the filmmakers know what we thought. Here are the best of the best, of the best. In some cases we’re still arguing (Best Short and Best Actress). We’ll keep you in the loop. We started The Transparent Film Festival because, as filmmakers, we were tired of getting ripped off. Ripped off by “film festivals” collecting submissions fees and sending us a form letter. In many cases, we weren’t even told whether we were accepted or rejected. Sundance (we’ll never bother with that again), had 13,000 submissions in 2018 and maybe a dozen people “to screen them”. Do the math. And factor in features.
So our concept was, start a festival, get qualified judges, watch every frame and prove it by showing on Facebook that we watched your film and when, let the filmmakers know what we thought.
Here are the best of the best, of the best. In some cases we’re still arguing (Best Short and Best Actress). We’ll keep you in the loop.
Apr 11, 2020, 3:32 AM
UPDATE: We have so much news we'd like to share, but we're so busy working that I'll just have to encapsulate a few items. 1. Just got this statistic (photo attached). While many film festivals talk a good game, we deliver. 2. The next episode of Transparent Film Festival Presents (Episode 26) will air Friday night at 9pm EST. The show will feature the two finalists in each category. We can tell you that the choices have been made in all film categories but the SHORTS category where there is an intense debate going on. In Bet Feature, Documentary, Mobile, Foreign, Performance, and Horror - we have narrowed the field. And it was hard!!!! 3. Episode 27 of Transparent Film Festival Presents (Bubble), will feature some of the films that could have easily been selected. They were that good. But we just couldn't select everyone. So this "Honorable Mention" show is for them. 4. We will. be announcing our first writing challenge with a Cash Prize for the winning submission. It involves finishing a script that was half finished. More about that soon. UPDATE: We have so much news we'd like to share, but we're so busy working that I'll just have to encapsulate a few items.
1. Just got this statistic (photo attached). While many film festivals talk a good game, we deliver.
2. The next episode of Transparent Film Festival Presents (Episode 26) will air Friday night at 9pm EST. The show will feature the two finalists in each category. We can tell you that the choices have been made in all film categories but the SHORTS category where there is an intense debate going on. In Bet Feature, Documentary, Mobile, Foreign, Performance, and Horror - we have narrowed the field. And it was hard!!!!
3. Episode 27 of Transparent Film Festival Presents (Bubble), will feature some of the films that could have easily been selected. They were that good. But we just couldn't select everyone. So this "Honorable Mention" show is for them.
4. We will. be announcing our first writing challenge with a Cash Prize for the winning submission. It involves finishing a script that was half finished. More about that soon.
Apr 8, 2020, 10:22 PM
We're grateful to those of you that took the time to give us a review on Film Freeway, and hope that more of you will do so. https://filmfreeway.com/TransparentFilmFestival#reviews We're grateful to those of you that took the time to give us a review on Film Freeway, and hope that more of you will do so.
https://filmfreeway.com/TransparentFilmFestival#reviews
Apr 7, 2020, 3:27 PM
Transparent Film Festival 2020 by the numbers. Transparent Film Festival 2020 by the numbers.
Apr 7, 2020, 3:24 PM Transparent Film Festival updated their status. This Friday Night on this page will be our Finalists Show for all categories. Each will have a Winner and a Runner-Up:
An email has been sent to all filmmakers Regarding next steps. Please check your email.
Apr 7, 2020, 12:58 AM Transparent Film Festival updated their status. The Transparent Film Festival 2020 has now officially concluded.
Winners will be announced soon.
Thank you all.
🙏
Apr 6, 2020, 3:10 AM You tagged George Hardy
This is it! This is the day so.... Meet Your Judges! We're looking for not only well produced movies, but movies with swagger and buzz. Production Value and Performance are certainly big parts of the equation, but we're also looking at the intangibles as well. You have all been great! Let the Judging begin! BRIAN MCLANE In addition to producing and starring in 2018's horror rock musical "The Killer's Requiem", Brian started The Transparent Film Festival. He has extensively written for television and film including for MTV, Discovery Channel, PBS, and The Food Network. He is in script on 2 new features. TIM RUSS Tim Russ is known for his roles as Lieutenant Commander Tuvok on Star Trek: Voyager, as Frank on Samantha Who?, and as Principal Franklin, a recurring character on the Nickelodeon live-action teen sitcom iCarly. He has an Emmy. MICHAEL FARR Michael is a Producer and Director of over 2000 hours of prime time television programming for both domestic and international markets. He has produced numerous live concerts including for Prince, Elton John as well as worked with U2, Justin Timberlake and Green Day. He has worked on Mission Impossible 3, Dream Girls, and with Ken Burns. CHRIS CONWAY Chris Conway is best known for his work with Eminem on The Marshall Mathers LP (Best Rap Album at the 43rd Grammy Awards). Chris has worked with pretty much every major rapper from Puffy and Biggie Smalls to Wiz Khalifa. He works out of No Mystery studios in NYC. JAMIE LYNN SHEERIN Jamie is a marketing executive who started out working for Wyndham, before transitioning to film. She helped establish Palm Aisles Pictures, a New York film production company, in 2015. A film buff herself, Jamie is also a co-host of the travel show Adventures Over 55 with Festival Organizer Brian McLane. GEORGE HARDY After making his debut in the widely popular cult film favorite Troll 2, George Hardy received widespread critical and audience acclaim for Best Worst Movie. After a slew of character actor appearances including "Father Davenport" in The Killer's Requiem (2018), George landed the lead role as Sgt. Travis Delmore in Texas Cotton (2018). He is currently in production on "CYST". MICHAEL ATTALI Michael started apprenticing under renowned Directors Bruno Dumont and Rachid Bouchareb, and he has never looked back. Films he has worked on include; "Ma Loute" (2016) nominated at Canne’s Festival and the Cesars (French Oscars), "Jeanne" (2019), Lil’ Quinquin (2015-2018), and "By a half Clear Morning" (2020). He is currently directing “A Voodoo’s Tale”. This is it! This is the day so.... Meet Your Judges! We're looking for not only well produced movies, but movies with swagger and buzz. Production Value and Performance are certainly big parts of the equation, but we're also looking at the intangibles as well. You have all been great! Let the Judging begin!
BRIAN MCLANE In addition to producing and starring in 2018's horror rock musical "The Killer's Requiem", Brian started The Transparent Film Festival. He has extensively written for television and film including for MTV, Discovery Channel, PBS, and The Food Network. He is in script on 2 new features.
TIM RUSS
Tim Russ is known for his roles as Lieutenant Commander Tuvok on Star Trek: Voyager, as Frank on Samantha Who?, and as Principal Franklin, a recurring character on the Nickelodeon live-action teen sitcom iCarly. He has an Emmy.
MICHAEL FARR
Michael is a Producer and Director of over 2000 hours of prime time television programming for both domestic and international markets. He has produced numerous live concerts including for Prince, Elton John as well as worked with U2, Justin Timberlake and Green Day. He has worked on Mission Impossible 3, Dream Girls, and with Ken Burns.
CHRIS CONWAY
Chris Conway is best known for his work with Eminem on The Marshall Mathers LP (Best Rap Album at the 43rd Grammy Awards). Chris has worked with pretty much every major rapper from Puffy and Biggie Smalls to Wiz Khalifa. He works out of No Mystery studios in NYC.
JAMIE LYNN SHEERIN
Jamie is a marketing executive who started out working for Wyndham, before transitioning to film. She helped establish Palm Aisles Pictures, a New York film production company, in 2015. A film buff herself, Jamie is also a co-host of the travel show Adventures Over 55 with Festival Organizer Brian McLane.
GEORGE HARDY
After making his debut in the widely popular cult film favorite Troll 2, George Hardy received widespread critical and audience acclaim for Best Worst Movie. After a slew of character actor appearances including "Father Davenport" in The Killer's Requiem (2018), George landed the lead role as Sgt. Travis Delmore in Texas Cotton (2018). He is currently in production on "CYST".
MICHAEL ATTALI
Michael started apprenticing under renowned Directors Bruno Dumont and Rachid Bouchareb, and he has never looked back. Films he has worked on include; "Ma Loute" (2016) nominated at Canne’s Festival and the Cesars (French Oscars), "Jeanne" (2019), Lil’ Quinquin (2015-2018), and "By a half Clear Morning" (2020). He is currently directing “A Voodoo’s Tale”.
Apr 5, 2020, 10:06 AM Transparent Film Festival updated their status. Festival in full swing and we are definitely looking at which films have more complete views when determining winners. We have great analytics.
UPDATE SUNDAY NIGHT 12:15 EST
Transparent Film Festival 2020 is coming to a close.
We want to thank everyone for what will surely be a most memorable film festival. We screened every frame of close to 300 films, provided our notes and ratings, and chose the films we thought were the best of the best. Then, the Coronavirus.
We will leave the programs up until 11:59 pm PCT (Pacific) to accommodate all those great films from California and Vancouver.
Tomorrow (Monday), we'll send out an update as to what happens next. We will post here, as well as email everyone.
Again, great job to you all. When FilmFreeway.com as if you want to leave a review, we hope you will.
TFF
UPDATE SUNDAY NIGHT 9:30 EST The numbers don’t lie. Very strong TV Pilots but o e is standing out. And our Judge Tim Russ has just sent in his comments.
“... ___________________ is my number 1 choice for the pilots. It's clever, well shot, well edited, well acted and well paced. Quite good! tim
UPDATE AS OF 10:30 EST Virtually all of the interest seems to be in Programs 4 and 7.
UPDATE AS OF 4:30 EST A marked increase in tickets being purchased with lots of views starting to come in. Definitely fascinating to watch from the backend!
Lots of great posts below below btw.
Apr 4, 2020, 3:02 PM Transparent Film Festival updated their status. Guys it’s simple... click the button. Choose the program you want. Pay for your ticket.
One of the things we’re hearing back is how impressed each of you are - with EVERYONE ELSE that they’re competing against. We’ve given out comps to approximately half the filmmakers. The rest I guess don’t know or didn’t care.
We posted several months ago, that completing your film is only the beginning. Making money and being successful in the business, is a whole ‘nother thing. Interacting with and collaborating with others, learning and teaching others, attending screenings and the film markets and making connections, and so on.
If you think that people are just going to drop out of the sky and love your film, good luck. 😉 However, seeing the methods and techniques utilized by your fellow filmmakers, is alone worth the price of admission. The All Access Subscription for $25 (for a year!!!) is like a semester of film school. That’s how incredible these films are. And they are from all over the world. Especially the foreign films competition, it’s mind-boggling.
You can’t become a successful filmmaker in a vacuum.
Apr 4, 2020, 10:17 AM Updated Apr 4, 2020, 10:13 AM
Apr 4, 2020, 10:13 AM Transparent Film Festival posted in Independent Horror Filmmakers. Great Horror Program (Program 4)
This weekend, support the best in True Indie. In these difficult times, these incredible filmmakers from around the world have so much to say - and the quality will blow you away.
https://www.transparentfilmfestival.com/transparent-film-festival-2020 Apr 4, 2020, 8:19 AM
People have asked how much the All Access Ticket to all programs is. The answer is $24.99. Here is the link: https://www.transparentfilmfestival.com/ People have asked how much the All Access Ticket to all programs is. The answer is $24.99. Here is the link: https://www.transparentfilmfestival.com/
Apr 3, 2020, 10:24 PM Transparent Film Festival posted in New York Filmmakers and Actors. This weekend, support the best in True Indie. In these difficult times, these incredible filmmakers from around the world have so much to say - and the quality will blow you away.
https://www.transparentfilmfestival.com/transparent-film-festival-2020 Apr 3, 2020, 6:53 PM Transparent Film Festival posted in Asian Actors & Filmmakers Community. This weekend, support the best in True Indie. In these difficult times, these incredible filmmakers from around the world have so much to say - and the quality will blow you away.
https://www.transparentfilmfestival.com/transparent-film-festival-2020 Apr 3, 2020, 6:52 PM Transparent Film Festival posted in Low Budget films, Indie (Indy) Filmaking ( Low budget Film Makers). This weekend, support the best in True Indie. https://www.transparentfilmfestival.com/transparent-film-festival-2020 Apr 3, 2020, 6:39 PM The Festival is Live and will be extended through until Sunday at 11:59pm EST. https://www.transparentfilmfestival.com/transparent-film-festival-2020
Comps for filmmakers are available until midnight. We have someone standing by to accommodate you.
Please understand that anyone attempting to use an email login from multiple IP addresses will have their access revoked, and their film disqualified from competition. Updated Apr 2, 2020, 9:49 PM
Apr 2, 2020, 9:49 PM Transparent Film Festival updated their status. IMPORTANT UPDATE
We want to thank all of our judges, techs and staff for helping us pull this off at this most difficult time. That's how much we honor all of you.
Several filmmakers have now received their comp to the program in which their film is screening. We have verified with several of you already that the process works. The comps are just that, for you. However, please understand we have excellent analytics.
Anyone trying to use a comp email login from multiple IP addresses, will have their access revoked - and their film removed from the competition.
It takes a lot of time to set this up, for each individual comp. This is why we aren't just automatically adding people unless they requested access. It would take more time than we have, especially with a skeleton staff due to the virus.
As we said in our email to all filmmakers, whose films are screening as part of the festival - YOU MUST REQUEST YOUR COMP BY MIDNIGHT TONIGHT (EST - NYC Time).
Now for a bit of bad news.
A friend of the festival with whom some of us worked back in November, has died of the Coronavirus. So, there's that.
We will check in again just before the official start of the festival at midnight tonight and make sure every filmmaker who has requested a comp for their program, has one.
Apr 2, 2020, 7:43 PM Transparent Film Festival added a new photo.
Program 3 – Foreign Film Competition Crusher� Dagny Forgotten Alive Gravediggers Hoek’s Delta Kukli La Herencia De Marti Nice to Meet You NOM Romance Silent Brainwash The Broadcast The Elephants The Wrecker What Remains Apr 1, 2020, 11:39 PM Transparent Film Festival added a new photo.
Program 4 – Crime & Horror Competition Angels Gone Apocalyptic Life Applecalypse Assistance Done Waiting Family Bond Hello Baby Help Me Killing Time Kung Pao Corpse Reyna Thirst Voracious Apr 1, 2020, 11:39 PM Transparent Film Festival added a new photo.
Program 5 – Features Competition A Ship Of Human Skin Broke The Day After Halloween Background Noise The Fall Exit 0 Apr 1, 2020, 11:39 PM Transparent Film Festival added a new photo.
Program 2 – Short Film Competition Cashed Dream Cafe Flamboyanes Panic Attack Stuffed Tableaux The Beez & The Chickadeez The Holdout The Tea The Trouble With Cats You Look Great Apr 1, 2020, 11:39 PM Transparent Film Festival added a new photo.
Program 1 – Short Film Competition Ada After You’ve Gone� Borrowing Dance With A Demon Good Intentions MLK Jr. Blvd. Power Out Silence The End Of June The Interning The Marionette Doll The Norm Apr 1, 2020, 11:39 PM Transparent Film Festival added a new photo.
Program 7 – TV Pilots After Life� The Blue Marble The Queen’s English L'uomo Nuovo Highbread Apr 1, 2020, 11:39 PM Transparent Film Festival added a new photo.
Program 6 - Docs, Mobile & Performance Video Competition The Stop After The Flood Bend Low Sweet Branch Coastline Crazy Hapax Elegy Immortality� Irradiate Like Meat Outside Vegetable Skin Apr 1, 2020, 11:39 PM Transparent Film Festival added a new photo.
Transparent Film Festival 2020 Selection The Queen's English (TV Pilot) Mar 30, 2020, 5:40 PM Transparent Film Festival added a new photo.
Transparent Film Festival 2020 Selection Forgotten Alive (Short) Mar 30, 2020, 4:46 PM Transparent Film Festival added a new photo.
Transparent Film Festival 2020 Selection Kung Pao Corpse (Short) Mar 30, 2020, 4:28 PM Transparent Film Festival added a new photo.
Transparent Film Festival 2020 Selection Hapax Elegy (Performance) Mar 30, 2020, 3:36 PM Transparent Film Festival added a new photo.
Mar 30, 2020, 3:29 PM Transparent Film Festival updated their status. IMPORTANT - FILMMAKERS PLEASE READ
5 QUICK FACTS re TRANSPARENT 2020 (COVID-19 Version)
As New York City is on "lockdown", we will continue with our festival online. When restrictions are lifted and it is deemed safe to hold our festival, we will get new dates and hold our live film festival event.
The film festival will take place here: www.transparentfilmfestival.com
Filmmakers please take note:
1. We need your 1920 x 1080 compressed movs or mp4s no later than 5 pm Tuesday to be included in the festival (online). There will be no exceptions. We have to sequence several programs. Programs will be a mix of genres and will time at approximately 3 hours each.
2. Films that screen online will be given priority with respect to the live festival when scheduled. This means that we don't know what's going to happen in the future with the pandemic situation and what we're going to have access to in the future. However, those films that screen this weekend, will be given first consideration if and when the live festival is scheduled.
3. Films that screen online as part of the festival will be uploaded to TransparentFilmFestival.com. After the festival, all films selected and uploaded for the 2020 Transparent Film Festival will be promptly deleted UPON NOTIFICATION BY SUBMITTING FILMMAKER.
4. There will be two types of digital tickets available: The first is per program and the second is for "ALL ACCESS" which will allow for the viewing of all festival content.
5. Each filmmaker taking part in the online festival will receive 1 comp to view the program in which their film is included. To receive your comp access, or if you have any questions at all, please email us at [email protected].
Mar 30, 2020, 1:56 PM Transparent Film Festival added a new photo.
Transparent Film Festival 2020 Selection Bend Low, Sweet Branch, Bend Low (Performance) Mar 29, 2020, 12:08 AM Transparent Film Festival added a new photo.
Transparent Film Festival 2020 Selection Done Waiting (Short) Mar 29, 2020, 12:08 AM Transparent Film Festival added a new photo.
Transparent Film Festival 2020 Selection Dance With A Demon (Performance) Mar 29, 2020, 12:08 AM Transparent Film Festival added a new photo.
Transparent Film Festival 2020 Selection Dagny (Short) Mar 29, 2020, 12:08 AM Transparent Film Festival added a new photo.
Transparent Film Festival 2020 Selection Crusher (Short) Mar 29, 2020, 12:08 AM Transparent Film Festival added a new photo.
Transparent Film Festival 2020 Selection Crazy (Performance) Mar 29, 2020, 12:08 AM Transparent Film Festival added a new photo.
Transparent Film Festival 2020 Selection Claire (Short) Mar 29, 2020, 12:08 AM Transparent Film Festival added a new photo.
Transparent Film Festival 2020 Selection Cashed (Short) Mar 29, 2020, 12:08 AM Transparent Film Festival added a new photo.
Transparent Film Festival 2020 Selection Broke (Feature) Mar 29, 2020, 12:08 AM Transparent Film Festival added a new photo.
Transparent Film Festival 2020 Selection Borrowing (Short) Mar 29, 2020, 12:08 AM Transparent Film Festival added a new photo.
Transparent Film Festival 2020 Selection Assistance (Horror) Mar 29, 2020, 12:08 AM Transparent Film Festival added a new photo.
Transparent Film Festival 2020 Selection Background Noise (Feature) Mar 29, 2020, 12:08 AM Transparent Film Festival added a new photo.
Transparent Film Festival 2020 Selection Gravediggers (Short) Mar 29, 2020, 12:08 AM Transparent Film Festival added a new photo.
Transparent Film Festival 2020 Selection Astronaut (Performance) Mar 29, 2020, 12:08 AM Transparent Film Festival added a new photo.
Transparent Film Festival 2020 Selection Applecalypse (Short) Mar 29, 2020, 12:08 AM Transparent Film Festival added a new photo.
Transparent Film Festival 2020 Selection Angels Gone (Horror) Mar 29, 2020, 12:08 AM Transparent Film Festival added a new photo.
Transparent Film Festival 2020 Selection After You've Gone (Short) Mar 29, 2020, 12:08 AM Transparent Film Festival added a new photo.
Transparent Film Festival 2020 Selection After The Flood (Mobile) Mar 29, 2020, 12:08 AM Transparent Film Festival added a new photo.
Transparent Film Festival 2020 Selection After Life (TV Pilot) Mar 29, 2020, 12:08 AM Transparent Film Festival added a new photo.
Transparent Film Festival 2020 Selection Ada (Short) Mar 29, 2020, 12:08 AM Transparent Film Festival added a new photo.
Transparent Film Festival 2020 Selection A Ship Of Human Skin (Feature) Mar 29, 2020, 12:08 AM Transparent Film Festival added a new photo.
Transparent Film Festival 2020 Selection Exit 0 (Feature) Mar 29, 2020, 12:08 AM Transparent Film Festival added a new photo.
Transparent Film Festival 2020 Selection Apocalyptic Life (Short) Mar 29, 2020, 12:08 AM Transparent Film Festival added a new photo.
Transparent Film Festival 2020 Selection Dream Cafe (Short) Mar 29, 2020, 12:08 AM Transparent Film Festival added a new photo.
Transparent Film Festival 2020 Selection Family Bond (Short) Mar 29, 2020, 12:08 AM Transparent Film Festival added a new photo.
Transparent Film Festival 2020 Selection The Broadcast (Mobile) Mar 29, 2020, 12:08 AM Transparent Film Festival added a new photo.
Transparent Film Festival 2020 Selection What Remains (Short) Mar 29, 2020, 12:08 AM Transparent Film Festival added a new photo.
Transparent Film Festival 2020 Selection Voracious (Horror) Mar 29, 2020, 12:08 AM Transparent Film Festival added a new photo.
Transparent Film Festival 2020 Selection Flamboyanes (Short) Mar 29, 2020, 12:08 AM Transparent Film Festival added a new photo.
Transparent Film Festival 2020 Selection Vegetable Skin, An Interview (Short) Mar 29, 2020, 12:08 AM Transparent Film Festival added a new photo.
Transparent Film Festival 2020 Selection Thirst (Short) Mar 29, 2020, 12:08 AM Transparent Film Festival added a new photo.
Transparent Film Festival 2020 Selection Stuffed (Short) Mar 29, 2020, 12:08 AM Transparent Film Festival added a new photo.
Transparent Film Festival 2020 Selection Tableaux (Short) Mar 29, 2020, 12:08 AM Transparent Film Festival added a new photo.
Transparent Film Festival 2020 Selection The Beez & The Chickadeez (Short) Mar 29, 2020, 12:08 AM Transparent Film Festival added a new photo.
Transparent Film Festival 2020 Selection The Blue Marble (TV Pilot) Mar 29, 2020, 12:08 AM Transparent Film Festival added a new photo.
Transparent Film Festival 2020 Selection The Day After Halloween (Feature) Mar 29, 2020, 12:08 AM Transparent Film Festival added a new photo.
Transparent Film Festival 2020 Selection Sisters Of Sorrow (TV Pilot) Mar 29, 2020, 12:08 AM Transparent Film Festival added a new photo.
Transparent Film Festival 2020 Selection The Elephants (Short) Mar 29, 2020, 12:08 AM Transparent Film Festival added a new photo.
Transparent Film Festival 2020 Selection The End of June (Short) Mar 29, 2020, 12:08 AM Transparent Film Festival added a new photo.
Transparent Film Festival 2020 Selection The Fall (Feature) Mar 29, 2020, 12:08 AM Transparent Film Festival added a new photo.
Transparent Film Festival 2020 Selection The Holdout (Short) Mar 29, 2020, 12:08 AM Transparent Film Festival added a new photo.
Transparent Film Festival 2020 Selection The Interning (Short) Mar 29, 2020, 12:08 AM Transparent Film Festival added a new photo.
Transparent Film Festival 2020 Selection The Marionette Doll (Short) Mar 29, 2020, 12:08 AM Transparent Film Festival added a new photo.
Transparent Film Festival 2020 Selection The Norm (Short) Mar 29, 2020, 12:08 AM Transparent Film Festival added a new photo.
Transparent Film Festival 2020 Selection The Stop (Performance) Mar 29, 2020, 12:08 AM Transparent Film Festival added a new photo.
Transparent Film Festival 2020 Selection The Tea (Mobile) Mar 29, 2020, 12:08 AM Transparent Film Festival added a new photo.
Transparent Film Festival 2020 Selection You Look Great (Short) Mar 29, 2020, 12:08 AM Transparent Film Festival added a new photo.
Transparent Film Festival 2020 Selection Silent Brainwash (Short) Mar 29, 2020, 12:08 AM Transparent Film Festival added a new photo.
Transparent Film Festival 2020 Selection The Wrecker (Short) Mar 29, 2020, 12:08 AM Transparent Film Festival added a new photo.
Transparent Film Festival 2020 Selection La Herencia de Martí (Short) Mar 29, 2020, 12:08 AM Transparent Film Festival added a new photo.
Transparent Film Festival 2020 Selection Good Intentions (Short) Mar 29, 2020, 12:08 AM Transparent Film Festival added a new photo.
Transparent Film Festival 2020 Selection Hello Baby (Horror) Mar 29, 2020, 12:08 AM Transparent Film Festival added a new photo.
Transparent Film Festival 2020 Selection Help Me (Horror) Mar 29, 2020, 12:08 AM Transparent Film Festival added a new photo.
Transparent Film Festival 2020 Selection High Bread (Short) Mar 29, 2020, 12:08 AM Transparent Film Festival added a new photo.
Transparent Film Festival 2020 Selection Hoek's Delta (Short) Mar 29, 2020, 12:08 AM Transparent Film Festival added a new photo.
Transparent Film Festival 2020 Selection Immortality (Performance) Mar 29, 2020, 12:08 AM Transparent Film Festival added a new photo.
Transparent Film Festival 2020 Selection Irradiate (Mobile) Mar 29, 2020, 12:08 AM Transparent Film Festival added a new photo.
Transparent Film Festival 2020 Selection Killing Time (Short) Mar 29, 2020, 12:08 AM Transparent Film Festival added a new photo.
Transparent Film Festival 2020 Selection Kukli (Short) Mar 29, 2020, 12:08 AM Transparent Film Festival added a new photo.
Transparent Film Festival 2020 Selection L'Uomo Nuovo (TV Pilot) Mar 29, 2020, 12:08 AM Transparent Film Festival added a new photo.
Transparent Film Festival 2020 Selection Silence (Short) Mar 29, 2020, 12:08 AM Transparent Film Festival added a new photo.
Transparent Film Festival 2020 Selection Like Meat (Performance Video) Mar 29, 2020, 12:08 AM Transparent Film Festival added a new photo.
Transparent Film Festival 2020 Selection MLK JR BLVD. (Short) Mar 29, 2020, 12:08 AM Transparent Film Festival added a new photo.
Transparent Film Festival 2020 Selection Nice To Meet You (Short) Mar 29, 2020, 12:08 AM Transparent Film Festival added a new photo.
Transparent Film Festival 2020 Selection NOM (Short) Mar 29, 2020, 12:08 AM Transparent Film Festival added a new photo.
Transparent Film Festival 2020 Selection Outside (Performance) Mar 29, 2020, 12:08 AM Transparent Film Festival added a new photo.
Transparent Film Festival 2020 Selection Panic Attack (Short) Mar 29, 2020, 12:08 AM Transparent Film Festival added a new photo.
Transparent Film Festival 2020 Selection Power Out (Short) Mar 29, 2020, 12:08 AM Transparent Film Festival added a new photo.
Transparent Film Festival 2020 Selection Reyna (Short) Mar 29, 2020, 12:08 AM Transparent Film Festival added a new photo.
Transparent Film Festival 2020 Selection R O M A N C E (Short) Mar 29, 2020, 12:08 AM Transparent Film Festival added a new photo.
Transparent Film Festival 2020 Selection The Trouble With Cats (Short) Mar 29, 2020, 12:08 AM Click for video:
Transparent Film Festival 2020 Nominations Special Transparent Film Festival 2020 Nominations Special
Mar 27, 2020, 10:01 PM instagram.comInstagram Post by Brian McLane • March 27, 2020 at 07:20PM EDThttps://www.instagram.com/p/B-QVhh0FYQB/?igshid=rmn50p3jxvxh Here is the Instagram Version of the Transparent Film Festival 2020 trailer
https://www.instagram.com/p/B-QVhh0FYQB/?igshid=rmn50p3jxvxh - Please Share Updated Mar 27, 2020, 7:22 PM
Mar 27, 2020, 7:22 PM
TONIGHT AT 10 PM New York City Time Right Here On This Page TONIGHT AT 10 PM New York City Time Right Here On This Page
Mar 27, 2020, 6:14 PM Transparent Film Festival updated their status. We are being inundated with emails and requests. Just to make sure - everyone, please read. We're dealing with a lot, more than most being in New York. Please watch the Selections Special Show (below). There are a handful of selections that will be added, clips from which will be included in next Friday night's Best in Category Nominations Special.
In light of the current situation (Coronavirus), we want to make sure we honor our commitment to our filmmakers.
Film Freeway is supposed to notify filmmakers on March 22. Just in case, we are providing screenshots of our dashboard, showing our ratings. Every single filmmaker has individually received an email during the past year with our notes and ratings.
Transparent Film Festival Ratings Guide
SELECTED - BLUE FLAG (Priority for live screening) Will receive Selected and Nominations laurels and will screen live (pending venue considerations in light of the pandemic).
SELECTED - PURPLE FLAG Will receive Selected laurels and is scheduled for live screening (pending venue considerations in light of the pandemic).
GREEN FLAG - (With a Trophy Rating) Will not screen live but will receive Semi-Finalist laurels. May have a performance nomination for Best Actor or Best Actress.
GREEN FLAG - Will not screen live but will receive Semi-Finalist laurels, and offered to screen online.
ORANGE FLAG Not selected for live screening but will receive Honorable Mention laurels, and offered to screen online.
RED FLAG Just not for us.
NOTES ON SELECTION: 1. Some films marked “Not Selected” - would have been selected, but the filmmakers did not respond to our requests.
2. The first film (Exit 0) and the last film (Gravediggers) were both Selected.
3. PLEASE MONITOR THIS FEED FOR FURTHER INFORMATION
Mar 22, 2020, 9:15 PM Transparent Film Festival added a new photo.
Mar 22, 2020, 5:42 PM Transparent Film Festival added a new photo.
Mar 22, 2020, 5:42 PM Transparent Film Festival added a new photo.
Mar 22, 2020, 5:42 PM Transparent Film Festival added a new photo.
Mar 22, 2020, 5:42 PM Transparent Film Festival added a new photo.
Mar 22, 2020, 5:42 PM Transparent Film Festival added a new photo.
Mar 22, 2020, 5:42 PM Transparent Film Festival added a new photo.
Mar 22, 2020, 5:42 PM Transparent Film Festival added a new photo.
Mar 22, 2020, 5:42 PM Transparent Film Festival added a new photo.
Mar 22, 2020, 5:42 PM Transparent Film Festival added a new photo.
Mar 22, 2020, 5:42 PM Transparent Film Festival added a new photo.
Mar 22, 2020, 5:42 PM Transparent Film Festival added a new photo.
Mar 22, 2020, 5:42 PM Transparent Film Festival added a new photo.
Mar 22, 2020, 5:42 PM Transparent Film Festival added a new photo.
Mar 22, 2020, 5:42 PM Transparent Film Festival added a new photo.
Mar 22, 2020, 5:42 PM Transparent Film Festival added a new photo.
Mar 22, 2020, 5:42 PM Transparent Film Festival added a new photo.
Mar 22, 2020, 5:42 PM Transparent Film Festival added a new photo.
Mar 22, 2020, 5:42 PM Transparent Film Festival added a new photo.
Mar 22, 2020, 5:42 PM Transparent Film Festival added a new photo.
Mar 22, 2020, 5:42 PM Transparent Film Festival added a new photo.
Mar 22, 2020, 5:42 PM Transparent Film Festival added a new photo.
Mar 22, 2020, 5:42 PM Transparent Film Festival added a new photo.
Mar 22, 2020, 5:42 PM Transparent Film Festival added a new photo.
Mar 22, 2020, 5:42 PM Transparent Film Festival added a new photo.
Mar 22, 2020, 5:42 PM Transparent Film Festival added a new photo.
Mar 22, 2020, 5:42 PM Transparent Film Festival added a new photo.
Mar 22, 2020, 5:42 PM Transparent Film Festival added a new photo.
Mar 22, 2020, 5:42 PM Transparent Film Festival added a new photo.
Mar 22, 2020, 5:42 PM Transparent Film Festival added a new photo.
Mar 22, 2020, 5:42 PM Transparent Film Festival added a new photo.
Mar 22, 2020, 5:42 PM Transparent Film Festival added a new photo.
Mar 22, 2020, 5:42 PM Transparent Film Festival added a new photo.
Mar 22, 2020, 5:42 PM Transparent Film Festival added a new photo.
Mar 22, 2020, 5:42 PM Transparent Film Festival added a new photo.
Mar 22, 2020, 5:42 PM
GRAVEDIGGERS Caro Alexis Rennes, France
Screened Saturday March 21
Out for revenge, a man kidnaps the owner of the company from which he was downsized.
Mar 21, 2020, 11:01 PM
MA$ERATI MONEY BEYOND BELIEF Maserati Money Bronx, NY
Screened Saturday March 21
A man born with Spinal Bifeda beats the odds.
Mar 21, 2020, 10:56 PM Click for video:
The Transparent Film Festival Presents 2020 Selections Special The Transparent Film Festival Presents 2020 Selections Special
Mar 21, 2020, 6:51 AM Click for video:
The best in TRUE INDIE Filmmaking including clips from shorts, features, docs, horror, TV Pilots, mobile and music performances. Support independent filmmaking from around the world at its best. Visit TransparentFilmFestival.com and subscribe for 1 year for 29.99 includes 1 free submission to the 2021 Transparent Film Festival. The best in TRUE INDIE Filmmaking including clips from shorts, features, docs, horror, TV Pilots, mobile and music performances. Support independent filmmaking from around the world at its best.
Visit TransparentFilmFestival.com and subscribe for 1 year for 29.99 includes 1 free submission to the 2021 Transparent Film Festival.
Mar 21, 2020, 6:51 AM Transparent Film Festival updated their status. To accommodate some late entries, we're pushing back the selections special by an hour or so as we have to cut in and re-render. Please stand by. Thanks for your patience. I promise you it's excellent. You all did a great job
Mar 20, 2020, 9:00 PM
SELECTIONS TONIGHT AT 9PM Due to some late entries, we had to re-edit (of course). So instead of 8PM New York time, the selections, along with nominations for Best Actor, Best Actress and Best Director will be pushed back an hour. But it's definitely happening tonight!!! SELECTIONS TONIGHT AT 9PM
Due to some late entries, we had to re-edit (of course). So instead of 8PM New York time, the selections, along with nominations for Best Actor, Best Actress and Best Director will be pushed back an hour. But it's definitely happening tonight!!!
Mar 20, 2020, 5:57 PM
WHISKEY & WINGS Brian Hilario & Justin Butler Pensacola, FL Screened Friday March 20 Two men hit the road to sample the best whiskey and wings. Mar 20, 2020, 4:56 PM
Potions Lesson Julie Reedy Webster Groves, MO Screened Friday March 20 A witch tells a joke to her apprentice. Potions Lesson Julie Reedy Webster Groves, MO
Screened Friday March 20
A witch tells a joke to her apprentice.
Mar 20, 2020, 12:57 PM
The Beez & The Chickadeez Christopher Milton Boston, MA
Screened Friday March 20
Deciding to give up one's virginity often doesn't go as planned.
Mar 20, 2020, 12:26 PM
An Introduction To Thribing Rohil Aniruth South Africa Screened Thursday March 19 The challenges of being an international student living in NYC. An Introduction To Thribing Rohil Aniruth South Africa
Screened Thursday March 19
The challenges of being an international student living in NYC.
Mar 19, 2020, 9:54 PM
Richard Wilbur - Keeping the Difficult Balance Ralph Hammann Williamstown, MA Screened Thursday March 19 A thorough look at the 2nd Poet Laureate of the United States. Richard Wilbur - Keeping the Difficult Balance Ralph Hammann Williamstown, MA
Screened Thursday March 19
A thorough look at the 2nd Poet Laureate of the United States.
Mar 19, 2020, 9:50 PM
PHOSPHENE Bryan Ribeiro Mount Vernon, NY
Screened Thursday March 19
Two artistic souls, addicted to drugs, find each other.
Mar 19, 2020, 8:44 PM Transparent Film Festival updated their status. IMPORTANT UPDATE
Filmmakers please read.
NYC has closed down. We will be most likely postponing the live screenings however, we will screen the selected films online on Friday Night April 3rd and Saturday Night April 4th at the same times as they would have screened.
This will also be the same order that they will screen not if, but WHEN we secure our new dates at the same venue - the Producers Club - which has been paid for in full.
We will also be able to screen additional films that deserve the selection laurels because they are that well produced, but wouldn't have been able to screen live due to time constraints. We were going to get a 3rd day, but then things changed in a hurry.
A good thing is that we will be able to screen more features instead of only when.
We will let you know about new dates (if indeed we need to postpone the live screenings) as soon as the danger from COVID-19 has passed. But for now, all entertainment venues in NYC are closed, and no public gatherings with more than 10 people are permitted. So it's out of our control.
THAT SAID.... we will be airing our Selections Special this Friday night right here on Facebook while doing watch parties with about 20 filmmaking groups at 8PM EST.
It's going to be a great show. Please all of you stay safe.
Mar 18, 2020, 3:57 PM
THE DAY AFTER HALLOWEEN Chad Ostrom Brooklyn, NY
Screened Monday March 16
After a drive-in closes for the season, gore and witty banter fill the air in rural Pennsylvania.
Mar 17, 2020, 12:53 AM Transparent Film Festival updated their status. Part of being a successful filmmaker, goes beyond making the film.
It's taking advantage of opportunities when they present themselves, and of course being communicative as well as accommodating.
We are starting to notify filmmakers.
If we've reached out to you already and you haven't responded with what we've requested, your film will not screen.
Mar 15, 2020, 10:10 PM
HIGHBREAD - C’EST LA WEED Jayesh Jaidka Boston, MA
Screened Saturday March 14
A student taking the MCATs has a revelation despite his parents protestations.
Mar 15, 2020, 11:46 AM
LIE LIKE ME John Lewis James Vancouver, BC
Screened Wednesday March 11
A girl assumes the identity of her classmate.
Mar 11, 2020, 10:18 PM
DIAGNOSIS Tanner Craft Kirkwood, MO
Screened Tuesday March 10
A single mother struggles to cope as she tries to get help for her autistic son.
Mar 11, 2020, 12:55 AM
RIVENSE Amelia Spilger Fairfax, CA
Screened Tuesday March 10
A upstart coffee family from Costa Rica tries to make the right connections in the USA.
Mar 10, 2020, 8:50 PM
SLOW JAM GIRL Jack Quint New York, NY
Screened Monday March 9
The second coming of Weird Al Yankovic
Mar 9, 2020, 11:09 PM
FICTION ABOUT COUNTRY C Xueqing Yin Nanchang, Jiangxi, China
Screened Monday March 9
A woman encounters resistance in her quest for a baby via in vitro fertilization.
Mar 9, 2020, 11:06 PM Transparent Film Festival updated their status. We will be adding two awards categories:
BEST SUPPORTING ACTOR BEST SUPPORTING ACTRESS
Mar 9, 2020, 12:29 AM
SILENCE Cathy HC New York, NY
Screened Sunday March 8
When kids grow up around screaming parents...
Mar 9, 2020, 12:06 AM
HOEK’S DELTA Vadim Lasca Caracas, Venezuela
Screened Sunday March 8
A day spent with friends goes horribly wrong after they head home.
Mar 8, 2020, 11:50 PM
ALREADY LUCKY Matthew Cassani Los Angeles, CA
Screened Sunday March 8
Sometimes getting high can make your problems go away, and sometimes they can’t.
Mar 8, 2020, 6:24 PM
BROKEN HALOS Michael Kampa Myrtle Beach, SC
Screened Sunday March 8
After a cop loses his partner, supernatural events occur leading him to seek justice and redemption.
Mar 8, 2020, 2:42 PM
VEGETABLE SKIN, AN INTERVIEW Giovanni Soletta Codrongianos, IT Screened Sunday March 8 A moving portrait of the artist Bruno Petretto. Mar 8, 2020, 1:46 PM
STRAIGHT AND NARROW Jesse Hutchins Dubois, PA
Screened Sunday March 8
A musician on the rebound can’t get out of his own way.
Mar 8, 2020, 1:23 PM
ODYSSEUS Sofia-Chryssanthi Touboura Thessaloniki, GR Screened Sunday March 8 Somber visual art piece about off shore drilling. Mar 8, 2020, 1:10 PM
CRAZY (MUSIC VIDEO) Lee Milby (Artist J-Key) New York
Screened Saturday March 7
Is she cheating on him, or is he imagining things?
Mar 7, 2020, 8:07 PM
THE FORMERLY SESSION Cherry Fu Queens NY
Screened Friday March 6
A young woman must take desperate measures to free herself from her controlling boyfriend.
Mar 7, 2020, 2:41 AM Click for video:
Transparent Film Presents Episode 24 - Pool with special guest Director Dylan Bank. 6 of the films that have been selected for this year's Transparent Film Festival. Music Performance by Hall & Oates. Transparent Film Presents Episode 24 - Pool with special guest Director Dylan Bank.
6 of the films that have been selected for this year's Transparent Film Festival. Music Performance by Hall & Oates.
Mar 7, 2020, 1:43 AM Transparent Film Festival premiered a video. Transparent Film Presents Episode 24 - Pool with special guest Director Dylan Bank.
6 of the films that have been selected for this year's Transparent Film Festival. Music Performance by Hall & Oates.
Mar 7, 2020, 1:28 AM Click for video:
Transparent Film Presents Episode 24 - Pool with special guest Director Dylan Bank. 6 of the films that have been selected for this year's Transparent Film Festival. Music Performance by Hall & Oates. Transparent Film Presents Episode 24 - Pool with special guest Director Dylan Bank.
6 of the films that have been selected for this year's Transparent Film Festival. Music Performance by Hall & Oates. Mar 7, 2020, 1:28 AM Transparent Film Festival updated their status. NOTICE TO ALL FILMMAKERS:
The first selections have been announced.
We keep getting emails from some of you mistakenly thinking that your film has already screened, like in some online festival.
When we do a post about your film, with stills, and a screened on day and date, that is just the proof of our having watched (or “screened”), your film.
What sets us apart from every other festival (and why we are a top 100 festival on Film Freeway), is because we can document when we watched your film. If you want, you can cross reference with Vimeo or YouTube analytics for that day, so you can see we watched your entire film.
Generally, the more screen grabs, the more we liked your film. Though this is not always the case.
The festival itself is on Friday night, April 3rd, and Saturday night April 4 with a party after the Awards Ceremony.
Selections will first be announced on the Friday night March 20 episode of Transparent film Festival presents.
Lastly, if you’re not already, you may want to follow this page. Important information as well as announcements will be posted here especially as we draw closer to the festival.
Mar 6, 2020, 5:18 PM
FLAMBOYANES Javier Montes d'Arce Astoria, NY
Screened Thursday March 5
A man finds a way to spend time with his deceased wife.
Mar 6, 2020, 2:17 AM
BACKGROUND NOISE (FEATURE) Robert Brooks Portland, OR
Screened Thursday March 5
Strange goings on off campus in the Northwest... not too far from Twin Peaks.
Mar 6, 2020, 12:03 AM
THE SANTA CRUISE Maxwell Mueller Brooklyn, NY
Screened Sunday March 1
A group of would be Santas go on an Alaskan cruise to learn how to be Santa.
Mar 1, 2020, 10:14 PM
BACKBEAT Martin Blank Bethesda, MD
Screened Sunday March 1
It’s tough being a musician.
Mar 1, 2020, 9:55 PM
IMMORTALITY Ian Graziano Greenlawn, NY
Screened Sunday March 1
Something has a hold of this girl.
Mar 1, 2020, 9:44 PM
BORROWING Joe Stramowski Brooklyn, NY
Screened Sunday March 1
A women struggles with her infertility, which leads her to a dark place.
Mar 1, 2020, 9:34 PM
VORACIOUS Gabriel Chiriboga Brooklyn, NY
Screened Sunday March 1
Not a good idea to walk around an industrial part of Brooklyn after hours.
Mar 1, 2020, 8:50 PM
CONOCER Joey Doyle New York, NY
Screened Sunday February 23
Two men learning Spanish become acquainted with each other’s personal stories.
Feb 23, 2020, 3:40 PM
INDIANA JONES AND THE SECRET OF THE QUEENS GIANT John Galligan Queens, NY
Screened Sunday February 23
A lost installment of the treasure hunter saga which takes place in the most unlikeliest of locations.
Feb 23, 2020, 2:32 PM
HELP ME Jim Morton Erie, PA
Screened Sunday February 23
A soldier suffering from the effects of PTSD can’t save his wife from...
Feb 23, 2020, 1:46 PM
TABLEAUX Wyatt D'Emilia New York, NY
Screened Sunday February 23
A painting sews discord amongst all who view it.
Feb 23, 2020, 1:25 PM Transparent Film Festival premiered a video. From No Mystery Studios in NYC. Clips from films including: Sisters of Sorrow; Stuck On Probation; After You've Gone; Killing Time; A Ship Of Human Skin. Special Musical Guest - Esa "Like Meat"
Feb 22, 2020, 1:30 AM Click for video:
From No Mystery Studios in NYC. Clips from films including: Sisters of Sorrow; Stuck On Probation; Killing Time; After You've Gone. Special Musical Guest - Esa "Like Meat" From No Mystery Studios in NYC. Clips from films including: Sisters of Sorrow; Stuck On Probation; Killing Time; After You've Gone. Special Musical Guest - Esa "Like Meat" Feb 22, 2020, 1:29 AM
Tonight at 12:30 am NYC time, Transparent Film Festival Presents Episode 23. Sisters of Sorrow Stuck On Probation After You’ve Gone Killing Time A Ship Of Human Skin Special Musical Guest: Mariella Pacey “Like Meat” Click Here: https://www.mnn.org/watch/channels/community-channel Feb 21, 2020, 8:31 PM Transparent Film Festival posted an offer.
https://filmfreeway.com/TransparentFilmFestival/tickets https://filmfreeway.com/TransparentFilmFestival/tickets
Feb 18, 2020, 11:10 PM
GOOD INTENTIONS Simone Kisiel New York, NY
Screened Monday February 17
A mother woman who seemingly has everything, makes one bad decision and loses it, all...
Feb 17, 2020, 10:26 PM
AFTER YOU’VE GONE Emily Olcott Brooklyn, NY
A painter has difficulty coming to terms with the loss of his sister.
Feb 17, 2020, 9:41 PM
Some of the films that are now being screened in the second round....
To remind, our judges are film veterans and collectively have either an Oscar, an Emmy, and/or a Grammy win or nomination.
We will begin revealing some of our selections on this Friday’s episode of Transparent Film Festival Presents (Feb. 21); and subsequently for the four weeks that follow. The full festival schedule will be revealed on the Friday March 20th episode.
Feb 17, 2020, 12:42 AM
KILLING TIME Cody Lovorn San Antonio, TX
Screened Sunday February 16
After the death of the woman he loves, a man with a special gift grapples with killing himself.
Feb 16, 2020, 11:50 PM Go here now! Episode 22
https://www.mnn.org/watch/channels/community-channel Updated Feb 15, 2020, 12:30 AM
Feb 15, 2020, 12:30 AM
SISTERS OF SORROW Gerald Auger Vancouver, British Columbia
Screened Friday February 14
Native American Biker Babes track a thief.
Feb 14, 2020, 6:29 PM
BIRTH OF SPEED Werner Maritz Capetown, SA
Screened Sunday February 9
Portrait of two cheetah mothers and their cubs.
Feb 10, 2020, 12:25 AM
MAKE ME A BUTTERFLY Amy Barbera Coral Springs, FL
Screened Sunday February 9
A woman pays homage to her deceased grandmother.
Feb 9, 2020, 8:23 PM
PUNTA ALLEN Simon Keller Zurich, CH
Screened Sunday February 9
Portrait of a sleepy Mexican seaside village.
Feb 9, 2020, 7:53 PM
THE STRANGER MANUEL Enzo Cellucci New York, NY Screened Sunday February 9 A man washes up on a beach and encounters a native. Feb 9, 2020, 7:37 PM
LIKE MEAT Mariella Pacey Queensland, AU
Screened Wednesday February 5
A group of dancers will do anything to succeed, including eating each other.
Feb 6, 2020, 12:58 AM
CROSSROADS Ari Hock Seattle, WA Screened Wednesday February 5 A man grapples with retirement. Feb 5, 2020, 11:59 PM More Great Films and Special Music Guest Lenny Kaye!
https://www.transparentfilmfestival.com/nyc-television-show/videos/transparent-film-festival-presents-episode-22-snow
Support True Independent Film. Submit at filmfreeway.com/transparentfilmfestival Updated Feb 5, 2020, 9:21 AM
Feb 5, 2020, 9:21 AM Click for video:
Transparent Film Festival Presents 2020 Trailer Transparent Film Festival Presents 2020 Trailer
Feb 4, 2020, 7:51 PM
Feb 1, 2020, 11:31 PM
Received this email today. We're very proud to announce that we're a Top 100 Festival on Film Freeway. Received this email today. We're very proud to announce that we're a Top 100 Festival on Film Freeway.
Feb 1, 2020, 10:35 PM
ADA Steven Kammerer Vancouver, B.C.
Screened Thursday January 30
A visionary struggles to bring her invention to market in 1850’s London.
Jan 30, 2020, 11:11 AM
HOLISTIC HEALTH COMMUNITY Cornelia Wathen Stone Ridge, NY
Screened Sunday January 26
A small town’s communal approach to health care.
Jan 26, 2020, 11:44 PM
COFFEE AND A DONUT Cary Patrick Martin Astoria, NY
Screened Sunday January 26
A man’s limited knowledge of English keeps him from ordering the food he wants.
Jan 26, 2020, 11:33 PM
AFTER LIFE Mike Cerisano Brooklyn, NY
Screened Thursday January 23
It’s ghosts versus ghost hunters in this paranormal version of “The Office”.
Jan 24, 2020, 12:06 AM
ASSISTANCE Christian Gillis Greenwich, CT
Screened Wednesday January 22
One more reason to not work at a convenience store.
Jan 23, 2020, 12:21 AM
I DO Gianni McLaughlin Hoboken, NJ
Screened Wednesday January 22
She’s nuts. He’s worse. Sounds like the perfect marriage.
Jan 23, 2020, 12:01 AM Click for video:
One of our most popular episodes of Transparent Film Festival Presents: Episode 21 - Apple Clips from: DML (UK), Reyna (Baltimore, MD), The Meeting (Sicily), The Trouble With Cats (Redwood City, CA), The Dream Cafe (NYC); Special Musical Guest Ivan Anderson See past episodes at www.transparentfilmfestival.com One of our most popular episodes of Transparent Film Festival Presents: Episode 21 - Apple
Clips from: DML (UK), Reyna (Baltimore, MD), The Meeting (Sicily), The Trouble With Cats (Redwood City, CA), The Dream Cafe (NYC); Special Musical Guest Ivan Anderson
See past episodes at www.transparentfilmfestival.com
Jan 21, 2020, 11:36 AM
STUCK ON PROBATION Larry Hardison New York, NY
Screened Saturday January 18
The wacky lives of probation officers.
Jan 19, 2020, 12:17 AM
MLK JR. BLVD. Kareem McMichael Savannah, GA
Screened Saturday January 18
A young man with a chip on his shoulder falls victim to the odds against him.
Jan 18, 2020, 11:29 PM
GARAGE Phil E. Eichinger Rahway, NJ
Screened Saturday January 18
A man has mixed emotions when it falls to him to clean out his father’s garage.
Jan 18, 2020, 10:56 PM
A MANHATTAN LOVE STORY Jim Mendrinos New York, NY
Screened Friday January 17
Boy meets girl, then another girl.
Jan 17, 2020, 10:59 PM
Happily Married After Alison Guessou Golden Valley, Minnesota
Screened Sunday January 12
Secrets and dishonesty are the secret to this couple's happiness.
Jan 12, 2020, 6:32 PM
Mythomaniac Ricky Scott New York, NY Screened Sunday January 12 Fueled by her need for attention, a girl becomes detached from reality. Mythomaniac Ricky Scott New York, NY
Screened Sunday January 12
Fueled by her need for attention, a girl becomes detached from reality.
Jan 12, 2020, 6:00 PM
Tonight in 20 minutes, the 20th Episode of Transparent Film Festival Presents. The first episode of 2020! CLICK HERE!!!! https://www.mnn.org/watch/channels/community-channel Tonight in 20 minutes, the 20th Episode of Transparent Film Festival Presents. The first episode of 2020!
CLICK HERE!!!! https://www.mnn.org/watch/channels/community-channel
Jan 11, 2020, 12:09 AM
REYNA Akinola Hassan Laurel, MD
Screened Friday January 10
Baltimore Crime Caper where nothing goes the way it’s supposed to.
Jan 10, 2020, 10:34 PM
HIGH MAINTENANCE Michael McNeil Savannah, GA
Screened Thursday January 9
A man drags his friend out to hear about his bad breakfast experience.
Jan 9, 2020, 12:20 PM
THE TROUBLE WITH CATS Tim Schafer Los Angeles, CA
Screened Sunday January 5
A young woman has the worst luck with dating.
Jan 6, 2020, 12:28 AM
DREAM CAFE Darya Khritankova (NYC) Siberia, RU
Screened Saturday January 4
A man goes to extraordinary lengths to make his dreams come true.
Jan 4, 2020, 1:18 PM Transparent Film Festival updated their status. NOTE
No selections have been made. Please do not confuse our confirmation of screening with being selected.
Jan 4, 2020, 11:03 AM
CRUSHER Arshia Zeinali Rakesh Gilman, Iran
Screened Friday January 3
An out of work laborer decides he wants to go out in a blaze of glory.
Jan 4, 2020, 1:38 AM Transparent Film Festival updated their status. Clips from Angels Gone; House Of Redemption; Ketu; Romanace and Forgotten Alive; Music guests The On and Ons; posted from the Metropolitan Opera!
Jan 4, 2020, 12:37 AM Tune in now (Every Friday Night at 12:30 EST)
https://www.mnn.org/watch/channels/community-channel Updated Jan 4, 2020, 12:29 AM
Jan 4, 2020, 12:29 AM
PLASTIC BOY (FEATURE) Sam Plommer London, UK
Screened Friday January 3
Despite one bad break after the next, a young man rolls with the punches.
Jan 3, 2020, 11:34 PM Transparent Film Festival updated their status. IMPORTANT
We just had to disqualify (for the first time) a submission that included questionable material (and that could be considered illegal in some jurisdictions).
If we have no way to verify you are a real person, cannot find you online, and have no information about you and/or your project other than an email address, your submission will be disqualified.
Jan 3, 2020, 6:01 PM
FULL CIRCLE (FEATURE) Noel Brady Dublin, Ireland
Screened Friday January 3
A war journalist whose credibility is suspect stumbles onto the story of a lifetime.
Jan 3, 2020, 5:28 PM
SLIP AWAY Thais Sherell Edison, NJ
Screened Friday January 3
A woman struggles with depression.
Jan 3, 2020, 5:03 PM
MAMA KNOWS Guy Kapulnik Los Angeles, CA Screened Wednesday January 1 A new breed of Advice Columnist has plenty to say. Jan 2, 2020, 12:55 AM
TWENTY TWENTY Nicholas M. Garofolo/Dave Sweeney Brooklyn, NY
Screened Wednesday Jan. 1
A writer fights off attempts by evil forces to turn him into a Manchurian candidate.
Jan 2, 2020, 12:46 AM
HIDDEN STILL Monica Goldberg Sydney, AU Screened Tuesday December 31 Artistic homage to a grandfather who went missing. Dec 31, 2019, 7:38 PM
BEND LOW, SWEET BRANCH, BEND LOW The Gentlemen Losers Helsinki, FIN
Screened Sunday December 29
If shucking oysters were this sexy everyone would be doing it.
Dec 29, 2019, 11:23 PM
ANGELS GONE Roger Salazar Floral Park, NY
Screened Sunday December 29
A restaurant manager finds himself in the midst of what appears to be a worldwide paranormal event.
Dec 29, 2019, 10:26 PM
AFTER THE FLOOD Alexandra Guillossou St. Louis, MO
Screened Sunday December 29
Two floods sweep through St. Louis as seen through the eyes of a teenager.
Dec 29, 2019, 10:04 PM
ROMANCE Giorgi Tkemaladze Tbilisi GE
Screened Wednesday December 25
A father resorts to extreme measures when he gets wind of his daughter’s romance.
Dec 25, 2019, 11:55 PM
WHIRLPOOL Amir Taghavi Tehran, IR Screened Wednesday December 25 Running to, running from, in the end we’ll be overcome. Dec 25, 2019, 11:08 PM
LUPERCA RETURNS Alexis Ramirez Bronx, NY
Screened Wednesday December 25
A monster whose powers have faded has a chance encounter with an alien.
Dec 25, 2019, 10:54 PM
THE INTERNING Samrat Chakrabarti New York, NY
Screened Wednesday December 25
Political correctness threatens to destroy a young man’s life.
Dec 25, 2019, 6:53 PM
KETU Anderia Noshad Tehran, IR
Screened Friday December 20
Metal Music Meets Modern Dance
Dec 21, 2019, 1:02 AM Transparent Film Festival posted in Independent Horror Filmmakers. Transparent Film Festivalfacebook.com If a filmmaker requests to know why they were accepted or rejected, it shouldn't be too hard to provide a reason. That is what makes us different than most festivals, and why our first year was a HUGE success.
We screened every frame of every film and returned our detailed notes. Please see our screening receipts at [http://Facebook.com/transparentfilmfestival](https://www.facebook.com/transparentfilmfestival/?ref=gs&__tn__=%2CdK-R-R&eid=ARD1ZCrsZ_JQzKWKQOkk3JePtiVszPuX53SvKGwJdQzrwc1tYyqy2Bltbo9TXQozbyPRuy6sJ99s9R4R&fref=gs&dti=334368756043&hc_location=group)
We also have a cable show every Friday Night on FIOS, Spectrum and RCN here in Manhattan where we spotlight the best submissions. We keep our fees low!
Inbox us to find out more or submit here: [http://filmfreeway.com/transparentfilmfestival](https://l.facebook.com/l.php?u=http%3A%2F%2Ffilmfreeway.com%2Ftransparentfilmfestival%3Ffbclid%3DIwAR3hTp78MkrcaTwYANmmTd17Qg5QDeh1Q57-z4YkzpAnePR7SbmMFMj2WHs&h=AT2EI1P7-S7cC-pKFqX3p6p70yxMgvr_pRUoQMA-VeYeoU4j3t2PBsXWBoT-O3LqkpmjFAy4W_CQkKjA-Nb5kv7KQuc6Nz4Ra0D50qzNtdC0UQ5_s7qDxv8rBFNj-hc8m0REcX7ZUslwr-lB8PAe65Zi0w6MEve-Jw)
Check out our streaming channel at [http://transparentfilmfestival.com/](http://transparentfilmfestival.com/?fbclid=IwAR2o2LYfbk7pvpwh9tZ4hOAyk_O6zez2y8MVG73insdXgSK-_0_Qdr4BRKs) Dec 20, 2019, 2:18 PM
VENGEFUL LOVE Alexandra Balda Caracas, VZ
Screened Wednesday December 18
Not the reaction you want when telling your husband you’re pregnant!
Dec 19, 2019, 12:43 AM Click for video:
Our Sponsor Joseph Victori Wines has a wine called "Sweet Bitch." It is a Chilean brand and it is DELICIOUS. It's sooooo good it's... well, just see for yourself! Our Sponsor Joseph Victori Wines has a wine called "Sweet Bitch." It is a Chilean brand and it is DELICIOUS. It's sooooo good it's... well, just see for yourself! Dec 16, 2019, 2:15 PM
THE PAIN, THE PARK, AND OTHER THINGS Jim Mendrinos New York, NY
Screened Saturday December 14
Two middle age men have different perspectives on aging.
Dec 15, 2019, 1:52 AM
LOST Elijah Rodriguez New York, NY
Screened Saturday December 14
A young women agonizes over her mother’s death.
Dec 14, 2019, 9:29 PM
A SUPER SURPRISE Pat Campo Los Angeles, CA
Screened Friday December 13
A group of boys is stalked in this mix between Scream and The Hangover.
Dec 14, 2019, 12:18 AM Click for video:
Episode 18 of Transparent Film Festival Presents: Clips from short films include Dance With A Demon, The Malfunction and Hysterium; Special Musical Performance by The Charlie Daniels Band Episode 18 of Transparent Film Festival Presents:
Clips from short films include Dance With A Demon, The Malfunction and Hysterium; Special Musical Performance by The Charlie Daniels Band Dec 11, 2019, 6:57 PM
Cat Scratch Fever Michael Volpe Washington DC
Screened Wednesday Dec. 11
Rather than loafing, this cat breaks it down old school.
Dec 11, 2019, 5:41 PM Transparent Film Festival posted in New York Filmmakers and Actors. Transparent Film Festivalfacebook.com If a filmmaker requests to know why they were accepted or rejected, it shouldn't be too hard to provide a reason. That is what makes us different than most festivals, and why our first year was a HUGE success.
We screened every frame of every film and returned our detailed notes. Please see our screening receipts at http://Facebook.com/transparentfilmfestival
We also have a cable show every Friday Night on FIOS, Spectrum and RCN here in Manhattan where we spotlight the best submissions. We keep our fees low!
Inbox us to find out more or submit here: http://filmfreeway.com/transparentfilmfestival
Check out our streaming channel at http://transparentfilmfestival.com/ Dec 10, 2019, 12:50 PM Transparent Film Festival posted in Asian Actors & Filmmakers Community. Transparent Film Festivalfacebook.com If a filmmaker requests to know why they were accepted or rejected, it shouldn't be too hard to provide a reason. That is what makes us different than most festivals, and why our first year was a HUGE success.
We screened every frame of every film and returned our detailed notes. Please see our screening receipts at http://Facebook.com/transparentfilmfestival
We also have a cable show every Friday Night on FIOS, Spectrum and RCN here in Manhattan where we spotlight the best submissions. We keep our fees low!
Inbox us to find out more or submit here:
http://filmfreeway.com/transparentfilmfestival
Check out our streaming channel at http://transparentfilmfestival.com/ Dec 10, 2019, 12:49 PM
IN THE NIGHT Stephanie Gene Newburgh, NY
Screened Tuesday December 3
A young girl takes off on a nightmarish ride through her dreams.
Dec 3, 2019, 10:46 PM
DINNER FOR SIX (FEATURE) Ryan Willer Manhattan Beach, CA
Screened Sunday November 24
One secret after the next comes out after a dinner deteriorates into the mother of all bull sessions.
Nov 24, 2019, 1:56 PM
YES VIRGINIA Harvey Puttock London, ENG
Screened Sunday November 24
A woman with a gift no longer wishes it.
Nov 24, 2019, 1:05 PM
THE LAST HARVEST Alexis Spradic Watsonville, CA
Screened Saturday November 23
The dire need for a guest worker program is explored in this important documentary about the state of agriculture in the US.
Nov 23, 2019, 8:40 PM
MALFUNCTION Devan Prabhakar Allen, TX
Screened Saturday November 23
Just how real are you?
Nov 23, 2019, 7:59 PM https://m.youtube.com/watch?v=M1F0lBnsnkE&fbclid=IwAR1p1iKyn1_9byOZvK6WuOyV0j35NBa4vYhq6oYoHARxXHWt3Cn-zl5b8Kc Updated Nov 23, 2019, 12:26 AM
Nov 23, 2019, 12:26 AM
WHAT REMAINS Matthieu DUVAL Brettonneux, FR
Screened Monday November 18
A man suffers from PTSD and his family along with him.
Nov 19, 2019, 1:10 AM
THE RABBIT HOLE Richard Bakewell Burbank, CA
Screened Monday November 18
Daddy comes home from the war.... and brings the monsters with him.
Nov 19, 2019, 12:41 AM
NEVER TWICE THE SAME COLOR Guli Silberstein Tel Aviv, Israel
Screened Monday November 18
Ghostly images taken by a group of friends who move from Israel to New York shortly before 9/11
Nov 18, 2019, 12:52 PM
TEQUILA FLAVORED CIGARETTES Bobby McGruther New York, NY
Screened Monday November 18
Friends reunite to celebrate a birthday, but when alcohol is involved, anything can happen.
Nov 18, 2019, 12:10 PM
NEW TO THE NEIGHBORHOOD Aaron Slone Pikeville, KY
Screened Saturday November 16
House hunters run into a satanic cult.
Nov 16, 2019, 3:08 PM
U & ME Vamshi Srinivas Parsippany, NJ
Screened Saturday November 16
A man neglects his beautiful wife.
Nov 16, 2019, 2:46 PM
DANCE WITH A DEMON Mitch Bax Vancouver, BC
Screened Saturday November 16
Imaginative tale about a mother battling the demon of depression in the Neverworld.
Nov 16, 2019, 2:23 PM
WHERE THE SUNRISE SETS Nathaniel Avila San Antonio, TX
Screened Saturday November 16
A young woman sacrifices everything to help her mother escape an abusive marriage.
Nov 16, 2019, 1:50 PM
NAPS Martinique Watson Queens, NY
Screened Saturday November 16
A young woman makes a radical decision concerning her hair.
Nov 16, 2019, 12:57 PM
NICE TO MEET YOU Stefano Sgarbi Mirandola, IT
Screened Friday November 15
When it’s meant to be, it’s meant to be.
Nov 15, 2019, 8:33 PM
HYSTERIUM John Lofton IV Baltimore, MD
Screened Friday November 15
Everything was fine.... until her mother put down her poetry.
Nov 15, 2019, 8:01 PM
KUNG PAO CORPSE Zack Burkett Atlanta, GA
Screened Friday November 15
Even the dead love Chinese food.
Nov 15, 2019, 7:07 PM
WAKE FROM DEATH AND RETURN TO LIFE Andrew Ferrer Atlanta, GA
Screened Friday November 15
The setting is a Japanese garden as two women look to the future after the death of a loved one.
Nov 15, 2019, 6:32 PM
A FOREST BATH Bryce Saucier Houston, TX
Screened Friday November 15
Getting stood up by her “friend” may be the best thing that ever happened to this girl.
Nov 15, 2019, 6:10 PM
CLOUDS AND SHADOWS Andrew Payne Bedford, England Screened Friday November 15 Experimenting with light and shadows caused by passing clouds. Nov 15, 2019, 5:45 PM
DAGNY Bjørn Sortland Bergen, NO
Screened Friday November 15
A woman takes a bus to the sanitarium.
Nov 15, 2019, 4:52 PM
HOW MUCH? Heath Bernstein Los Angeles, CA
Screened Friday November 15
Two friends challenge one another with hypotheticals.
Nov 15, 2019, 4:32 PM
DONE WAITING Jesse Hutchins Pittsburgh, PA
Screened Sunday November 10
When the cops can’t help, a man takes matters into his own hands in an attempt to rescue his wife.
Nov 10, 2019, 11:25 PM
THE NORM Jamal Bilal New York, NY
Screened Sunday November 10
A hospice nurse does her best to cope and help others.
Nov 10, 2019, 10:30 PM
THE TELLTALE WATCH Nima Nabavinejad Melville, NY
Screened Sunday November 10
A homeless man is murdered for his watch.
Nov 10, 2019, 9:49 PM
JUMBLED Ricky Scott Independence, LA Screened Saturday November 9 Angels and demons compete for a young man’s soul. Nov 10, 2019, 2:19 PM
LIVING CONDITIONS Jacob R. Colon Linden, NJ
Screened Saturday November 9
A man squares off with his super who is overwhelmed.
Nov 10, 2019, 2:11 PM
SERI Violetta Licari Palm Coast, FL Screened Saturday November 9 A family grieves for a loved one who has passed. Nov 10, 2019, 2:07 PM Today only!
https://vimeo.com/221890757/73c52a2a8a Updated Nov 7, 2019, 11:34 AM
Nov 7, 2019, 11:34 AM
Watch live from NYC in 15 Min!! https://www.mnn.org/watch/channels/community-channel Nov 2, 2019, 12:15 AM
FOR THE GOBLINS Yuan Wang Burbank, CA
Screened Friday November 1
A man agrees to train a goblin in exchange for some magic wine.
Nov 1, 2019, 5:49 PM
PLEDGE IT Rachel Sonnenberg Tenafly, NJ
Screened Thurs. October 31
A teacher has a meltdown when kids decide they don't want to recite the Pledge of Allegiance.
Oct 31, 2019, 4:15 PM
Filtered Scotty Morris Hamilton, Ontario
Screened Thursday Oct. 31
Two teenager girls go after each other as seen from a mobile phone.
Oct 31, 2019, 2:55 PM
BrLooklyn Maria T Alvarado Brooklyn, NY
Screened Monday Oct. 31
A girl wanders around Brooklyn looking for a friend.
Oct 31, 2019, 2:25 PM
FAMILY BOND Chris Guzzo Staten Island, NY
Screened Monday October 21
A woman disappears from her family but her husband tracks her down...
Oct 21, 2019, 11:13 PM
RESTLESS Ela Acur Oakland Gardens, NY
Screened Sunday October 21
A young woman struggles to fight off a demon after the death of her mother.
Oct 21, 2019, 1:35 AM
YES (Feature) Tim Realbuto Brooklyn, NY
Screened Sunday Oct. 21
A acting teacher digs dangerously deep to bring out the best in his student.
Oct 21, 2019, 1:02 AM
HEY DREAMER David Erba Brooklyn NY
Screened Sunday October 20
Seemingly unconnected people try to make the world a better place.
Oct 20, 2019, 12:42 PM
APPLECALYPSE Nobuhiro Futaki Brooklyn, NY
Screened Friday October 18
A scientist realizes he’s been hired to work on a bio-weapon.
Oct 18, 2019, 7:43 PM
THE TEA Levi Morris Los Angeles, CA
Screened Wednesday October 16
BBC like period piece spoof.
Oct 16, 2019, 11:53 PM
DROWNING Valerio Casali New York, NY
Screened Monday October 14
A screenwriter has a meltdown.
Oct 14, 2019, 3:24 PM Click for video:
For Those of you who missed it, Episode 13 of Transparent Film Festival Presents. Films this week include clips from: "You Look Great", "Married and Loving It!, and "Dead Water"; Special music guest Jelly Bean with premiere of "Astronaut". From Comic Con in New York City. For Those of you who missed it, Episode 13 of Transparent Film Festival Presents.
Films this week include clips from: "You Look Great", "Married and Loving It!, and "Dead Water"; Special music guest Jelly Bean with premiere of "Astronaut".
From Comic Con in New York City. Oct 13, 2019, 12:05 AM Go here now https://www.mnn.org/watch/channels/community-channel Updated Oct 12, 2019, 12:32 AM
Oct 12, 2019, 12:32 AM
PLATFORMS Kayla Arendts Agoura Hills, CA
Screened Friday October 11
A young woman has to decide if she’s going to get serious about dancing.
Oct 11, 2019, 7:31 PM
THE TOYMAN Rachael Sonnenberg Tenafly, NJ
Screened Friday October 11
There are, apparently, still a few kids left who play with actual toys (that occasionally need fixing).
Oct 11, 2019, 6:21 PM
THE FALL Ben Senior Winnetka, IL
Screened Friday October 11
Moody coming of age tale about two teens (one of whom is suffering from mental illness) who fall in love.
Oct 11, 2019, 6:10 PM
Tonight on Transparent Film Festival Presents:
Clips from: You Look Great, Married and Loving It; Dead Water. Special Musical Guest Jelly Bean.
On Manhattan cable - FIOS Ch. 33; Spectrum Ch. 34 and RCN Ch. 82
See it stream live from Manhattan at 12:30 am NYC time: https://www.mnn.org/watch/channels/community-channel
Hosted from Carnegie Hall, Yankee Stadium and Comic Con. Extra Special guest Mark Franzblau.
Oct 11, 2019, 4:54 PM
ASTRONAUT Pierre Petrovsky Nord, France
Screened Monday October 7
Retro Anime a la 80’s Music Video
Oct 8, 2019, 1:13 AM
THE CHERRY TREE CAROL Barbara West Davis, CA
Screened Sunday October 6
A version of the immaculate conception you most likely haven’t yet heard.
Oct 7, 2019, 1:59 AM
MARRIED AND LOVING IT Ryan McDonald Ogden, UT
Screened Sunday October 6
A look at married life through the eyes of four young couples.
Oct 7, 2019, 1:41 AM You tagged Mark A. Franzblau
Quite a thrill to meet Nichelle Nichols today. That’s Mark Franzblau to the right. Oct 6, 2019, 10:36 PM
FORTUNE COOKIES AND MEN’S EYES Al Sutton New York, NY
Screened Sunday October 6
A couple runs into God and his secretary making fortune cookies in the back of a Chinese restaurant.
Oct 6, 2019, 10:33 PM
HAPPY BIRTHDAY Al Sutton New York
Screened Saturday October 5
Parents are concerned about their daughter who is living in New York.
Oct 5, 2019, 11:53 PM
THE WRECKER Li Geqi China
Screened Saturday October 5
Gangsters come in all shapes and sizes.
Oct 5, 2019, 11:33 PM Click for video:
7 Day Free Trial at transparentfilmfestival.com 7 Day Free Trial at transparentfilmfestival.com
Sep 29, 2019, 11:40 PM
Attn: Filmmakers: Please introduce yourself by clicking the link below. So many of you are so talented, that the idea is for all of you to be exchanging ideas, networking and possibly working together. https://www.transparentfilmfestival.com/forums/general/36663-filmmaker-rolecall Attn: Filmmakers: Please introduce yourself by clicking the link below.
So many of you are so talented, that the idea is for all of you to be exchanging ideas, networking and possibly working together.
https://www.transparentfilmfestival.com/forums/general/36663-filmmaker-rolecall
Sep 29, 2019, 7:53 PM
OWN YOUR STUPIDITY Sids Ahky Stevens Brooklyn, NY
Screened Sunday September 29
A girl faces long odds and an uphill battle on her way to find happiness.
Sep 29, 2019, 1:52 PM
CENTER ICE Nichole Huenergardt Sherman Oaks, CA
Screened Saturday September 28
Documentary about Competitive Team Figuring Skating
Sep 28, 2019, 4:02 PM
FORGOTTEN ALIVE Kiriakos Kotsinis Greece
Screened Saturday September 28
A mystery unfolds after a man escapes from his grave
Sep 28, 2019, 1:53 PM Click for video:
Promoting amazing indie filmmakers from around the world. Go to www.transparentfilmfestival.com and GET YOUR 7 DAY FREE TRIAL! Promoting amazing indie filmmakers from around the world. Go to www.transparentfilmfestival.com and GET YOUR 7 DAY FREE TRIAL! Sep 28, 2019, 1:13 AM
MILLENNIAL SUCCESS STORIES: FE-MALE CEO Rachel Annette Helson Brooklyn, NY
Screened Friday September 27
A business/life coach gives three aspiring woman business owners a crash course in what it takes to make it big!
Sep 28, 2019, 12:20 AM
ZOMBIE SHARKAGEDDON II John Cunningham Melbourne, AU Screened Friday September 27 A man gets carried away with a video game. Sep 27, 2019, 10:40 PM
Promoting TRUE INDEPENDENT FILM. From Hong Kong to Iran, Sweden to Brooklyn, we spotlight amazing filmmakers from around the world. 1st 12 episodes of Transparent Film Festival Presents available online now. Click www.transparentfilmfestival.com for 7 DAY FREE TRIAL Sep 27, 2019, 5:13 PM Transparent Film Festival posted in Independent Horror Filmmakers.
Transparent Film Festival's First Digital Release!!! The Killer's Requiem (91 min) Starring Tim Russ (Star Trek Voyager), Lloyd Kaufman (Toxic Avenger), George Hardy (Troll 2), and Jean Beauvoir (Plasmatics) Lots of great extras!!! https://www.transparentfilmfestival.com/products/the-killer-s-requiem Sep 26, 2019, 11:31 AM
Transparent Film Festival's First Digital Release!!! The Killer's Requiem (91 min) Starring Tim Russ (Star Trek Voyager), Lloyd Kaufman (Toxic Avenger), George Hardy (Troll 2), and Jean Beauvoir (Plasmatics) Lots of great extras!!! https://www.transparentfilmfestival.com/products/the-killer-s-requiem Transparent Film Festival's First Digital Release!!!
The Killer's Requiem (91 min) Starring Tim Russ (Star Trek Voyager), Lloyd Kaufman (Toxic Avenger), George Hardy (Troll 2), and Jean Beauvoir (Plasmatics)
Lots of great extras!!!
https://www.transparentfilmfestival.com/products/the-killer-s-requiem
Sep 26, 2019, 9:38 AM
MOTHERLAND Baset Zandi Tehran, Iran
Screened Sunday September 22
Dark drama about a man who wakes from a coma only to return home and find out that nothing is the same.
Sep 22, 2019, 11:41 PM
THE LIMBO CAFE Jason Burke Pittsburgh, PA
Screened Sunday September 22
Those who’ve committed unspeakable acts wind up here.
Sep 22, 2019, 2:39 PM
THROUGH THE EYES OF A WRESTLER Veronica White Coraopolis, PA
Screened Friday September 20
An up and coming wrestler on the indie circuit talks about the life.
Sep 21, 2019, 12:52 AM
THE ELEPHANTS Olle Christiansen Meijer Skurup, Skåne, Sweden
Screened Thursday Sept. 19
4 seemingly unrelated vignettes that have one thing in common... feeling unwanted.
Sep 19, 2019, 3:30 PM
MOTHER'S PRESENCE J. Alexander Fernandez Clearwater, FL
Screened Thursday Sept. 19
A young man seeks revenge on his father's mistress.
Sep 19, 2019, 3:03 PM
YOU LOOK GREAT Justin Andrew Davis New York, NY
Screened Wednesday September 18
A young man’s eating disorder turns into a living nightmare.
Sep 18, 2019, 10:55 PM
THIRST Wylie Rush New York, NY
Screened Wednesday September 18
I guy gets more than he bargained for when he brings two girls home to party.
Sep 18, 2019, 10:17 PM
THE BLUE MARBLE Ralph Toporoff New York, NY
Screened Saturday September 14
A group of souls await their planetary assignments after the big bang, but are held up by bureaucratic red tape.
Sep 14, 2019, 9:50 PM
THE TENT Kyle Couch Pontiac, MI
Screened Saturday September 14
A Father and his daughter battle monsters on the fringes of a post apocalyptic world.
Sep 14, 2019, 9:07 PM
DISINTEGRANTS John Harlan Norris Lexington, KY
Screened Saturday September 14
The concept of the portrait reimagined.
Sep 14, 2019, 3:07 PM
DREAM NO. 37,091 Jon Reitzel Brooklyn, NY
Screened Friday Sept. 13
Offbeat characters living in a non-linear reality that looks a little like Brooklyn.
Sep 13, 2019, 4:06 PM Tonight at Midnight NYC time:
From Eastern Tennessee
https://www.mnn.org/watch/channels/lifestyle-channel Updated Sep 12, 2019, 10:44 PM
Sep 12, 2019, 10:44 PM Wow....
https://m.youtube.com/watch?v=4de-JbJHg7E&feature=youtu.be Updated Sep 12, 2019, 12:27 AM
Sep 12, 2019, 12:27 AM FORUMS ARE NOW OPEN! https://www.transparentfilmfestival.com/forums/general/36663-filmmaker-rolecall If you have submitted to The Transparent Film Festival, please check in on the site as we're going to be introducing many filmmaker resources. Updated Sep 8, 2019, 11:00 PM
Sep 8, 2019, 11:00 PM Click for video:
THE ANGRY MAN Melissa Malone Weehawken, NJ Screened Sunday September 8 A woman struggles to get over a childhood trauma only to find it’s starting again... THE ANGRY MAN Melissa Malone Weehawken, NJ
Screened Sunday September 8
A woman struggles to get over a childhood trauma only to find it’s starting again...
Sep 8, 2019, 11:36 AM
THE PEOPLE’S PYRAMID Buby Demetrio Coconut Creek, FL Screened Saturday September 7 The best and worst of humanity encapsulated in a geometric scrawl Sep 7, 2019, 7:40 PM
STOP REQUESTED Nick Philomeno Brazil Screened Saturday September 7 A heartbroken girl is reminded about how much she is loved. Sep 7, 2019, 7:24 PM
SHOWING UP Neil Fennell New York, NY
Screened Saturday September 7
After a funeral, a woman gets a surprise visit from a former love.
Sep 7, 2019, 7:14 PM Transparent Film Festival posted an offer.
You can see the first 11 episodes of Transparent Film Festival Presents (our cable television show) here: https://www.transparentfilmfestival.com/manhattan-cable-tv-show-thursday-night-midnight The Season 1 Finale is tonight at Midnight EST (NY time). You can watch online at https://www.mnn.org/watch/channels/lifestyle-channel The cable show is free to all. Subscribe to our streaming channel at TransparentFilmFestival.com for the best in independent film and music. You can see the first 11 episodes of Transparent Film Festival Presents (our cable television show) here: https://www.transparentfilmfestival.com/manhattan-cable-tv-show-thursday-night-midnight
The Season 1 Finale is tonight at Midnight EST (NY time). You can watch online at https://www.mnn.org/watch/channels/lifestyle-channel
The cable show is free to all. Subscribe to our streaming channel at TransparentFilmFestival.com for the best in independent film and music.
Sep 5, 2019, 4:39 PM
Last week's show hosted from Dollywood and in Eastern TN. https://www.transparentfilmfestival.com/manhattan-cable-tv-show-thursday-night-midnight/videos/transparent-film-festival-presents-episode-11-dolly Last week's show hosted from Dollywood and in Eastern TN.
https://www.transparentfilmfestival.com/manhattan-cable-tv-show-thursday-night-midnight/videos/transparent-film-festival-presents-episode-11-dolly
Sep 5, 2019, 4:12 PM Can’t stop watching this video. Cost: $500
https://m.youtube.com/watch?v=_0zb9D1lndg Updated Sep 5, 2019, 10:13 AM
Sep 5, 2019, 10:13 AM
EDDY 3.0 John Perivolaris Glasgow, Scotland
Screened Monday Sept. 2
A husband and the family robot do not get a long... at all.
Sep 3, 2019, 12:00 AM
POSITIVELY VENTING Milla Spivey Cupertino, CA
Screened Monday Sept. 2
The parents are having a hard enough time, but their worthless son is adding to their aggravation!
Sep 2, 2019, 11:34 PM
CHOLO Joe Saldana and Aaron Huisenfeldt Los Angeles, CA
Screened Sunday Sept. 1
An addict finds out he was betrayed by his gang.
Sep 1, 2019, 2:41 PM
THE DARK GOODBYE Mitch Ross Noblesville, IN
Screened Sunday Sept. 1
A good killer is one who can stop himself from killing.
Sep 1, 2019, 1:14 PM
HANBLOGLAKA Trier Guy Paris, France Screened Sunday Sept. 1 A Rorschach test set to techno music. What do you see? HANBLOGLAKA Trier Guy Paris, France
Screened Sunday Sept. 1
A Rorschach test set to techno music. What do you see?
Sep 1, 2019, 12:50 PM
SHIP OF HUMAN SKIN Richard Bailey Dallas, TX
Screened Monday August 26
Stranded in a rural nightmare of crime and drugs, two friends struggle to rid themselves of self inflicted torment.
Aug 27, 2019, 12:17 AM
PANIC ATTACK Eileen O'Meara West Hollywood, CA
Screened Sunday August 25
On her drive to work, a woman worries about EVERYTHING - only to realize it was for nothing.... or... was it😉
Aug 25, 2019, 9:53 PM
CREATION Nathaniel Mervar Fort Wayne, IN
Screened Sunday August 25
LEGO like film short about the story of creation.
Aug 25, 2019, 9:55 AM
THE BROADCAST Daria Sviridenko Moscow, Russian Federation
Screened Saturday August 24
A brutal coming of age tale as seen through the lenses of various mobile phones.
Aug 24, 2019, 7:25 PM
THE STRAIGHT MAN Paul Laudicano Middletown, NJ
Screened Saturday August 24
A once famous comedian decides to get back into show business with some help from an upstart.
Aug 24, 2019, 4:52 PM
LA HERENCIA DE MARTI Marc Pascual Dubai, United Arab Emirates
Screened Saturday August 24
A woman and her stepmother enter into a complicated relationship after the death of her father.
Aug 24, 2019, 12:08 PM
THE REDHEAD Natalie MacMahon Berlin, Germany
Screened Friday August 23
A young man trapped in a tedious call center job escapes to a fantasy world with beautiful maidens.
Aug 23, 2019, 4:40 PM Click for video:
In case you missed it - Season 2 Episode 3 of Transparent Film Festival Presents from Met Life Stadium: Films After (CA), Alter (Mexico) and Krampon (Turkey); special musical Guests The Rolling Stones In case you missed it - Season 2 Episode 3 of Transparent Film Festival Presents from Met Life Stadium: Films After (CA), Alter (Mexico) and Krampon (Turkey); special musical Guests The Rolling Stones Aug 23, 2019, 12:51 AM Transparent Film Festival updated their status. I’m still waiting for an explanation but according to Production, so many people logged in to watch it crash their server!
Aug 23, 2019, 12:24 AM Go here in 3 minutes
https://www.mnn.org/watch/channels/lifestyle-channel Updated Aug 22, 2019, 11:58 PM
Aug 22, 2019, 11:58 PM
Just a quick note. Selections will not be made until early next year. We’ve seen some great films but the competition is going to be fierce. Early bird submissions will be closing shortly. Aug 21, 2019, 11:18 AM
A MISSING PIECE Grace Chang Brooklyn, NY
Screened Sunday August 18
A single mother tries to teach her rapper son about the importance of tradition.
Aug 18, 2019, 10:50 PM
Like Nutella? This is much better for you, and the environment. Wanna know why check out Keepers Of The Forest (runner up for last season’s best documentary. Check out a clip at our website: http://transparentfilmfestival.com while we’re still offering a free trial. Aug 18, 2019, 10:24 AM
THE QUEEN’S ENGLISH Tyler Dwiggins New York, NY
Screened Sunday August 18
A quirky group of friends copes with the complexities of city living, while dealing with the complexities of their relationships.
Aug 18, 2019, 8:57 AM
ACTIVISM THROUGH ENLIGHTENMENT Rebecca Rauch-Thane Bar Harbour, FL
Screened Thursday Aug. 15
A young woman delves into Theravada Buddhism.
Aug 16, 2019, 12:31 AM
RISING FLAME Federico Carro La Spezia, Italy
Screened Wednesday August 14
A racing car mechanic fantasizes about winning the race and getting the girl.
Aug 14, 2019, 5:25 PM
DEAR OLD NEW WORLD Valentina Danelli/Alberto Mettuno Milan, Italy
Screened Tuesday August 13
I’m not sure what this film was about (no subtitles), but it’s fucking cool to look at!
Aug 13, 2019, 9:21 PM
MORANA Kathy Vargas Elmwood Park, NJ Screened Tuesday August 3 The effects of domestic violence are widespread. Aug 13, 2019, 9:01 PM
BEAUTY Zhang Xiaoan Melbourne. Australia
Screened Tuesday Aug. 13
A young woman struggles to cope with an ever changing socialized ideal of beauty.
Aug 13, 2019, 8:49 PM
REVELATION Elijah Rodriguez New York, NY
Screened Thursday August 8
A young woman tries to cope with the loss of a loved one by escaping into a virtual world.
Aug 8, 2019, 9:36 PM
POWER OUT Benjamin Myers New York, NY
Screened Thursday August 8
A get together takes an unexpected turn when NYC goes dark.
Aug 8, 2019, 9:16 PM
ALTER Marcos Obregón Mexico
Screened Sunday August 4
A young woman is stalked.
Aug 4, 2019, 11:41 PM
AFTER YOU Christelle Korichi Paris, FR
Screened Wednesday July 31
3 women struggle with domestic violence in their relationships.
Aug 1, 2019, 1:56 AM
BROKE Luke Bond New York, NY
Screened Sunday July 28
Uncomfortable portrait of a talented malcontent and the band she sabotages emotionally.
Jul 29, 2019, 1:53 AM
CUPID’S COMEBACK Monica Furman Brooklyn, NY
Screened Saturday July 27
Cupid and Venus are still at it in the age of dating apps.
Jul 28, 2019, 12:20 AM Go here now https://www.mnn.org/live/2-lifestyle-channel Updated Jul 26, 2019, 12:01 AM
Jul 26, 2019, 12:01 AM
Tonight - Midnight Eastern Time. (45 min from now)! On tonight’s episode, clips from Hello Baby (Lung Lee), The Holdout (Javier Augusto Nunez), and Losing (Sarah Allyn). Also Arshay Cooper from Row New York, Fashion Byblos Rowing Blazers; Music Guest Monique Sherrell Brown. https://www.mnn.org/live/2-lifestyle-channel Jul 25, 2019, 11:10 PM TONIGHT AT MIDNIGHT- EST
Arshay Cooper, Monique Sherrell Brown on https://www.mnn.org/live/2-lifestyle-channel Updated Jul 25, 2019, 8:52 PM
Jul 25, 2019, 8:52 PM
OUTSIDE (Daniel Laurent) Jeffrey Blake Palmer Providence, RI
Screened Wednesday July 24
Unique and lyrical approach towards the pitfalls awaiting black youth.
Jul 24, 2019, 1:50 PM
AFTER Joe Saldana / Aaron Huisenfeldt San Diego, CA
Screened Wednesday July 24
Friends and lovers wrapped up in murder and suicide.
Jul 24, 2019, 1:11 PM Click for video:
Every Thursday night at Midnight (EST - New York) our show streams live with new clips from films, musical guest, and New York stuff! Watch it online here: https://www.mnn.org/live/2-lifestyle-channel - If you miss it see it on www.transparentfilmfestival.com Every Thursday night at Midnight (EST - New York) our show streams live with new clips from films, musical guest, and New York stuff!
Watch it online here: https://www.mnn.org/live/2-lifestyle-channel - If you miss it see it on www.transparentfilmfestival.com
Jul 21, 2019, 11:39 PM Transparent Film Festival added a cover video. Click for video:
Jul 21, 2019, 11:34 PM
IMPORTANT ANNOUNCEMENT! 2019 was our 1st year and a HUGE success. Judging from what we are seeing for 2020, it's going to be even better with more films, from more places, and even better quality. Our attorneys are making final edits to the revenue sharing agreement for The Transparent Film Festival Presents: www.transparentfilmfestival.com If your film is on the site, and you want it to earn revenue, you don't need to do anything except wait for the agreement, which we will be sending out next week. If for any reason you no longer want your film on our site, please email us at: [email protected] or inbox us here, and we will promptly remove your film. We are offering a free trial for a week. Have a look at what your fellow filmmakers are doing. [NOTE: YOU DO NOT HAVE TO SIGN UP FOR A FREE TRIAL TO SEE IF YOUR FILM IS ON THE SITE. A THUMBNAIL WILL TELL YOU.] We take our hats off to all the truly independent filmmakers from around the world, who are making it happen. IMPORTANT ANNOUNCEMENT!
2019 was our 1st year and a HUGE success. Judging from what we are seeing for 2020, it's going to be even better with more films, from more places, and even better quality.
Our attorneys are making final edits to the revenue sharing agreement for The Transparent Film Festival Presents: www.transparentfilmfestival.com
If your film is on the site, and you want it to earn revenue, you don't need to do anything except wait for the agreement, which we will be sending out next week.
If for any reason you no longer want your film on our site, please email us at: [email protected] or inbox us here, and we will promptly remove your film.
We are offering a free trial for a week. Have a look at what your fellow filmmakers are doing. [NOTE: YOU DO NOT HAVE TO SIGN UP FOR A FREE TRIAL TO SEE IF YOUR FILM IS ON THE SITE. A THUMBNAIL WILL TELL YOU.]
We take our hats off to all the truly independent filmmakers from around the world, who are making it happen.
Jul 20, 2019, 7:12 PM
HIDDEN OR THOUGHTLESS Carina Silva Newark, NJ
Screened Saturday July 20
Timely look at some of the causes, and possible means for preventing suicides.
Jul 20, 2019, 6:08 PM
WISHING YOU THE BEST Monique Sherell Brown New York, NY
Screened Saturday July 20
Dirty blues track right out of True Detective.
Jul 20, 2019, 5:43 PM
HACKED Grey Keith Oakland, CA
Screened Wednesday July 17
The Batman of Hackers squares off against a tech billionaire in Cyberspace.
Jul 18, 2019, 12:44 AM
KARA Octavia Vaughn Brooklyn, NY
Screened Wednesday July 17
After the death of her father, a young woman drowns her emotional pain with alcohol.
Jul 18, 2019, 12:22 AM
KUKLI JAYANTI MAJHI Balisore, India
Screened Wednesday July 17
A little girl struggles to find happiness despite the extreme poverty around her.
Jul 17, 2019, 11:57 PM
SILENT SOUND Bianca Mihailescu Winetka, CA
Screened Tuesday July 16
Three siblings struggle to come to terms with their father’s death.
Jul 16, 2019, 2:33 PM
ON AIR Ryan Conway Sandy Hook, CT Screened Thursday, July 11 Wackiness at a radio station abounds when a new boss shows up after the old one gets fired. ON AIR Ryan Conway Sandy Hook, CT
Screened Thursday, July 11
Wackiness at a radio station abounds when a new boss shows up after the old one gets fired.
Jul 12, 2019, 1:10 AM Go here now! Click Watch live and then click play!
https://www.mnn.org/live/2-lifestyle-channel Updated Jul 12, 2019, 12:03 AM
Jul 12, 2019, 12:03 AM
THE HOLDOUT Javier Augusto Nunez San Diego, CA
Screened Sunday July 7th
Two angels work to help a young man find true love.
Jul 7, 2019, 11:57 PM
UNTIL MORNING Pernille Kaufmann Copenhagen, Denmark Screened Sunday July 7th Experimental film about the goings on inside a food truck set to poetry. Jul 7, 2019, 6:02 PM
FLETCHER’S HIT Eli Jordan Dublin, Ireland
Screened Sunday July 7th
After a break-up, a suicidal man tries to take back the contract hit he put on himself.
Jul 7, 2019, 5:28 PM
FAMILY GATHERING Nicole Brokaw Forest Hill, MD
Screened Sunday July 7
Insightful doc about an enclave of glass blowers in Corning New York.
Jul 7, 2019, 5:03 PM
THE STOP Liudmila Komrakova St. Petersburg, Russia
Screened Thursday July 4
A couple does the Tango in the rain at a bus stop in St. Petersburg.
Jul 4, 2019, 10:26 PM
SOCial MEdia MADness Michael Delaney New York, NY
Screened Thursday July 4
A man hires a social media consultant to get likes for his Instagram posts.
Jul 4, 2019, 11:11 AM
HELLO BABY Lee Siu Lung Kwun Tong, Kowloon, Hong Kong
Screened Tuesday July 2
A woman seeks help from an exorcist after she is tormented by her aborted fetus.
Jul 3, 2019, 3:32 AM
CASHED Ethan Itzkow Brooklyn, NY
Screened Sunday June 26
Normally weed doesn’t cause problems like this!
Jun 30, 2019, 6:58 PM
SIGNS POINT TO YES Matthew Diulus Pittsburgh, PA
Screened Sunday June 30
After losing everything from his job to his girlfriend, a man escapes into a virtual casino.
Jun 30, 2019, 5:27 PM
AHOM William Bottini San Francisco, CA Screened Sunday June 30 Animated glass with a secret message. Jun 30, 2019, 3:21 PM
CLAIRE Francesca Ravera Rome, Italy
Screened Tuesday June 25
A young woman can’t let go after she loses the love of her life to an accident.
Jun 25, 2019, 5:05 PM
SILENT BRAINWASH Dominik Nimar Munich, Germany
Screened Sunday June 23
An operative is forced to remember a crime he forgot. What's amazing about this film is it's done in Sign Language. Stunning.
Jun 24, 2019, 1:28 AM
The HOUSEGUEST Elijah B. Rodriguez New York, NY
Screened Friday June 21
Two women locked in a deadly game of cat and mouse.
Jun 21, 2019, 4:33 PM
THE FIFTH OF NOVEMBER Javier Augusto Nunez San Diego, CA
Screened Saturday June 15
A woman struggles to cope with the loss of her fiancé after a mass shooting.
Jun 15, 2019, 2:45 PM
I, DREAMING Martin Del Carpio Venezuela
Screened Friday June 14
A man on the other side of sleep is confronted by his evil alter ego on the streets of New York.
Jun 15, 2019, 12:17 AM
SUCCESSION Elijah Rodriguez New York, NY
Screened Friday June 14
Malevolent spirit isn’t willing to share her home.
Jun 14, 2019, 11:48 PM On right now
https://www.mnn.org/live/2-lifestyle-channel Updated Jun 14, 2019, 12:02 AM
Jun 14, 2019, 12:02 AM
Episode 9 of TransparentDTV - The Transparent Film Festival Presents: This week previews of shorts Irradiate, Nour, The Pickup and Coastline; Musical Guests Cheap Trick and Alessi Brothers; hosted by Sofia McLane from Pier 96 in NYC; Movie Review of Godzilla - King of the Monsters. Episode 9 of TransparentDTV - The Transparent Film Festival Presents:
This week previews of shorts Irradiate, Nour, The Pickup and Coastline; Musical Guests Cheap Trick and Alessi Brothers; hosted by Sofia McLane from Pier 96 in NYC; Movie Review of Godzilla - King of the Monsters.
Jun 10, 2019, 8:18 AM
MARIONETTE DOLL Cherry Fu Flushing, NY
Screened Saturday June 8
A home health aide moonlights as a dominatrix.
Jun 9, 2019, 1:24 AM
THE END OF JUNE Wayne Stephens Jersey City, NJ
Screened Saturday June 8
Stylish yet bleak depiction of events that could potentially befall us all.
Jun 9, 2019, 12:34 AM
IRRADIATE Paul London and Tracey Moberly Wales, UK Screened Saturday June 8 You have never seen a film about alpha particles before, much less shot on an iPhone. Jun 8, 2019, 11:57 PM
HOUSE, NOT HOME Eric Purcell Miami, FL Screened Saturday June 8 Radio station employees stay behind during a hurricane. Jun 8, 2019, 11:49 PM
COASTLINE Gus Hawley Manchester Massachusetts
Screened Sunday 6/2
Amazing true fish tale as told through the eyes of a young enthusiast. Gorgeous cinematography.
Jun 2, 2019, 1:49 PM
DISPLACEMENT Maxime Corbeil-Perron Montreal Canada
Screened Sat. June 1
Visual and stylish, music combined with stop motion shots convey a plethora of uneasiness.
Jun 2, 2019, 3:39 AM
NOUR John Cunningham Melbourne, AU
Screened Sat. June 1
A young girl is separated from her mother in a refugee camp.
Jun 2, 2019, 3:13 AM
THE PICK UP Phil Reissman New York, NY
Screened Sat. June 1
The DOJ puts the screws to a would be cooperator.
Jun 2, 2019, 3:05 AM
At NY Premiere of Godzilla with Brad Whitford and Millie Bobbie Brown!!! Jun 2, 2019, 2:41 AM You tagged Nodari Marjanidze
Tonight on Verizon FIOS (Ch. 34) and Spectrum (Ch. 25) another new episode of Transparent DTV with clips from short films: The Fruitful (Ireland) Keepers of The Forest (Australia) Minor Key (Germany) The Waiting (Mexico) Also artist Tom Grindberg (Marvel), Pirosmani Restaurant. Musical performance from End Of The Universe Live at The Gramercy! https://transparent.vhx.tv/transparent-dtv-2/videos/transparent-dtv-on-mnn-episode-8 May 30, 2019, 9:24 PM Updated May 29, 2019, 1:59 PM
May 29, 2019, 1:59 PM
L'UOMO NUOVO Andrea Murchio Italy
Screened Sunday May 26
Political thriller in the style of 24, that takes place in a not too distant dystopian society.
May 26, 2019, 1:48 PM
LOSING Sarah Allyn Brooklyn, NY
Screened Sunday May 26
Heartbreaking story about losing a loved one to drugs.
May 26, 2019, 1:05 PM
NOM Angel Hernández Suárez Las Palmas, Spain
Screened Sunday May 26
Stylish short about a Vampire Cyclist!
May 26, 2019, 12:33 PM
Don't Worry Ryan Ng Roslyn, NY
Screened Sunday May 26
Every father's worst nightmare.
May 26, 2019, 11:42 AM
MORSE Tom Bessoir New York, NY Screened Sunday May 26 Clever animation about the history and usage of the Morse code. MORSE Tom Bessoir New York, NY
Screened Sunday May 26
Clever animation about the history and usage of the Morse code.
May 26, 2019, 11:30 AM
THE DIGITS OF PI Tom Bessoir New York, NY Screened Saturday May 25 Clever multimedia piece about 3.14159265359 May 26, 2019, 1:00 AM
WHISPERS FROM INDIAN CREEK Linda Lee Sparta, TN Screened Saturday May 25 A young woman faces a myriad of challenges after a car accident. May 26, 2019, 12:57 AM
RAISING PHIL AND ERNIE Linda Lee Sparta, TN Screened Saturday May 25 A guy, down on his luck, catches a few breaks in this Tennessee version of the Odd Couple May 26, 2019, 12:38 AM
THE STUFF OF NIGHTMARES Ian Boyd New York Screened Thursday May 23 Concept short about a man with a split personality. May 26, 2019, 12:28 AM
https://transparent.vhx.tv/transparent-dtv-2/videos/1075299-0503-transparent-dtv-on-mnn-episode-4 Apr 29, 2019, 11:06 PM
Okay so..... this is the latest. We've been pretty busy believe it or not, SINCE THE FESTIVAL ENDED! We've been approached by several potential sponsors including one of the large Automobile Manufacturers for Transparent 2020. We're trying our best to get everyone their customized laurels - because you all deserve them. That said... There are some fake Transparent Film Festival Laurels out there so just know - if they don't look like this.... they don't mean anything. Okay so..... this is the latest.
We've been pretty busy believe it or not, SINCE THE FESTIVAL ENDED! We've been approached by several potential sponsors including one of the large Automobile Manufacturers for Transparent 2020.
We're trying our best to get everyone their customized laurels - because you all deserve them. That said...
There are some fake Transparent Film Festival Laurels out there so just know - if they don't look like this.... they don't mean anything.
Apr 27, 2019, 12:43 AM
Updated Apr 9, 2019, 1:05 AM
Apr 9, 2019, 1:05 AM
3 days - what a ride. The film’s were great, the performances were great, and the people who turned out (over 250) were just the best.
Videos are going to combined into (what else) a 3 to 4 minute clip. Here are just some stills from the event.
Apr 7, 2019, 11:13 PM
Apr 7, 2019, 10:57 PM
Apr 7, 2019, 12:32 AM
In case you missed it - tomorrow's going to be off the hook. Lots of new friends and contacts. Here's a sneak peak of what's happened and what's next.
https://www.facebook.com/transparentfilmfestival/videos/276719146599756 Updated Apr 7, 2019, 12:14 AM
Apr 7, 2019, 12:14 AM Click for video:
I can't believe the last two days. Excellent films, awesome people, many new friends and contacts. Tomorrow is going to be off the hook. Here's a sneak peak - just for Facebook. And don't forget The Transparent Film Festival is sponsored by... SWEET BITCH! I can't believe the last two days. Excellent films, awesome people, many new friends and contacts. Tomorrow is going to be off the hook.
Here's a sneak peak - just for Facebook.
And don't forget The Transparent Film Festival is sponsored by...
SWEET BITCH!
Apr 7, 2019, 12:03 AM
It was packed and dark, and I was talking to so many people at the same time.... But I managed to obtain one photo from last night - it gets even better today! Apr 6, 2019, 10:04 AM Spotlight International https://transparent.vhx.tv/products/international-spotlight Updated Apr 5, 2019, 8:08 PM
Apr 5, 2019, 8:08 PM
SPOTLIGHT USA Filmmakers https://transparent.vhx.tv/products/spotlight-usa SPOTLIGHT USA Filmmakers https://transparent.vhx.tv/products/spotlight-usa
Apr 5, 2019, 8:07 PM
SCI-FI https://transparent.vhx.tv/products/sci-fi SCI-FI https://transparent.vhx.tv/products/sci-fi
Apr 5, 2019, 8:07 PM Crime In The USA https://transparent.vhx.tv/products/crime-in-the-usa Updated Apr 5, 2019, 8:06 PM
Apr 5, 2019, 8:06 PM Dramas From The U.K. https://transparent.vhx.tv/products/dramas-from-the-u-k Updated Apr 5, 2019, 8:05 PM
Apr 5, 2019, 8:05 PM Films From Iran https://transparent.vhx.tv/products/transparent-film-festival-online Updated Apr 5, 2019, 8:04 PM
Apr 5, 2019, 8:04 PM 2 more great Indie features: https://transparent.vhx.tv/transparent-film-festival-online-best-feature-program-2
Starts tonight at 8PM on http://transparentDTV.com/ Updated Apr 5, 2019, 7:13 PM
Apr 5, 2019, 7:13 PM 3 Awesome featuers from Candada, Egypt and Russia https://transparent.vhx.tv/transparent-film-festival-online-best-feature-competition-1
Remember we could only screen 1 feature live - but these films would have made it - had we more time.
The Orchestration of Audrey (Canada) This Much (USA by way of Russia) Mesteka & Rehan (Egypt) Updated Apr 5, 2019, 1:56 PM
Apr 5, 2019, 1:56 PM Our second of 2 great online international shorts programs. AND THIS ONE'S REALLY GOOD!!!
https://transparent.vhx.tv/transparent-film-festival-online-program-6-international-spotlight-2 Updated Apr 5, 2019, 1:16 PM
Apr 5, 2019, 1:16 PM
Great independent filmmakers from around the USA battle it out for BEST SHORT at: https://transparent.vhx.tv/transparent-film-festival-online-program-5-usa-spotlight Great independent filmmakers from around the USA battle it out for BEST SHORT at:
https://transparent.vhx.tv/transparent-film-festival-online-program-5-usa-spotlight
Apr 5, 2019, 12:24 PM
The ONLINE Sci Fi Program https://transparent.vhx.tv/transparent-film-festival-online-program-4-sci-fi GOES LIVE FRIDAY NIGHT 4/5 at 8:00 PM The ONLINE Sci Fi Program
https://transparent.vhx.tv/transparent-film-festival-online-program-4-sci-fi
GOES LIVE FRIDAY NIGHT 4/5 at 8:00 PM
Apr 5, 2019, 3:01 AM CRIME IN THE USA
https://transparent.vhx.tv/the-transparent-film-festival-online-program-3-crime-in-the-usa
ALL ONLINE PROGRAMS WILL GO LIVE 8PM Friday 4/5 Updated Apr 5, 2019, 2:42 AM
Apr 5, 2019, 2:42 AM https://transparent.vhx.tv/the-transparent-film-festival-online-program-2-uk-dramas
ACCESS BEGINS TOMORROW NIGHT AT 8PM Updated Apr 5, 2019, 1:05 AM
Apr 5, 2019, 1:05 AM https://transparent.vhx.tv/the-transparent-film-festival-online-program-1-films-from-iran
ACCESS BEGINS TOMORROW NIGHT AT 8PM Updated Apr 5, 2019, 12:33 AM
Apr 5, 2019, 12:33 AM
FINALLY - The Transparent Film Festival Presents: The Online Competition The Programs Will Be Seen Here beginning at 8PM tomorrow night - FRIDAY, APRIL 5, 2019 http://transparentDTV.com Rules for determining BEST FEATURE and BEST SHORT 25% Total view minutes from unique IP addresses 25% Comments, Likes and Shares on Facebook 50% Transparent Judges Online Festival Program 1 - Films From Iran� Forouzan (Mystery) 12:00 Brotherhood (Documentary) 37 min The Hunt (Short) 13 min� In Perspective (Short) 12 min� The Dome of the Universe (Documentary) 29 min �Online Festival Program 2 - Dramas From The UK� Happy Retirement (Short) 20 min �A Date With Shillelagh (Short) 12 min 32 min� Long Gone (Short) 12 min� The Caterer’s Reckoning (Short) 19:54 �Home Truths (Short) 19:55 Online Festival Program 3 - Crime in the USA Duct Tape (Dark Comedy) 7:57 It's My House (Dark Comedy) 17:24 Purgatory (Crime) 23:00 Payback (Crime) 16:08 Jigsaw (Drama) 16:12 The Room (Crime) 5:01 Online Festival - Program 4 Science Fiction Tapferkeit (WWII Drama) 19:17 FM 132.9 (Sci-Fi) 23:58 Blakemore (Sci-Fi) 15:00 Blink (Sci-Fi) 6:05 Ocularis (Sci Fi) 24:00 Online Festival - Program 5 - USA Spotlight Only You Know (Drama) 11:59 The Garden 1910 (Animation) 30:00 Hands (6:54) Pay Me No Mime (4:46) The Newcomer (13:48) Reflection (3:01) Online Festival - Program 6 - International Spotlight Ibasho (Japan) 8:34 Fling (Canada) 5:31 Blue Tomorrow (Turkey) 14:53 DML (United Kingdom) 4:00 A Frame For Solitude (Armenia) 29:59 14+2 Days (Iran) 19:26 Online Festival - Program 7a - Best Feature Competition The Orchestration of Audrey (Canada) 1 Hr. 5 min This Much (USA) 1 Hr. 13 min Online Festival - Program 7b - Best Feature Competition One More Thing 1 Hr. 43 min (USA) Mesteka & Rehan (Egypt) 60 min FINALLY - The Transparent Film Festival Presents: The Online Competition
The Programs Will Be Seen Here beginning at 8PM tomorrow night - FRIDAY, APRIL 5, 2019 http://transparentDTV.com/
Rules for determining BEST FEATURE and BEST SHORT 25% Total view minutes from unique IP addresses 25% Comments, Likes and Shares on Facebook 50% Transparent Judges
Online Festival Program 1 - Films From Iran� Forouzan (Mystery) 12:00 Brotherhood (Documentary) 37 min The Hunt (Short) 13 min� In Perspective (Short) 12 min� The Dome of the Universe (Documentary) 29 min
�Online Festival Program 2 - Dramas From The UK� Happy Retirement (Short) 20 min �A Date With Shillelagh (Short) 12 min 32 min� Long Gone (Short) 12 min� The Caterer’s Reckoning (Short) 19:54 �Home Truths (Short) 19:55
Online Festival Program 3 - Crime in the USA Duct Tape (Dark Comedy) 7:57 It's My House (Dark Comedy) 17:24 Purgatory (Crime) 23:00 Payback (Crime) 16:08 Jigsaw (Drama) 16:12 The Room (Crime) 5:01
Online Festival - Program 4 Science Fiction Tapferkeit (WWII Drama) 19:17 FM 132.9 (Sci-Fi) 23:58 Blakemore (Sci-Fi) 15:00 Blink (Sci-Fi) 6:05 Ocularis (Sci Fi) 24:00
Online Festival - Program 5 - USA Spotlight Only You Know (Drama) 11:59 The Garden 1910 (Animation) 30:00 Hands (6:54) Pay Me No Mime (4:46) The Newcomer (13:48) Reflection (3:01)
Online Festival - Program 6 - International Spotlight Ibasho (Japan) 8:34 Fling (Canada) 5:31 Blue Tomorrow (Turkey) 14:53 DML (United Kingdom) 4:00 A Frame For Solitude (Armenia) 29:59 14+2 Days (Iran) 19:26
Online Festival - Program 7a - Best Feature Competition The Orchestration of Audrey (Canada) 1 Hr. 5 min This Much (USA) 1 Hr. 13 min
Online Festival - Program 7b - Best Feature Competition One More Thing 1 Hr. 43 min (USA) Mesteka & Rehan (Egypt) 60 min
Apr 4, 2019, 9:46 PM Transparent Film Festival updated their status. ONLINE FESTIVAL WILL BEGIN at 8 PM on http://TRANSPARENTDTV.com/
Apr 4, 2019, 4:48 PM
Quick update: Opening Night and Program 6 are sold out. Program 5 is close to sold out. Trailers below in previous posts. ONLINE Film Festival starts Friday Night, April 5 at 8PM with 6 programs (listings below in previous post). You will be able to purchase tickets for, and view the online programs at http://transparentDTV.com Quick update: Opening Night and Program 6 are sold out. Program 5 is close to sold out.
Trailers below in previous posts.
ONLINE Film Festival starts Friday Night, April 5 at 8PM with 6 programs (listings below in previous post).
You will be able to purchase tickets for, and view the online programs at http://transparentDTV.com/
Apr 4, 2019, 12:02 AM THE AWARD WINNERS - SOLD OUT!!!!
Please message us for Waiting List. Preference will be given to ticket holders for Best Feature - Program 5
https://transparent.vhx.tv/transparent-film-festival/videos/program-6-trailer-award-winning-films-sun-april-7-4-pm-kraine-theater-brought-to-you-by-sweet-bitch-wine Updated Mar 31, 2019, 10:52 PM
Mar 31, 2019, 10:52 PM This is the Canadian film that won Best Feature "The Lower Plateau". Preceded by 15 minutes of some of the best shorts we received: "The Waiting", "I'm Not Tired" & "The Leshiy".
https://transparent.vhx.tv/transparent-film-festival/videos/transparent-film-festival-program-5-best-feature-kraine-theater-sun-april-7-2pm
Tickets available at: http://filmfreeway.com/transparentfilmfestival/tickets Updated Mar 31, 2019, 3:55 PM
Mar 31, 2019, 3:55 PM Crime & Horror Program Sun. 4/7 Kraine Theater Noon
https://transparent.vhx.tv/transparent-film-festival/videos/transparent-film-festival-program-4-trailer-crime-and-horror-the-kraine-theater-sun-april-7-12-pm Updated Mar 31, 2019, 3:14 PM
Mar 31, 2019, 3:14 PM Spotlight Trailer (Program 3 Sat. 4/6) at:
https://transparent.vhx.tv/transparent-film-festival/videos/transparent-film-festival-program-3-trailer-kraine-theater-saturday-april-6th-4pm Updated Mar 31, 2019, 2:10 PM
Mar 31, 2019, 2:10 PM LGBTQ Program 2 Trailer at:
https://transparent.vhx.tv/videos/transparent-film-festival-lgbtq-trailer Updated Mar 31, 2019, 2:04 PM
Mar 31, 2019, 2:04 PM Around The World Trailer at: https://transparent.vhx.tv/videos/transparent-film-festival-around-the-world-program-1-trailer-kraine-theater-sat-april-6-12pm Updated Mar 31, 2019, 1:58 PM
Mar 31, 2019, 1:58 PM Opening Night Trailer at:
https://transparent.vhx.tv/transparent-film-festival/videos/transparent-film-festival-opening-night-program-trailer Updated Mar 31, 2019, 1:56 PM
Mar 31, 2019, 1:56 PM Check out the Spotlight Trailer (Sat. 4/6 4pm) At The Kraine Theater 85 E4th St. NYC
https://transparent.vhx.tv/transparent-film-festival/videos/transparent-film-festival-program-3-trailer-kraine-theater-saturday-april-6th-4pm
Tickets at: http://filmfreeway.com/transparentfilmfestival/tickets Updated Mar 31, 2019, 1:46 PM
Mar 31, 2019, 1:46 PM We're creating something really special here. Opening Night at The Producers Club going to be a blast.
https://transparent.vhx.tv/transparent-film-festival/videos/transparent-film-festival-opening-night-program-trailer
Tickets at http://filmfreeway.com/transparentfilmfestival Updated Mar 31, 2019, 1:08 AM
Mar 31, 2019, 1:08 AM Transparent Film Festival updated their status. There are exactly 10 tickets left for Program 6 on Sunday April 7 (which includes the Awards Ceremony).
All discount tickets (2 for 1) have been grabbed.
I know I’ve said this before but there will be no ticket sales at the door. There is a strict number on people that can be let in due to the fire code.
Mar 30, 2019, 6:36 PM I would like to draw everyone’s attention to Program 2 on Saturday April 6th (2pm) a Time The Kraine Theater.
Use code STRATFS for 2 for 1 tickets for this awesome program featuring some amazing LGBTQ themed films.
Here is just one of the trailers: https://youtu.be/WmtnMnO0RZQ Updated Mar 29, 2019, 3:30 PM
Mar 29, 2019, 3:30 PM
All systems go at the world famous Producers Club! https://filmfreeway.com/transparentfilmfestival/tickets Mar 26, 2019, 9:25 PM
Note to filmmakers- the 60fps won’t work or at 4K.
As it states in the festival description we need 1920 x 1080 at 30fps.
Otherwise your film may sputter. No sputtering allowed.
Inbox me if you think your film fits this description.
Thanks
Mar 26, 2019, 12:21 AM Transparent Film Festival updated their status. Quick reminder: There will be no tickets available available for purchase at the door on Fri 4/5 (Producer’s Club), or Sat 4/6 and 4/7 (Kraine Theater).
People will show up and be disappointed unfortunately so we just have to say it again and again here.
Mar 26, 2019, 12:08 AM Just look at these performances. The Best Actress Nominees are....
https://transparent.vhx.tv/transparent-film-festival/videos/transparent-film-festival-best-actress-2019 Updated Mar 24, 2019, 8:35 PM
Mar 24, 2019, 8:35 PM Check out these incredible up and coming actors! https://transparent.vhx.tv/videos/transparent-film-festival-best-actor-nominees-2019 Inbox me re tickets. Friday night about sold out. Updated Mar 24, 2019, 12:56 AM
Mar 24, 2019, 12:56 AM
It has begun. Rehearsals for The Transparent Film Festival have begun. Mar 21, 2019, 3:40 PM
Please don’t let this happen to you. I haven’t even emailed my friends, band mates people from work or the gym. When that happens there will be no tickets left. Please understand- there will be NO TICKETS AT THE DOOR - NO EXCEPTIONS. The fire code is strict at 100 persons. Mar 20, 2019, 7:37 PM Transparent Film Festival updated their status. At this point all filmmakers should have their comps.
If your film has someone nominated for Best Actor or Best Actress, you will need to let them know by the end of next week (Friday 3/22).
ALL COMPS NEED TO BE RECONCILED BY FRIDAY MARCH 22 - NO EXCEPTIONS.
Please note Program 6 will soon be completely sold. There will be no tickets available at the door.
Mar 15, 2019, 1:44 PM
Transparent Film Festival- Online Program 2 FILMS FROM THE UNITED KINGDOM Happy Retirement 20 min A Date With Shillelagh 12 min 32 min Long Gone 12 min The Caterer’s Reckoning 19:54 Home Truths 19:55 Mar 14, 2019, 11:44 PM
Next Online Program to be announced shortly- Films From The U.K. Mar 14, 2019, 3:35 AM
Online Festival Program 1 - Films From Iran Are You Volleyball (Short) 15 min Brotherhood (Documentary) 37 min The Hunt (Short) 13 min Breath (Short) 1 min In Perspective (Short) 12 min The Dome of the Universe (Documentary) 29 min Online Festival Program 1 - Films From Iran
Are You Volleyball (Short) 15 min Brotherhood (Documentary) 37 min The Hunt (Short) 13 min Breath (Short) 1 min In Perspective (Short) 12 min The Dome of the Universe (Documentary) 29 min
Mar 12, 2019, 9:14 PM
Two incredibly exciting pieces of news. 1). Our cable television show begins airing in Manhattan on Friday Night - April 5th - immediately after our opening night Gala. Totally thrilled that we were able to work out the premiere and our Friday night at midnight time slot with Cablevision. That means - EVERY FRIDAY NIGHT in Manhattan (up to 620,000 households can watch the best of Transparent DTV and the Transparent Film Festival on their big screen living room TVs!!! http://transparentDTV.com and... 2) The first Transparent Film Festival Online Program will be announced tomorrow (International Program 1). Two incredibly exciting pieces of news. 1). Our cable television show begins airing in Manhattan on Friday Night - April 5th - immediately after our opening night Gala.
Totally thrilled that we were able to work out the premiere and our Friday night at midnight time slot with Cablevision. That means - EVERY FRIDAY NIGHT in Manhattan (up to 620,000 households can watch the best of Transparent DTV and the Transparent Film Festival on their big screen living room TVs!!!
http://transparentDTV.com/
and...
2) The first Transparent Film Festival Online Program will be announced tomorrow (International Program 1).
Mar 12, 2019, 7:45 PM Tickets on Sale here: https://filmfreeway.com/TransparentFilmFestival/tickets
First come, first serve. Please be aware that each program will sell out. There will be no tickets available at the door. Updated Mar 10, 2019, 2:00 PM
Mar 10, 2019, 2:00 PM
The Transparent Film Festival screening schedule, category winners and award nominees are as follows: See attached.
Mar 10, 2019, 3:11 AM Transparent Film Festival updated their status. Filmmakers please check your email
Mar 9, 2019, 5:19 PM
IT’S OFFICIAL- 3RD NIGHT ADDED Opening Night Gala with special screening of the best Semi-Finalist Films (2 hours).
Full Schedule to be announced Sunday here on Facebook along with Ticket Sale Link.
Mar 7, 2019, 8:49 PM Transparent Film Festival updated their status. Filmmakers,
I'm getting some wacky ways of delivery. Let's keep it simple.
Just send via Wetransfer.com
Also - please make every effort to keep the file size down. No 15 or 20 gb files.
Thanks
Mar 4, 2019, 8:33 PM
Other festivals talk but we back it up as our filmmakers now know. Great buzz happening and the data doesn't lie. Other festivals talk but we back it up as our filmmakers now know. Great buzz happening and the data doesn't lie.
Mar 3, 2019, 12:11 PM Transparent Film Festival updated their status. Quick heads up. Memorialized here for all to see.
We've received about 1/3 of the downloads so far for the Live Event.
Some filmmakers who were chosen as finalists.... their emails bounced. Others who were chosen as finalists haven't acknowledged their status.
We're going to have a real short leash because everything is about putting on a show now.
Please get your download links at 1920x1080 with reasonable compression by the end of this week at the latest. We need to test on the screen.
If we don't have your downloads soon, we'll pivot to the Semi-Finalists.
Mar 3, 2019, 10:11 AM https://m.youtube.com/watch?v=Oj5jp2yIeRo
Oh it’s gonna be a good one! Updated Mar 2, 2019, 11:41 PM
Mar 2, 2019, 11:41 PM
And now for some of the Transparent Men.
Mar 2, 2019, 8:04 PM Transparent Film Festival updated their status. Official Notifications will go out from Film Freeway on March 10. If your film is on this list, you are a Finalist for screening and should inbox or email us at: [email protected]
Congratulations
All Of This!!! Almost Home Are You Volleyball? Between Love Blood Falls Blue Lies Breath Casualidad Rodriguez Conditioning Desir Pastel Dis/Quiet Enthusiasm Abounds First Seating Free Ride From The Heart Going Under Gold Light (Two Left Feet) Happy Birthday Art Here’s To House Of Redemption I Wanna Be A Russian Vector I’m Not Tired Keepers Of The Forest Kushtaka Less Heat In Arizona Life In A Tiny Town Lighten Up Batman Little Noah’s Last Adventure Lover And A Giver MAGA Maniac Met@slash Mister Pig Obscured Reflection Opera Of The Bells Return From The Stars RUOK Shadows Silence Is Deadly Some Terrible Grief Stari Grad (Old Town) The Absent Carer The Babysitter The Covet The Deepest Cut The Fruitful The Hunt The Last Embrace The Leshy The Lower Plateau The Meeting The Office Party The Painting The Waiting This Isn’t Me Thoughtless Three Trees (In Three Parts Trail Of The Sphinx Welcome To The Powder Keg Yawth
Mar 2, 2019, 1:23 AM
We can now confirm that The Transparent Film Festival has added a third night - Friday Night April 5th. We can now confirm that The Transparent Film Festival has added a third night - Friday Night April 5th.
Mar 2, 2019, 12:33 AM
SOME TERRIBLE GRIEF Adam Noyes USA Screened Fri. March 1 A man struggles to tell his wife he has a male lover, while his son listens from afar. SOME TERRIBLE GRIEF Adam Noyes USA
Screened Fri. March 1
A man struggles to tell his wife he has a male lover, while his son listens from afar.
Mar 1, 2019, 1:49 PM
THE ORCHESTRATION OF AUDREY Brian MacDonald Canada Screened Fri. March 1 You don't want this lawyer mad at you. THE ORCHESTRATION OF AUDREY Brian MacDonald Canada
Screened Fri. March 1
You don't want this lawyer mad at you.
Mar 1, 2019, 1:07 PM
FISH BOWL Williamscott Sorge USA (NYC) Screened Thursday Feb. 28 A sister comes to a cross roads when it comes to helping her brother break out of a rut. Mar 1, 2019, 3:11 AM
THE PAINTING Matteo Belletta USA (NYC) Screened Thursday February 28 An artist Has trouble coming up with an idea for the subject of his next painting. Mar 1, 2019, 2:53 AM
UNPAID SPOT Danny Rathbun USA (Brooklyn) Screened Thursday Feb. 28 Comedians quibble with each other while competing for time at an open mic. Mar 1, 2019, 2:20 AM
I WANNA BE A RUSSIAN VECTOR Thomas Florek USA (NJ) Screened Thursday Feb. 28 Devo like song and video about 45 Mar 1, 2019, 1:52 AM
ASHENG Mohamad Sadeq Esmaeili Iran Screened Tues. Feb. 26 4 courageous woman battle to get their husbands out of prison. ASHENG Mohamad Sadeq Esmaeili Iran
Screened Tues. Feb. 26
4 courageous woman battle to get their husbands out of prison.
Feb 27, 2019, 12:09 AM
GAI JATRA Gopal Shivakoti Nepal Screened Tues. Feb. 26 The LGBQT community takes to the streets in Nepal for the festival of Gai Jatra. GAI JATRA Gopal Shivakoti Nepal
Screened Tues. Feb. 26
The LGBQT community takes to the streets in Nepal for the festival of Gai Jatra.
Feb 26, 2019, 11:54 PM
MOUSSE John Hellberg Sweden Screened Tues. Feb. 26 A man desperate to save his pal takes hostages amidst the backdrop of a horse race. MOUSSE John Hellberg Sweden
Screened Tues. Feb. 26
A man desperate to save his pal takes hostages amidst the backdrop of a horse race.
Feb 26, 2019, 11:13 PM
So far, not an American Feature entry that stands up. Have two to watch and crossing my fingers. But so far nothing at the level of these four. If you're an American Filmmaker and think you can grab the throne, please click this. Because as of now, the first ever Best Feature for Transparent is going out of the country along with a check! http://filmfreeway.com/transparentfilmfestival So far, not an American Feature entry that stands up. Have two to watch and crossing my fingers. But so far nothing at the level of these four.
If you're an American Filmmaker and think you can grab the throne, please click this. Because as of now, the first ever Best Feature for Transparent is going out of the country along with a check!
http://filmfreeway.com/transparentfilmfestival
Feb 26, 2019, 6:36 PM
FM 132.9 Adam Noyes USA (Ca) Screened Monday Feb. 25 The staff at a small radio station relays the news to a oceanside community, that a monster has emerged from the sea, to rampage to through their town. Feb 26, 2019, 3:37 AM
HAPPY BIRTHDAY ART Roy Boucher USA Screened Tues. Feb. 26 Quirky little comedy about infidelity. Feb 26, 2019, 3:34 AM
LAST SEASON Hamidreza Arashkia Iran Screened Monday Feb. 24 Avant-garde art piece that is a filmed play about the five stages of life. Feb 25, 2019, 8:18 AM
FOROUZAN Mirabbas Khosravinezhad Iran Screened Mon. Feb. 24 An old woman prepares to do battle with poachers. Feb 25, 2019, 7:39 AM
SUBLIMINAL Imparato Mario Italy Screened Sunday Feb. 23 A man bothered by the hypocrisy of parishioners tries to get answers from Jesus and Lucifer before shooting up a church. Feb 24, 2019, 10:22 AM
Les Femmes Fatales
Want to know why New York City's Transparent Film Festival Is The Best Film Festival on Film Freeway?
Here's a reason. Actually 10
Submissions Close March 15 http://filmfreeway.com/transparentfilmfestival
Feb 23, 2019, 7:08 PM
VIETNAM AFTERMATH Tom Phillips USA (NJ) Screened Saturday Feb. 23 Powerful documentary about the after effects felt by a generation of soldiers who answered the call of their country, and what they still suffer through as a result all these years later. Feb 23, 2019, 8:56 AM
LAID BACK APPROACH Gino Ceriachi Italy Screened Fri. Feb. 22 A couple's boundless love for their severely handicapped little girl. LAID BACK APPROACH Gino Ceriachi Italy
Screened Fri. Feb. 22
A couple's boundless love for their severely handicapped little girl.
Feb 22, 2019, 12:29 PM
ALMOST HOME Melinda Simon USA (CA) Screened Thurs. Feb. 20 A homesick truck-driver and a dysfunctional family cross paths on a meaningful road trip. Feb 21, 2019, 1:05 AM
LIGHTEN UP BATMAN Vince Arrigo USA (Brooklyn) Screened Wed. Feb. 20 Batman can deal with super-villains, but when it comes to his care free older brother, he’s met his match LMAO Feb 20, 2019, 11:28 PM
SWAP Andre Gaumond Canada Screened Tues. Feb. 19 Two techies invent a mind swap app. Feb 19, 2019, 11:01 PM
TWO ON TWO Ruby Green USA (LA) Screened Tues. Feb. 19 A hot head alienates everyone over a game of basketball. Feb 19, 2019, 9:07 PM
STARI GRAD (OLD TOWN) Jack Beck USA Screened Tues. Feb. 19 At the height of the Balkan wars (1992), a boy wakes up to find his village deserted. Feb 19, 2019, 8:35 PM
HOUSE OF REDEMPTION Tammy Klembith USA Screened Tues. Feb. 19 People who rent this house are confronted by their guilt. Feb 19, 2019, 7:51 PM
KEEPERS OF THE FOREST Roxy Rogan Australia
Screened Mon. Feb. 18
A filmmaker goes to Northern Sumatra to document the impact of deforestation, and human/wildlife conflict, specifically as they relate to the demand for palm oil. I’ll never eat Nutella again.
Feb 18, 2019, 3:59 PM Transparent Film Festival updated their status. VICTOR NOT VICTIM Bethany Hazelitt USA (MI)
Screened Monday Feb. 18
After being assaulted, a young woman has trust issues.
Feb 18, 2019, 2:48 PM
MUSIC VIDEO: LOVER AND A GIVER Jordan Siwek USA (NY) Screened Mon. Feb. 18 A New York love story. MUSIC VIDEO: LOVER AND A GIVER Jordan Siwek USA (NY)
Screened Mon. Feb. 18
A New York love story.
Feb 18, 2019, 1:13 AM
A WASTE IN TIME Wolf Turner USA Screened Mon. Feb. 18 While fishing, a father relays to his son how his parents met. A WASTE IN TIME Wolf Turner USA
Screened Mon. Feb. 18
While fishing, a father relays to his son how his parents met.
Feb 18, 2019, 12:38 AM
TWO LEFT FEET (Gold Light) Kempson Bellington USA (NC) Screened Feb. 17 Moody video. A man deals with the fact that he's fallen out of love with his wife and in love with another. TWO LEFT FEET (Gold Light) Kempson Bellington USA (NC)
Screened Feb. 17
Moody video. A man deals with the fact that he's fallen out of love with his wife and in love with another.
Feb 18, 2019, 12:15 AM
Here's To Kelsey Ferrell USA (TN) Screened Sun. Feb. 17 A young filmmaker's poignant montage about the good and bad aspects of becoming adults. Here's To Kelsey Ferrell USA (TN)
Screened Sun. Feb. 17
A young filmmaker's poignant montage about the good and bad aspects of becoming adults.
Feb 17, 2019, 11:32 PM
REBEL WITHIN Calen Fox UK Screened Sun. Feb. 17 A man gets out of prison after 5 years and confronts the owner of a strip club who owes him money. REBEL WITHIN Calen Fox UK
Screened Sun. Feb. 17
A man gets out of prison after 5 years and confronts the owner of a strip club who owes him money.
Feb 17, 2019, 11:16 PM
Welcome To The Powder Keg Hannah ireland UK Screened Sun. Feb. 17 His mother's illness forces an ex-con into the underground fight world. Welcome To The Powder Keg Hannah ireland UK
Screened Sun. Feb. 17
His mother's illness forces an ex-con into the underground fight world.
Feb 17, 2019, 10:45 PM
THE ROOM Ziyang Lucia Wang USA (CA) Screened Sunday Feb. 17 A rapist picked the wrong gal. Feb 17, 2019, 8:10 PM
FREE RIDE Ziyang Lucia Wang USA (CA) Screened Sunday Feb. 17 One of these mental patients is a bank robber. Feb 17, 2019, 7:51 PM
NO LIES Carlo Hofmann Austria Screened Sun. Feb. 17 Behind the scenes with the Austrian Broadcasting Corporation (ORF), and it’s take on the responsibility of the press to organize the public discourse. Feb 17, 2019, 2:00 AM Transparent Film Festival updated their status. LIL DETECTIVES Chad Crepko USA
Screened Sun. Feb. 17
Kid detectives track a killer.
Feb 17, 2019, 1:32 AM
MONI MojyShow Media USA (FL) Screened Sat. Feb. 16 A young woman goes on a date.... with the wrong guy. Feb 16, 2019, 5:33 PM
TRAIL OF THE SPHINX Mike Mannetta USA (NY) Screened Sat. Feb. 16 Beautifully choreographed homage to Ancient Egypt Feb 16, 2019, 4:25 PM
CONDITIONING Philip Pugh UK Screened Sat. Feb. 16 A gay couple falls prey to a twisted family of serial killers. NOTE: This film May be too disturbing to screen. Flawless. Feb 16, 2019, 4:13 PM
SILENCE IS DEADLY Brice VENEZIANO France Screened Sat. Feb. 16 A human rights message from Chechnya Feb 16, 2019, 3:56 PM
THIS ISN’T ME Ben Kawaller USA (Ca) Screened Sat. Feb. 16 A man tries to find his way through some unusual situations both at work as a tutor, and online in the dating world. Feb 16, 2019, 9:41 AM
RETURN FROM THE STARS Vis Vitalis Russia Screened Sat. Feb. 16 A boy with dreams of becoming a cosmonaut travels back to his childhood home many years later to see if he can find out where it all went wrong. Feb 16, 2019, 9:16 AM
THE MAN WHO DWELT BELOW abram.d.hammer USA (Ca) Screened Sat. Feb. 16 A compassionate sewer dweller helps a young girl Feb 16, 2019, 3:37 AM
UNHINGED Carona Diop USA (NJ) Screened Sat. Feb. 16 Lizzie Borden took an axe.... Feb 16, 2019, 3:09 AM
MANIAC Sean King (USA) NY Screened Sat. Feb. 16 Someone fucked up. Make that two. Feb 16, 2019, 3:06 AM
THE UNTOLD STORY OF ROMEO AND JULIET John Tso USA (CA) Screened Fri. Feb. 15 Shakespeare meets Adult Swim Feb 15, 2019, 11:59 PM
THE WAITING José Betancourt Soler Mexico Screened Fri. Feb. 15 A daughter worries that she’ll miss her flight because of her father... Feb 15, 2019, 11:45 PM
Eternal Paradise Walter Becker Argentina Screened Fri. Feb. 15 After his girlfriend is brutally mugged, a young man is compelled to continue his father's research on the after life. Eternal Paradise Walter Becker Argentina
Screened Fri. Feb. 15
After his girlfriend is brutally mugged, a young man is compelled to continue his father's research on the after life.
Feb 15, 2019, 1:35 AM
DOME OF THE UNIVERSE Mahmoud Nazeri Iran Screened Thurs. Feb. 14 Aerial Tour of Damavand Province in Iran. DOME OF THE UNIVERSE Mahmoud Nazeri Iran
Screened Thurs. Feb. 14
Aerial Tour of Damavand Province in Iran.
Feb 15, 2019, 1:28 AM
MONARCH BUTTERFLY Deborah Kuhn USA (NY) Screened Mon. Feb. 11 A man is unable to cope with his wife's imaginary friend which leads to a showdown. MONARCH BUTTERFLY Deborah Kuhn USA (NY)
Screened Mon. Feb. 11
A man is unable to cope with his wife's imaginary friend which leads to a showdown.
Feb 12, 2019, 12:25 AM
THE PRIDE OF WISDOM dharani rajendran India Screened Mon. Feb. 11 A controversial, and polarizing filmmaker makes a comeback after a few box office bombs. THE PRIDE OF WISDOM dharani rajendran India
Screened Mon. Feb. 11
A controversial, and polarizing filmmaker makes a comeback after a few box office bombs.
Feb 11, 2019, 11:47 PM
THE LOWER PLATEAU Liz Singh Canada Screened Mon. Feb. 11 A musician who resorts to dealing pot after one of the members of her band goes onto stardom struggles to find happiness with the help of her friends. THE LOWER PLATEAU Liz Singh Canada
Screened Mon. Feb. 11
A musician who resorts to dealing pot after one of the members of her band goes onto stardom struggles to find happiness with the help of her friends.
Feb 11, 2019, 12:30 PM
THE BESTOWAL Andrew de Burgh USA (Los Angeles) Screened Sun. Feb. 10 A man is visited by an inter-dimensional being before committing suicide. Feb 11, 2019, 2:11 AM
BLOOD FALLS Joe Leone NYC Screened Sun. Feb. 10 A young woman goes away to watch a relative's house with her friend where she is tormented by witches. BLOOD FALLS Joe Leone NYC
Screened Sun. Feb. 10
A young woman goes away to watch a relative's house with her friend where she is tormented by witches.
Feb 10, 2019, 12:19 PM
THE BABYSITTER Greg Klepper NYC Screened Sat. Feb. 9 A girl thinks she's lucked out with a babysitting job but if it's seems too good to be true... THE BABYSITTER Greg Klepper NYC
Screened Sat. Feb. 9
A girl thinks she's lucked out with a babysitting job but if it's seems too good to be true...
Feb 10, 2019, 12:42 AM Transparent Film Festival updated their status. THE DENTAL PLAN Wayne Diu Ottawa CA
Screened Sat. Feb. 9
A maniac takes over a dentist's office.
Feb 10, 2019, 12:26 AM
Battledream Chronicles Alain Bidard Martinique Screened Sat. Feb. 9 Similar to Tron, people from the real world have to fight to survive inside a game. Battledream Chronicles Alain Bidard Martinique
Screened Sat. Feb. 9
Similar to Tron, people from the real world have to fight to survive inside a game.
Feb 9, 2019, 3:44 PM
FIRST SEATING Mac Brydon USA (NYC) Screened Sat. Feb. 9 Quirky, off-beat comedy about two couples (neighbors), who try to resolve their differences at a weird little bar restaurant. FIRST SEATING Mac Brydon USA (NYC)
Screened Sat. Feb. 9
Quirky, off-beat comedy about two couples (neighbors), who try to resolve their differences at a weird little bar restaurant.
Feb 9, 2019, 2:05 PM
LESS HEAT IN ARIZONA Tom Wardach USA (NJ) Screened Sat. Feb. 9 An unlikely group of criminals deal with the ramifications of a heist that doesn't go according to plan. LESS HEAT IN ARIZONA Tom Wardach USA (NJ)
Screened Sat. Feb. 9
An unlikely group of criminals deal with the ramifications of a heist that doesn't go according to plan.
Feb 9, 2019, 1:56 PM Transparent Film Festival updated their status. Before I continue with the Screening Notes I just have to pause to say OMG - the quality of the films we are getting are now on another level.
Feb 9, 2019, 1:50 PM
PAIR DOWN Jim Menza USA (Chicago) Screened Fri. Feb. 8 Sort of a faith-based version of Dogma. PAIR DOWN Jim Menza USA (Chicago)
Screened Fri. Feb. 8
Sort of a faith-based version of Dogma.
Feb 8, 2019, 3:39 PM
ALL OF THIS Daryl Jones USA (Oakland, CA) Screened Thurs. Feb. 7 A series of vignettes about a group of artists who face challenges and rejection. ALL OF THIS Daryl Jones USA (Oakland, CA)
Screened Thurs. Feb. 7
A series of vignettes about a group of artists who face challenges and rejection.
Feb 7, 2019, 9:23 PM
THE OFFICE PARTY Paul West USA (NJ) Screened Thurs. Feb. 7 A recovering addict waxes nostalgic for the 80's but it's back to the future in his Soho Office. THE OFFICE PARTY Paul West USA (NJ)
Screened Thurs. Feb. 7
A recovering addict waxes nostalgic for the 80's but it's back to the future in his Soho Office.
Feb 7, 2019, 8:43 PM
JIGSAW Paul West USA (NJ) Screened Thurs. Feb. 7 A priest struggles with his fleshly desires. JIGSAW Paul West USA (NJ)
Screened Thurs. Feb. 7
A priest struggles with his fleshly desires.
Feb 7, 2019, 7:40 PM
DOME OF THE UNIVERSE Hamzeh Mohammad Hosseini Iran Screened Tuesday Feb. 5 Beautiful documentary about Iran, its history and its people. Feb 5, 2019, 7:21 PM
JOURNEY OF LIFE Paint VK Thailand Screened Tues. Feb. 5 A young girl learns a hard lesson about self reliance. Feb 5, 2019, 6:45 PM
DML Tone Davies United Kingdom Screened Tuesday Feb. 5 A young girl’s boyfriend isn’t who she thinks he is. Feb 5, 2019, 6:14 PM
FRIEND SPIRIT Paint VK USA (Burbank, CA) Screened Sun. Dec. 3, 2019 A student is visited by the ghost of someone he once wronged. FRIEND SPIRIT Paint VK USA (Burbank, CA)
Screened Sun. Dec. 3, 2019
A student is visited by the ghost of someone he once wronged.
Feb 3, 2019, 5:56 PM
THE MEETING Vito Sugameli Italy Screened Sun. Feb. 3, 2019 Two old friends can't seem to get past an old grievance which leads to tragic consequences. THE MEETING Vito Sugameli Italy
Screened Sun. Feb. 3, 2019
Two old friends can't seem to get past an old grievance which leads to tragic consequences.
Feb 3, 2019, 5:36 PM
14+2 Days erfan ghasempour Iran Screened Thursday Jan. 31 A worker, working on a gas platform in the Persian Gulf, may not make it home for his wedding ceremony. Feb 1, 2019, 12:01 AM
The Deepest Cut Alexandra Guillossou USA (Missouri) Screened Jan. 29 2019 A sobering but warm portrait of a man and his family who are coping with him having MS. Jan 29, 2019, 12:24 PM
Life In A Tiny Town Dhara Visual India Screened Monday Jan. 28 Colorful and visual slice of life in rural India. Jan 28, 2019, 11:52 PM
Our Latest Logo Our Latest Logo
Jan 28, 2019, 12:59 AM Transparent Film Festival updated their status. I can tell everyone now... one of the countries with the strongest films is Iran.
The stuff I'm seeing out of Iran is superb, made by young filmmakers. It's a total disconnect for me given what we hear, see and read about Iran in the news.
Jan 23, 2019, 5:44 PM
Met@slash Sotiris Petridis, Dimitris Tsakaleas Greece Screened Tues. Jan. 22 The debut of Emoji face. Chilling Met@slash Sotiris Petridis, Dimitris Tsakaleas Greece
Screened Tues. Jan. 22
The debut of Emoji face. Chilling
Jan 22, 2019, 7:10 PM
Squeeky Keys Virginia Cook USA Screened Tues. Jan. 22 Story of a piano player who attempts to make it into the Guinness Book of World Records. Squeeky Keys Virginia Cook USA
Screened Tues. Jan. 22
Story of a piano player who attempts to make it into the Guinness Book of World Records.
Jan 22, 2019, 7:02 PM
Now that we have a Facebook page - we will be posting our notes here - one post at a time rather than as part of one thread.
To see the proof of screening of all submissions up through today - you can go here:
https://www.facebook.com/palmaislespictures/photos/131724617593081/329511651147709/ Updated Jan 22, 2019, 2:16 PM
Jan 22, 2019, 2:16 PM Transparent Film Festival added a cover video. Click for video:
Jan 16, 2019, 1:53 PM Updated Jan 16, 2019, 10:58 AM
Jan 16, 2019, 10:58 AM
Going to start posting these thank you notes. It’s why we do what we do. Nov 4, 2018, 3:05 PM Generated by Brian McLane on Wednesday, September 2, 2020 at 2:04 AM UTC-04:00
0 notes
Link
Chitown’s King of the Streets, more commonly referred to as KOTS is the original no prep race established in 2008. We are a blue collar middle class grass roots type of race where everyone is welcome. We can guarantee you will see some of the baddest small tire, big tire, and street cars in the country racing for big money. If two wheels is what you like we have three groups that will have you on the edge of your seat. You just never know what you may see at KOTS!
KOTS is pleased to formally announce our 2019 event classes and rules!
KOTS 2019 OFFICIAL RULES
NOTABLE CHANGES FROM 2018
Addition of Yacht Club Class.
Change of venue to US41.
No double entries.
Burnouts allowed past the starting line.
Changes to max field size on several classes.
Sr. Class buy in: $1.
Changes to format of Jr. Bikes Class cruise.
Removal of Sr. Bike Class.
Please review rules carefully for any other changes not listed here, these are just the major notables.
GENERAL GUIDELINES FOR 2019
KOTS is the original “no prep” event–the track will be untouched throughout the event (unless a wreck/oil down occurs)
All races will be “1st round off the trailer”–NO test passes on race day.
Burnouts must start in the designated burnout box, and may be driven out as far as desired (WATER ONLY)
After first round matches are drawn a bracket will be followed after that.
STARTING METHOD: .400 Pro Tree (7 second timeout) for Sr, Unlimited, Gangster and Extreme Bike. All other classes will run a .100 Pro Tree (7 second timeout)
BYE RUNS: Last racer drawn in the round, will automatically advance (no pass allowed) to race against the first racer drawn in the following round. The drawing racer will have lane choice
LANE CHOICE: Regular draw, 1st name out (of each pair) has lane choice Bye run: 1st name drawn (against the previous round’s bye) has lane choice
CENTER LINE: Crossing the center line in front of your opponent (before the win light indicates a winner) will result in a DQ. (crossing behind does not DQ, but use your head please!!)
DOUBLE RED LIGHTS: In the event of a double red light, the generally accepted “first/worst” rule will apply. (Race coordinator will prove first/worst to the racers ONLY, upon request)
RED LIGHT & CENTER LINE: In the unlikely event that one racer red lights, and the other crosses the center line in front of said red lit opponent, “first/worst” will again apply (example: Most likely, the red light happened first, so they would be out)
ALL cars must have NHRA approved diapers or retention pans (fabricated ok), must meet NHRA requirements
US41 will have their concessions stand & bar open and available for everyone (NO ALCOHOL FOR DRIVERS, SUBJECT TO DQ). Any patrons who appear to be over-served or belligerent will be denied alcohol sales, and may be ejected into police custody if they are a problem
ANY UNAUTHORIZED PEOPLE IN ANY RESTRICTED AREA WITHOUT PROPER CREDENTIALS WILL BE EJECTED IMMEDIATELY WITHOUT A REFUND
VIP spectator areas are strictly off limits to children under 18
NO GLASS BOTTLES/CONTAINERS ARE ALLOWED ANYWHERE ON THE PROPERTY–THEY WILL BE CONFISCATED AND DISCARDED, AND YOU MAY BE EJECTED
RULINGS: Starter has authority to DQ any car while on the line (as with any event). Any other rulings will come from the race coordinator. All rulings are final.
A racer may be DQ’d for any reason not listed here at the discretion of track or KOTS staff.
DQ will result in forfeit of any & all entry fees
There will at least 15 minutes between rounds, save for any unexpected delays (wrecks/oil downs). (time frame will be adjusted if necessary to ensure timely completion of event)
CLASS RULES
RULES FOR STREET LIFE CLASSES
All entries must be legitimately registered and insured.
The cruise will be between 10-45 miles.
Passing lead car or falling behind chase vehicle will result in DQ. (This includes getting pulled over.)
When we return stay in staging lanes until released.
At no point may the driving tires or rims be removed. What you cruise on is what you race on.
Must complete entire cruise under vehicles own power, unassisted.
Engine diapers are not required.
HARD TIRE “220” STREET LIFE CLASS
Minimum 220 UTQG treadwear rating
No AWD
1/4 mile
Any FWD stages with back tire
$100 entry (due @ lock in)
80/20
16 car field
275 STREET LIFE CLASS
Max 275 drag radial
AWD ok
Any FWD stages with back tire
1/8 mile
$200 entry (due @ lock in)
80/20, Sponsored by 1320 Wheels
16 car field
KOTS YACHT CLUB STREET LIFE CLASS
17” DOT drag radial or bigger
No AWD
Must weigh at least 4100lbs
After pass don’t miss scales by the ticket booth or you will be DQ’d
Must be driven to and from pits
Must have factory glass, full exhaust to the bumper, and no cut outs.
1/4 mile
BUY IN: $200 (all due at lock in)
PAY OUT: 80/20 winner/runner up.
KOTS JR. CLASS
Tire: Any 26″ tall, DOT-approved Drag Radial (M/T PRO BRACKET RADIALS ARE *NOT* DOT DRAG RADIALS, FYI) COMMONLY AVAILABLE APPROVED SIZES: 15″ (235/60R15, 275/50R15) 16″ (245/50R16, 255/50R16, 255/60R16) 17″ (245/45R17, 275/40R17, 315/35R17, 335/35R17) 18″ (275/35R18, 285/35R18, 295/35R18, 305/35R18, 315/30R18, 335/30R18, 345/30R18) *****If a tire has a “flotation” size (i.e.: 26×10-15) it is not a DOT drag radial; MUST be “P-metric” sizing (if a tire is not listed here, please ask.)*****
Suspension: ALL stock type, aftermarket parts OK. OEM STYLE FORWARD MOUNTING LOCATIONS (reinforcements/battle boxes OK; ***NEW multi-hole OK***); axle/rear control arm mounts may be adjustable/multi-hole, but must retain stock bracket location. No 4-link/ladder bars. Anti-hop, Slide-a-link, South Side Machine or Caltracs OK. Coilovers OK, minor relocating of shock OK; Relocated Leaf Springs (inboard for clearance) OK; ***NEW: ARB OK***
Cage/chassis: Highly encouraged, NO 25.x, NO back half/tubular frame rails; No wheelie bars
Exterior: Must have ALL DOT required equipment installed and fully functional (lights, horn, etc)
Firewall: Must have complete OEM firewall in factory location
Glass: Must have all OEM-type glass windows (unless otherwise equipped (standard or optional) by manufacturer); lightweight laminated/film tinted glass OK. Door windows must roll up/down (no pinned regulators); EXCEPTION FOR EL CAMINO/RANCHERO/PICKUP TRUCK–MAY HAVE LEXAN/POLYCARBONATE REAR CAB WINDOW TO ACCOMMODATE REAR ROLLBAR/CAGE BARS (All other poly/lexan is prohibited–this includes Optic Armor and similar products)
Exhaust: Must run mufflers (bullets OK); Exhaust must exit at rear axle (flush to axle tubes OK); Wastegates exempt; No open cut outs/bypasses; Factory sidepipes OK (ie: Viper; Corvette; Shelby)
Interior: Must have all factory-type interior (headliner, carpet, door panels, dash, two front seats-aftermarket OK) Rear Seat Delete OK w/upholstery. NO EXPOSED TIN.
Powertrain: Internal Combustion/Compression Engine only; No restrictions
Power adders: No restrictions
1/4 mile
BUY IN: $200 (all due @ lock in)
PAY OUT: $5,000 to win, $1,000 runner up. Sponsored by Mutant Motorsports.
Field size: 8 min, 32 max
KOTS BANGER CLASS
28″ Tire (any)
Any “H” pattern manual trans
Any foot operated, manual clutch
Stock Suspension (F/R)
NO wheelie bars
Must have doors
1/4 mile
BUY IN: $200 (all due @ lock in)
PAY OUT: 100%; 80/20 winner/runner up (guaranteed minimum, not including sponsor/promoter added money)
Field Size: 8 min, 16 max
KOTS GANGSTER CLASS
26” tire (any)
Any rear suspension
Stock style front
NO wheelie bars
Must have doors
PLEASE USE YOUR CHUTES!
1/8 mile
BUY IN: $200 (all due @ lock in)
PAY OUT: $8,000 to win, $1,000 runner up. Sponsored by DK Contractors.
FIELD SIZE: 8 min, 16 max
KOTS UNLIMITED CLASS
Must have doors (no rail/funny car)
Minimum tire height 29″ (any width)
1/8 mile
PLEASE USE YOUR CHUTES!
(That’s it. Really. We even allow pro mods)
BUY IN: $200 (all due @ lock in)
PAY OUT: $3,000 to win, $1,000 runner up. Sponsored by Monkeyshine.
Field Size: 8 min, 16 max
KOTS SR. CLASS
28 x 10.5 non-W or 275/60-15
Any rear suspension
Any front suspension (Wheelbase +/- 2″ from OEM)
No wheelie bars
Must have doors
PLEASE USE YOUR CHUTES!
1/8 mile
BUY IN: $1 (all due @ lock in)
PAY OUT: $10,000 to win! Sponsored by Traction by Boosted and Midwest Tint and Vinyl.
Field Size: 8 min, 32 max
MOTORCYCLE RULES
KOTS EXTREME BIKE CLASS
One rule: No wheelie bars.
Anything else goes
1/8 Mile
BUY IN: TBA
PAY OUT: 100% 80/20 winner/runner up.
FIELD SIZE: 8 min, 16 max
KOTS JR. BIKE CLASS
True Hand Clutch; DOT Tire; No Bars
MUST COMPLETE CRUISE (details below)
Must run same fuel tank in cruise and competition
1/8 mile
BUY IN: $150 (all due at lock in)
PAY OUT: Based on bike count @ 8 bikes Heyyo adds $500.
FIELD SIZE: 32 max
JR. BIKE CRUISE RULES
All Jr Bike Entries must successfully complete the Cruise to compete!
Rider entered must be on their entered bike, fully suited, during Cruise
Riders should maintain stagger formation and adequate spacing
Riders may not pass Cruise Leader (unless they crash and you can’t stop quick enough), nor fall behind Chase Vehicle–either is an automatic DQ
Two tires on the ground at all times
Any leaks or breakdowns—DQ
Any crashes–DQ (exception if somebody hits you, or you hit a rider who crashes in front of you–allow adequate spacing)
No outside assistance of any kind allowed
Cruise is restricted to riders only (nobody allowed on race track during Cruise)
JR. BIKE CRUISE FORMAT
Be prepared to ride from 5-30 laps. Upon completion of final lap:
All bikes will go to the staging lanes.
Bikes will be shut off.
Bikes must be restarted within 5 minutes of shut down.
Upon successful restart, cruise will be complete and racers may return to pits.
The post CHITOWN’S KING OF THE STREETS – THE ORIGINAL NO-PREP RACE 2019 appeared first on No Prep Racing NoPrep.com.
0 notes
Text
A Machine Learning Guide for Average Humans
A Machine Learning Guide for Average Humans
Posted by alexis-sanders
//<![CDATA[ (function($) { // code using $ as alias to jQuery $(function() { // Hide the hypotext content. $('.hypotext-content').hide(); // When a hypotext link is clicked. $('a.hypotext.closed').click(function (e) { // custom handling here e.preventDefault(); // Create the class reference from the rel value. var id = '.' + $(this).attr('rel'); // If the content is hidden, show it now. if ( $(id).css('display') == 'none' ) { $(id).show('slow'); if (jQuery.ui) { // UI loaded $(id).effect("highlight", {}, 1000); } } // If the content is shown, hide it now. else { $(id).hide('slow'); } }); // If we have a hash value in the url. if (window.location.hash) { // If the anchor is within a hypotext block, expand it, by clicking the // relevant link. console.log(window.location.hash); var anchor = $(window.location.hash); var hypotextLink = $('#' + anchor.parents('.hypotext-content').attr('rel')); console.log(hypotextLink); hypotextLink.click(); // Wait until the content has expanded before jumping to anchor. //$.delay(1000); setTimeout(function(){ scrollToAnchor(window.location.hash); }, 1000); } }); function scrollToAnchor(id) { var anchor = $(id); $('html,body').animate({scrollTop: anchor.offset().top},'slow'); } })(jQuery); //]]>
Machine learning (ML) has grown consistently in worldwide prevalence. Its implications have stretched from small, seemingly inconsequential victories to groundbreaking discoveries. The SEO community is no exception. An understanding and intuition of machine learning can support our understanding of the challenges and solutions Google's engineers are facing, while also opening our minds to ML's broader implications.
The advantages of gaining an general understanding of machine learning include:
Gaining empathy for engineers, who are ultimately trying to establish the best results for users
Understanding what problems machines are solving for, their current capabilities and scientists' goals
Understanding the competitive ecosystem and how companies are using machine learning to drive results
Preparing oneself for for what many industry leaders call a major shift in our society (Andrew Ng refers to AI as a "new electricity")
Understanding basic concepts that often appear within research (it's helped me with understanding certain concepts that appear within Google Brain's Research)
Growing as an individual and expanding your horizons (you might really enjoy machine learning!)
When code works and data is produced, it's a very fulfilling, empowering feeling (even if it's a very humble result)
I spent a year taking online courses, reading books, and learning about learning (...as a machine). This post is the fruit borne of that labor -- it covers 17 machine learning resources (including online courses, books, guides, conference presentations, etc.) comprising the most affordable and popular machine learning resources on the web (through the lens of a complete beginner). I've also added a summary of "If I were to start over again, how I would approach it."
This article isn't about credit or degrees. It's about regular Joes and Joannas with an interest in machine learning, and who want to spend their learning time efficiently. Most of these resources will consume over 50 hours of commitment. Ain't nobody got time for a painful waste of a work week (especially when this is probably completed during your personal time). The goal here is for you to find the resource that best suits your learning style. I genuinely hope you find this research useful, and I encourage comments on which materials prove most helpful (especially ones not included)! #HumanLearningMachineLearning
Executive summary:
Here's everything you need to know in a chart:
Machine Learning Resource
Time (hours)
Cost ($)
Year
Credibility
Code
Math
Enjoyability
Jason Maye's Machine Learning 101 slidedeck: 2 years of headbanging, so you don't have to
2
$0
'17
{ML} Recipes with Josh Gordon Playlist
2
$0
'16
Machine Learning Crash Course
15
$0
'18
OCDevel Machine Learning Guide Podcast
30
$0
'17-
Kaggle's Machine Learning Track (part 1)
6
$0
'17
Fast.ai (part 1)
70
$70*
'16
Hands-On Machine Learning with Scikit-Learn and TensorFlow: Concepts, Tools, and Techniques to Build Intelligent Systems
20
$25
'17
Udacity's Intro to Machine Learning (Kate/Sebastian)
60
$0
'15
Andrew Ng's Coursera Machine Learning
55
$0
'11
iPullRank Machine Learning Guide
3
$0
'17
Review Google PhD
2
$0
'17
Caltech Machine Learning on iTunes
27
$0
'12
Pattern Recognition & Machine Learning by Christopher Bishop
150
$75
'06
N/A
Machine Learning: Hands-on for Developers and Technical Professionals
15
$50
'15
Introduction to Machine Learning with Python: A Guide for Data Scientists
15
$25
'16
Udacity's Machine Learning by Georgia Tech
96
$0
'15
Machine Learning Stanford iTunes by Andrew Ng
25
$0
'08
N/A
*Free, but there is the cost of running an AWS EC2 instance (~$70 when I finished, but I did tinker a ton and made a Rick and Morty script generator, which I ran many epochs [rounds] of...)
Here's my suggested program:
1. Starting out (estimated 60 hours)
Start with shorter content targeting beginners. This will allow you to get the gist of what's going on with minimal time commitment.
Commit three hours to Jason Maye's Machine Learning 101 slidedeck: 2 years of headbanging, so you don't have to.
Commit two hours to watch Google's {ML} Recipes with Josh Gordon YouTube Playlist.
Sign up for Sam DeBrule's Machine Learnings newsletter.
Work through Google's Machine Learning Crash Course.
Start listening to OCDevel's Machine Learning Guide Podcast (skip episodes 1, 3, 16, 21, and 26) in your car, working out, and/or when using hands and eyes for other activities.
Commit two days to working through Kaggle's Machine Learning Track part 1.
2. Ready to commit (estimated 80 hours)
By this point, learners would understand their interest levels. Continue with content focused on applying relevant knowledge as fast as possible.
Commit to Fast.ai 10 hours per week, for 7 weeks. If you have a friend/mentor that can help you work through AWS setup, definitely lean on any support in installation (it's 100% the worst part of ML).
Acquire Hands-On Machine Learning with Scikit-Learn and TensorFlow: Concepts, Tools, and Techniques to Build Intelligent Systems, and read the first two chapters immediately. Then use this as supplemental to the Fast.ai course.
3. Broadening your horizons (estimated 115 hours)
If you've made it through the last section and are still hungry for more knowledge, move on to broadening your horizons. Read content focused on teaching the breadth of machine learning -- building an intuition for what the algorithms are trying to accomplish (whether visual or mathematically).
Start watching videos and participating in Udacity's Intro to Machine Learning (by Sebastian Thrun and Katie Malone).
Work through Andrew Ng's Coursera Machine Learning course.
Your next steps
By this point, you will already have AWS running instances, a mathematical foundation, and an overarching view of machine learning. This is your jumping-off point to determine what you want to do.
You should be able to determine your next step based on your interest, whether it's entering Kaggle competitions; doing Fast.ai part two; diving deep into the mathematics with Pattern Recognition & Machine Learning by Christopher Bishop; giving Andrew Ng's newer Deeplearning.ai course on Coursera; learning more about specific tech stacks (TensorFlow, Scikit-Learn, Keras, Pandas, Numpy, etc.); or applying machine learning to your own problems.
Why am I recommending these steps and resources?
I am not qualified to write an article on machine learning. I don't have a PhD. I took one statistics class in college, which marked the first moment I truly understood "fight or flight" reactions. And to top it off, my coding skills are lackluster (at their best, they're chunks of reverse-engineered code from Stack Overflow). Despite my many shortcomings, this piece had to be written by someone like me, an average person.
Statistically speaking, most of us are average (ah, the bell curve/Gaussian distribution always catches up to us). Since I'm not tied to any elitist sentiments, I can be real with you. Below contains a high-level summary of my reviews on all of the classes I took, along with a plan for how I would approach learning machine learning if I could start over. Click to expand each course for the full version with notes.
In-depth reviews of machine learning courses:
Starting out
Jason Maye's Machine Learning 101 slidedeck: 2 years of head-banging, so you don't have to ↓
Need to Know: A stellar high-level overview of machine learning fundamentals in an engaging and visually stimulating format.
Loved:
Very user-friendly, engaging, and playful slidedeck.
Has the potential to take some of the pain out of the process, through introducing core concepts.
Breaks up content by beginner/need-to-know (green), and intermediate/less-useful noise (specifically for individuals starting out) (blue).
Provides resources to dive deeper into machine learning.
Provides some top people to follow in machine learning.
Disliked:
That there is not more! Jason's creativity, visual-based teaching approach, and quirky sense of humor all support the absorption of the material.
Lecturer:
Jason Mayes:
Senior Creative Technologist and Research Engineer at Google
Masters in Computer Science from University of Bristols
Personal Note: He's also kind on Twitter! :)
Links:
Machine Learning 101 slide deck
Tips on Watching:
Set aside 2-4 hours to work through the deck once.
Since there is a wealth of knowledge, refer back as needed (or as a grounding source).
Identify areas of interest and explore the resources provided.
{ML} Recipes with Josh Gordon ↓
Need to Know: This mini-series YouTube-hosted playlist covers the very fundamentals of machine learning with opportunities to complete exercises.
Loved:
It is genuinely beginner-focused.
They make no assumption of any prior knowledge.
Gloss over potentially complex topics that may serve as noise.
Playlist ~2 hours
Very high-quality filming, audio, and presentation, almost to the point where it had its own aesthetic.
Covers some examples in scikit-learn and TensorFlow, which felt modern and practical.
Josh Gordon was an engaging speaker.
Disliked:
I could not get Dockers on Windows (suggested package manager). This wasn't a huge deal, since I already had my AWS setup by this point; however, a bit of a bummer since it made it impossible to follow certain steps exactly.
Issue: Every time I tried to download (over the course of two weeks), the .exe file would recursively start and keep spinning until either my memory ran out, computer crashed, or I shut my computer down. I sent this to Docker's Twitter account to no avail.
Lecturer:
Josh Gordon:
Developer Advocate for at TensorFlow at Google
Leads Machine Learning advocacy at Google
Member of the Udacity AI & Data Industry Advisory Board
Masters in Computer Science from Columbia University
Links:
Hello World - Machine Learning Recipes #1 (YouTube)
GitHub: Machine Learning Recipes with Josh Gordon
Tips on Watching:
The playlist is short (only ~1.5 hours screen time). However, it can be a bit fast-paced at times (especially if you like mimicking the examples), so set aside 3-4 hours to play around with examples and allow time for installation, pausing, and following along.
Take time to explore code labs.
Google's Machine Learning Crash Course with TensorFlow APIs ↓
Need to Know: A Google researcher-made crash course on machine learning that is interactive and offers its own built-in coding system!
Loved:
Different formats of learning: high-quality video (with ability to adjust speed, closed captioning), readings, quizzes (with explanations), visuals (including whiteboarding), interactive components/ playgrounds, code lab exercises (run directly in your browser (no setup required!))
Non-intimidating
One of my favorite quotes: "You don't need to understand the math to be able to take a look at the graphical interpretation."
Broken down into digestible sections
Introduces key terms
Disliked:
N/A
Lecturers:
Multiple Google researchers participated in this course, including:
Peter Norvig
Director of Research at Google Inc.
Previously he directed Google's core search algorithms group.
He is co-author of Artificial Intelligence: A Modern Approach
D. Sculley
Senior Staff Software Engineer at Google
KDD award-winning papers
Works on massive-scale ML systems for online advertising
Was part of a research ML paper on optimizing chocolate chip cookies
According to his personal website, he prefers to go by "D."
Cassandra Xia
Programmer, Software Engineer at Google
She has some really cool (and cute) projects based on learning statistics concepts interactively
Maya Gupta
Leads Glassbox Machine Learning R&D team at Google
Associate Professor of Electrical Engineering at the University of Washington (2003-2012)
In 2007, Gupta received the PECASE award from President George Bush for her work in classifying uncertain (e.g. random) signals
Gupta also runs Artifact Puzzles, the second-largest US maker of wooden jigsaw puzzles
Sally Goldman
Research Scientist at Google
Co-author of A Practical Guide to Data Structures and Algorithms Using Java
Numerous journals, classes taught at Washington University, and contributions to the ML community
Links:
Machine Learning Crash Course
Tips on Doing:
Actively work through playground and coding exercises
OCDevel's Machine Learning Guide Podcast ↓
Need to Know: This podcast focuses on the high-level fundamentals of machine learning, including basic intuition, algorithms, math, languages, and frameworks. It also includes references to learn more on each episode's topic.
Loved:
Great for trips (when traveling a ton, it was an easy listen).
The podcast makes machine learning fun with interesting and compelling analogies.
Tyler is a big fan of Andrew Ng's Coursera course and reviews concepts in Coursera course very well, such that both pair together nicely.
Covers the canonical resources for learning more on a particular topic.
Disliked:
Certain courses were more theory-based; all are interesting, yet impractical.
Due to limited funding the project is a bit slow to update and has less than 30 episodes.
Podcaster:
Tyler Renelle:
Machine learning engineer focused on time series and reinforcement
Background in full-stack JavaScript, 10 years web and mobile
Creator of HabitRPG, an app that treats habits as an RPG game
Links:
Machine Learning Guide podcast
Machine Learning Guide podcast (iTunes)
Tips on Listening:
Listen along your journey to help solidify understanding of topics.
Skip episodes 1, 3, 16, 21, and 26 (unless their topics interest and inspire you!).
Kaggle Machine Learning Track (Lesson 1) ↓
Need to Know: A simple code lab that covers the very basics of machine learning with scikit-learn and Panda through the application of the examples onto another set of data.
Loved:
A more active form of learning.
An engaging code lab that encourages participants to apply knowledge.
This track offers has a built-in Python notebook on Kaggle with all input files included. This removed any and all setup/installation issues.
Side note: It's a bit different than Jupyter notebook (e.g., have to click into a cell to add another cell).
Each lesson is short, which made the entire lesson go by very fast.
Disliked:
The writing in the first lesson didn't initially make it clear that one would need to apply the knowledge in the lesson to their workbook.
It wasn't a big deal, but when I started referencing files in the lesson, I had to dive into the files in my workbook to find they didn't exist, only to realize that the knowledge was supposed to be applied and not transcribed.
Lecturer:
Dan Becker:
Data Scientist at Kaggle
Undergrad in Computer Science, PhD in Econometrics
Supervised data science consultant for six Fortune 100 companies
Contributed to the Keras and Tensorflow libraries
Finished 2nd (out of 1353 teams) in $3 million Heritage Health Prize data mining competition
Speaks at deep learning workshops at events and conferences
Links:
https://www.kaggle.com/learn/machine-learning
Tips on Doing:
Read the exercises and apply to your dataset as you go.
Try lesson 2, which covers more complex/abstract topics (note: this second took a bit longer to work through).
Ready to commit
Fast.ai (part 1 of 2) ↓
Need to Know: Hands-down the most engaging and active form of learning ML. The source I would most recommend for anyone (although the training plan does help to build up to this course). This course is about learning through coding. This is the only course that I started to truly see the practical mechanics start to come together. It involves applying the most practical solutions to the most common problems (while also building an intuition for those solutions).
Loved:
Course Philosophy:
Active learning approach
"Go out into the world and understand underlying mechanics (of machine learning by doing)."
Counter-culture to the exclusivity of the machine learning field, focusing on inclusion.
"Let's do shit that matters to people as quickly as possible."
Highly pragmatic approach with tools that are currently being used (Jupyter Notebooks, scikit-learn, Keras, AWS, etc.).
Show an end-to-end process that you get to complete and play with in a development environment.
Math is involved, but is not prohibitive. Excel files helped to consolidate information/interact with information in a different way, and Jeremy spends a lot of time recapping confusing concepts.
Amazing set of learning resources that allow for all different styles of learning, including:
Video Lessons
Notes
Jupyter Notebooks
Assignments
Highly active forums
Resources on Stackoverflow
Readings/resources
Jeremy often references popular academic texts
Jeremy's TEDx talk in Brussels
Jeremy really pushes one to do extra and put in the effort by teaching interesting problems and engaging one in solving them.
It's a huge time commitment; however, it's worth it.
All of the course's profits are donated.
Disliked:
Overview covers their approach to learning (obviously I'm a fan!). If you're already drinking the Kool-aid, skip past.
I struggled through the AWS setup (13-minute video) for about five hours (however, it felt so good when it was up and running!).
Because of its practicality and concentration on solutions used today to solve popular problem types (image recognition, text generation, etc.), it lacks breadth of machine learning topics.
Lecturers:
Jeremy Howard:
Distinguished Research Scientist at the University of San Francisco
Faculty member at Singularity University
Young Global Leader with the World Economic Forum
Founder of Enlitic (the first company to apply deep learning to medicine)
Former President and Chief Scientist of the data science platform Kaggle
Rachel Thomas:
PhD in Math from Duke
One of Forbes' "20 Incredible Women Advancing AI Research"
Researcher-in-residence at the University of San Francisco Data Institute
Teaches in the Masters in Data Science program
Links:
http://course.fast.ai/start.html
http://wiki.fast.ai/index.php/Main_Page
https://github.com/fastai/courses/tree/master/deeplearning1/nbs
Tips on Doing:
Set expectations with yourself that installation is going to probably take a few hours.
Prepare to spend about ~70 hours for this course (it's worth it).
Don't forget to shut off your AWS instance.
Balance out machine learning knowledge with a course with more breadth.
Consider giving part two of the Fast.ai program a shot!
Hands-On Machine Learning with Scikit-Learn and TensorFlow: Concepts, Tools, and Techniques to Build Intelligent Systems ↓
Need to Know: This book is an Amazon best seller for a reason. It covers a lot of ground quickly, empowers readers to walk through a machine learning problem by chapter two, and contains practical up-to-date machine learning skills.
Loved:
Book contains an amazing introduction to machine learning that briskly provides an overarching quick view of the machine learning ecosystem.
Chapter 2 immediately walks the reader through an end-to-end machine learning problem.
Immediately afterwards, Aurélien pushes a user to attempt to apply this solution to another problem, which was very empowering.
There are review questions at the end of each chapter to ensure on has grasped the content within the chapter and to push the reader to explore more.
Once installation was completed, it was easy to follow and all code is available on GitHub.
Chapters 11-14 were very tough reading; however, they were a great reference when working through Fast.ai.
Contains some powerful analogies.
Each chapter's introductions were very useful and put everything into context. This general-to-specifics learning was very useful.
Disliked:
Installation was a common source of issues during the beginning of my journey; the text glided over this. I felt the frustration that most people experience from installation should have been addressed with more resources.
Writer:
Aurélien Géron:
Led the YouTube video classification team from 2013 to 2016
Currently a machine Learning consultant
Founder and CTO of Wifirst and Polyconseil
Published technical books (on C++, Wi-Fi, and Internet architectures)
Links:
https://www.amazon.com/_/dp/1491962291?tag=oreilly20-20
http://shop.oreilly.com/product/0636920052289.do
https://github.com/ageron/handson-ml
Tips on Using:
Get a friend with Python experience to help with installation.
Read the introductions to each chapter thoroughly, read the chapter (pay careful attention to code), review the questions at the end (highlight any in-text answer), make a copy of Aurélien's GitHub and make sure everything works on your setup, re-type the notebooks, go to Kaggle and try on other datasets.
Broadening your horizons
Udacity: Intro to Machine Learning (Kate/Sebastian) ↓
Need to Know: A course that covers a range of machine learning topics, supports building of intuition via visualization and simple examples, offers coding challenges, and a certificate (upon completion of a final project). The biggest challenge with this course is bridging the gap between the hand-holding lectures and the coding exercises.
Loved:
Focus on developing a visual intuition on what each model is trying to accomplish.
This visual learning mathematics approach is very useful.
Cover a vast variety and breadth of models and machine learning basics.
In terms of presenting the concept, there was a lot of hand-holding (which I completely appreciated!).
Many people have done this training, so their GitHub accounts can be used as reference for the mini-projects.
Katie actively notes documentation and suggests where viewers can learn more/reference material.
Disliked:
All of the conceptual hand-holding in the lessons is a stark contrast to the challenges of installation, coding exercises, and mini-projects.
This is the first course started and the limited instructions on setting up the environment and many failed attempts caused me to break down crying at least a handful of times.
The mini-projects are intimidating.
There is extra code added to support the viewers; however, it's done so with little acknowledgement as to what it's actually doing. This made learning a bit harder.
Lecturer:
Caitlin (Katie) Malone:
Director of Data Science Research and Development at Civis Analytics
Stanford PhD in Experimental Particle Physics
Intern at Udacity in summer 2014
Graduate Researcher at the SLAC National Accelerator Laboratory
https://www6.slac.stanford.edu/
Podcaster with Ben Jaffe (currently Facebook UI Engineer and a music aficionado) on a machine learning podcast Linear Digressions (100+ episodes)
Sebastian Thrun:
CEO of the Kitty Hawk Corporation
Chairman and co-founder of Udacity
One of my favorite Sebastian quotes: "It occurred to me, I could be at Google and build a self-driving car, or I can teach 10,000 students how to build self-driving cars."
Former Google VP
Founded Google X
Led development of the robotic vehicle Stanley
Professor of Computer Science at Stanford University
Formerly a professor at Carnegie Mellon University.
Links:
https://www.udacity.com/course/intro-to-machine-learning--ud120
Udacity also offers a next step, the Machine Learning Engineer Nanodegree, which will set one back about $1K.
Tips on Watching:
Get a friend to help you set up your environment.
Print mini-project instructions to check off each step.
Andrew Ng's Coursera Machine Learning Course ↓
Need to Know: The Andrew Ng Coursera course is the most referenced online machine learning course. It covers a broad set of fundamental, evergreen topics with a strong focus in building mathematical intuition behind machine learning models. Also, one can submit assignments and earn a grade for free. If you want to earn a certificate, one can subscribe or apply for financial aid.
Loved:
This course has a high level of credibility.
Introduces all necessary machine learning terminology and jargon.
Contains a very classic machine learning education approach with a high level of math focus.
Quizzes interspersed in courses and after each lesson support understanding and overall learning.
The sessions for the course are flexible, the option to switch into a different section is always available.
Disliked:
The mathematic notation was hard to process at times.
The content felt a bit dated and non-pragmatic. For example, the main concentration was MATLAB and Octave versus more modern languages and resources.
Video quality was less than average and could use a refresh.
Lecturer:
Andrew Ng:
Adjunct Professor, Stanford University (focusing on AI, Machine Learning, and Deep Learning)
Co-founder of Coursera
Former head of Baidu AI Group
Founder and previous head of Google Brain (deep learning) project
Former Director of the Stanford AI Lab
Chairman of the board of Woebot (a machine learning bot that focuses on Cognitive Behavior Therapy)
Links:
https://www.coursera.org/learn/machine-learning/
Andrew Ng recently launched a new course (August 2017) called DeepLearning.ai, a ~15 week course containing five mini-courses ($49 USD per month to continue learning after trial period of 7 days ends).
Course: https://www.coursera.org/specializations/deep-learning
Course 1: Neural Networks and Deep Learning
Course 2: Improving Deep Neural Networks: Hyperparameter tuning, Regularization and Optimization
Course 3: Structuring Machine Learning Projects
Course 4: Convolutional Neural Networks
Course 5: Sequence Models
Tips on Watching:
Be disciplined with setting aside timing (even if it's only 15 minutes a day) to help power through some of the more boring concepts.
Don't do this course first, because it's intimidating, requires a large time commitment, and isn't a very energizing experience.
Additional machine learning opportunities
iPullRank Machine Learning Guide ↓
Need to Know: A machine learning e-book targeted at marketers.
Loved:
Targeted at marketers and applied to organic search.
Covers a variety of machine learning topics.
Some good examples, including real-world blunders.
Gives some practical tools for non-data scientists (including: MonkeyLearn and Orange)
I found Orange to be a lot of fun. It struggled with larger datasets; however, it has a very visual interface that was more user-friendly and offers potential to show some pretty compelling stories.
Example: World Happiness Dataset by:
X-axis: Happiness Score
Y-axis: Economy
Color: Health
Disliked:
Potential to break up content more with relevant imagery -- the content was very dense.
Writers:
iPullRank Team (including Mike King):
Mike King has a few slide decks on the basics of machine learnings and AI
iPullRank has a few data scientists on staff
Links:
http://ipullrank.com/machine-learning-guide/
Tips on Reading:
Read chapters 1-6 and the rest depending upon personal interest.
Review Google PhD ↓
Need to Know: A two-hour presentation from Google's 2017 IO conference that walks through getting 99% accuracy on the MNIST dataset (a famous dataset containing a bunch of handwritten numbers, which the machine must learn to identify the numbers).
Loved:
This talk struck me as very modern, covering the cutting edge.
Found this to be very complementary to Fast.ai, as it covered similar topics (e.g. ReLu, CNNs, RNNs, etc.)
Amazing visuals that help to put everything into context.
Disliked:
The presentation is only a short conference solution and not a comprehensive view of machine learning.
Also, a passive form of learning.
Presenter:
Martin Görner:
Developer Relations, Google (since 2011)
Started Mobipocket, a startup that later became the software part of the Amazon Kindle and its mobile variants
Links:
Part 1 - https://www.youtube.com/watch?v=u4alGiomYP4
Part 2 - https://www.youtube.com/watch?v=fTUwdXUFfI8
Tips on Watching:
Google any concepts you're unfamiliar with.
Take your time with this one; 2 hours of screen time doesn't count all of the Googling and processing time for this one.
Caltech Machine Learning iTunes ↓
Need to Know: If math is your thing, this course does a stellar job of building the mathematic intuition behind many machine learning models. Dr. Abu-Mostafa is a raconteur, includes useful visualizations, relevant real-world examples, and compelling analogies.
Loved:
First and foremost, this is a real Caltech course, meaning it's not a watered-down version and contains fundamental concepts that are vital to understanding the mechanics of machine learning.
On iTunes, audio downloads are available, which can be useful for on-the-go learning.
Dr. Abu-Mostafa is a skilled speaker, making the 27 hours spent listening much easier!
Dr. Abu-Mostafa offers up some strong real-world examples and analogies which makes the content more relatable.
As an example, he asks students: "Why do I give you practice exams and not just give you the final exam?" as an illustration of why a testing set is useful. If he were to just give students the final, they would just memorize the answers (i.e., they would overfit to the data) and not genuinely learn the material. The final is a test to show how much students learn.
The last 1/2 hour of the class is always a Q&A, where students can ask questions. Their questions were useful to understanding the topic more in-depth.
The video and audio quality was strong throughout. There were a few times when I couldn't understand a question in the Q&A, but overall very strong.
This course is designed to build mathematical intuition of what's going on under the hood of specific machine learning models.
Caution: Dr. Abu-Mostafa uses mathematical notation, but it's different from Andrew Ng's (e.g., theta = w).
The final lecture was the most useful, as it pulled a lot of the conceptual puzzle pieces together. The course on neural networks was a close second!
Disliked:
Although it contains mostly evergreen content, being released in 2012, it could use a refresh.
Very passive form of learning, as it wasn't immediately actionable.
Lecturer:
Dr. Yaser S. Abu-Mostafa:
Professor of Electrical Engineering and Computer Science at the California Institute of Technology
Chairman of Machine Learning Consultants LLC
Serves on a number of scientific advisory boards
Has served as a technical consultant on machine learning for several companies (including Citibank).
Multiple articles in Scientific American
Links:
https://work.caltech.edu/telecourse.html
https://itunes.apple.com/us/course/machine-learning/id515364596
Tips on Watching:
Consider listening to the last lesson first, as it pulls together the course overall conceptually. The map of the course, below, was particularly useful to organizing the information taught in the courses.
Image source: http://work.caltech.edu/slides/slides18.pdf
"Pattern Recognition & Machine Learning" by Christopher Bishop ↓
Need to Know: This is a very popular college-level machine learning textbook. I've heard it likened to a bible for machine learning. However, after spending a month trying to tackle the first few chapters, I gave up. It was too much math and pre-requisites to tackle (even with a multitude of Google sessions).
Loved:
The text of choice for many major universities, so if you can make it through this text and understand all of the concepts, you're probably in a very good position.
I appreciated the history aside sections, where Bishop talked about influential people and their career accomplishments in statistics and machine learning.
Despite being a highly mathematically text, the textbook actually has some pretty visually intuitive imagery.
Disliked:
I couldn't make it through the text, which was a bit frustrating. The statistics and mathematical notation (which is probably very benign for a student in this topic) were too much for me.
The sunk cost was pretty high here (~$75).
Writer:
Christopher Bishop:
Laboratory Director at Microsoft Research Cambridge
Professor of Computer Science at the University of Edinburgh
Fellow of Darwin College, Cambridge
PhD in Theoretical Physics from the University of Edinburgh
Links:
https://www.amazon.com/Pattern-Recognition-Learning-Information-Statistics/dp/0387310738/ref=sr_1_2?ie=UTF8&qid=1516839475&sr=8-2&keywords=Pattern+Recognition+%26+Machine+Learning
Tips on Reading:
Don't start your machine learning journey with this book.
Get a friend in statistics to walk you through anything complicated (my plan is to get a mentor in statistics).
Consider taking a (free) online statistics course (Khan Academy and Udacity both have some great content on statistics, calculus, math, and data analysis).
Machine Learning: Hands-on for Developers and Technical Professionals ↓
Need to Know: A fun, non-intimidating end-to-end launching pad/whistle stop for machine learning in action.
Loved:
Talks about practical issues that many other sources didn't really address (e.g. data-cleansing).
Covered the basics of machine learning in a non-intimidating way.
Offers abridged, consolidated versions of the content.
Added fun anecdotes that makes it easier to read.
Overall the writer has a great sense of humor.
Writer talks to the reader as if they're a real human being (i.e., doesn't expect you to go out and do proofs; acknowledges the challenge of certain concepts).
Covers a wide variety of topics.
Because it was well-written, I flew through the book (even though it's about ~300 pages).
Disliked:
N/A
Writer:
Jason Bell:
Technical architect, lecturer, and startup consultant
Data Engineer at MastodonC
Former section editor for Java Developer's Journal
Former writer on IBM DeveloperWorks
Links:
https://www.amazon.com/Machine-Learning-Hands-Developers-Professionals/dp/1118889061
https://www.wiley.com/en-us/Machine+Learning%3A+Hands+On+for+Developers+and+Technical+Professionals-p-9781118889060
Jason's Blog: https://dataissexy.wordpress.com/
Tips on Reading:
Download and explore Weka's interface beforehand.
Give some of the exercises a shot.
Introduction to Machine Learning with Python: A Guide for Data Scientists ↓
Need to Know: This was a was a well-written piece on machine learning, making it a quick read.
Loved:
Quick, smooth read.
Easy-to-follow code examples.
The first few chapters served as a stellar introduction to the basics of machine learning.
Contain subtle jokes that add a bit of fun.
Tip to use the Python package manager Anaconda with Jupyter Notebooks was helpful.
Disliked:
Once again, installation was a challenge.
The "mglearn" utility library threw me for a loop. I had to reread the first few chapters before I figured out it was support for the book.
Although I liked the book, I didn't love it. Overall it just missed the "empowering" mark.
Writers:
Andreas C. Müller:
PhD in Computer Science
Lecturer at the Data Science Institute at Columbia University
Worked at the NYU Center for Data Science on open source and open science
Former Machine Learning Scientist at Amazon
Speaks often on Machine Learning and scikit-learn (a popular machine learning library)
And he makes some pretty incredibly useful graphics, such as this scikit-learn cheat sheet:
Image source: http://peekaboo-vision.blogspot.com/2013/01/machin...
Sarah Guido:
Former senior data scientist at Mashable
Lead data scientist at Bitly
2018 SciPy Conference Data Science track co-chair
Links:
https://www.amazon.com/Introduction-Machine-Learning-Python-Scientists/dp/1449369413/ref=sr_1_7?s=books&ie=UTF8&qid=1516734322&sr=1-7&keywords=python+machine+learning
http://shop.oreilly.com/product/0636920030515.do
Tips on Reading:
Type out code examples.
Beware of the "mglearn" utility library.
Udacity: Machine Learning by Georgia Tech ↓
Need to Know: A mix between an online learning experience and a university machine learning teaching approach. The lecturers are fun, but the course still fell a bit short in terms of active learning.
Loved:
This class is offered as CS7641 at Georgia Tech, where it is a part of the Online Masters Degree. Although taking this course here will not earn credit towards the OMS degree, it's still a non-watered-down college teaching philosophy approach.
Covers a wide variety of topics, many of which reminded me of the Caltech course (including: VC Dimension versus Bayesian, Occam's razor, etc.)
Discusses Markov Decision Chains, which is something that didn't really come up in many other introductory machine learning course, but they are referenced within Google patents.
The lecturers have a great dynamic, are wicked smart, and displayed a great sense of (nerd) humor, which make the topics less intimidating.
The course has quizzes, which give the course a slight amount of interaction.
Disliked:
Some videos were very long, which made the content a bit harder to digest.
The course overall was very time consuming.
Despite the quizzes, the course was a very passive form of learning with no assignments and little coding.
Many videos started with a bunch of content already written out. Having the content written out was probably a big time-saver, but it was also a bit jarring for a viewer to see so much information all at once, while also trying to listen.
It's vital to pay very close attention to notation, which compounds in complexity quickly.
Tablet version didn't function flawlessly: some was missing content (which I had to mark down and review on a desktop), the app would crash randomly on the tablet, and sometimes the audio wouldn't start.
There were no subtitles available on tablet, which I found not only to be a major accessibility blunder, but also made it harder for me to process (since I'm not an audio learner).
Lecturer:
Michael Littman:
Professor of Computer Science at Brown University.
Was granted a patent for one of the earliest systems for Cross-language information retrieval
Perhaps the most interesting man in the world:
Been in two TEDx talks
How I Learned to Stop Worrying and Be Realistic About AI
A Cooperative Path to Artificial Intelligence
During his time at Duke, he worked on an automated crossword solver (PROVERB)
Has a Family Quartet
He has appeared in a TurboTax commercial
Charles Isbell:
Professor and Executive Associate Dean at School of Interactive Computing at Georgia Tech
Focus on statistical machine learning and "interactive" artificial intelligence.
Links:
https://www.udacity.com/course/machine-learning--ud262
Tips on Watching:
Pick specific topics of interest and focusing on those lessons.
Andrew Ng's Stanford's Machine Learning iTunes ↓
Need to Know: A non-watered-down Stanford course. It's outdated (filmed in 2008), video/audio are a bit poor, and most links online now point towards the Coursera course. Although the idea of watching a Stanford course was energizing for the first few courses, it became dreadfully boring. I made it to course six before calling it.
Loved:
Designed for students, so you know you're not missing out on anything.
This course provides a deeper study into the mathematical and theoretical foundation behind machine learning to the point that the students could create their own machine learning algorithms. This isn't necessarily very practical for the everyday machine learning user.
Has some powerful real-world examples (although they're outdated).
There is something about the kinesthetic nature of watching someone write information out. The blackboard writing helped me to process certain ideas.
Disliked:
Video and audio quality were pain to watch.
Many questions asked by students were hard to hear.
On-screen visuals range from hard to impossible to see.
Found myself counting minutes.
Dr. Ng mentions TA classes, supplementary learning, but these are not available online.
Sometimes the video showed students, which I felt was invasive.
Lecturer:
Andrew Ng (see above)
Links:
https://itunes.apple.com/us/course/machine-learning/id495053006
https://www.youtube.com/watch?v=UzxYlbK2c7E
Tips on Watching:
Only watch if you're looking to gain a deeper understanding of the math presented in the Coursera course.
Skip the first half of the first lecture, since it's mostly class logistics.
Additional Resources
Fast.ai (part 2) - free access to materials, cost for AWS EC2 instance
Deeplearning.ai - $50/month
Udacity Machine Learning Engineer Nanodegree - $1K
https://machinelearningmastery.com/
Motivations and inspiration
If you're wondering why I spent a year doing this, then I'm with you. I'm genuinely not sure why I set my sights on this project, much less why I followed through with it. I saw Mike King give a session on Machine Learning. I was caught off guard, since I knew nothing on the topic. It gave me a pesky, insatiable curiosity itch. It started with one course and then spiraled out of control. Eventually it transformed into an idea: a review guide on the most affordable and popular machine learning resources on the web (through the lens of a complete beginner). Hopefully you found it useful, or at least somewhat interesting. Be sure to share your thoughts or questions in the comments!
Sign up for The Moz Top 10, a semimonthly mailer updating you on the top ten hottest pieces of SEO news, tips, and rad links uncovered by the Moz team. Think of it as your exclusive digest of stuff you don't have time to hunt down but want to read!
https://ift.tt/2q13Myy xem thêm tại: https://ift.tt/2mb4VST để biết thêm về địa chỉ bán tai nghe không dây giá rẻ A Machine Learning Guide for Average Humans https://ift.tt/2rFyAUY xem thêm tại: https://ift.tt/2mb4VST để biết thêm về địa chỉ bán tai nghe không dây giá rẻ A Machine Learning Guide for Average Humans https://ift.tt/2rFyAUY xem thêm tại: https://ift.tt/2mb4VST để biết thêm về địa chỉ bán tai nghe không dây giá rẻ A Machine Learning Guide for Average Humans https://ift.tt/2rFyAUY xem thêm tại: https://ift.tt/2mb4VST để biết thêm về địa chỉ bán tai nghe không dây giá rẻ A Machine Learning Guide for Average Humans https://ift.tt/2rFyAUY xem thêm tại: https://ift.tt/2mb4VST để biết thêm về địa chỉ bán tai nghe không dây giá rẻ A Machine Learning Guide for Average Humans https://ift.tt/2rFyAUY xem thêm tại: https://ift.tt/2mb4VST để biết thêm về địa chỉ bán tai nghe không dây giá rẻ A Machine Learning Guide for Average Humans https://ift.tt/2rFyAUY xem thêm tại: https://ift.tt/2mb4VST để biết thêm về địa chỉ bán tai nghe không dây giá rẻ A Machine Learning Guide for Average Humans https://ift.tt/2rFyAUY xem thêm tại: https://ift.tt/2mb4VST để biết thêm về địa chỉ bán tai nghe không dây giá rẻ A Machine Learning Guide for Average Humans https://ift.tt/2rFyAUY xem thêm tại: https://ift.tt/2mb4VST để biết thêm về địa chỉ bán tai nghe không dây giá rẻ A Machine Learning Guide for Average Humans https://ift.tt/2rFyAUY xem thêm tại: https://ift.tt/2mb4VST để biết thêm về địa chỉ bán tai nghe không dây giá rẻ A Machine Learning Guide for Average Humans https://ift.tt/2rFyAUY xem thêm tại: https://ift.tt/2mb4VST để biết thêm về địa chỉ bán tai nghe không dây giá rẻ A Machine Learning Guide for Average Humans https://ift.tt/2rFyAUY xem thêm tại: https://ift.tt/2mb4VST để biết thêm về địa chỉ bán tai nghe không dây giá rẻ A Machine Learning Guide for Average Humans https://ift.tt/2rFyAUY xem thêm tại: https://ift.tt/2mb4VST để biết thêm về địa chỉ bán tai nghe không dây giá rẻ A Machine Learning Guide for Average Humans https://ift.tt/2rFyAUY xem thêm tại: https://ift.tt/2mb4VST để biết thêm về địa chỉ bán tai nghe không dây giá rẻ A Machine Learning Guide for Average Humans https://ift.tt/2rFyAUY xem thêm tại: https://ift.tt/2mb4VST để biết thêm về địa chỉ bán tai nghe không dây giá rẻ A Machine Learning Guide for Average Humans https://ift.tt/2rFyAUY xem thêm tại: https://ift.tt/2mb4VST để biết thêm về địa chỉ bán tai nghe không dây giá rẻ A Machine Learning Guide for Average Humans https://ift.tt/2rFyAUY xem thêm tại: https://ift.tt/2mb4VST để biết thêm về địa chỉ bán tai nghe không dây giá rẻ A Machine Learning Guide for Average Humans https://ift.tt/2rFyAUY xem thêm tại: https://ift.tt/2mb4VST để biết thêm về địa chỉ bán tai nghe không dây giá rẻ A Machine Learning Guide for Average Humans https://ift.tt/2rFyAUY xem thêm tại: https://ift.tt/2mb4VST để biết thêm về địa chỉ bán tai nghe không dây giá rẻ A Machine Learning Guide for Average Humans https://ift.tt/2rFyAUY xem thêm tại: https://ift.tt/2mb4VST để biết thêm về địa chỉ bán tai nghe không dây giá rẻ A Machine Learning Guide for Average Humans https://ift.tt/2rFyAUY xem thêm tại: https://ift.tt/2mb4VST để biết thêm về địa chỉ bán tai nghe không dây giá rẻ A Machine Learning Guide for Average Humans https://ift.tt/2rFyAUY xem thêm tại: https://ift.tt/2mb4VST để biết thêm về địa chỉ bán tai nghe không dây giá rẻ A Machine Learning Guide for Average Humans https://ift.tt/2rFyAUY xem thêm tại: https://ift.tt/2mb4VST để biết thêm về địa chỉ bán tai nghe không dây giá rẻ A Machine Learning Guide for Average Humans https://ift.tt/2rFyAUY xem thêm tại: https://ift.tt/2mb4VST để biết thêm về địa chỉ bán tai nghe không dây giá rẻ A Machine Learning Guide for Average Humans https://ift.tt/2rFyAUY xem thêm tại: https://ift.tt/2mb4VST để biết thêm về địa chỉ bán tai nghe không dây giá rẻ A Machine Learning Guide for Average Humans https://ift.tt/2rFyAUY xem thêm tại: https://ift.tt/2mb4VST để biết thêm về địa chỉ bán tai nghe không dây giá rẻ A Machine Learning Guide for Average Humans https://ift.tt/2rFyAUY xem thêm tại: https://ift.tt/2mb4VST để biết thêm về địa chỉ bán tai nghe không dây giá rẻ A Machine Learning Guide for Average Humans https://ift.tt/2rFyAUY xem thêm tại: https://ift.tt/2mb4VST để biết thêm về địa chỉ bán tai nghe không dây giá rẻ A Machine Learning Guide for Average Humans https://ift.tt/2rFyAUY xem thêm tại: https://ift.tt/2mb4VST để biết thêm về địa chỉ bán tai nghe không dây giá rẻ A Machine Learning Guide for Average Humans https://ift.tt/2rFyAUY xem thêm tại: https://ift.tt/2mb4VST để biết thêm về địa chỉ bán tai nghe không dây giá rẻ A Machine Learning Guide for Average Humans https://ift.tt/2rFyAUY xem thêm tại: https://ift.tt/2mb4VST để biết thêm về địa chỉ bán tai nghe không dây giá rẻ A Machine Learning Guide for Average Humans https://ift.tt/2rFyAUY xem thêm tại: https://ift.tt/2mb4VST để biết thêm về địa chỉ bán tai nghe không dây giá rẻ A Machine Learning Guide for Average Humans https://ift.tt/2rFyAUY xem thêm tại: https://ift.tt/2mb4VST để biết thêm về địa chỉ bán tai nghe không dây giá rẻ A Machine Learning Guide for Average Humans https://ift.tt/2rFyAUY xem thêm tại: https://ift.tt/2mb4VST để biết thêm về địa chỉ bán tai nghe không dây giá rẻ A Machine Learning Guide for Average Humans https://ift.tt/2rFyAUY xem thêm tại: https://ift.tt/2mb4VST để biết thêm về địa chỉ bán tai nghe không dây giá rẻ A Machine Learning Guide for Average Humans https://ift.tt/2rFyAUY xem thêm tại: https://ift.tt/2mb4VST để biết thêm về địa chỉ bán tai nghe không dây giá rẻ A Machine Learning Guide for Average Humans https://ift.tt/2rFyAUY xem thêm tại: https://ift.tt/2mb4VST để biết thêm về địa chỉ bán tai nghe không dây giá rẻ A Machine Learning Guide for Average Humans https://ift.tt/2rFyAUY xem thêm tại: https://ift.tt/2mb4VST để biết thêm về địa chỉ bán tai nghe không dây giá rẻ A Machine Learning Guide for Average Humans https://ift.tt/2rFyAUY xem thêm tại: https://ift.tt/2mb4VST để biết thêm về địa chỉ bán tai nghe không dây giá rẻ A Machine Learning Guide for Average Humans https://ift.tt/2rFyAUY xem thêm tại: https://ift.tt/2mb4VST để biết thêm về địa chỉ bán tai nghe không dây giá rẻ A Machine Learning Guide for Average Humans https://ift.tt/2rFyAUY xem thêm tại: https://ift.tt/2mb4VST để biết thêm về địa chỉ bán tai nghe không dây giá rẻ A Machine Learning Guide for Average Humans https://ift.tt/2rFyAUY xem thêm tại: https://ift.tt/2mb4VST để biết thêm về địa chỉ bán tai nghe không dây giá rẻ A Machine Learning Guide for Average Humans https://ift.tt/2rFyAUY xem thêm tại: https://ift.tt/2mb4VST để biết thêm về địa chỉ bán tai nghe không dây giá rẻ A Machine Learning Guide for Average Humans https://ift.tt/2rFyAUY xem thêm tại: https://ift.tt/2mb4VST để biết thêm về địa chỉ bán tai nghe không dây giá rẻ A Machine Learning Guide for Average Humans https://ift.tt/2rFyAUY xem thêm tại: https://ift.tt/2mb4VST để biết thêm về địa chỉ bán tai nghe không dây giá rẻ A Machine Learning Guide for Average Humans https://ift.tt/2rFyAUY xem thêm tại: https://ift.tt/2mb4VST để biết thêm về địa chỉ bán tai nghe không dây giá rẻ A Machine Learning Guide for Average Humans https://ift.tt/2rFyAUY xem thêm tại: https://ift.tt/2mb4VST để biết thêm về địa chỉ bán tai nghe không dây giá rẻ A Machine Learning Guide for Average Humans https://ift.tt/2rFyAUY xem thêm tại: https://ift.tt/2mb4VST để biết thêm về địa chỉ bán tai nghe không dây giá rẻ A Machine Learning Guide for Average Humans https://ift.tt/2rFyAUY xem thêm tại: https://ift.tt/2mb4VST để biết thêm về địa chỉ bán tai nghe không dây giá rẻ A Machine Learning Guide for Average Humans https://ift.tt/2rFyAUY xem thêm tại: https://ift.tt/2mb4VST để biết thêm về địa chỉ bán tai nghe không dây giá rẻ A Machine Learning Guide for Average Humans https://ift.tt/2rFyAUY xem thêm tại: https://ift.tt/2mb4VST để biết thêm về địa chỉ bán tai nghe không dây giá rẻ A Machine Learning Guide for Average Humans https://ift.tt/2rFyAUY xem thêm tại: https://ift.tt/2mb4VST để biết thêm về địa chỉ bán tai nghe không dây giá rẻ A Machine Learning Guide for Average Humans https://ift.tt/2rFyAUY xem thêm tại: https://ift.tt/2mb4VST để biết thêm về địa chỉ bán tai nghe không dây giá rẻ A Machine Learning Guide for Average Humans https://ift.tt/2rFyAUY xem thêm tại: https://ift.tt/2mb4VST để biết thêm về địa chỉ bán tai nghe không dây giá rẻ A Machine Learning Guide for Average Humans https://ift.tt/2rFyAUY xem thêm tại: https://ift.tt/2mb4VST để biết thêm về địa chỉ bán tai nghe không dây giá rẻ A Machine Learning Guide for Average Humans https://ift.tt/2rFyAUY xem thêm tại: https://ift.tt/2mb4VST để biết thêm về địa chỉ bán tai nghe không dây giá rẻ A Machine Learning Guide for Average Humans https://ift.tt/2rFyAUY xem thêm tại: https://ift.tt/2mb4VST để biết thêm về địa chỉ bán tai nghe không dây giá rẻ A Machine Learning Guide for Average Humans https://ift.tt/2rFyAUY xem thêm tại: https://ift.tt/2mb4VST để biết thêm về địa chỉ bán tai nghe không dây giá rẻ A Machine Learning Guide for Average Humans https://ift.tt/2rFyAUY xem thêm tại: https://ift.tt/2mb4VST để biết thêm về địa chỉ bán tai nghe không dây giá rẻ A Machine Learning Guide for Average Humans https://ift.tt/2rFyAUY xem thêm tại: https://ift.tt/2mb4VST để biết thêm về địa chỉ bán tai nghe không dây giá rẻ A Machine Learning Guide for Average Humans https://ift.tt/2rFyAUY xem thêm tại: https://ift.tt/2mb4VST để biết thêm về địa chỉ bán tai nghe không dây giá rẻ A Machine Learning Guide for Average Humans https://ift.tt/2rFyAUY xem thêm tại: https://ift.tt/2mb4VST để biết thêm về địa chỉ bán tai nghe không dây giá rẻ A Machine Learning Guide for Average Humans https://ift.tt/2rFyAUY xem thêm tại: https://ift.tt/2mb4VST để biết thêm về địa chỉ bán tai nghe không dây giá rẻ A Machine Learning Guide for Average Humans https://ift.tt/2rFyAUY xem thêm tại: https://ift.tt/2mb4VST để biết thêm về địa chỉ bán tai nghe không dây giá rẻ A Machine Learning Guide for Average Humans https://ift.tt/2rFyAUY xem thêm tại: https://ift.tt/2mb4VST để biết thêm về địa chỉ bán tai nghe không dây giá rẻ A Machine Learning Guide for Average Humans https://ift.tt/2rFyAUY xem thêm tại: https://ift.tt/2mb4VST để biết thêm về địa chỉ bán tai nghe không dây giá rẻ A Machine Learning Guide for Average Humans https://ift.tt/2rFyAUY xem thêm tại: https://ift.tt/2mb4VST để biết thêm về địa chỉ bán tai nghe không dây giá rẻ A Machine Learning Guide for Average Humans https://ift.tt/2rFyAUY xem thêm tại: https://ift.tt/2mb4VST để biết thêm về địa chỉ bán tai nghe không dây giá rẻ A Machine Learning Guide for Average Humans https://ift.tt/2rFyAUY xem thêm tại: https://ift.tt/2mb4VST để biết thêm về địa chỉ bán tai nghe không dây giá rẻ A Machine Learning Guide for Average Humans https://ift.tt/2rFyAUY xem thêm tại: https://ift.tt/2mb4VST để biết thêm về địa chỉ bán tai nghe không dây giá rẻ A Machine Learning Guide for Average Humans https://ift.tt/2rFyAUY xem thêm tại: https://ift.tt/2mb4VST để biết thêm về địa chỉ bán tai nghe không dây giá rẻ A Machine Learning Guide for Average Humans https://ift.tt/2rFyAUY xem thêm tại: https://ift.tt/2mb4VST để biết thêm về địa chỉ bán tai nghe không dây giá rẻ A Machine Learning Guide for Average Humans https://ift.tt/2rFyAUY xem thêm tại: https://ift.tt/2mb4VST để biết thêm về địa chỉ bán tai nghe không dây giá rẻ A Machine Learning Guide for Average Humans https://ift.tt/2rFyAUY xem thêm tại: https://ift.tt/2mb4VST để biết thêm về địa chỉ bán tai nghe không dây giá rẻ A Machine Learning Guide for Average Humans https://ift.tt/2rFyAUY xem thêm tại: https://ift.tt/2mb4VST để biết thêm về địa chỉ bán tai nghe không dây giá rẻ A Machine Learning Guide for Average Humans https://ift.tt/2rFyAUY xem thêm tại: https://ift.tt/2mb4VST để biết thêm về địa chỉ bán tai nghe không dây giá rẻ A Machine Learning Guide for Average Humans https://ift.tt/2rFyAUY xem thêm tại: https://ift.tt/2mb4VST để biết thêm về địa chỉ bán tai nghe không dây giá rẻ A Machine Learning Guide for Average Humans https://ift.tt/2rFyAUY xem thêm tại: https://ift.tt/2mb4VST để biết thêm về địa chỉ bán tai nghe không dây giá rẻ A Machine Learning Guide for Average Humans https://ift.tt/2rFyAUY xem thêm tại: https://ift.tt/2mb4VST để biết thêm về địa chỉ bán tai nghe không dây giá rẻ A Machine Learning Guide for Average Humans https://ift.tt/2rFyAUY xem thêm tại: https://ift.tt/2mb4VST để biết thêm về địa chỉ bán tai nghe không dây giá rẻ A Machine Learning Guide for Average Humans https://ift.tt/2rFyAUY xem thêm tại: https://ift.tt/2mb4VST để biết thêm về địa chỉ bán tai nghe không dây giá rẻ A Machine Learning Guide for Average Humans https://ift.tt/2rFyAUY xem thêm tại: https://ift.tt/2mb4VST để biết thêm về địa chỉ bán tai nghe không dây giá rẻ A Machine Learning Guide for Average Humans https://ift.tt/2rFyAUY xem thêm tại: https://ift.tt/2mb4VST để biết thêm về địa chỉ bán tai nghe không dây giá rẻ A Machine Learning Guide for Average Humans https://ift.tt/2rFyAUY xem thêm tại: https://ift.tt/2mb4VST để biết thêm về địa chỉ bán tai nghe không dây giá rẻ A Machine Learning Guide for Average Humans https://ift.tt/2rFyAUY xem thêm tại: https://ift.tt/2mb4VST để biết thêm về địa chỉ bán tai nghe không dây giá rẻ A Machine Learning Guide for Average Humans https://ift.tt/2rFyAUY Bạn có thể xem thêm địa chỉ mua tai nghe không dây tại đây https://ift.tt/2mb4VST
0 notes
Text
A Machine Learning Guide for Average Humans
Posted by alexis-sanders
//<![CDATA[ (function($) { // code using $ as alias to jQuery $(function() { // Hide the hypotext content. $('.hypotext-content').hide(); // When a hypotext link is clicked. $('a.hypotext.closed').click(function (e) { // custom handling here e.preventDefault(); // Create the class reference from the rel value. var id = '.' + $(this).attr('rel'); // If the content is hidden, show it now. if ( $(id).css('display') == 'none' ) { $(id).show('slow'); if (jQuery.ui) { // UI loaded $(id).effect("highlight", {}, 1000); } } // If the content is shown, hide it now. else { $(id).hide('slow'); } }); // If we have a hash value in the url. if (window.location.hash) { // If the anchor is within a hypotext block, expand it, by clicking the // relevant link. console.log(window.location.hash); var anchor = $(window.location.hash); var hypotextLink = $('#' + anchor.parents('.hypotext-content').attr('rel')); console.log(hypotextLink); hypotextLink.click(); // Wait until the content has expanded before jumping to anchor. //$.delay(1000); setTimeout(function(){ scrollToAnchor(window.location.hash); }, 1000); } }); function scrollToAnchor(id) { var anchor = $(id); $('html,body').animate({scrollTop: anchor.offset().top},'slow'); } })(jQuery); //]]>
Machine learning (ML) has grown consistently in worldwide prevalence. Its implications have stretched from small, seemingly inconsequential victories to groundbreaking discoveries. The SEO community is no exception. An understanding and intuition of machine learning can support our understanding of the challenges and solutions Google's engineers are facing, while also opening our minds to ML's broader implications.
The advantages of gaining an general understanding of machine learning include:
Gaining empathy for engineers, who are ultimately trying to establish the best results for users
Understanding what problems machines are solving for, their current capabilities and scientists' goals
Understanding the competitive ecosystem and how companies are using machine learning to drive results
Preparing oneself for for what many industry leaders call a major shift in our society (Andrew Ng refers to AI as a "new electricity")
Understanding basic concepts that often appear within research (it's helped me with understanding certain concepts that appear within Google Brain's Research)
Growing as an individual and expanding your horizons (you might really enjoy machine learning!)
When code works and data is produced, it's a very fulfilling, empowering feeling (even if it's a very humble result)
I spent a year taking online courses, reading books, and learning about learning (...as a machine). This post is the fruit borne of that labor -- it covers 17 machine learning resources (including online courses, books, guides, conference presentations, etc.) comprising the most affordable and popular machine learning resources on the web (through the lens of a complete beginner). I've also added a summary of "If I were to start over again, how I would approach it."
This article isn't about credit or degrees. It's about regular Joes and Joannas with an interest in machine learning, and who want to spend their learning time efficiently. Most of these resources will consume over 50 hours of commitment. Ain't nobody got time for a painful waste of a work week (especially when this is probably completed during your personal time). The goal here is for you to find the resource that best suits your learning style. I genuinely hope you find this research useful, and I encourage comments on which materials prove most helpful (especially ones not included)! #HumanLearningMachineLearning
Executive summary:
Here's everything you need to know in a chart:
Machine Learning Resource
Time (hours)
Cost ($)
Year
Credibility
Code
Math
Enjoyability
Jason Maye's Machine Learning 101 slidedeck: 2 years of headbanging, so you don't have to
2
$0
'17
{ML} Recipes with Josh Gordon Playlist
2
$0
'16
Machine Learning Crash Course
15
$0
'18
OCDevel Machine Learning Guide Podcast
30
$0
'17-
Kaggle's Machine Learning Track (part 1)
6
$0
'17
Fast.ai (part 1)
70
$70*
'16
Hands-On Machine Learning with Scikit-Learn and TensorFlow: Concepts, Tools, and Techniques to Build Intelligent Systems
20
$25
'17
Udacity's Intro to Machine Learning (Kate/Sebastian)
60
$0
'15
Andrew Ng's Coursera Machine Learning
55
$0
'11
iPullRank Machine Learning Guide
3
$0
'17
Review Google PhD
2
$0
'17
Caltech Machine Learning on iTunes
27
$0
'12
Pattern Recognition & Machine Learning by Christopher Bishop
150
$75
'06
N/A
Machine Learning: Hands-on for Developers and Technical Professionals
15
$50
'15
Introduction to Machine Learning with Python: A Guide for Data Scientists
15
$25
'16
Udacity's Machine Learning by Georgia Tech
96
$0
'15
Machine Learning Stanford iTunes by Andrew Ng
25
$0
'08
N/A
*Free, but there is the cost of running an AWS EC2 instance (~$70 when I finished, but I did tinker a ton and made a Rick and Morty script generator, which I ran many epochs [rounds] of...)
Here's my suggested program:
1. Starting out (estimated 60 hours)
Start with shorter content targeting beginners. This will allow you to get the gist of what's going on with minimal time commitment.
Commit three hours to Jason Maye's Machine Learning 101 slidedeck: 2 years of headbanging, so you don't have to.
Commit two hours to watch Google's {ML} Recipes with Josh Gordon YouTube Playlist.
Sign up for Sam DeBrule's Machine Learnings newsletter.
Work through Google's Machine Learning Crash Course.
Start listening to OCDevel's Machine Learning Guide Podcast (skip episodes 1, 3, 16, 21, and 26) in your car, working out, and/or when using hands and eyes for other activities.
Commit two days to working through Kaggle's Machine Learning Track part 1.
2. Ready to commit (estimated 80 hours)
By this point, learners would understand their interest levels. Continue with content focused on applying relevant knowledge as fast as possible.
Commit to Fast.ai 10 hours per week, for 7 weeks. If you have a friend/mentor that can help you work through AWS setup, definitely lean on any support in installation (it's 100% the worst part of ML).
Acquire Hands-On Machine Learning with Scikit-Learn and TensorFlow: Concepts, Tools, and Techniques to Build Intelligent Systems, and read the first two chapters immediately. Then use this as supplemental to the Fast.ai course.
3. Broadening your horizons (estimated 115 hours)
If you've made it through the last section and are still hungry for more knowledge, move on to broadening your horizons. Read content focused on teaching the breadth of machine learning -- building an intuition for what the algorithms are trying to accomplish (whether visual or mathematically).
Start watching videos and participating in Udacity's Intro to Machine Learning (by Sebastian Thrun and Katie Malone).
Work through Andrew Ng's Coursera Machine Learning course.
Your next steps
By this point, you will already have AWS running instances, a mathematical foundation, and an overarching view of machine learning. This is your jumping-off point to determine what you want to do.
You should be able to determine your next step based on your interest, whether it's entering Kaggle competitions; doing Fast.ai part two; diving deep into the mathematics with Pattern Recognition & Machine Learning by Christopher Bishop; giving Andrew Ng's newer Deeplearning.ai course on Coursera; learning more about specific tech stacks (TensorFlow, Scikit-Learn, Keras, Pandas, Numpy, etc.); or applying machine learning to your own problems.
Why am I recommending these steps and resources?
I am not qualified to write an article on machine learning. I don't have a PhD. I took one statistics class in college, which marked the first moment I truly understood "fight or flight" reactions. And to top it off, my coding skills are lackluster (at their best, they're chunks of reverse-engineered code from Stack Overflow). Despite my many shortcomings, this piece had to be written by someone like me, an average person.
Statistically speaking, most of us are average (ah, the bell curve/Gaussian distribution always catches up to us). Since I'm not tied to any elitist sentiments, I can be real with you. Below contains a high-level summary of my reviews on all of the classes I took, along with a plan for how I would approach learning machine learning if I could start over. Click to expand each course for the full version with notes.
In-depth reviews of machine learning courses:
Starting out
Jason Maye's Machine Learning 101 slidedeck: 2 years of head-banging, so you don't have to ↓
Need to Know: A stellar high-level overview of machine learning fundamentals in an engaging and visually stimulating format.
Loved:
Very user-friendly, engaging, and playful slidedeck.
Has the potential to take some of the pain out of the process, through introducing core concepts.
Breaks up content by beginner/need-to-know (green), and intermediate/less-useful noise (specifically for individuals starting out) (blue).
Provides resources to dive deeper into machine learning.
Provides some top people to follow in machine learning.
Disliked:
That there is not more! Jason's creativity, visual-based teaching approach, and quirky sense of humor all support the absorption of the material.
Lecturer:
Jason Mayes:
Senior Creative Technologist and Research Engineer at Google
Masters in Computer Science from University of Bristols
Personal Note: He's also kind on Twitter! :)
Links:
Machine Learning 101 slide deck
Tips on Watching:
Set aside 2-4 hours to work through the deck once.
Since there is a wealth of knowledge, refer back as needed (or as a grounding source).
Identify areas of interest and explore the resources provided.
{ML} Recipes with Josh Gordon ↓
Need to Know: This mini-series YouTube-hosted playlist covers the very fundamentals of machine learning with opportunities to complete exercises.
Loved:
It is genuinely beginner-focused.
They make no assumption of any prior knowledge.
Gloss over potentially complex topics that may serve as noise.
Playlist ~2 hours
Very high-quality filming, audio, and presentation, almost to the point where it had its own aesthetic.
Covers some examples in scikit-learn and TensorFlow, which felt modern and practical.
Josh Gordon was an engaging speaker.
Disliked:
I could not get Dockers on Windows (suggested package manager). This wasn't a huge deal, since I already had my AWS setup by this point; however, a bit of a bummer since it made it impossible to follow certain steps exactly.
Issue: Every time I tried to download (over the course of two weeks), the .exe file would recursively start and keep spinning until either my memory ran out, computer crashed, or I shut my computer down. I sent this to Docker's Twitter account to no avail.
Lecturer:
Josh Gordon:
Developer Advocate for at TensorFlow at Google
Leads Machine Learning advocacy at Google
Member of the Udacity AI & Data Industry Advisory Board
Masters in Computer Science from Columbia University
Links:
Hello World - Machine Learning Recipes #1 (YouTube)
GitHub: Machine Learning Recipes with Josh Gordon
Tips on Watching:
The playlist is short (only ~1.5 hours screen time). However, it can be a bit fast-paced at times (especially if you like mimicking the examples), so set aside 3-4 hours to play around with examples and allow time for installation, pausing, and following along.
Take time to explore code labs.
Google's Machine Learning Crash Course with TensorFlow APIs ↓
Need to Know: A Google researcher-made crash course on machine learning that is interactive and offers its own built-in coding system!
Loved:
Different formats of learning: high-quality video (with ability to adjust speed, closed captioning), readings, quizzes (with explanations), visuals (including whiteboarding), interactive components/ playgrounds, code lab exercises (run directly in your browser (no setup required!))
Non-intimidating
One of my favorite quotes: "You don't need to understand the math to be able to take a look at the graphical interpretation."
Broken down into digestible sections
Introduces key terms
Disliked:
N/A
Lecturers:
Multiple Google researchers participated in this course, including:
Peter Norvig
Director of Research at Google Inc.
Previously he directed Google's core search algorithms group.
He is co-author of Artificial Intelligence: A Modern Approach
D. Sculley
Senior Staff Software Engineer at Google
KDD award-winning papers
Works on massive-scale ML systems for online advertising
Was part of a research ML paper on optimizing chocolate chip cookies
According to his personal website, he prefers to go by "D."
Cassandra Xia
Programmer, Software Engineer at Google
She has some really cool (and cute) projects based on learning statistics concepts interactively
Maya Gupta
Leads Glassbox Machine Learning R&D team at Google
Associate Professor of Electrical Engineering at the University of Washington (2003-2012)
In 2007, Gupta received the PECASE award from President George Bush for her work in classifying uncertain (e.g. random) signals
Gupta also runs Artifact Puzzles, the second-largest US maker of wooden jigsaw puzzles
Sally Goldman
Research Scientist at Google
Co-author of A Practical Guide to Data Structures and Algorithms Using Java
Numerous journals, classes taught at Washington University, and contributions to the ML community
Links:
Machine Learning Crash Course
Tips on Doing:
Actively work through playground and coding exercises
OCDevel's Machine Learning Guide Podcast ↓
Need to Know: This podcast focuses on the high-level fundamentals of machine learning, including basic intuition, algorithms, math, languages, and frameworks. It also includes references to learn more on each episode's topic.
Loved:
Great for trips (when traveling a ton, it was an easy listen).
The podcast makes machine learning fun with interesting and compelling analogies.
Tyler is a big fan of Andrew Ng's Coursera course and reviews concepts in Coursera course very well, such that both pair together nicely.
Covers the canonical resources for learning more on a particular topic.
Disliked:
Certain courses were more theory-based; all are interesting, yet impractical.
Due to limited funding the project is a bit slow to update and has less than 30 episodes.
Podcaster:
Tyler Renelle:
Machine learning engineer focused on time series and reinforcement
Background in full-stack JavaScript, 10 years web and mobile
Creator of HabitRPG, an app that treats habits as an RPG game
Links:
Machine Learning Guide podcast
Machine Learning Guide podcast (iTunes)
Tips on Listening:
Listen along your journey to help solidify understanding of topics.
Skip episodes 1, 3, 16, 21, and 26 (unless their topics interest and inspire you!).
Kaggle Machine Learning Track (Lesson 1) ↓
Need to Know: A simple code lab that covers the very basics of machine learning with scikit-learn and Panda through the application of the examples onto another set of data.
Loved:
A more active form of learning.
An engaging code lab that encourages participants to apply knowledge.
This track offers has a built-in Python notebook on Kaggle with all input files included. This removed any and all setup/installation issues.
Side note: It's a bit different than Jupyter notebook (e.g., have to click into a cell to add another cell).
Each lesson is short, which made the entire lesson go by very fast.
Disliked:
The writing in the first lesson didn't initially make it clear that one would need to apply the knowledge in the lesson to their workbook.
It wasn't a big deal, but when I started referencing files in the lesson, I had to dive into the files in my workbook to find they didn't exist, only to realize that the knowledge was supposed to be applied and not transcribed.
Lecturer:
Dan Becker:
Data Scientist at Kaggle
Undergrad in Computer Science, PhD in Econometrics
Supervised data science consultant for six Fortune 100 companies
Contributed to the Keras and Tensorflow libraries
Finished 2nd (out of 1353 teams) in $3 million Heritage Health Prize data mining competition
Speaks at deep learning workshops at events and conferences
Links:
https://www.kaggle.com/learn/machine-learning
Tips on Doing:
Read the exercises and apply to your dataset as you go.
Try lesson 2, which covers more complex/abstract topics (note: this second took a bit longer to work through).
Ready to commit
Fast.ai (part 1 of 2) ↓
Need to Know: Hands-down the most engaging and active form of learning ML. The source I would most recommend for anyone (although the training plan does help to build up to this course). This course is about learning through coding. This is the only course that I started to truly see the practical mechanics start to come together. It involves applying the most practical solutions to the most common problems (while also building an intuition for those solutions).
Loved:
Course Philosophy:
Active learning approach
"Go out into the world and understand underlying mechanics (of machine learning by doing)."
Counter-culture to the exclusivity of the machine learning field, focusing on inclusion.
"Let's do shit that matters to people as quickly as possible."
Highly pragmatic approach with tools that are currently being used (Jupyter Notebooks, scikit-learn, Keras, AWS, etc.).
Show an end-to-end process that you get to complete and play with in a development environment.
Math is involved, but is not prohibitive. Excel files helped to consolidate information/interact with information in a different way, and Jeremy spends a lot of time recapping confusing concepts.
Amazing set of learning resources that allow for all different styles of learning, including:
Video Lessons
Notes
Jupyter Notebooks
Assignments
Highly active forums
Resources on Stackoverflow
Readings/resources
Jeremy often references popular academic texts
Jeremy's TEDx talk in Brussels
Jeremy really pushes one to do extra and put in the effort by teaching interesting problems and engaging one in solving them.
It's a huge time commitment; however, it's worth it.
All of the course's profits are donated.
Disliked:
Overview covers their approach to learning (obviously I'm a fan!). If you're already drinking the Kool-aid, skip past.
I struggled through the AWS setup (13-minute video) for about five hours (however, it felt so good when it was up and running!).
Because of its practicality and concentration on solutions used today to solve popular problem types (image recognition, text generation, etc.), it lacks breadth of machine learning topics.
Lecturers:
Jeremy Howard:
Distinguished Research Scientist at the University of San Francisco
Faculty member at Singularity University
Young Global Leader with the World Economic Forum
Founder of Enlitic (the first company to apply deep learning to medicine)
Former President and Chief Scientist of the data science platform Kaggle
Rachel Thomas:
PhD in Math from Duke
One of Forbes' "20 Incredible Women Advancing AI Research"
Researcher-in-residence at the University of San Francisco Data Institute
Teaches in the Masters in Data Science program
Links:
http://course.fast.ai/start.html
http://wiki.fast.ai/index.php/Main_Page
https://github.com/fastai/courses/tree/master/deeplearning1/nbs
Tips on Doing:
Set expectations with yourself that installation is going to probably take a few hours.
Prepare to spend about ~70 hours for this course (it's worth it).
Don't forget to shut off your AWS instance.
Balance out machine learning knowledge with a course with more breadth.
Consider giving part two of the Fast.ai program a shot!
Hands-On Machine Learning with Scikit-Learn and TensorFlow: Concepts, Tools, and Techniques to Build Intelligent Systems ↓
Need to Know: This book is an Amazon best seller for a reason. It covers a lot of ground quickly, empowers readers to walk through a machine learning problem by chapter two, and contains practical up-to-date machine learning skills.
Loved:
Book contains an amazing introduction to machine learning that briskly provides an overarching quick view of the machine learning ecosystem.
Chapter 2 immediately walks the reader through an end-to-end machine learning problem.
Immediately afterwards, Aurélien pushes a user to attempt to apply this solution to another problem, which was very empowering.
There are review questions at the end of each chapter to ensure on has grasped the content within the chapter and to push the reader to explore more.
Once installation was completed, it was easy to follow and all code is available on GitHub.
Chapters 11-14 were very tough reading; however, they were a great reference when working through Fast.ai.
Contains some powerful analogies.
Each chapter's introductions were very useful and put everything into context. This general-to-specifics learning was very useful.
Disliked:
Installation was a common source of issues during the beginning of my journey; the text glided over this. I felt the frustration that most people experience from installation should have been addressed with more resources.
Writer:
Aurélien Géron:
Led the YouTube video classification team from 2013 to 2016
Currently a machine Learning consultant
Founder and CTO of Wifirst and Polyconseil
Published technical books (on C++, Wi-Fi, and Internet architectures)
Links:
https://www.amazon.com/_/dp/1491962291?tag=oreilly20-20
http://shop.oreilly.com/product/0636920052289.do
https://github.com/ageron/handson-ml
Tips on Using:
Get a friend with Python experience to help with installation.
Read the introductions to each chapter thoroughly, read the chapter (pay careful attention to code), review the questions at the end (highlight any in-text answer), make a copy of Aurélien's GitHub and make sure everything works on your setup, re-type the notebooks, go to Kaggle and try on other datasets.
Broadening your horizons
Udacity: Intro to Machine Learning (Kate/Sebastian) ↓
Need to Know: A course that covers a range of machine learning topics, supports building of intuition via visualization and simple examples, offers coding challenges, and a certificate (upon completion of a final project). The biggest challenge with this course is bridging the gap between the hand-holding lectures and the coding exercises.
Loved:
Focus on developing a visual intuition on what each model is trying to accomplish.
This visual learning mathematics approach is very useful.
Cover a vast variety and breadth of models and machine learning basics.
In terms of presenting the concept, there was a lot of hand-holding (which I completely appreciated!).
Many people have done this training, so their GitHub accounts can be used as reference for the mini-projects.
Katie actively notes documentation and suggests where viewers can learn more/reference material.
Disliked:
All of the conceptual hand-holding in the lessons is a stark contrast to the challenges of installation, coding exercises, and mini-projects.
This is the first course started and the limited instructions on setting up the environment and many failed attempts caused me to break down crying at least a handful of times.
The mini-projects are intimidating.
There is extra code added to support the viewers; however, it's done so with little acknowledgement as to what it's actually doing. This made learning a bit harder.
Lecturer:
Caitlin (Katie) Malone:
Director of Data Science Research and Development at Civis Analytics
Stanford PhD in Experimental Particle Physics
Intern at Udacity in summer 2014
Graduate Researcher at the SLAC National Accelerator Laboratory
https://www6.slac.stanford.edu/
Podcaster with Ben Jaffe (currently Facebook UI Engineer and a music aficionado) on a machine learning podcast Linear Digressions (100+ episodes)
Sebastian Thrun:
CEO of the Kitty Hawk Corporation
Chairman and co-founder of Udacity
One of my favorite Sebastian quotes: "It occurred to me, I could be at Google and build a self-driving car, or I can teach 10,000 students how to build self-driving cars."
Former Google VP
Founded Google X
Led development of the robotic vehicle Stanley
Professor of Computer Science at Stanford University
Formerly a professor at Carnegie Mellon University.
Links:
https://www.udacity.com/course/intro-to-machine-learning--ud120
Udacity also offers a next step, the Machine Learning Engineer Nanodegree, which will set one back about $1K.
Tips on Watching:
Get a friend to help you set up your environment.
Print mini-project instructions to check off each step.
Andrew Ng's Coursera Machine Learning Course ↓
Need to Know: The Andrew Ng Coursera course is the most referenced online machine learning course. It covers a broad set of fundamental, evergreen topics with a strong focus in building mathematical intuition behind machine learning models. Also, one can submit assignments and earn a grade for free. If you want to earn a certificate, one can subscribe or apply for financial aid.
Loved:
This course has a high level of credibility.
Introduces all necessary machine learning terminology and jargon.
Contains a very classic machine learning education approach with a high level of math focus.
Quizzes interspersed in courses and after each lesson support understanding and overall learning.
The sessions for the course are flexible, the option to switch into a different section is always available.
Disliked:
The mathematic notation was hard to process at times.
The content felt a bit dated and non-pragmatic. For example, the main concentration was MATLAB and Octave versus more modern languages and resources.
Video quality was less than average and could use a refresh.
Lecturer:
Andrew Ng:
Adjunct Professor, Stanford University (focusing on AI, Machine Learning, and Deep Learning)
Co-founder of Coursera
Former head of Baidu AI Group
Founder and previous head of Google Brain (deep learning) project
Former Director of the Stanford AI Lab
Chairman of the board of Woebot (a machine learning bot that focuses on Cognitive Behavior Therapy)
Links:
https://www.coursera.org/learn/machine-learning/
Andrew Ng recently launched a new course (August 2017) called DeepLearning.ai, a ~15 week course containing five mini-courses ($49 USD per month to continue learning after trial period of 7 days ends).
Course: https://www.coursera.org/specializations/deep-learning
Course 1: Neural Networks and Deep Learning
Course 2: Improving Deep Neural Networks: Hyperparameter tuning, Regularization and Optimization
Course 3: Structuring Machine Learning Projects
Course 4: Convolutional Neural Networks
Course 5: Sequence Models
Tips on Watching:
Be disciplined with setting aside timing (even if it's only 15 minutes a day) to help power through some of the more boring concepts.
Don't do this course first, because it's intimidating, requires a large time commitment, and isn't a very energizing experience.
Additional machine learning opportunities
iPullRank Machine Learning Guide ↓
Need to Know: A machine learning e-book targeted at marketers.
Loved:
Targeted at marketers and applied to organic search.
Covers a variety of machine learning topics.
Some good examples, including real-world blunders.
Gives some practical tools for non-data scientists (including: MonkeyLearn and Orange)
I found Orange to be a lot of fun. It struggled with larger datasets; however, it has a very visual interface that was more user-friendly and offers potential to show some pretty compelling stories.
Example: World Happiness Dataset by:
X-axis: Happiness Score
Y-axis: Economy
Color: Health
Disliked:
Potential to break up content more with relevant imagery -- the content was very dense.
Writers:
iPullRank Team (including Mike King):
Mike King has a few slide decks on the basics of machine learnings and AI
iPullRank has a few data scientists on staff
Links:
http://ipullrank.com/machine-learning-guide/
Tips on Reading:
Read chapters 1-6 and the rest depending upon personal interest.
Review Google PhD ↓
Need to Know: A two-hour presentation from Google's 2017 IO conference that walks through getting 99% accuracy on the MNIST dataset (a famous dataset containing a bunch of handwritten numbers, which the machine must learn to identify the numbers).
Loved:
This talk struck me as very modern, covering the cutting edge.
Found this to be very complementary to Fast.ai, as it covered similar topics (e.g. ReLu, CNNs, RNNs, etc.)
Amazing visuals that help to put everything into context.
Disliked:
The presentation is only a short conference solution and not a comprehensive view of machine learning.
Also, a passive form of learning.
Presenter:
Martin Görner:
Developer Relations, Google (since 2011)
Started Mobipocket, a startup that later became the software part of the Amazon Kindle and its mobile variants
Links:
Part 1 - https://www.youtube.com/watch?v=u4alGiomYP4
Part 2 - https://www.youtube.com/watch?v=fTUwdXUFfI8
Tips on Watching:
Google any concepts you're unfamiliar with.
Take your time with this one; 2 hours of screen time doesn't count all of the Googling and processing time for this one.
Caltech Machine Learning iTunes ↓
Need to Know: If math is your thing, this course does a stellar job of building the mathematic intuition behind many machine learning models. Dr. Abu-Mostafa is a raconteur, includes useful visualizations, relevant real-world examples, and compelling analogies.
Loved:
First and foremost, this is a real Caltech course, meaning it's not a watered-down version and contains fundamental concepts that are vital to understanding the mechanics of machine learning.
On iTunes, audio downloads are available, which can be useful for on-the-go learning.
Dr. Abu-Mostafa is a skilled speaker, making the 27 hours spent listening much easier!
Dr. Abu-Mostafa offers up some strong real-world examples and analogies which makes the content more relatable.
As an example, he asks students: "Why do I give you practice exams and not just give you the final exam?" as an illustration of why a testing set is useful. If he were to just give students the final, they would just memorize the answers (i.e., they would overfit to the data) and not genuinely learn the material. The final is a test to show how much students learn.
The last 1/2 hour of the class is always a Q&A, where students can ask questions. Their questions were useful to understanding the topic more in-depth.
The video and audio quality was strong throughout. There were a few times when I couldn't understand a question in the Q&A, but overall very strong.
This course is designed to build mathematical intuition of what's going on under the hood of specific machine learning models.
Caution: Dr. Abu-Mostafa uses mathematical notation, but it's different from Andrew Ng's (e.g., theta = w).
The final lecture was the most useful, as it pulled a lot of the conceptual puzzle pieces together. The course on neural networks was a close second!
Disliked:
Although it contains mostly evergreen content, being released in 2012, it could use a refresh.
Very passive form of learning, as it wasn't immediately actionable.
Lecturer:
Dr. Yaser S. Abu-Mostafa:
Professor of Electrical Engineering and Computer Science at the California Institute of Technology
Chairman of Machine Learning Consultants LLC
Serves on a number of scientific advisory boards
Has served as a technical consultant on machine learning for several companies (including Citibank).
Multiple articles in Scientific American
Links:
https://work.caltech.edu/telecourse.html
https://itunes.apple.com/us/course/machine-learning/id515364596
Tips on Watching:
Consider listening to the last lesson first, as it pulls together the course overall conceptually. The map of the course, below, was particularly useful to organizing the information taught in the courses.
Image source: http://work.caltech.edu/slides/slides18.pdf
"Pattern Recognition & Machine Learning" by Christopher Bishop ↓
Need to Know: This is a very popular college-level machine learning textbook. I've heard it likened to a bible for machine learning. However, after spending a month trying to tackle the first few chapters, I gave up. It was too much math and pre-requisites to tackle (even with a multitude of Google sessions).
Loved:
The text of choice for many major universities, so if you can make it through this text and understand all of the concepts, you're probably in a very good position.
I appreciated the history aside sections, where Bishop talked about influential people and their career accomplishments in statistics and machine learning.
Despite being a highly mathematically text, the textbook actually has some pretty visually intuitive imagery.
Disliked:
I couldn't make it through the text, which was a bit frustrating. The statistics and mathematical notation (which is probably very benign for a student in this topic) were too much for me.
The sunk cost was pretty high here (~$75).
Writer:
Christopher Bishop:
Laboratory Director at Microsoft Research Cambridge
Professor of Computer Science at the University of Edinburgh
Fellow of Darwin College, Cambridge
PhD in Theoretical Physics from the University of Edinburgh
Links:
https://www.amazon.com/Pattern-Recognition-Learning-Information-Statistics/dp/0387310738/ref=sr_1_2?ie=UTF8&qid=1516839475&sr=8-2&keywords=Pattern+Recognition+%26+Machine+Learning
Tips on Reading:
Don't start your machine learning journey with this book.
Get a friend in statistics to walk you through anything complicated (my plan is to get a mentor in statistics).
Consider taking a (free) online statistics course (Khan Academy and Udacity both have some great content on statistics, calculus, math, and data analysis).
Machine Learning: Hands-on for Developers and Technical Professionals ↓
Need to Know: A fun, non-intimidating end-to-end launching pad/whistle stop for machine learning in action.
Loved:
Talks about practical issues that many other sources didn't really address (e.g. data-cleansing).
Covered the basics of machine learning in a non-intimidating way.
Offers abridged, consolidated versions of the content.
Added fun anecdotes that makes it easier to read.
Overall the writer has a great sense of humor.
Writer talks to the reader as if they're a real human being (i.e., doesn't expect you to go out and do proofs; acknowledges the challenge of certain concepts).
Covers a wide variety of topics.
Because it was well-written, I flew through the book (even though it's about ~300 pages).
Disliked:
N/A
Writer:
Jason Bell:
Technical architect, lecturer, and startup consultant
Data Engineer at MastodonC
Former section editor for Java Developer's Journal
Former writer on IBM DeveloperWorks
Links:
https://www.amazon.com/Machine-Learning-Hands-Developers-Professionals/dp/1118889061
https://www.wiley.com/en-us/Machine+Learning%3A+Hands+On+for+Developers+and+Technical+Professionals-p-9781118889060
Jason's Blog: https://dataissexy.wordpress.com/
Tips on Reading:
Download and explore Weka's interface beforehand.
Give some of the exercises a shot.
Introduction to Machine Learning with Python: A Guide for Data Scientists ↓
Need to Know: This was a was a well-written piece on machine learning, making it a quick read.
Loved:
Quick, smooth read.
Easy-to-follow code examples.
The first few chapters served as a stellar introduction to the basics of machine learning.
Contain subtle jokes that add a bit of fun.
Tip to use the Python package manager Anaconda with Jupyter Notebooks was helpful.
Disliked:
Once again, installation was a challenge.
The "mglearn" utility library threw me for a loop. I had to reread the first few chapters before I figured out it was support for the book.
Although I liked the book, I didn't love it. Overall it just missed the "empowering" mark.
Writers:
Andreas C. Müller:
PhD in Computer Science
Lecturer at the Data Science Institute at Columbia University
Worked at the NYU Center for Data Science on open source and open science
Former Machine Learning Scientist at Amazon
Speaks often on Machine Learning and scikit-learn (a popular machine learning library)
And he makes some pretty incredibly useful graphics, such as this scikit-learn cheat sheet:
Image source: http://peekaboo-vision.blogspot.com/2013/01/machin...
Sarah Guido:
Former senior data scientist at Mashable
Lead data scientist at Bitly
2018 SciPy Conference Data Science track co-chair
Links:
https://www.amazon.com/Introduction-Machine-Learning-Python-Scientists/dp/1449369413/ref=sr_1_7?s=books&ie=UTF8&qid=1516734322&sr=1-7&keywords=python+machine+learning
http://shop.oreilly.com/product/0636920030515.do
Tips on Reading:
Type out code examples.
Beware of the "mglearn" utility library.
Udacity: Machine Learning by Georgia Tech ↓
Need to Know: A mix between an online learning experience and a university machine learning teaching approach. The lecturers are fun, but the course still fell a bit short in terms of active learning.
Loved:
This class is offered as CS7641 at Georgia Tech, where it is a part of the Online Masters Degree. Although taking this course here will not earn credit towards the OMS degree, it's still a non-watered-down college teaching philosophy approach.
Covers a wide variety of topics, many of which reminded me of the Caltech course (including: VC Dimension versus Bayesian, Occam's razor, etc.)
Discusses Markov Decision Chains, which is something that didn't really come up in many other introductory machine learning course, but they are referenced within Google patents.
The lecturers have a great dynamic, are wicked smart, and displayed a great sense of (nerd) humor, which make the topics less intimidating.
The course has quizzes, which give the course a slight amount of interaction.
Disliked:
Some videos were very long, which made the content a bit harder to digest.
The course overall was very time consuming.
Despite the quizzes, the course was a very passive form of learning with no assignments and little coding.
Many videos started with a bunch of content already written out. Having the content written out was probably a big time-saver, but it was also a bit jarring for a viewer to see so much information all at once, while also trying to listen.
It's vital to pay very close attention to notation, which compounds in complexity quickly.
Tablet version didn't function flawlessly: some was missing content (which I had to mark down and review on a desktop), the app would crash randomly on the tablet, and sometimes the audio wouldn't start.
There were no subtitles available on tablet, which I found not only to be a major accessibility blunder, but also made it harder for me to process (since I'm not an audio learner).
Lecturer:
Michael Littman:
Professor of Computer Science at Brown University.
Was granted a patent for one of the earliest systems for Cross-language information retrieval
Perhaps the most interesting man in the world:
Been in two TEDx talks
How I Learned to Stop Worrying and Be Realistic About AI
A Cooperative Path to Artificial Intelligence
During his time at Duke, he worked on an automated crossword solver (PROVERB)
Has a Family Quartet
He has appeared in a TurboTax commercial
Charles Isbell:
Professor and Executive Associate Dean at School of Interactive Computing at Georgia Tech
Focus on statistical machine learning and "interactive" artificial intelligence.
Links:
https://www.udacity.com/course/machine-learning--ud262
Tips on Watching:
Pick specific topics of interest and focusing on those lessons.
Andrew Ng's Stanford's Machine Learning iTunes ↓
Need to Know: A non-watered-down Stanford course. It's outdated (filmed in 2008), video/audio are a bit poor, and most links online now point towards the Coursera course. Although the idea of watching a Stanford course was energizing for the first few courses, it became dreadfully boring. I made it to course six before calling it.
Loved:
Designed for students, so you know you're not missing out on anything.
This course provides a deeper study into the mathematical and theoretical foundation behind machine learning to the point that the students could create their own machine learning algorithms. This isn't necessarily very practical for the everyday machine learning user.
Has some powerful real-world examples (although they're outdated).
There is something about the kinesthetic nature of watching someone write information out. The blackboard writing helped me to process certain ideas.
Disliked:
Video and audio quality were pain to watch.
Many questions asked by students were hard to hear.
On-screen visuals range from hard to impossible to see.
Found myself counting minutes.
Dr. Ng mentions TA classes, supplementary learning, but these are not available online.
Sometimes the video showed students, which I felt was invasive.
Lecturer:
Andrew Ng (see above)
Links:
https://itunes.apple.com/us/course/machine-learning/id495053006
https://www.youtube.com/watch?v=UzxYlbK2c7E
Tips on Watching:
Only watch if you're looking to gain a deeper understanding of the math presented in the Coursera course.
Skip the first half of the first lecture, since it's mostly class logistics.
Additional Resources
Fast.ai (part 2) - free access to materials, cost for AWS EC2 instance
Deeplearning.ai - $50/month
Udacity Machine Learning Engineer Nanodegree - $1K
https://machinelearningmastery.com/
Motivations and inspiration
If you're wondering why I spent a year doing this, then I'm with you. I'm genuinely not sure why I set my sights on this project, much less why I followed through with it. I saw Mike King give a session on Machine Learning. I was caught off guard, since I knew nothing on the topic. It gave me a pesky, insatiable curiosity itch. It started with one course and then spiraled out of control. Eventually it transformed into an idea: a review guide on the most affordable and popular machine learning resources on the web (through the lens of a complete beginner). Hopefully you found it useful, or at least somewhat interesting. Be sure to share your thoughts or questions in the comments!
Sign up for The Moz Top 10, a semimonthly mailer updating you on the top ten hottest pieces of SEO news, tips, and rad links uncovered by the Moz team. Think of it as your exclusive digest of stuff you don't have time to hunt down but want to read!
0 notes
Text
A Machine Learning Guide for Average Humans
Posted by alexis-sanders
//<![CDATA[ (function($) { // code using $ as alias to jQuery $(function() { // Hide the hypotext content. $('.hypotext-content').hide(); // When a hypotext link is clicked. $('a.hypotext.closed').click(function (e) { // custom handling here e.preventDefault(); // Create the class reference from the rel value. var id = '.' + $(this).attr('rel'); // If the content is hidden, show it now. if ( $(id).css('display') == 'none' ) { $(id).show('slow'); if (jQuery.ui) { // UI loaded $(id).effect("highlight", {}, 1000); } } // If the content is shown, hide it now. else { $(id).hide('slow'); } }); // If we have a hash value in the url. if (window.location.hash) { // If the anchor is within a hypotext block, expand it, by clicking the // relevant link. console.log(window.location.hash); var anchor = $(window.location.hash); var hypotextLink = $('#' + anchor.parents('.hypotext-content').attr('rel')); console.log(hypotextLink); hypotextLink.click(); // Wait until the content has expanded before jumping to anchor. //$.delay(1000); setTimeout(function(){ scrollToAnchor(window.location.hash); }, 1000); } }); function scrollToAnchor(id) { var anchor = $(id); $('html,body').animate({scrollTop: anchor.offset().top},'slow'); } })(jQuery); //]]>
Machine learning (ML) has grown consistently in worldwide prevalence. Its implications have stretched from small, seemingly inconsequential victories to groundbreaking discoveries. The SEO community is no exception. An understanding and intuition of machine learning can support our understanding of the challenges and solutions Google's engineers are facing, while also opening our minds to ML's broader implications.
The advantages of gaining an general understanding of machine learning include:
Gaining empathy for engineers, who are ultimately trying to establish the best results for users
Understanding what problems machines are solving for, their current capabilities and scientists' goals
Understanding the competitive ecosystem and how companies are using machine learning to drive results
Preparing oneself for for what many industry leaders call a major shift in our society (Andrew Ng refers to AI as a "new electricity")
Understanding basic concepts that often appear within research (it's helped me with understanding certain concepts that appear within Google Brain's Research)
Growing as an individual and expanding your horizons (you might really enjoy machine learning!)
When code works and data is produced, it's a very fulfilling, empowering feeling (even if it's a very humble result)
I spent a year taking online courses, reading books, and learning about learning (...as a machine). This post is the fruit borne of that labor -- it covers 17 machine learning resources (including online courses, books, guides, conference presentations, etc.) comprising the most affordable and popular machine learning resources on the web (through the lens of a complete beginner). I've also added a summary of "If I were to start over again, how I would approach it."
This article isn't about credit or degrees. It's about regular Joes and Joannas with an interest in machine learning, and who want to spend their learning time efficiently. Most of these resources will consume over 50 hours of commitment. Ain't nobody got time for a painful waste of a work week (especially when this is probably completed during your personal time). The goal here is for you to find the resource that best suits your learning style. I genuinely hope you find this research useful, and I encourage comments on which materials prove most helpful (especially ones not included)! #HumanLearningMachineLearning
Executive summary:
Here's everything you need to know in a chart:
Machine Learning Resource
Time (hours)
Cost ($)
Year
Credibility
Code
Math
Enjoyability
Jason Maye's Machine Learning 101 slidedeck: 2 years of headbanging, so you don't have to
2
$0
'17
{ML} Recipes with Josh Gordon Playlist
2
$0
'16
Machine Learning Crash Course
15
$0
'18
OCDevel Machine Learning Guide Podcast
30
$0
'17-
Kaggle's Machine Learning Track (part 1)
6
$0
'17
Fast.ai (part 1)
70
$70*
'16
Hands-On Machine Learning with Scikit-Learn and TensorFlow: Concepts, Tools, and Techniques to Build Intelligent Systems
20
$25
'17
Udacity's Intro to Machine Learning (Kate/Sebastian)
60
$0
'15
Andrew Ng's Coursera Machine Learning
55
$0
'11
iPullRank Machine Learning Guide
3
$0
'17
Review Google PhD
2
$0
'17
Caltech Machine Learning on iTunes
27
$0
'12
Pattern Recognition & Machine Learning by Christopher Bishop
150
$75
'06
N/A
Machine Learning: Hands-on for Developers and Technical Professionals
15
$50
'15
Introduction to Machine Learning with Python: A Guide for Data Scientists
15
$25
'16
Udacity's Machine Learning by Georgia Tech
96
$0
'15
Machine Learning Stanford iTunes by Andrew Ng
25
$0
'08
N/A
*Free, but there is the cost of running an AWS EC2 instance (~$70 when I finished, but I did tinker a ton and made a Rick and Morty script generator, which I ran many epochs [rounds] of...)
Here's my suggested program:
1. Starting out (estimated 60 hours)
Start with shorter content targeting beginners. This will allow you to get the gist of what's going on with minimal time commitment.
Commit three hours to Jason Maye's Machine Learning 101 slidedeck: 2 years of headbanging, so you don't have to.
Commit two hours to watch Google's {ML} Recipes with Josh Gordon YouTube Playlist.
Sign up for Sam DeBrule's Machine Learnings newsletter.
Work through Google's Machine Learning Crash Course.
Start listening to OCDevel's Machine Learning Guide Podcast (skip episodes 1, 3, 16, 21, and 26) in your car, working out, and/or when using hands and eyes for other activities.
Commit two days to working through Kaggle's Machine Learning Track part 1.
2. Ready to commit (estimated 80 hours)
By this point, learners would understand their interest levels. Continue with content focused on applying relevant knowledge as fast as possible.
Commit to Fast.ai 10 hours per week, for 7 weeks. If you have a friend/mentor that can help you work through AWS setup, definitely lean on any support in installation (it's 100% the worst part of ML).
Acquire Hands-On Machine Learning with Scikit-Learn and TensorFlow: Concepts, Tools, and Techniques to Build Intelligent Systems, and read the first two chapters immediately. Then use this as supplemental to the Fast.ai course.
3. Broadening your horizons (estimated 115 hours)
If you've made it through the last section and are still hungry for more knowledge, move on to broadening your horizons. Read content focused on teaching the breadth of machine learning -- building an intuition for what the algorithms are trying to accomplish (whether visual or mathematically).
Start watching videos and participating in Udacity's Intro to Machine Learning (by Sebastian Thrun and Katie Malone).
Work through Andrew Ng's Coursera Machine Learning course.
Your next steps
By this point, you will already have AWS running instances, a mathematical foundation, and an overarching view of machine learning. This is your jumping-off point to determine what you want to do.
You should be able to determine your next step based on your interest, whether it's entering Kaggle competitions; doing Fast.ai part two; diving deep into the mathematics with Pattern Recognition & Machine Learning by Christopher Bishop; giving Andrew Ng's newer Deeplearning.ai course on Coursera; learning more about specific tech stacks (TensorFlow, Scikit-Learn, Keras, Pandas, Numpy, etc.); or applying machine learning to your own problems.
Why am I recommending these steps and resources?
I am not qualified to write an article on machine learning. I don't have a PhD. I took one statistics class in college, which marked the first moment I truly understood "fight or flight" reactions. And to top it off, my coding skills are lackluster (at their best, they're chunks of reverse-engineered code from Stack Overflow). Despite my many shortcomings, this piece had to be written by someone like me, an average person.
Statistically speaking, most of us are average (ah, the bell curve/Gaussian distribution always catches up to us). Since I'm not tied to any elitist sentiments, I can be real with you. Below contains a high-level summary of my reviews on all of the classes I took, along with a plan for how I would approach learning machine learning if I could start over. Click to expand each course for the full version with notes.
In-depth reviews of machine learning courses:
Starting out
Jason Maye's Machine Learning 101 slidedeck: 2 years of head-banging, so you don't have to ↓
Need to Know: A stellar high-level overview of machine learning fundamentals in an engaging and visually stimulating format.
Loved:
Very user-friendly, engaging, and playful slidedeck.
Has the potential to take some of the pain out of the process, through introducing core concepts.
Breaks up content by beginner/need-to-know (green), and intermediate/less-useful noise (specifically for individuals starting out) (blue).
Provides resources to dive deeper into machine learning.
Provides some top people to follow in machine learning.
Disliked:
That there is not more! Jason's creativity, visual-based teaching approach, and quirky sense of humor all support the absorption of the material.
Lecturer:
Jason Mayes:
Senior Creative Technologist and Research Engineer at Google
Masters in Computer Science from University of Bristols
Personal Note: He's also kind on Twitter! :)
Links:
Machine Learning 101 slide deck
Tips on Watching:
Set aside 2-4 hours to work through the deck once.
Since there is a wealth of knowledge, refer back as needed (or as a grounding source).
Identify areas of interest and explore the resources provided.
{ML} Recipes with Josh Gordon ↓
Need to Know: This mini-series YouTube-hosted playlist covers the very fundamentals of machine learning with opportunities to complete exercises.
Loved:
It is genuinely beginner-focused.
They make no assumption of any prior knowledge.
Gloss over potentially complex topics that may serve as noise.
Playlist ~2 hours
Very high-quality filming, audio, and presentation, almost to the point where it had its own aesthetic.
Covers some examples in scikit-learn and TensorFlow, which felt modern and practical.
Josh Gordon was an engaging speaker.
Disliked:
I could not get Dockers on Windows (suggested package manager). This wasn't a huge deal, since I already had my AWS setup by this point; however, a bit of a bummer since it made it impossible to follow certain steps exactly.
Issue: Every time I tried to download (over the course of two weeks), the .exe file would recursively start and keep spinning until either my memory ran out, computer crashed, or I shut my computer down. I sent this to Docker's Twitter account to no avail.
Lecturer:
Josh Gordon:
Developer Advocate for at TensorFlow at Google
Leads Machine Learning advocacy at Google
Member of the Udacity AI & Data Industry Advisory Board
Masters in Computer Science from Columbia University
Links:
Hello World - Machine Learning Recipes #1 (YouTube)
GitHub: Machine Learning Recipes with Josh Gordon
Tips on Watching:
The playlist is short (only ~1.5 hours screen time). However, it can be a bit fast-paced at times (especially if you like mimicking the examples), so set aside 3-4 hours to play around with examples and allow time for installation, pausing, and following along.
Take time to explore code labs.
Google's Machine Learning Crash Course with TensorFlow APIs ↓
Need to Know: A Google researcher-made crash course on machine learning that is interactive and offers its own built-in coding system!
Loved:
Different formats of learning: high-quality video (with ability to adjust speed, closed captioning), readings, quizzes (with explanations), visuals (including whiteboarding), interactive components/ playgrounds, code lab exercises (run directly in your browser (no setup required!))
Non-intimidating
One of my favorite quotes: "You don't need to understand the math to be able to take a look at the graphical interpretation."
Broken down into digestible sections
Introduces key terms
Disliked:
N/A
Lecturers:
Multiple Google researchers participated in this course, including:
Peter Norvig
Director of Research at Google Inc.
Previously he directed Google's core search algorithms group.
He is co-author of Artificial Intelligence: A Modern Approach
D. Sculley
Senior Staff Software Engineer at Google
KDD award-winning papers
Works on massive-scale ML systems for online advertising
Was part of a research ML paper on optimizing chocolate chip cookies
According to his personal website, he prefers to go by "D."
Cassandra Xia
Programmer, Software Engineer at Google
She has some really cool (and cute) projects based on learning statistics concepts interactively
Maya Gupta
Leads Glassbox Machine Learning R&D team at Google
Associate Professor of Electrical Engineering at the University of Washington (2003-2012)
In 2007, Gupta received the PECASE award from President George Bush for her work in classifying uncertain (e.g. random) signals
Gupta also runs Artifact Puzzles, the second-largest US maker of wooden jigsaw puzzles
Sally Goldman
Research Scientist at Google
Co-author of A Practical Guide to Data Structures and Algorithms Using Java
Numerous journals, classes taught at Washington University, and contributions to the ML community
Links:
Machine Learning Crash Course
Tips on Doing:
Actively work through playground and coding exercises
OCDevel's Machine Learning Guide Podcast ↓
Need to Know: This podcast focuses on the high-level fundamentals of machine learning, including basic intuition, algorithms, math, languages, and frameworks. It also includes references to learn more on each episode's topic.
Loved:
Great for trips (when traveling a ton, it was an easy listen).
The podcast makes machine learning fun with interesting and compelling analogies.
Tyler is a big fan of Andrew Ng's Coursera course and reviews concepts in Coursera course very well, such that both pair together nicely.
Covers the canonical resources for learning more on a particular topic.
Disliked:
Certain courses were more theory-based; all are interesting, yet impractical.
Due to limited funding the project is a bit slow to update and has less than 30 episodes.
Podcaster:
Tyler Renelle:
Machine learning engineer focused on time series and reinforcement
Background in full-stack JavaScript, 10 years web and mobile
Creator of HabitRPG, an app that treats habits as an RPG game
Links:
Machine Learning Guide podcast
Machine Learning Guide podcast (iTunes)
Tips on Listening:
Listen along your journey to help solidify understanding of topics.
Skip episodes 1, 3, 16, 21, and 26 (unless their topics interest and inspire you!).
Kaggle Machine Learning Track (Lesson 1) ↓
Need to Know: A simple code lab that covers the very basics of machine learning with scikit-learn and Panda through the application of the examples onto another set of data.
Loved:
A more active form of learning.
An engaging code lab that encourages participants to apply knowledge.
This track offers has a built-in Python notebook on Kaggle with all input files included. This removed any and all setup/installation issues.
Side note: It's a bit different than Jupyter notebook (e.g., have to click into a cell to add another cell).
Each lesson is short, which made the entire lesson go by very fast.
Disliked:
The writing in the first lesson didn't initially make it clear that one would need to apply the knowledge in the lesson to their workbook.
It wasn't a big deal, but when I started referencing files in the lesson, I had to dive into the files in my workbook to find they didn't exist, only to realize that the knowledge was supposed to be applied and not transcribed.
Lecturer:
Dan Becker:
Data Scientist at Kaggle
Undergrad in Computer Science, PhD in Econometrics
Supervised data science consultant for six Fortune 100 companies
Contributed to the Keras and Tensorflow libraries
Finished 2nd (out of 1353 teams) in $3 million Heritage Health Prize data mining competition
Speaks at deep learning workshops at events and conferences
Links:
https://www.kaggle.com/learn/machine-learning
Tips on Doing:
Read the exercises and apply to your dataset as you go.
Try lesson 2, which covers more complex/abstract topics (note: this second took a bit longer to work through).
Ready to commit
Fast.ai (part 1 of 2) ↓
Need to Know: Hands-down the most engaging and active form of learning ML. The source I would most recommend for anyone (although the training plan does help to build up to this course). This course is about learning through coding. This is the only course that I started to truly see the practical mechanics start to come together. It involves applying the most practical solutions to the most common problems (while also building an intuition for those solutions).
Loved:
Course Philosophy:
Active learning approach
"Go out into the world and understand underlying mechanics (of machine learning by doing)."
Counter-culture to the exclusivity of the machine learning field, focusing on inclusion.
"Let's do shit that matters to people as quickly as possible."
Highly pragmatic approach with tools that are currently being used (Jupyter Notebooks, scikit-learn, Keras, AWS, etc.).
Show an end-to-end process that you get to complete and play with in a development environment.
Math is involved, but is not prohibitive. Excel files helped to consolidate information/interact with information in a different way, and Jeremy spends a lot of time recapping confusing concepts.
Amazing set of learning resources that allow for all different styles of learning, including:
Video Lessons
Notes
Jupyter Notebooks
Assignments
Highly active forums
Resources on Stackoverflow
Readings/resources
Jeremy often references popular academic texts
Jeremy's TEDx talk in Brussels
Jeremy really pushes one to do extra and put in the effort by teaching interesting problems and engaging one in solving them.
It's a huge time commitment; however, it's worth it.
All of the course's profits are donated.
Disliked:
Overview covers their approach to learning (obviously I'm a fan!). If you're already drinking the Kool-aid, skip past.
I struggled through the AWS setup (13-minute video) for about five hours (however, it felt so good when it was up and running!).
Because of its practicality and concentration on solutions used today to solve popular problem types (image recognition, text generation, etc.), it lacks breadth of machine learning topics.
Lecturers:
Jeremy Howard:
Distinguished Research Scientist at the University of San Francisco
Faculty member at Singularity University
Young Global Leader with the World Economic Forum
Founder of Enlitic (the first company to apply deep learning to medicine)
Former President and Chief Scientist of the data science platform Kaggle
Rachel Thomas:
PhD in Math from Duke
One of Forbes' "20 Incredible Women Advancing AI Research"
Researcher-in-residence at the University of San Francisco Data Institute
Teaches in the Masters in Data Science program
Links:
http://course.fast.ai/start.html
http://wiki.fast.ai/index.php/Main_Page
https://github.com/fastai/courses/tree/master/deeplearning1/nbs
Tips on Doing:
Set expectations with yourself that installation is going to probably take a few hours.
Prepare to spend about ~70 hours for this course (it's worth it).
Don't forget to shut off your AWS instance.
Balance out machine learning knowledge with a course with more breadth.
Consider giving part two of the Fast.ai program a shot!
Hands-On Machine Learning with Scikit-Learn and TensorFlow: Concepts, Tools, and Techniques to Build Intelligent Systems ↓
Need to Know: This book is an Amazon best seller for a reason. It covers a lot of ground quickly, empowers readers to walk through a machine learning problem by chapter two, and contains practical up-to-date machine learning skills.
Loved:
Book contains an amazing introduction to machine learning that briskly provides an overarching quick view of the machine learning ecosystem.
Chapter 2 immediately walks the reader through an end-to-end machine learning problem.
Immediately afterwards, Aurélien pushes a user to attempt to apply this solution to another problem, which was very empowering.
There are review questions at the end of each chapter to ensure on has grasped the content within the chapter and to push the reader to explore more.
Once installation was completed, it was easy to follow and all code is available on GitHub.
Chapters 11-14 were very tough reading; however, they were a great reference when working through Fast.ai.
Contains some powerful analogies.
Each chapter's introductions were very useful and put everything into context. This general-to-specifics learning was very useful.
Disliked:
Installation was a common source of issues during the beginning of my journey; the text glided over this. I felt the frustration that most people experience from installation should have been addressed with more resources.
Writer:
Aurélien Géron:
Led the YouTube video classification team from 2013 to 2016
Currently a machine Learning consultant
Founder and CTO of Wifirst and Polyconseil
Published technical books (on C++, Wi-Fi, and Internet architectures)
Links:
https://www.amazon.com/_/dp/1491962291?tag=oreilly20-20
http://shop.oreilly.com/product/0636920052289.do
https://github.com/ageron/handson-ml
Tips on Using:
Get a friend with Python experience to help with installation.
Read the introductions to each chapter thoroughly, read the chapter (pay careful attention to code), review the questions at the end (highlight any in-text answer), make a copy of Aurélien's GitHub and make sure everything works on your setup, re-type the notebooks, go to Kaggle and try on other datasets.
Broadening your horizons
Udacity: Intro to Machine Learning (Kate/Sebastian) ↓
Need to Know: A course that covers a range of machine learning topics, supports building of intuition via visualization and simple examples, offers coding challenges, and a certificate (upon completion of a final project). The biggest challenge with this course is bridging the gap between the hand-holding lectures and the coding exercises.
Loved:
Focus on developing a visual intuition on what each model is trying to accomplish.
This visual learning mathematics approach is very useful.
Cover a vast variety and breadth of models and machine learning basics.
In terms of presenting the concept, there was a lot of hand-holding (which I completely appreciated!).
Many people have done this training, so their GitHub accounts can be used as reference for the mini-projects.
Katie actively notes documentation and suggests where viewers can learn more/reference material.
Disliked:
All of the conceptual hand-holding in the lessons is a stark contrast to the challenges of installation, coding exercises, and mini-projects.
This is the first course started and the limited instructions on setting up the environment and many failed attempts caused me to break down crying at least a handful of times.
The mini-projects are intimidating.
There is extra code added to support the viewers; however, it's done so with little acknowledgement as to what it's actually doing. This made learning a bit harder.
Lecturer:
Caitlin (Katie) Malone:
Director of Data Science Research and Development at Civis Analytics
Stanford PhD in Experimental Particle Physics
Intern at Udacity in summer 2014
Graduate Researcher at the SLAC National Accelerator Laboratory
https://www6.slac.stanford.edu/
Podcaster with Ben Jaffe (currently Facebook UI Engineer and a music aficionado) on a machine learning podcast Linear Digressions (100+ episodes)
Sebastian Thrun:
CEO of the Kitty Hawk Corporation
Chairman and co-founder of Udacity
One of my favorite Sebastian quotes: "It occurred to me, I could be at Google and build a self-driving car, or I can teach 10,000 students how to build self-driving cars."
Former Google VP
Founded Google X
Led development of the robotic vehicle Stanley
Professor of Computer Science at Stanford University
Formerly a professor at Carnegie Mellon University.
Links:
https://www.udacity.com/course/intro-to-machine-learning--ud120
Udacity also offers a next step, the Machine Learning Engineer Nanodegree, which will set one back about $1K.
Tips on Watching:
Get a friend to help you set up your environment.
Print mini-project instructions to check off each step.
Andrew Ng's Coursera Machine Learning Course ↓
Need to Know: The Andrew Ng Coursera course is the most referenced online machine learning course. It covers a broad set of fundamental, evergreen topics with a strong focus in building mathematical intuition behind machine learning models. Also, one can submit assignments and earn a grade for free. If you want to earn a certificate, one can subscribe or apply for financial aid.
Loved:
This course has a high level of credibility.
Introduces all necessary machine learning terminology and jargon.
Contains a very classic machine learning education approach with a high level of math focus.
Quizzes interspersed in courses and after each lesson support understanding and overall learning.
The sessions for the course are flexible, the option to switch into a different section is always available.
Disliked:
The mathematic notation was hard to process at times.
The content felt a bit dated and non-pragmatic. For example, the main concentration was MATLAB and Octave versus more modern languages and resources.
Video quality was less than average and could use a refresh.
Lecturer:
Andrew Ng:
Adjunct Professor, Stanford University (focusing on AI, Machine Learning, and Deep Learning)
Co-founder of Coursera
Former head of Baidu AI Group
Founder and previous head of Google Brain (deep learning) project
Former Director of the Stanford AI Lab
Chairman of the board of Woebot (a machine learning bot that focuses on Cognitive Behavior Therapy)
Links:
https://www.coursera.org/learn/machine-learning/
Andrew Ng recently launched a new course (August 2017) called DeepLearning.ai, a ~15 week course containing five mini-courses ($49 USD per month to continue learning after trial period of 7 days ends).
Course: https://www.coursera.org/specializations/deep-learning
Course 1: Neural Networks and Deep Learning
Course 2: Improving Deep Neural Networks: Hyperparameter tuning, Regularization and Optimization
Course 3: Structuring Machine Learning Projects
Course 4: Convolutional Neural Networks
Course 5: Sequence Models
Tips on Watching:
Be disciplined with setting aside timing (even if it's only 15 minutes a day) to help power through some of the more boring concepts.
Don't do this course first, because it's intimidating, requires a large time commitment, and isn't a very energizing experience.
Additional machine learning opportunities
iPullRank Machine Learning Guide ↓
Need to Know: A machine learning e-book targeted at marketers.
Loved:
Targeted at marketers and applied to organic search.
Covers a variety of machine learning topics.
Some good examples, including real-world blunders.
Gives some practical tools for non-data scientists (including: MonkeyLearn and Orange)
I found Orange to be a lot of fun. It struggled with larger datasets; however, it has a very visual interface that was more user-friendly and offers potential to show some pretty compelling stories.
Example: World Happiness Dataset by:
X-axis: Happiness Score
Y-axis: Economy
Color: Health
Disliked:
Potential to break up content more with relevant imagery -- the content was very dense.
Writers:
iPullRank Team (including Mike King):
Mike King has a few slide decks on the basics of machine learnings and AI
iPullRank has a few data scientists on staff
Links:
http://ipullrank.com/machine-learning-guide/
Tips on Reading:
Read chapters 1-6 and the rest depending upon personal interest.
Review Google PhD ↓
Need to Know: A two-hour presentation from Google's 2017 IO conference that walks through getting 99% accuracy on the MNIST dataset (a famous dataset containing a bunch of handwritten numbers, which the machine must learn to identify the numbers).
Loved:
This talk struck me as very modern, covering the cutting edge.
Found this to be very complementary to Fast.ai, as it covered similar topics (e.g. ReLu, CNNs, RNNs, etc.)
Amazing visuals that help to put everything into context.
Disliked:
The presentation is only a short conference solution and not a comprehensive view of machine learning.
Also, a passive form of learning.
Presenter:
Martin Görner:
Developer Relations, Google (since 2011)
Started Mobipocket, a startup that later became the software part of the Amazon Kindle and its mobile variants
Links:
Part 1 - https://www.youtube.com/watch?v=u4alGiomYP4
Part 2 - https://www.youtube.com/watch?v=fTUwdXUFfI8
Tips on Watching:
Google any concepts you're unfamiliar with.
Take your time with this one; 2 hours of screen time doesn't count all of the Googling and processing time for this one.
Caltech Machine Learning iTunes ↓
Need to Know: If math is your thing, this course does a stellar job of building the mathematic intuition behind many machine learning models. Dr. Abu-Mostafa is a raconteur, includes useful visualizations, relevant real-world examples, and compelling analogies.
Loved:
First and foremost, this is a real Caltech course, meaning it's not a watered-down version and contains fundamental concepts that are vital to understanding the mechanics of machine learning.
On iTunes, audio downloads are available, which can be useful for on-the-go learning.
Dr. Abu-Mostafa is a skilled speaker, making the 27 hours spent listening much easier!
Dr. Abu-Mostafa offers up some strong real-world examples and analogies which makes the content more relatable.
As an example, he asks students: "Why do I give you practice exams and not just give you the final exam?" as an illustration of why a testing set is useful. If he were to just give students the final, they would just memorize the answers (i.e., they would overfit to the data) and not genuinely learn the material. The final is a test to show how much students learn.
The last 1/2 hour of the class is always a Q&A, where students can ask questions. Their questions were useful to understanding the topic more in-depth.
The video and audio quality was strong throughout. There were a few times when I couldn't understand a question in the Q&A, but overall very strong.
This course is designed to build mathematical intuition of what's going on under the hood of specific machine learning models.
Caution: Dr. Abu-Mostafa uses mathematical notation, but it's different from Andrew Ng's (e.g., theta = w).
The final lecture was the most useful, as it pulled a lot of the conceptual puzzle pieces together. The course on neural networks was a close second!
Disliked:
Although it contains mostly evergreen content, being released in 2012, it could use a refresh.
Very passive form of learning, as it wasn't immediately actionable.
Lecturer:
Dr. Yaser S. Abu-Mostafa:
Professor of Electrical Engineering and Computer Science at the California Institute of Technology
Chairman of Machine Learning Consultants LLC
Serves on a number of scientific advisory boards
Has served as a technical consultant on machine learning for several companies (including Citibank).
Multiple articles in Scientific American
Links:
https://work.caltech.edu/telecourse.html
https://itunes.apple.com/us/course/machine-learning/id515364596
Tips on Watching:
Consider listening to the last lesson first, as it pulls together the course overall conceptually. The map of the course, below, was particularly useful to organizing the information taught in the courses.
Image source: http://work.caltech.edu/slides/slides18.pdf
"Pattern Recognition & Machine Learning" by Christopher Bishop ↓
Need to Know: This is a very popular college-level machine learning textbook. I've heard it likened to a bible for machine learning. However, after spending a month trying to tackle the first few chapters, I gave up. It was too much math and pre-requisites to tackle (even with a multitude of Google sessions).
Loved:
The text of choice for many major universities, so if you can make it through this text and understand all of the concepts, you're probably in a very good position.
I appreciated the history aside sections, where Bishop talked about influential people and their career accomplishments in statistics and machine learning.
Despite being a highly mathematically text, the textbook actually has some pretty visually intuitive imagery.
Disliked:
I couldn't make it through the text, which was a bit frustrating. The statistics and mathematical notation (which is probably very benign for a student in this topic) were too much for me.
The sunk cost was pretty high here (~$75).
Writer:
Christopher Bishop:
Laboratory Director at Microsoft Research Cambridge
Professor of Computer Science at the University of Edinburgh
Fellow of Darwin College, Cambridge
PhD in Theoretical Physics from the University of Edinburgh
Links:
https://www.amazon.com/Pattern-Recognition-Learning-Information-Statistics/dp/0387310738/ref=sr_1_2?ie=UTF8&qid=1516839475&sr=8-2&keywords=Pattern+Recognition+%26+Machine+Learning
Tips on Reading:
Don't start your machine learning journey with this book.
Get a friend in statistics to walk you through anything complicated (my plan is to get a mentor in statistics).
Consider taking a (free) online statistics course (Khan Academy and Udacity both have some great content on statistics, calculus, math, and data analysis).
Machine Learning: Hands-on for Developers and Technical Professionals ↓
Need to Know: A fun, non-intimidating end-to-end launching pad/whistle stop for machine learning in action.
Loved:
Talks about practical issues that many other sources didn't really address (e.g. data-cleansing).
Covered the basics of machine learning in a non-intimidating way.
Offers abridged, consolidated versions of the content.
Added fun anecdotes that makes it easier to read.
Overall the writer has a great sense of humor.
Writer talks to the reader as if they're a real human being (i.e., doesn't expect you to go out and do proofs; acknowledges the challenge of certain concepts).
Covers a wide variety of topics.
Because it was well-written, I flew through the book (even though it's about ~300 pages).
Disliked:
N/A
Writer:
Jason Bell:
Technical architect, lecturer, and startup consultant
Data Engineer at MastodonC
Former section editor for Java Developer's Journal
Former writer on IBM DeveloperWorks
Links:
https://www.amazon.com/Machine-Learning-Hands-Developers-Professionals/dp/1118889061
https://www.wiley.com/en-us/Machine+Learning%3A+Hands+On+for+Developers+and+Technical+Professionals-p-9781118889060
Jason's Blog: https://dataissexy.wordpress.com/
Tips on Reading:
Download and explore Weka's interface beforehand.
Give some of the exercises a shot.
Introduction to Machine Learning with Python: A Guide for Data Scientists ↓
Need to Know: This was a was a well-written piece on machine learning, making it a quick read.
Loved:
Quick, smooth read.
Easy-to-follow code examples.
The first few chapters served as a stellar introduction to the basics of machine learning.
Contain subtle jokes that add a bit of fun.
Tip to use the Python package manager Anaconda with Jupyter Notebooks was helpful.
Disliked:
Once again, installation was a challenge.
The "mglearn" utility library threw me for a loop. I had to reread the first few chapters before I figured out it was support for the book.
Although I liked the book, I didn't love it. Overall it just missed the "empowering" mark.
Writers:
Andreas C. Müller:
PhD in Computer Science
Lecturer at the Data Science Institute at Columbia University
Worked at the NYU Center for Data Science on open source and open science
Former Machine Learning Scientist at Amazon
Speaks often on Machine Learning and scikit-learn (a popular machine learning library)
And he makes some pretty incredibly useful graphics, such as this scikit-learn cheat sheet:
Image source: http://peekaboo-vision.blogspot.com/2013/01/machin...
Sarah Guido:
Former senior data scientist at Mashable
Lead data scientist at Bitly
2018 SciPy Conference Data Science track co-chair
Links:
https://www.amazon.com/Introduction-Machine-Learning-Python-Scientists/dp/1449369413/ref=sr_1_7?s=books&ie=UTF8&qid=1516734322&sr=1-7&keywords=python+machine+learning
http://shop.oreilly.com/product/0636920030515.do
Tips on Reading:
Type out code examples.
Beware of the "mglearn" utility library.
Udacity: Machine Learning by Georgia Tech ↓
Need to Know: A mix between an online learning experience and a university machine learning teaching approach. The lecturers are fun, but the course still fell a bit short in terms of active learning.
Loved:
This class is offered as CS7641 at Georgia Tech, where it is a part of the Online Masters Degree. Although taking this course here will not earn credit towards the OMS degree, it's still a non-watered-down college teaching philosophy approach.
Covers a wide variety of topics, many of which reminded me of the Caltech course (including: VC Dimension versus Bayesian, Occam's razor, etc.)
Discusses Markov Decision Chains, which is something that didn't really come up in many other introductory machine learning course, but they are referenced within Google patents.
The lecturers have a great dynamic, are wicked smart, and displayed a great sense of (nerd) humor, which make the topics less intimidating.
The course has quizzes, which give the course a slight amount of interaction.
Disliked:
Some videos were very long, which made the content a bit harder to digest.
The course overall was very time consuming.
Despite the quizzes, the course was a very passive form of learning with no assignments and little coding.
Many videos started with a bunch of content already written out. Having the content written out was probably a big time-saver, but it was also a bit jarring for a viewer to see so much information all at once, while also trying to listen.
It's vital to pay very close attention to notation, which compounds in complexity quickly.
Tablet version didn't function flawlessly: some was missing content (which I had to mark down and review on a desktop), the app would crash randomly on the tablet, and sometimes the audio wouldn't start.
There were no subtitles available on tablet, which I found not only to be a major accessibility blunder, but also made it harder for me to process (since I'm not an audio learner).
Lecturer:
Michael Littman:
Professor of Computer Science at Brown University.
Was granted a patent for one of the earliest systems for Cross-language information retrieval
Perhaps the most interesting man in the world:
Been in two TEDx talks
How I Learned to Stop Worrying and Be Realistic About AI
A Cooperative Path to Artificial Intelligence
During his time at Duke, he worked on an automated crossword solver (PROVERB)
Has a Family Quartet
He has appeared in a TurboTax commercial
Charles Isbell:
Professor and Executive Associate Dean at School of Interactive Computing at Georgia Tech
Focus on statistical machine learning and "interactive" artificial intelligence.
Links:
https://www.udacity.com/course/machine-learning--ud262
Tips on Watching:
Pick specific topics of interest and focusing on those lessons.
Andrew Ng's Stanford's Machine Learning iTunes ↓
Need to Know: A non-watered-down Stanford course. It's outdated (filmed in 2008), video/audio are a bit poor, and most links online now point towards the Coursera course. Although the idea of watching a Stanford course was energizing for the first few courses, it became dreadfully boring. I made it to course six before calling it.
Loved:
Designed for students, so you know you're not missing out on anything.
This course provides a deeper study into the mathematical and theoretical foundation behind machine learning to the point that the students could create their own machine learning algorithms. This isn't necessarily very practical for the everyday machine learning user.
Has some powerful real-world examples (although they're outdated).
There is something about the kinesthetic nature of watching someone write information out. The blackboard writing helped me to process certain ideas.
Disliked:
Video and audio quality were pain to watch.
Many questions asked by students were hard to hear.
On-screen visuals range from hard to impossible to see.
Found myself counting minutes.
Dr. Ng mentions TA classes, supplementary learning, but these are not available online.
Sometimes the video showed students, which I felt was invasive.
Lecturer:
Andrew Ng (see above)
Links:
https://itunes.apple.com/us/course/machine-learning/id495053006
https://www.youtube.com/watch?v=UzxYlbK2c7E
Tips on Watching:
Only watch if you're looking to gain a deeper understanding of the math presented in the Coursera course.
Skip the first half of the first lecture, since it's mostly class logistics.
Additional Resources
Fast.ai (part 2) - free access to materials, cost for AWS EC2 instance
Deeplearning.ai - $50/month
Udacity Machine Learning Engineer Nanodegree - $1K
https://machinelearningmastery.com/
Motivations and inspiration
If you're wondering why I spent a year doing this, then I'm with you. I'm genuinely not sure why I set my sights on this project, much less why I followed through with it. I saw Mike King give a session on Machine Learning. I was caught off guard, since I knew nothing on the topic. It gave me a pesky, insatiable curiosity itch. It started with one course and then spiraled out of control. Eventually it transformed into an idea: a review guide on the most affordable and popular machine learning resources on the web (through the lens of a complete beginner). Hopefully you found it useful, or at least somewhat interesting. Be sure to share your thoughts or questions in the comments!
Sign up for The Moz Top 10, a semimonthly mailer updating you on the top ten hottest pieces of SEO news, tips, and rad links uncovered by the Moz team. Think of it as your exclusive digest of stuff you don't have time to hunt down but want to read!
0 notes
Text
A Machine Learning Guide for Average Humans
Posted by alexis-sanders
//<![CDATA[ (function($) { // code using $ as alias to jQuery $(function() { // Hide the hypotext content. $('.hypotext-content').hide(); // When a hypotext link is clicked. $('a.hypotext.closed').click(function (e) { // custom handling here e.preventDefault(); // Create the class reference from the rel value. var id = '.' + $(this).attr('rel'); // If the content is hidden, show it now. if ( $(id).css('display') == 'none' ) { $(id).show('slow'); if (jQuery.ui) { // UI loaded $(id).effect("highlight", {}, 1000); } } // If the content is shown, hide it now. else { $(id).hide('slow'); } }); // If we have a hash value in the url. if (window.location.hash) { // If the anchor is within a hypotext block, expand it, by clicking the // relevant link. console.log(window.location.hash); var anchor = $(window.location.hash); var hypotextLink = $('#' + anchor.parents('.hypotext-content').attr('rel')); console.log(hypotextLink); hypotextLink.click(); // Wait until the content has expanded before jumping to anchor. //$.delay(1000); setTimeout(function(){ scrollToAnchor(window.location.hash); }, 1000); } }); function scrollToAnchor(id) { var anchor = $(id); $('html,body').animate({scrollTop: anchor.offset().top},'slow'); } })(jQuery); //]]>
Machine learning (ML) has grown consistently in worldwide prevalence. Its implications have stretched from small, seemingly inconsequential victories to groundbreaking discoveries. The SEO community is no exception. An understanding and intuition of machine learning can support our understanding of the challenges and solutions Google's engineers are facing, while also opening our minds to ML's broader implications.
The advantages of gaining an general understanding of machine learning include:
Gaining empathy for engineers, who are ultimately trying to establish the best results for users
Understanding what problems machines are solving for, their current capabilities and scientists' goals
Understanding the competitive ecosystem and how companies are using machine learning to drive results
Preparing oneself for for what many industry leaders call a major shift in our society (Andrew Ng refers to AI as a "new electricity")
Understanding basic concepts that often appear within research (it's helped me with understanding certain concepts that appear within Google Brain's Research)
Growing as an individual and expanding your horizons (you might really enjoy machine learning!)
When code works and data is produced, it's a very fulfilling, empowering feeling (even if it's a very humble result)
I spent a year taking online courses, reading books, and learning about learning (...as a machine). This post is the fruit borne of that labor -- it covers 17 machine learning resources (including online courses, books, guides, conference presentations, etc.) comprising the most affordable and popular machine learning resources on the web (through the lens of a complete beginner). I've also added a summary of "If I were to start over again, how I would approach it."
This article isn't about credit or degrees. It's about regular Joes and Joannas with an interest in machine learning, and who want to spend their learning time efficiently. Most of these resources will consume over 50 hours of commitment. Ain't nobody got time for a painful waste of a work week (especially when this is probably completed during your personal time). The goal here is for you to find the resource that best suits your learning style. I genuinely hope you find this research useful, and I encourage comments on which materials prove most helpful (especially ones not included)! #HumanLearningMachineLearning
Executive summary:
Here's everything you need to know in a chart:
Machine Learning Resource
Time (hours)
Cost ($)
Year
Credibility
Code
Math
Enjoyability
Jason Maye's Machine Learning 101 slidedeck: 2 years of headbanging, so you don't have to
2
$0
'17
{ML} Recipes with Josh Gordon Playlist
2
$0
'16
Machine Learning Crash Course
15
$0
'18
OCDevel Machine Learning Guide Podcast
30
$0
'17-
Kaggle's Machine Learning Track (part 1)
6
$0
'17
Fast.ai (part 1)
70
$70*
'16
Hands-On Machine Learning with Scikit-Learn and TensorFlow: Concepts, Tools, and Techniques to Build Intelligent Systems
20
$25
'17
Udacity's Intro to Machine Learning (Kate/Sebastian)
60
$0
'15
Andrew Ng's Coursera Machine Learning
55
$0
'11
iPullRank Machine Learning Guide
3
$0
'17
Review Google PhD
2
$0
'17
Caltech Machine Learning on iTunes
27
$0
'12
Pattern Recognition & Machine Learning by Christopher Bishop
150
$75
'06
N/A
Machine Learning: Hands-on for Developers and Technical Professionals
15
$50
'15
Introduction to Machine Learning with Python: A Guide for Data Scientists
15
$25
'16
Udacity's Machine Learning by Georgia Tech
96
$0
'15
Machine Learning Stanford iTunes by Andrew Ng
25
$0
'08
N/A
*Free, but there is the cost of running an AWS EC2 instance (~$70 when I finished, but I did tinker a ton and made a Rick and Morty script generator, which I ran many epochs [rounds] of...)
Here's my suggested program:
1. Starting out (estimated 60 hours)
Start with shorter content targeting beginners. This will allow you to get the gist of what's going on with minimal time commitment.
Commit three hours to Jason Maye's Machine Learning 101 slidedeck: 2 years of headbanging, so you don't have to.
Commit two hours to watch Google's {ML} Recipes with Josh Gordon YouTube Playlist.
Sign up for Sam DeBrule's Machine Learnings newsletter.
Work through Google's Machine Learning Crash Course.
Start listening to OCDevel's Machine Learning Guide Podcast (skip episodes 1, 3, 16, 21, and 26) in your car, working out, and/or when using hands and eyes for other activities.
Commit two days to working through Kaggle's Machine Learning Track part 1.
2. Ready to commit (estimated 80 hours)
By this point, learners would understand their interest levels. Continue with content focused on applying relevant knowledge as fast as possible.
Commit to Fast.ai 10 hours per week, for 7 weeks. If you have a friend/mentor that can help you work through AWS setup, definitely lean on any support in installation (it's 100% the worst part of ML).
Acquire Hands-On Machine Learning with Scikit-Learn and TensorFlow: Concepts, Tools, and Techniques to Build Intelligent Systems, and read the first two chapters immediately. Then use this as supplemental to the Fast.ai course.
3. Broadening your horizons (estimated 115 hours)
If you've made it through the last section and are still hungry for more knowledge, move on to broadening your horizons. Read content focused on teaching the breadth of machine learning -- building an intuition for what the algorithms are trying to accomplish (whether visual or mathematically).
Start watching videos and participating in Udacity's Intro to Machine Learning (by Sebastian Thrun and Katie Malone).
Work through Andrew Ng's Coursera Machine Learning course.
Your next steps
By this point, you will already have AWS running instances, a mathematical foundation, and an overarching view of machine learning. This is your jumping-off point to determine what you want to do.
You should be able to determine your next step based on your interest, whether it's entering Kaggle competitions; doing Fast.ai part two; diving deep into the mathematics with Pattern Recognition & Machine Learning by Christopher Bishop; giving Andrew Ng's newer Deeplearning.ai course on Coursera; learning more about specific tech stacks (TensorFlow, Scikit-Learn, Keras, Pandas, Numpy, etc.); or applying machine learning to your own problems.
Why am I recommending these steps and resources?
I am not qualified to write an article on machine learning. I don't have a PhD. I took one statistics class in college, which marked the first moment I truly understood "fight or flight" reactions. And to top it off, my coding skills are lackluster (at their best, they're chunks of reverse-engineered code from Stack Overflow). Despite my many shortcomings, this piece had to be written by someone like me, an average person.
Statistically speaking, most of us are average (ah, the bell curve/Gaussian distribution always catches up to us). Since I'm not tied to any elitist sentiments, I can be real with you. Below contains a high-level summary of my reviews on all of the classes I took, along with a plan for how I would approach learning machine learning if I could start over. Click to expand each course for the full version with notes.
In-depth reviews of machine learning courses:
Starting out
Jason Maye's Machine Learning 101 slidedeck: 2 years of head-banging, so you don't have to ↓
Need to Know: A stellar high-level overview of machine learning fundamentals in an engaging and visually stimulating format.
Loved:
Very user-friendly, engaging, and playful slidedeck.
Has the potential to take some of the pain out of the process, through introducing core concepts.
Breaks up content by beginner/need-to-know (green), and intermediate/less-useful noise (specifically for individuals starting out) (blue).
Provides resources to dive deeper into machine learning.
Provides some top people to follow in machine learning.
Disliked:
That there is not more! Jason's creativity, visual-based teaching approach, and quirky sense of humor all support the absorption of the material.
Lecturer:
Jason Mayes:
Senior Creative Technologist and Research Engineer at Google
Masters in Computer Science from University of Bristols
Personal Note: He's also kind on Twitter! :)
Links:
Machine Learning 101 slide deck
Tips on Watching:
Set aside 2-4 hours to work through the deck once.
Since there is a wealth of knowledge, refer back as needed (or as a grounding source).
Identify areas of interest and explore the resources provided.
{ML} Recipes with Josh Gordon ↓
Need to Know: This mini-series YouTube-hosted playlist covers the very fundamentals of machine learning with opportunities to complete exercises.
Loved:
It is genuinely beginner-focused.
They make no assumption of any prior knowledge.
Gloss over potentially complex topics that may serve as noise.
Playlist ~2 hours
Very high-quality filming, audio, and presentation, almost to the point where it had its own aesthetic.
Covers some examples in scikit-learn and TensorFlow, which felt modern and practical.
Josh Gordon was an engaging speaker.
Disliked:
I could not get Dockers on Windows (suggested package manager). This wasn't a huge deal, since I already had my AWS setup by this point; however, a bit of a bummer since it made it impossible to follow certain steps exactly.
Issue: Every time I tried to download (over the course of two weeks), the .exe file would recursively start and keep spinning until either my memory ran out, computer crashed, or I shut my computer down. I sent this to Docker's Twitter account to no avail.
Lecturer:
Josh Gordon:
Developer Advocate for at TensorFlow at Google
Leads Machine Learning advocacy at Google
Member of the Udacity AI & Data Industry Advisory Board
Masters in Computer Science from Columbia University
Links:
Hello World - Machine Learning Recipes #1 (YouTube)
GitHub: Machine Learning Recipes with Josh Gordon
Tips on Watching:
The playlist is short (only ~1.5 hours screen time). However, it can be a bit fast-paced at times (especially if you like mimicking the examples), so set aside 3-4 hours to play around with examples and allow time for installation, pausing, and following along.
Take time to explore code labs.
Google's Machine Learning Crash Course with TensorFlow APIs ↓
Need to Know: A Google researcher-made crash course on machine learning that is interactive and offers its own built-in coding system!
Loved:
Different formats of learning: high-quality video (with ability to adjust speed, closed captioning), readings, quizzes (with explanations), visuals (including whiteboarding), interactive components/ playgrounds, code lab exercises (run directly in your browser (no setup required!))
Non-intimidating
One of my favorite quotes: "You don't need to understand the math to be able to take a look at the graphical interpretation."
Broken down into digestible sections
Introduces key terms
Disliked:
N/A
Lecturers:
Multiple Google researchers participated in this course, including:
Peter Norvig
Director of Research at Google Inc.
Previously he directed Google's core search algorithms group.
He is co-author of Artificial Intelligence: A Modern Approach
D. Sculley
Senior Staff Software Engineer at Google
KDD award-winning papers
Works on massive-scale ML systems for online advertising
Was part of a research ML paper on optimizing chocolate chip cookies
According to his personal website, he prefers to go by "D."
Cassandra Xia
Programmer, Software Engineer at Google
She has some really cool (and cute) projects based on learning statistics concepts interactively
Maya Gupta
Leads Glassbox Machine Learning R&D team at Google
Associate Professor of Electrical Engineering at the University of Washington (2003-2012)
In 2007, Gupta received the PECASE award from President George Bush for her work in classifying uncertain (e.g. random) signals
Gupta also runs Artifact Puzzles, the second-largest US maker of wooden jigsaw puzzles
Sally Goldman
Research Scientist at Google
Co-author of A Practical Guide to Data Structures and Algorithms Using Java
Numerous journals, classes taught at Washington University, and contributions to the ML community
Links:
Machine Learning Crash Course
Tips on Doing:
Actively work through playground and coding exercises
OCDevel's Machine Learning Guide Podcast ↓
Need to Know: This podcast focuses on the high-level fundamentals of machine learning, including basic intuition, algorithms, math, languages, and frameworks. It also includes references to learn more on each episode's topic.
Loved:
Great for trips (when traveling a ton, it was an easy listen).
The podcast makes machine learning fun with interesting and compelling analogies.
Tyler is a big fan of Andrew Ng's Coursera course and reviews concepts in Coursera course very well, such that both pair together nicely.
Covers the canonical resources for learning more on a particular topic.
Disliked:
Certain courses were more theory-based; all are interesting, yet impractical.
Due to limited funding the project is a bit slow to update and has less than 30 episodes.
Podcaster:
Tyler Renelle:
Machine learning engineer focused on time series and reinforcement
Background in full-stack JavaScript, 10 years web and mobile
Creator of HabitRPG, an app that treats habits as an RPG game
Links:
Machine Learning Guide podcast
Machine Learning Guide podcast (iTunes)
Tips on Listening:
Listen along your journey to help solidify understanding of topics.
Skip episodes 1, 3, 16, 21, and 26 (unless their topics interest and inspire you!).
Kaggle Machine Learning Track (Lesson 1) ↓
Need to Know: A simple code lab that covers the very basics of machine learning with scikit-learn and Panda through the application of the examples onto another set of data.
Loved:
A more active form of learning.
An engaging code lab that encourages participants to apply knowledge.
This track offers has a built-in Python notebook on Kaggle with all input files included. This removed any and all setup/installation issues.
Side note: It's a bit different than Jupyter notebook (e.g., have to click into a cell to add another cell).
Each lesson is short, which made the entire lesson go by very fast.
Disliked:
The writing in the first lesson didn't initially make it clear that one would need to apply the knowledge in the lesson to their workbook.
It wasn't a big deal, but when I started referencing files in the lesson, I had to dive into the files in my workbook to find they didn't exist, only to realize that the knowledge was supposed to be applied and not transcribed.
Lecturer:
Dan Becker:
Data Scientist at Kaggle
Undergrad in Computer Science, PhD in Econometrics
Supervised data science consultant for six Fortune 100 companies
Contributed to the Keras and Tensorflow libraries
Finished 2nd (out of 1353 teams) in $3 million Heritage Health Prize data mining competition
Speaks at deep learning workshops at events and conferences
Links:
https://www.kaggle.com/learn/machine-learning
Tips on Doing:
Read the exercises and apply to your dataset as you go.
Try lesson 2, which covers more complex/abstract topics (note: this second took a bit longer to work through).
Ready to commit
Fast.ai (part 1 of 2) ↓
Need to Know: Hands-down the most engaging and active form of learning ML. The source I would most recommend for anyone (although the training plan does help to build up to this course). This course is about learning through coding. This is the only course that I started to truly see the practical mechanics start to come together. It involves applying the most practical solutions to the most common problems (while also building an intuition for those solutions).
Loved:
Course Philosophy:
Active learning approach
"Go out into the world and understand underlying mechanics (of machine learning by doing)."
Counter-culture to the exclusivity of the machine learning field, focusing on inclusion.
"Let's do shit that matters to people as quickly as possible."
Highly pragmatic approach with tools that are currently being used (Jupyter Notebooks, scikit-learn, Keras, AWS, etc.).
Show an end-to-end process that you get to complete and play with in a development environment.
Math is involved, but is not prohibitive. Excel files helped to consolidate information/interact with information in a different way, and Jeremy spends a lot of time recapping confusing concepts.
Amazing set of learning resources that allow for all different styles of learning, including:
Video Lessons
Notes
Jupyter Notebooks
Assignments
Highly active forums
Resources on Stackoverflow
Readings/resources
Jeremy often references popular academic texts
Jeremy's TEDx talk in Brussels
Jeremy really pushes one to do extra and put in the effort by teaching interesting problems and engaging one in solving them.
It's a huge time commitment; however, it's worth it.
All of the course's profits are donated.
Disliked:
Overview covers their approach to learning (obviously I'm a fan!). If you're already drinking the Kool-aid, skip past.
I struggled through the AWS setup (13-minute video) for about five hours (however, it felt so good when it was up and running!).
Because of its practicality and concentration on solutions used today to solve popular problem types (image recognition, text generation, etc.), it lacks breadth of machine learning topics.
Lecturers:
Jeremy Howard:
Distinguished Research Scientist at the University of San Francisco
Faculty member at Singularity University
Young Global Leader with the World Economic Forum
Founder of Enlitic (the first company to apply deep learning to medicine)
Former President and Chief Scientist of the data science platform Kaggle
Rachel Thomas:
PhD in Math from Duke
One of Forbes' "20 Incredible Women Advancing AI Research"
Researcher-in-residence at the University of San Francisco Data Institute
Teaches in the Masters in Data Science program
Links:
http://course.fast.ai/start.html
http://wiki.fast.ai/index.php/Main_Page
https://github.com/fastai/courses/tree/master/deeplearning1/nbs
Tips on Doing:
Set expectations with yourself that installation is going to probably take a few hours.
Prepare to spend about ~70 hours for this course (it's worth it).
Don't forget to shut off your AWS instance.
Balance out machine learning knowledge with a course with more breadth.
Consider giving part two of the Fast.ai program a shot!
Hands-On Machine Learning with Scikit-Learn and TensorFlow: Concepts, Tools, and Techniques to Build Intelligent Systems ↓
Need to Know: This book is an Amazon best seller for a reason. It covers a lot of ground quickly, empowers readers to walk through a machine learning problem by chapter two, and contains practical up-to-date machine learning skills.
Loved:
Book contains an amazing introduction to machine learning that briskly provides an overarching quick view of the machine learning ecosystem.
Chapter 2 immediately walks the reader through an end-to-end machine learning problem.
Immediately afterwards, Aurélien pushes a user to attempt to apply this solution to another problem, which was very empowering.
There are review questions at the end of each chapter to ensure on has grasped the content within the chapter and to push the reader to explore more.
Once installation was completed, it was easy to follow and all code is available on GitHub.
Chapters 11-14 were very tough reading; however, they were a great reference when working through Fast.ai.
Contains some powerful analogies.
Each chapter's introductions were very useful and put everything into context. This general-to-specifics learning was very useful.
Disliked:
Installation was a common source of issues during the beginning of my journey; the text glided over this. I felt the frustration that most people experience from installation should have been addressed with more resources.
Writer:
Aurélien Géron:
Led the YouTube video classification team from 2013 to 2016
Currently a machine Learning consultant
Founder and CTO of Wifirst and Polyconseil
Published technical books (on C++, Wi-Fi, and Internet architectures)
Links:
https://www.amazon.com/_/dp/1491962291?tag=oreilly20-20
http://shop.oreilly.com/product/0636920052289.do
https://github.com/ageron/handson-ml
Tips on Using:
Get a friend with Python experience to help with installation.
Read the introductions to each chapter thoroughly, read the chapter (pay careful attention to code), review the questions at the end (highlight any in-text answer), make a copy of Aurélien's GitHub and make sure everything works on your setup, re-type the notebooks, go to Kaggle and try on other datasets.
Broadening your horizons
Udacity: Intro to Machine Learning (Kate/Sebastian) ↓
Need to Know: A course that covers a range of machine learning topics, supports building of intuition via visualization and simple examples, offers coding challenges, and a certificate (upon completion of a final project). The biggest challenge with this course is bridging the gap between the hand-holding lectures and the coding exercises.
Loved:
Focus on developing a visual intuition on what each model is trying to accomplish.
This visual learning mathematics approach is very useful.
Cover a vast variety and breadth of models and machine learning basics.
In terms of presenting the concept, there was a lot of hand-holding (which I completely appreciated!).
Many people have done this training, so their GitHub accounts can be used as reference for the mini-projects.
Katie actively notes documentation and suggests where viewers can learn more/reference material.
Disliked:
All of the conceptual hand-holding in the lessons is a stark contrast to the challenges of installation, coding exercises, and mini-projects.
This is the first course started and the limited instructions on setting up the environment and many failed attempts caused me to break down crying at least a handful of times.
The mini-projects are intimidating.
There is extra code added to support the viewers; however, it's done so with little acknowledgement as to what it's actually doing. This made learning a bit harder.
Lecturer:
Caitlin (Katie) Malone:
Director of Data Science Research and Development at Civis Analytics
Stanford PhD in Experimental Particle Physics
Intern at Udacity in summer 2014
Graduate Researcher at the SLAC National Accelerator Laboratory
https://www6.slac.stanford.edu/
Podcaster with Ben Jaffe (currently Facebook UI Engineer and a music aficionado) on a machine learning podcast Linear Digressions (100+ episodes)
Sebastian Thrun:
CEO of the Kitty Hawk Corporation
Chairman and co-founder of Udacity
One of my favorite Sebastian quotes: "It occurred to me, I could be at Google and build a self-driving car, or I can teach 10,000 students how to build self-driving cars."
Former Google VP
Founded Google X
Led development of the robotic vehicle Stanley
Professor of Computer Science at Stanford University
Formerly a professor at Carnegie Mellon University.
Links:
https://www.udacity.com/course/intro-to-machine-learning--ud120
Udacity also offers a next step, the Machine Learning Engineer Nanodegree, which will set one back about $1K.
Tips on Watching:
Get a friend to help you set up your environment.
Print mini-project instructions to check off each step.
Andrew Ng's Coursera Machine Learning Course ↓
Need to Know: The Andrew Ng Coursera course is the most referenced online machine learning course. It covers a broad set of fundamental, evergreen topics with a strong focus in building mathematical intuition behind machine learning models. Also, one can submit assignments and earn a grade for free. If you want to earn a certificate, one can subscribe or apply for financial aid.
Loved:
This course has a high level of credibility.
Introduces all necessary machine learning terminology and jargon.
Contains a very classic machine learning education approach with a high level of math focus.
Quizzes interspersed in courses and after each lesson support understanding and overall learning.
The sessions for the course are flexible, the option to switch into a different section is always available.
Disliked:
The mathematic notation was hard to process at times.
The content felt a bit dated and non-pragmatic. For example, the main concentration was MATLAB and Octave versus more modern languages and resources.
Video quality was less than average and could use a refresh.
Lecturer:
Andrew Ng:
Adjunct Professor, Stanford University (focusing on AI, Machine Learning, and Deep Learning)
Co-founder of Coursera
Former head of Baidu AI Group
Founder and previous head of Google Brain (deep learning) project
Former Director of the Stanford AI Lab
Chairman of the board of Woebot (a machine learning bot that focuses on Cognitive Behavior Therapy)
Links:
https://www.coursera.org/learn/machine-learning/
Andrew Ng recently launched a new course (August 2017) called DeepLearning.ai, a ~15 week course containing five mini-courses ($49 USD per month to continue learning after trial period of 7 days ends).
Course: https://www.coursera.org/specializations/deep-learning
Course 1: Neural Networks and Deep Learning
Course 2: Improving Deep Neural Networks: Hyperparameter tuning, Regularization and Optimization
Course 3: Structuring Machine Learning Projects
Course 4: Convolutional Neural Networks
Course 5: Sequence Models
Tips on Watching:
Be disciplined with setting aside timing (even if it's only 15 minutes a day) to help power through some of the more boring concepts.
Don't do this course first, because it's intimidating, requires a large time commitment, and isn't a very energizing experience.
Additional machine learning opportunities
iPullRank Machine Learning Guide ↓
Need to Know: A machine learning e-book targeted at marketers.
Loved:
Targeted at marketers and applied to organic search.
Covers a variety of machine learning topics.
Some good examples, including real-world blunders.
Gives some practical tools for non-data scientists (including: MonkeyLearn and Orange)
I found Orange to be a lot of fun. It struggled with larger datasets; however, it has a very visual interface that was more user-friendly and offers potential to show some pretty compelling stories.
Example: World Happiness Dataset by:
X-axis: Happiness Score
Y-axis: Economy
Color: Health
Disliked:
Potential to break up content more with relevant imagery -- the content was very dense.
Writers:
iPullRank Team (including Mike King):
Mike King has a few slide decks on the basics of machine learnings and AI
iPullRank has a few data scientists on staff
Links:
http://ipullrank.com/machine-learning-guide/
Tips on Reading:
Read chapters 1-6 and the rest depending upon personal interest.
Review Google PhD ↓
Need to Know: A two-hour presentation from Google's 2017 IO conference that walks through getting 99% accuracy on the MNIST dataset (a famous dataset containing a bunch of handwritten numbers, which the machine must learn to identify the numbers).
Loved:
This talk struck me as very modern, covering the cutting edge.
Found this to be very complementary to Fast.ai, as it covered similar topics (e.g. ReLu, CNNs, RNNs, etc.)
Amazing visuals that help to put everything into context.
Disliked:
The presentation is only a short conference solution and not a comprehensive view of machine learning.
Also, a passive form of learning.
Presenter:
Martin Görner:
Developer Relations, Google (since 2011)
Started Mobipocket, a startup that later became the software part of the Amazon Kindle and its mobile variants
Links:
Part 1 - https://www.youtube.com/watch?v=u4alGiomYP4
Part 2 - https://www.youtube.com/watch?v=fTUwdXUFfI8
Tips on Watching:
Google any concepts you're unfamiliar with.
Take your time with this one; 2 hours of screen time doesn't count all of the Googling and processing time for this one.
Caltech Machine Learning iTunes ↓
Need to Know: If math is your thing, this course does a stellar job of building the mathematic intuition behind many machine learning models. Dr. Abu-Mostafa is a raconteur, includes useful visualizations, relevant real-world examples, and compelling analogies.
Loved:
First and foremost, this is a real Caltech course, meaning it's not a watered-down version and contains fundamental concepts that are vital to understanding the mechanics of machine learning.
On iTunes, audio downloads are available, which can be useful for on-the-go learning.
Dr. Abu-Mostafa is a skilled speaker, making the 27 hours spent listening much easier!
Dr. Abu-Mostafa offers up some strong real-world examples and analogies which makes the content more relatable.
As an example, he asks students: "Why do I give you practice exams and not just give you the final exam?" as an illustration of why a testing set is useful. If he were to just give students the final, they would just memorize the answers (i.e., they would overfit to the data) and not genuinely learn the material. The final is a test to show how much students learn.
The last 1/2 hour of the class is always a Q&A, where students can ask questions. Their questions were useful to understanding the topic more in-depth.
The video and audio quality was strong throughout. There were a few times when I couldn't understand a question in the Q&A, but overall very strong.
This course is designed to build mathematical intuition of what's going on under the hood of specific machine learning models.
Caution: Dr. Abu-Mostafa uses mathematical notation, but it's different from Andrew Ng's (e.g., theta = w).
The final lecture was the most useful, as it pulled a lot of the conceptual puzzle pieces together. The course on neural networks was a close second!
Disliked:
Although it contains mostly evergreen content, being released in 2012, it could use a refresh.
Very passive form of learning, as it wasn't immediately actionable.
Lecturer:
Dr. Yaser S. Abu-Mostafa:
Professor of Electrical Engineering and Computer Science at the California Institute of Technology
Chairman of Machine Learning Consultants LLC
Serves on a number of scientific advisory boards
Has served as a technical consultant on machine learning for several companies (including Citibank).
Multiple articles in Scientific American
Links:
https://work.caltech.edu/telecourse.html
https://itunes.apple.com/us/course/machine-learning/id515364596
Tips on Watching:
Consider listening to the last lesson first, as it pulls together the course overall conceptually. The map of the course, below, was particularly useful to organizing the information taught in the courses.
Image source: http://work.caltech.edu/slides/slides18.pdf
"Pattern Recognition & Machine Learning" by Christopher Bishop ↓
Need to Know: This is a very popular college-level machine learning textbook. I've heard it likened to a bible for machine learning. However, after spending a month trying to tackle the first few chapters, I gave up. It was too much math and pre-requisites to tackle (even with a multitude of Google sessions).
Loved:
The text of choice for many major universities, so if you can make it through this text and understand all of the concepts, you're probably in a very good position.
I appreciated the history aside sections, where Bishop talked about influential people and their career accomplishments in statistics and machine learning.
Despite being a highly mathematically text, the textbook actually has some pretty visually intuitive imagery.
Disliked:
I couldn't make it through the text, which was a bit frustrating. The statistics and mathematical notation (which is probably very benign for a student in this topic) were too much for me.
The sunk cost was pretty high here (~$75).
Writer:
Christopher Bishop:
Laboratory Director at Microsoft Research Cambridge
Professor of Computer Science at the University of Edinburgh
Fellow of Darwin College, Cambridge
PhD in Theoretical Physics from the University of Edinburgh
Links:
https://www.amazon.com/Pattern-Recognition-Learning-Information-Statistics/dp/0387310738/ref=sr_1_2?ie=UTF8&qid=1516839475&sr=8-2&keywords=Pattern+Recognition+%26+Machine+Learning
Tips on Reading:
Don't start your machine learning journey with this book.
Get a friend in statistics to walk you through anything complicated (my plan is to get a mentor in statistics).
Consider taking a (free) online statistics course (Khan Academy and Udacity both have some great content on statistics, calculus, math, and data analysis).
Machine Learning: Hands-on for Developers and Technical Professionals ↓
Need to Know: A fun, non-intimidating end-to-end launching pad/whistle stop for machine learning in action.
Loved:
Talks about practical issues that many other sources didn't really address (e.g. data-cleansing).
Covered the basics of machine learning in a non-intimidating way.
Offers abridged, consolidated versions of the content.
Added fun anecdotes that makes it easier to read.
Overall the writer has a great sense of humor.
Writer talks to the reader as if they're a real human being (i.e., doesn't expect you to go out and do proofs; acknowledges the challenge of certain concepts).
Covers a wide variety of topics.
Because it was well-written, I flew through the book (even though it's about ~300 pages).
Disliked:
N/A
Writer:
Jason Bell:
Technical architect, lecturer, and startup consultant
Data Engineer at MastodonC
Former section editor for Java Developer's Journal
Former writer on IBM DeveloperWorks
Links:
https://www.amazon.com/Machine-Learning-Hands-Developers-Professionals/dp/1118889061
https://www.wiley.com/en-us/Machine+Learning%3A+Hands+On+for+Developers+and+Technical+Professionals-p-9781118889060
Jason's Blog: https://dataissexy.wordpress.com/
Tips on Reading:
Download and explore Weka's interface beforehand.
Give some of the exercises a shot.
Introduction to Machine Learning with Python: A Guide for Data Scientists ↓
Need to Know: This was a was a well-written piece on machine learning, making it a quick read.
Loved:
Quick, smooth read.
Easy-to-follow code examples.
The first few chapters served as a stellar introduction to the basics of machine learning.
Contain subtle jokes that add a bit of fun.
Tip to use the Python package manager Anaconda with Jupyter Notebooks was helpful.
Disliked:
Once again, installation was a challenge.
The "mglearn" utility library threw me for a loop. I had to reread the first few chapters before I figured out it was support for the book.
Although I liked the book, I didn't love it. Overall it just missed the "empowering" mark.
Writers:
Andreas C. Müller:
PhD in Computer Science
Lecturer at the Data Science Institute at Columbia University
Worked at the NYU Center for Data Science on open source and open science
Former Machine Learning Scientist at Amazon
Speaks often on Machine Learning and scikit-learn (a popular machine learning library)
And he makes some pretty incredibly useful graphics, such as this scikit-learn cheat sheet:
Image source: http://peekaboo-vision.blogspot.com/2013/01/machin...
Sarah Guido:
Former senior data scientist at Mashable
Lead data scientist at Bitly
2018 SciPy Conference Data Science track co-chair
Links:
https://www.amazon.com/Introduction-Machine-Learning-Python-Scientists/dp/1449369413/ref=sr_1_7?s=books&ie=UTF8&qid=1516734322&sr=1-7&keywords=python+machine+learning
http://shop.oreilly.com/product/0636920030515.do
Tips on Reading:
Type out code examples.
Beware of the "mglearn" utility library.
Udacity: Machine Learning by Georgia Tech ↓
Need to Know: A mix between an online learning experience and a university machine learning teaching approach. The lecturers are fun, but the course still fell a bit short in terms of active learning.
Loved:
This class is offered as CS7641 at Georgia Tech, where it is a part of the Online Masters Degree. Although taking this course here will not earn credit towards the OMS degree, it's still a non-watered-down college teaching philosophy approach.
Covers a wide variety of topics, many of which reminded me of the Caltech course (including: VC Dimension versus Bayesian, Occam's razor, etc.)
Discusses Markov Decision Chains, which is something that didn't really come up in many other introductory machine learning course, but they are referenced within Google patents.
The lecturers have a great dynamic, are wicked smart, and displayed a great sense of (nerd) humor, which make the topics less intimidating.
The course has quizzes, which give the course a slight amount of interaction.
Disliked:
Some videos were very long, which made the content a bit harder to digest.
The course overall was very time consuming.
Despite the quizzes, the course was a very passive form of learning with no assignments and little coding.
Many videos started with a bunch of content already written out. Having the content written out was probably a big time-saver, but it was also a bit jarring for a viewer to see so much information all at once, while also trying to listen.
It's vital to pay very close attention to notation, which compounds in complexity quickly.
Tablet version didn't function flawlessly: some was missing content (which I had to mark down and review on a desktop), the app would crash randomly on the tablet, and sometimes the audio wouldn't start.
There were no subtitles available on tablet, which I found not only to be a major accessibility blunder, but also made it harder for me to process (since I'm not an audio learner).
Lecturer:
Michael Littman:
Professor of Computer Science at Brown University.
Was granted a patent for one of the earliest systems for Cross-language information retrieval
Perhaps the most interesting man in the world:
Been in two TEDx talks
How I Learned to Stop Worrying and Be Realistic About AI
A Cooperative Path to Artificial Intelligence
During his time at Duke, he worked on an automated crossword solver (PROVERB)
Has a Family Quartet
He has appeared in a TurboTax commercial
Charles Isbell:
Professor and Executive Associate Dean at School of Interactive Computing at Georgia Tech
Focus on statistical machine learning and "interactive" artificial intelligence.
Links:
https://www.udacity.com/course/machine-learning--ud262
Tips on Watching:
Pick specific topics of interest and focusing on those lessons.
Andrew Ng's Stanford's Machine Learning iTunes ↓
Need to Know: A non-watered-down Stanford course. It's outdated (filmed in 2008), video/audio are a bit poor, and most links online now point towards the Coursera course. Although the idea of watching a Stanford course was energizing for the first few courses, it became dreadfully boring. I made it to course six before calling it.
Loved:
Designed for students, so you know you're not missing out on anything.
This course provides a deeper study into the mathematical and theoretical foundation behind machine learning to the point that the students could create their own machine learning algorithms. This isn't necessarily very practical for the everyday machine learning user.
Has some powerful real-world examples (although they're outdated).
There is something about the kinesthetic nature of watching someone write information out. The blackboard writing helped me to process certain ideas.
Disliked:
Video and audio quality were pain to watch.
Many questions asked by students were hard to hear.
On-screen visuals range from hard to impossible to see.
Found myself counting minutes.
Dr. Ng mentions TA classes, supplementary learning, but these are not available online.
Sometimes the video showed students, which I felt was invasive.
Lecturer:
Andrew Ng (see above)
Links:
https://itunes.apple.com/us/course/machine-learning/id495053006
https://www.youtube.com/watch?v=UzxYlbK2c7E
Tips on Watching:
Only watch if you're looking to gain a deeper understanding of the math presented in the Coursera course.
Skip the first half of the first lecture, since it's mostly class logistics.
Additional Resources
Fast.ai (part 2) - free access to materials, cost for AWS EC2 instance
Deeplearning.ai - $50/month
Udacity Machine Learning Engineer Nanodegree - $1K
https://machinelearningmastery.com/
Motivations and inspiration
If you're wondering why I spent a year doing this, then I'm with you. I'm genuinely not sure why I set my sights on this project, much less why I followed through with it. I saw Mike King give a session on Machine Learning. I was caught off guard, since I knew nothing on the topic. It gave me a pesky, insatiable curiosity itch. It started with one course and then spiraled out of control. Eventually it transformed into an idea: a review guide on the most affordable and popular machine learning resources on the web (through the lens of a complete beginner). Hopefully you found it useful, or at least somewhat interesting. Be sure to share your thoughts or questions in the comments!
Sign up for The Moz Top 10, a semimonthly mailer updating you on the top ten hottest pieces of SEO news, tips, and rad links uncovered by the Moz team. Think of it as your exclusive digest of stuff you don't have time to hunt down but want to read!
A Machine Learning Guide for Average Humans published first on https://steelcitygaragedoors.blogspot.com
0 notes
Text
Last-minute Christmas 2017 shopping list
Christmas is one week away and your list of gifts is not yet complete? Here are some ideas you may want to explore. This list, together with the Black Friday lists we published recently – some of the deals extend until New Year – will give you more options when it comes to creating your last-minute Christmas shopping list.
Get a FlatHat for your drone
Suitable for DJI Phantom 3, DJI Inspire, 3DR Solo, Parrot Bebop and similarly-sized drones, the 32” (80cm) FlatHat is a collapsible drone pad which provides easy-to-see landing target and “return-home” location. Ideal to protect your drone sensors and camera from rotor wash debris, it can also be used to perfect your landing skills and makes small drones on the ground easier to see.
Made with durable, heavy-duty fabric, the 32” FlatHat colapses to only 12″ diameter for storage and comes with integrated loops to attach FlatHat Lighting Kit, which comes as an offer in this special Christmas 2017 package from FlatHatLabs. The lighting kit incorporates a flexible, plastic coated electroluminescent wire that attaches to the perimeter of the 32” FlatHat Collapsible Drone Pad, allowing for better visibility in low-light flying conditions.
The whole kit has a special price of $29.95 instead of $47.90. Find more information about the product following the link.
Aurora and Luminar for Christmas
The HDR editor Aurora is Mac App of the Year by Apple, and to celebrate the fact, Macphun has a special offer on the Aurora HDR 2018 for Mac and Windows. The software is priced at $79 (against the regular $99) and comes with bonuses ($130 value). The bundle also includes Trey Ratcliff Deep Dive video, one ebokx explaining how to create realistic HDR images and 2 Preset Packs with 40 presets: “Winter Vibes” & “Dreamy Wonderland”.
The photo editor Luminar for Mac and Windows is also on offer, priced at $69 with bonuses totaling a $150 value. The uprade price for Luminar for Windows public beta users is also special: $59 and bonuses ($150 value), while Luminar users can upgrade for $49 plus bonuses ($150 value). The bundle includes 500+ Sky overlays for Sky replacement, a Preset pack: 20 Festive Texture Presets, the Shootkit ebook, a Sky Replacement video tutorial and “CAPTURE: Adventures in Photography” tips & inspirational ebook by motorcycle adventurers Simon & Lisa Thomas.
Power Director 16 costs 70% less
Cyberlink celebrates Christmas with 12 days of special prices that can go as low as 70% off or more, on different products from its catalog. One for video editors is Power Director 16, the most recent version of Cyberlink’s NLE, which is available for $74.99, instead of $399.99, in a bundle which includes AudioDirector 7, the Holiday Pack 9 and access to webinar for online classes, online presentation, and webinar.
If you want the whole package, then the Director Suite 6, including PowerDirector 16, PhotoDirector 9, ColorDirector 6, and AudioDirector 8, with the Holiday Pack 9 and Sound Effects as bonus, along with a gift card for an additional 10% off when buying PowerDVD 17 is your product. Save $100, paying only $189.99. These offers end December 26.
Affinity software with 20% off
Two of the hottest creative apps of 2017 – Affinity Photo and Affinity Designer – are now available with at least 20% off to celebrate the New Year. Available for a one-off payment, with no subscription, Affinity apps take all the leading professional file types, such as psd, svg, pdf or eps, comfortably in their stride. Or you can work in the proprietary Affinity file format, which offers complete compatibility across apps, including saved edit history, so you can switch between apps or pass work on to others in your team.
The 20 per cent discount runs from 18 December until 5 January and means the desktop apps are discounted by around 20 per cent to $39.99 / £38.99 / 43,99€ (subject to regional variations) for Mac from the Mac App Store, or for Windows direct from https://affinity.serif.com or Microsoft Store. Affinity Photo for iPad is discounted by around 25 per cent, and costs $14.99 / £14.99 / 16,99€ (subject to regional variations) from the App Store during the sale.
A eGPU for your PC or Mac
We’ve mentioned recently the Akitio Thunder3 Node Pro, but this Christmas list could not be complete without it, so here it is: the external boost to your PC or Mac. It’s the newest product from Akitio, a new external PCIe eGPU box able to take the full-size graphics card you can not fit inside your laptop of desktop computer. Suddenly you’ve the means to deal with intense graphic applications, without changing computer.
The Akitio Thunder3 Node Pro is available this December, just in time for Christmas, for $359.99. Follow the link if you want to find more about this solution for all your video editing problems.
Go Rogue with your flash
Rogue Photographic Design has a special package for photographers using flash: their Rogue FlashBender 2 XL Pro Lighting System, winner of Professional Photographer’s 2015 Hot One Award for Best SpeedLight Modifier, and a 20″ x 40″ Super Soft Silver Reflector, for $119.95.
Designed for portability, the FlashBender 2 XL Pro Lighting System is sized to fit a 15″ laptop compartment or lay flat to allow more room for lenses and other essential equipment in the gear bag. The Rogue 2-in-1 Super Soft Silver collapsible reflector is presented as a solution to avoid hot spots common with shiny silver reflectors. The other side of the reflector offers Natural White, said to provide a more subtle reflected light. The reflector collapses to 12″ diameter to easily stow in gear bag. Follow the link to read more about the special kit from Rogue Photographic Design.
ExpoDisc 2.0 and Color Correction Kit
The ExpoDisc 2.0 Professional White Balance Filter, allows users to set white balance, meter for exposure and dust map your camera sensor with the only calibrated and certified 18% transmission incident light metering filter. Easy to use, as a larger ExpoDisc can be used with smaller filter size lenses by simply holding the ExpoDisc over the lens during the white balance procedure, the accessory allows to quickly set white balance for photo and video.
The special package available now includes a Color Correction Kit using Rogue Flash Gels, ideal for speedlite photography. The kit includes 3 Rogue Gel tabbed attachment bands and 3x each of the following color correction gels: 1/4 CTO, 1/2 CTO, Full CTO, 1/2 CTB, Plus Green, Full White Diffusion Diffusion (1 Stop), making it ideal for portrait photographers working in mixed lighting environments. The kit, available with protective pouches, costs $ 74.95.
RapidMounts for Speedlights
For those regularly needing to places flashes in impossible places, TetherTools created the RapidMount Series, including grips and other solutions. Designed for location shoots, events, weddings, architectural shoots, and street photography, RapidMounts attach to walls and other vertical surfaces quickly and discreetly with the use of RapidStrips.
For quickly placing flashes, there is the RapidMount SLX with RapidStrips, priced at $ 24.95, is a multi-surface speedlight holder that allows mounting off-camera flashes to a wall and other surfaces using the included ten (10) specially-formulated, pressure-activated RapidStrips.The RapidStrips are a one-time use industrial-formula double-sided adhesive that uses a synthetic rubber, which provides superior performance while leaving no sticky residue upon removal – similar to gaffer tape.
Save with Vegas Pro family
Christmas is the time for family, and MAGIX is promoting the entire VEGAS Pro family of products at a special price… but only for a limited time… until December 20. Shop now and save. Free when you upgrade to any version of VEGAS, are Boris Continuum 3D Objects Unit, a US$299 value, NewBlueFX ColorFast2, a US$99 value, and NewBlueFX Stylizers 5 Imagine, a US$99 value. That’s a total of $497 in savings in plug-ins!
Upgrading costs $149 for the VEGAS Pro 15 Edit version, $199 for the VEGAS Pro 15 and $299 for the VEGAS Pro 15 Suite. For new buyers there are also special conditions, available until December 20, with prices starting at $149.00 (regularly $498.00) for the VEGAS Pro 15 Edit version, with the offer of NewBlueFX Stylizers 5 Imagine ($99 value).
A new 4K monitor for 2018
We recently published a selection of video and photo monitors for 2018, but decided to pick one for the Christmas shopping list, and we choose the Acer ProDesigner PE320QK launched this month in North America. The 4K UHD (3840 x 2160) monitor comes with visuals, precise color reproduction and an adjustable shading hood to meet the needs of photographers, video editors and graphic designers.
Engineered for professionals who require the most exacting quality standards, according to Acer,, the monitor is presented as the ideal choice for animators, film producers and other creative experts, who will be delighted with the performance the Acer ProDesigner PE320QK offers. The suggested price is $1199.
The post Last-minute Christmas 2017 shopping list appeared first on ProVideo Coalition.
First Found At: Last-minute Christmas 2017 shopping list
0 notes
Text
A Machine Learning Guide for Average Humans
A Machine Learning Guide for Average Humans
Posted by alexis-sanders
//<![CDATA[ (function($) { // code using $ as alias to jQuery $(function() { // Hide the hypotext content. $('.hypotext-content').hide(); // When a hypotext link is clicked. $('a.hypotext.closed').click(function (e) { // custom handling here e.preventDefault(); // Create the class reference from the rel value. var id = '.' + $(this).attr('rel'); // If the content is hidden, show it now. if ( $(id).css('display') == 'none' ) { $(id).show('slow'); if (jQuery.ui) { // UI loaded $(id).effect("highlight", {}, 1000); } } // If the content is shown, hide it now. else { $(id).hide('slow'); } }); // If we have a hash value in the url. if (window.location.hash) { // If the anchor is within a hypotext block, expand it, by clicking the // relevant link. console.log(window.location.hash); var anchor = $(window.location.hash); var hypotextLink = $('#' + anchor.parents('.hypotext-content').attr('rel')); console.log(hypotextLink); hypotextLink.click(); // Wait until the content has expanded before jumping to anchor. //$.delay(1000); setTimeout(function(){ scrollToAnchor(window.location.hash); }, 1000); } }); function scrollToAnchor(id) { var anchor = $(id); $('html,body').animate({scrollTop: anchor.offset().top},'slow'); } })(jQuery); //]]>
Machine learning (ML) has grown consistently in worldwide prevalence. Its implications have stretched from small, seemingly inconsequential victories to groundbreaking discoveries. The SEO community is no exception. An understanding and intuition of machine learning can support our understanding of the challenges and solutions Google's engineers are facing, while also opening our minds to ML's broader implications.
The advantages of gaining an general understanding of machine learning include:
Gaining empathy for engineers, who are ultimately trying to establish the best results for users
Understanding what problems machines are solving for, their current capabilities and scientists' goals
Understanding the competitive ecosystem and how companies are using machine learning to drive results
Preparing oneself for for what many industry leaders call a major shift in our society (Andrew Ng refers to AI as a "new electricity")
Understanding basic concepts that often appear within research (it's helped me with understanding certain concepts that appear within Google Brain's Research)
Growing as an individual and expanding your horizons (you might really enjoy machine learning!)
When code works and data is produced, it's a very fulfilling, empowering feeling (even if it's a very humble result)
I spent a year taking online courses, reading books, and learning about learning (...as a machine). This post is the fruit borne of that labor -- it covers 17 machine learning resources (including online courses, books, guides, conference presentations, etc.) comprising the most affordable and popular machine learning resources on the web (through the lens of a complete beginner). I've also added a summary of "If I were to start over again, how I would approach it."
This article isn't about credit or degrees. It's about regular Joes and Joannas with an interest in machine learning, and who want to spend their learning time efficiently. Most of these resources will consume over 50 hours of commitment. Ain't nobody got time for a painful waste of a work week (especially when this is probably completed during your personal time). The goal here is for you to find the resource that best suits your learning style. I genuinely hope you find this research useful, and I encourage comments on which materials prove most helpful (especially ones not included)! #HumanLearningMachineLearning
Executive summary:
Here's everything you need to know in a chart:
Machine Learning Resource
Time (hours)
Cost ($)
Year
Credibility
Code
Math
Enjoyability
Jason Maye's Machine Learning 101 slidedeck: 2 years of headbanging, so you don't have to
2
$0
'17
{ML} Recipes with Josh Gordon Playlist
2
$0
'16
Machine Learning Crash Course
15
$0
'18
OCDevel Machine Learning Guide Podcast
30
$0
'17-
Kaggle's Machine Learning Track (part 1)
6
$0
'17
Fast.ai (part 1)
70
$70*
'16
Hands-On Machine Learning with Scikit-Learn and TensorFlow: Concepts, Tools, and Techniques to Build Intelligent Systems
20
$25
'17
Udacity's Intro to Machine Learning (Kate/Sebastian)
60
$0
'15
Andrew Ng's Coursera Machine Learning
55
$0
'11
iPullRank Machine Learning Guide
3
$0
'17
Review Google PhD
2
$0
'17
Caltech Machine Learning on iTunes
27
$0
'12
Pattern Recognition & Machine Learning by Christopher Bishop
150
$75
'06
N/A
Machine Learning: Hands-on for Developers and Technical Professionals
15
$50
'15
Introduction to Machine Learning with Python: A Guide for Data Scientists
15
$25
'16
Udacity's Machine Learning by Georgia Tech
96
$0
'15
Machine Learning Stanford iTunes by Andrew Ng
25
$0
'08
N/A
*Free, but there is the cost of running an AWS EC2 instance (~$70 when I finished, but I did tinker a ton and made a Rick and Morty script generator, which I ran many epochs [rounds] of...)
Here's my suggested program:
1. Starting out (estimated 60 hours)
Start with shorter content targeting beginners. This will allow you to get the gist of what's going on with minimal time commitment.
Commit three hours to Jason Maye's Machine Learning 101 slidedeck: 2 years of headbanging, so you don't have to.
Commit two hours to watch Google's {ML} Recipes with Josh Gordon YouTube Playlist.
Sign up for Sam DeBrule's Machine Learnings newsletter.
Work through Google's Machine Learning Crash Course.
Start listening to OCDevel's Machine Learning Guide Podcast (skip episodes 1, 3, 16, 21, and 26) in your car, working out, and/or when using hands and eyes for other activities.
Commit two days to working through Kaggle's Machine Learning Track part 1.
2. Ready to commit (estimated 80 hours)
By this point, learners would understand their interest levels. Continue with content focused on applying relevant knowledge as fast as possible.
Commit to Fast.ai 10 hours per week, for 7 weeks. If you have a friend/mentor that can help you work through AWS setup, definitely lean on any support in installation (it's 100% the worst part of ML).
Acquire Hands-On Machine Learning with Scikit-Learn and TensorFlow: Concepts, Tools, and Techniques to Build Intelligent Systems, and read the first two chapters immediately. Then use this as supplemental to the Fast.ai course.
3. Broadening your horizons (estimated 115 hours)
If you've made it through the last section and are still hungry for more knowledge, move on to broadening your horizons. Read content focused on teaching the breadth of machine learning -- building an intuition for what the algorithms are trying to accomplish (whether visual or mathematically).
Start watching videos and participating in Udacity's Intro to Machine Learning (by Sebastian Thrun and Katie Malone).
Work through Andrew Ng's Coursera Machine Learning course.
Your next steps
By this point, you will already have AWS running instances, a mathematical foundation, and an overarching view of machine learning. This is your jumping-off point to determine what you want to do.
You should be able to determine your next step based on your interest, whether it's entering Kaggle competitions; doing Fast.ai part two; diving deep into the mathematics with Pattern Recognition & Machine Learning by Christopher Bishop; giving Andrew Ng's newer Deeplearning.ai course on Coursera; learning more about specific tech stacks (TensorFlow, Scikit-Learn, Keras, Pandas, Numpy, etc.); or applying machine learning to your own problems.
Why am I recommending these steps and resources?
I am not qualified to write an article on machine learning. I don't have a PhD. I took one statistics class in college, which marked the first moment I truly understood "fight or flight" reactions. And to top it off, my coding skills are lackluster (at their best, they're chunks of reverse-engineered code from Stack Overflow). Despite my many shortcomings, this piece had to be written by someone like me, an average person.
Statistically speaking, most of us are average (ah, the bell curve/Gaussian distribution always catches up to us). Since I'm not tied to any elitist sentiments, I can be real with you. Below contains a high-level summary of my reviews on all of the classes I took, along with a plan for how I would approach learning machine learning if I could start over. Click to expand each course for the full version with notes.
In-depth reviews of machine learning courses:
Starting out
Jason Maye's Machine Learning 101 slidedeck: 2 years of head-banging, so you don't have to ↓
Need to Know: A stellar high-level overview of machine learning fundamentals in an engaging and visually stimulating format.
Loved:
Very user-friendly, engaging, and playful slidedeck.
Has the potential to take some of the pain out of the process, through introducing core concepts.
Breaks up content by beginner/need-to-know (green), and intermediate/less-useful noise (specifically for individuals starting out) (blue).
Provides resources to dive deeper into machine learning.
Provides some top people to follow in machine learning.
Disliked:
That there is not more! Jason's creativity, visual-based teaching approach, and quirky sense of humor all support the absorption of the material.
Lecturer:
Jason Mayes:
Senior Creative Technologist and Research Engineer at Google
Masters in Computer Science from University of Bristols
Personal Note: He's also kind on Twitter! :)
Links:
Machine Learning 101 slide deck
Tips on Watching:
Set aside 2-4 hours to work through the deck once.
Since there is a wealth of knowledge, refer back as needed (or as a grounding source).
Identify areas of interest and explore the resources provided.
{ML} Recipes with Josh Gordon ↓
Need to Know: This mini-series YouTube-hosted playlist covers the very fundamentals of machine learning with opportunities to complete exercises.
Loved:
It is genuinely beginner-focused.
They make no assumption of any prior knowledge.
Gloss over potentially complex topics that may serve as noise.
Playlist ~2 hours
Very high-quality filming, audio, and presentation, almost to the point where it had its own aesthetic.
Covers some examples in scikit-learn and TensorFlow, which felt modern and practical.
Josh Gordon was an engaging speaker.
Disliked:
I could not get Dockers on Windows (suggested package manager). This wasn't a huge deal, since I already had my AWS setup by this point; however, a bit of a bummer since it made it impossible to follow certain steps exactly.
Issue: Every time I tried to download (over the course of two weeks), the .exe file would recursively start and keep spinning until either my memory ran out, computer crashed, or I shut my computer down. I sent this to Docker's Twitter account to no avail.
Lecturer:
Josh Gordon:
Developer Advocate for at TensorFlow at Google
Leads Machine Learning advocacy at Google
Member of the Udacity AI & Data Industry Advisory Board
Masters in Computer Science from Columbia University
Links:
Hello World - Machine Learning Recipes #1 (YouTube)
GitHub: Machine Learning Recipes with Josh Gordon
Tips on Watching:
The playlist is short (only ~1.5 hours screen time). However, it can be a bit fast-paced at times (especially if you like mimicking the examples), so set aside 3-4 hours to play around with examples and allow time for installation, pausing, and following along.
Take time to explore code labs.
Google's Machine Learning Crash Course with TensorFlow APIs ↓
Need to Know: A Google researcher-made crash course on machine learning that is interactive and offers its own built-in coding system!
Loved:
Different formats of learning: high-quality video (with ability to adjust speed, closed captioning), readings, quizzes (with explanations), visuals (including whiteboarding), interactive components/ playgrounds, code lab exercises (run directly in your browser (no setup required!))
Non-intimidating
One of my favorite quotes: "You don't need to understand the math to be able to take a look at the graphical interpretation."
Broken down into digestible sections
Introduces key terms
Disliked:
N/A
Lecturers:
Multiple Google researchers participated in this course, including:
Peter Norvig
Director of Research at Google Inc.
Previously he directed Google's core search algorithms group.
He is co-author of Artificial Intelligence: A Modern Approach
D. Sculley
Senior Staff Software Engineer at Google
KDD award-winning papers
Works on massive-scale ML systems for online advertising
Was part of a research ML paper on optimizing chocolate chip cookies
According to his personal website, he prefers to go by "D."
Cassandra Xia
Programmer, Software Engineer at Google
She has some really cool (and cute) projects based on learning statistics concepts interactively
Maya Gupta
Leads Glassbox Machine Learning R&D team at Google
Associate Professor of Electrical Engineering at the University of Washington (2003-2012)
In 2007, Gupta received the PECASE award from President George Bush for her work in classifying uncertain (e.g. random) signals
Gupta also runs Artifact Puzzles, the second-largest US maker of wooden jigsaw puzzles
Sally Goldman
Research Scientist at Google
Co-author of A Practical Guide to Data Structures and Algorithms Using Java
Numerous journals, classes taught at Washington University, and contributions to the ML community
Links:
Machine Learning Crash Course
Tips on Doing:
Actively work through playground and coding exercises
OCDevel's Machine Learning Guide Podcast ↓
Need to Know: This podcast focuses on the high-level fundamentals of machine learning, including basic intuition, algorithms, math, languages, and frameworks. It also includes references to learn more on each episode's topic.
Loved:
Great for trips (when traveling a ton, it was an easy listen).
The podcast makes machine learning fun with interesting and compelling analogies.
Tyler is a big fan of Andrew Ng's Coursera course and reviews concepts in Coursera course very well, such that both pair together nicely.
Covers the canonical resources for learning more on a particular topic.
Disliked:
Certain courses were more theory-based; all are interesting, yet impractical.
Due to limited funding the project is a bit slow to update and has less than 30 episodes.
Podcaster:
Tyler Renelle:
Machine learning engineer focused on time series and reinforcement
Background in full-stack JavaScript, 10 years web and mobile
Creator of HabitRPG, an app that treats habits as an RPG game
Links:
Machine Learning Guide podcast
Machine Learning Guide podcast (iTunes)
Tips on Listening:
Listen along your journey to help solidify understanding of topics.
Skip episodes 1, 3, 16, 21, and 26 (unless their topics interest and inspire you!).
Kaggle Machine Learning Track (Lesson 1) ↓
Need to Know: A simple code lab that covers the very basics of machine learning with scikit-learn and Panda through the application of the examples onto another set of data.
Loved:
A more active form of learning.
An engaging code lab that encourages participants to apply knowledge.
This track offers has a built-in Python notebook on Kaggle with all input files included. This removed any and all setup/installation issues.
Side note: It's a bit different than Jupyter notebook (e.g., have to click into a cell to add another cell).
Each lesson is short, which made the entire lesson go by very fast.
Disliked:
The writing in the first lesson didn't initially make it clear that one would need to apply the knowledge in the lesson to their workbook.
It wasn't a big deal, but when I started referencing files in the lesson, I had to dive into the files in my workbook to find they didn't exist, only to realize that the knowledge was supposed to be applied and not transcribed.
Lecturer:
Dan Becker:
Data Scientist at Kaggle
Undergrad in Computer Science, PhD in Econometrics
Supervised data science consultant for six Fortune 100 companies
Contributed to the Keras and Tensorflow libraries
Finished 2nd (out of 1353 teams) in $3 million Heritage Health Prize data mining competition
Speaks at deep learning workshops at events and conferences
Links:
https://www.kaggle.com/learn/machine-learning
Tips on Doing:
Read the exercises and apply to your dataset as you go.
Try lesson 2, which covers more complex/abstract topics (note: this second took a bit longer to work through).
Ready to commit
Fast.ai (part 1 of 2) ↓
Need to Know: Hands-down the most engaging and active form of learning ML. The source I would most recommend for anyone (although the training plan does help to build up to this course). This course is about learning through coding. This is the only course that I started to truly see the practical mechanics start to come together. It involves applying the most practical solutions to the most common problems (while also building an intuition for those solutions).
Loved:
Course Philosophy:
Active learning approach
"Go out into the world and understand underlying mechanics (of machine learning by doing)."
Counter-culture to the exclusivity of the machine learning field, focusing on inclusion.
"Let's do shit that matters to people as quickly as possible."
Highly pragmatic approach with tools that are currently being used (Jupyter Notebooks, scikit-learn, Keras, AWS, etc.).
Show an end-to-end process that you get to complete and play with in a development environment.
Math is involved, but is not prohibitive. Excel files helped to consolidate information/interact with information in a different way, and Jeremy spends a lot of time recapping confusing concepts.
Amazing set of learning resources that allow for all different styles of learning, including:
Video Lessons
Notes
Jupyter Notebooks
Assignments
Highly active forums
Resources on Stackoverflow
Readings/resources
Jeremy often references popular academic texts
Jeremy's TEDx talk in Brussels
Jeremy really pushes one to do extra and put in the effort by teaching interesting problems and engaging one in solving them.
It's a huge time commitment; however, it's worth it.
All of the course's profits are donated.
Disliked:
Overview covers their approach to learning (obviously I'm a fan!). If you're already drinking the Kool-aid, skip past.
I struggled through the AWS setup (13-minute video) for about five hours (however, it felt so good when it was up and running!).
Because of its practicality and concentration on solutions used today to solve popular problem types (image recognition, text generation, etc.), it lacks breadth of machine learning topics.
Lecturers:
Jeremy Howard:
Distinguished Research Scientist at the University of San Francisco
Faculty member at Singularity University
Young Global Leader with the World Economic Forum
Founder of Enlitic (the first company to apply deep learning to medicine)
Former President and Chief Scientist of the data science platform Kaggle
Rachel Thomas:
PhD in Math from Duke
One of Forbes' "20 Incredible Women Advancing AI Research"
Researcher-in-residence at the University of San Francisco Data Institute
Teaches in the Masters in Data Science program
Links:
http://course.fast.ai/start.html
http://wiki.fast.ai/index.php/Main_Page
https://github.com/fastai/courses/tree/master/deeplearning1/nbs
Tips on Doing:
Set expectations with yourself that installation is going to probably take a few hours.
Prepare to spend about ~70 hours for this course (it's worth it).
Don't forget to shut off your AWS instance.
Balance out machine learning knowledge with a course with more breadth.
Consider giving part two of the Fast.ai program a shot!
Hands-On Machine Learning with Scikit-Learn and TensorFlow: Concepts, Tools, and Techniques to Build Intelligent Systems ↓
Need to Know: This book is an Amazon best seller for a reason. It covers a lot of ground quickly, empowers readers to walk through a machine learning problem by chapter two, and contains practical up-to-date machine learning skills.
Loved:
Book contains an amazing introduction to machine learning that briskly provides an overarching quick view of the machine learning ecosystem.
Chapter 2 immediately walks the reader through an end-to-end machine learning problem.
Immediately afterwards, Aurélien pushes a user to attempt to apply this solution to another problem, which was very empowering.
There are review questions at the end of each chapter to ensure on has grasped the content within the chapter and to push the reader to explore more.
Once installation was completed, it was easy to follow and all code is available on GitHub.
Chapters 11-14 were very tough reading; however, they were a great reference when working through Fast.ai.
Contains some powerful analogies.
Each chapter's introductions were very useful and put everything into context. This general-to-specifics learning was very useful.
Disliked:
Installation was a common source of issues during the beginning of my journey; the text glided over this. I felt the frustration that most people experience from installation should have been addressed with more resources.
Writer:
Aurélien Géron:
Led the YouTube video classification team from 2013 to 2016
Currently a machine Learning consultant
Founder and CTO of Wifirst and Polyconseil
Published technical books (on C++, Wi-Fi, and Internet architectures)
Links:
https://www.amazon.com/_/dp/1491962291?tag=oreilly20-20
http://shop.oreilly.com/product/0636920052289.do
https://github.com/ageron/handson-ml
Tips on Using:
Get a friend with Python experience to help with installation.
Read the introductions to each chapter thoroughly, read the chapter (pay careful attention to code), review the questions at the end (highlight any in-text answer), make a copy of Aurélien's GitHub and make sure everything works on your setup, re-type the notebooks, go to Kaggle and try on other datasets.
Broadening your horizons
Udacity: Intro to Machine Learning (Kate/Sebastian) ↓
Need to Know: A course that covers a range of machine learning topics, supports building of intuition via visualization and simple examples, offers coding challenges, and a certificate (upon completion of a final project). The biggest challenge with this course is bridging the gap between the hand-holding lectures and the coding exercises.
Loved:
Focus on developing a visual intuition on what each model is trying to accomplish.
This visual learning mathematics approach is very useful.
Cover a vast variety and breadth of models and machine learning basics.
In terms of presenting the concept, there was a lot of hand-holding (which I completely appreciated!).
Many people have done this training, so their GitHub accounts can be used as reference for the mini-projects.
Katie actively notes documentation and suggests where viewers can learn more/reference material.
Disliked:
All of the conceptual hand-holding in the lessons is a stark contrast to the challenges of installation, coding exercises, and mini-projects.
This is the first course started and the limited instructions on setting up the environment and many failed attempts caused me to break down crying at least a handful of times.
The mini-projects are intimidating.
There is extra code added to support the viewers; however, it's done so with little acknowledgement as to what it's actually doing. This made learning a bit harder.
Lecturer:
Caitlin (Katie) Malone:
Director of Data Science Research and Development at Civis Analytics
Stanford PhD in Experimental Particle Physics
Intern at Udacity in summer 2014
Graduate Researcher at the SLAC National Accelerator Laboratory
https://www6.slac.stanford.edu/
Podcaster with Ben Jaffe (currently Facebook UI Engineer and a music aficionado) on a machine learning podcast Linear Digressions (100+ episodes)
Sebastian Thrun:
CEO of the Kitty Hawk Corporation
Chairman and co-founder of Udacity
One of my favorite Sebastian quotes: "It occurred to me, I could be at Google and build a self-driving car, or I can teach 10,000 students how to build self-driving cars."
Former Google VP
Founded Google X
Led development of the robotic vehicle Stanley
Professor of Computer Science at Stanford University
Formerly a professor at Carnegie Mellon University.
Links:
https://www.udacity.com/course/intro-to-machine-learning--ud120
Udacity also offers a next step, the Machine Learning Engineer Nanodegree, which will set one back about $1K.
Tips on Watching:
Get a friend to help you set up your environment.
Print mini-project instructions to check off each step.
Andrew Ng's Coursera Machine Learning Course ↓
Need to Know: The Andrew Ng Coursera course is the most referenced online machine learning course. It covers a broad set of fundamental, evergreen topics with a strong focus in building mathematical intuition behind machine learning models. Also, one can submit assignments and earn a grade for free. If you want to earn a certificate, one can subscribe or apply for financial aid.
Loved:
This course has a high level of credibility.
Introduces all necessary machine learning terminology and jargon.
Contains a very classic machine learning education approach with a high level of math focus.
Quizzes interspersed in courses and after each lesson support understanding and overall learning.
The sessions for the course are flexible, the option to switch into a different section is always available.
Disliked:
The mathematic notation was hard to process at times.
The content felt a bit dated and non-pragmatic. For example, the main concentration was MATLAB and Octave versus more modern languages and resources.
Video quality was less than average and could use a refresh.
Lecturer:
Andrew Ng:
Adjunct Professor, Stanford University (focusing on AI, Machine Learning, and Deep Learning)
Co-founder of Coursera
Former head of Baidu AI Group
Founder and previous head of Google Brain (deep learning) project
Former Director of the Stanford AI Lab
Chairman of the board of Woebot (a machine learning bot that focuses on Cognitive Behavior Therapy)
Links:
https://www.coursera.org/learn/machine-learning/
Andrew Ng recently launched a new course (August 2017) called DeepLearning.ai, a ~15 week course containing five mini-courses ($49 USD per month to continue learning after trial period of 7 days ends).
Course: https://www.coursera.org/specializations/deep-learning
Course 1: Neural Networks and Deep Learning
Course 2: Improving Deep Neural Networks: Hyperparameter tuning, Regularization and Optimization
Course 3: Structuring Machine Learning Projects
Course 4: Convolutional Neural Networks
Course 5: Sequence Models
Tips on Watching:
Be disciplined with setting aside timing (even if it's only 15 minutes a day) to help power through some of the more boring concepts.
Don't do this course first, because it's intimidating, requires a large time commitment, and isn't a very energizing experience.
Additional machine learning opportunities
iPullRank Machine Learning Guide ↓
Need to Know: A machine learning e-book targeted at marketers.
Loved:
Targeted at marketers and applied to organic search.
Covers a variety of machine learning topics.
Some good examples, including real-world blunders.
Gives some practical tools for non-data scientists (including: MonkeyLearn and Orange)
I found Orange to be a lot of fun. It struggled with larger datasets; however, it has a very visual interface that was more user-friendly and offers potential to show some pretty compelling stories.
Example: World Happiness Dataset by:
X-axis: Happiness Score
Y-axis: Economy
Color: Health
Disliked:
Potential to break up content more with relevant imagery -- the content was very dense.
Writers:
iPullRank Team (including Mike King):
Mike King has a few slide decks on the basics of machine learnings and AI
iPullRank has a few data scientists on staff
Links:
http://ipullrank.com/machine-learning-guide/
Tips on Reading:
Read chapters 1-6 and the rest depending upon personal interest.
Review Google PhD ↓
Need to Know: A two-hour presentation from Google's 2017 IO conference that walks through getting 99% accuracy on the MNIST dataset (a famous dataset containing a bunch of handwritten numbers, which the machine must learn to identify the numbers).
Loved:
This talk struck me as very modern, covering the cutting edge.
Found this to be very complementary to Fast.ai, as it covered similar topics (e.g. ReLu, CNNs, RNNs, etc.)
Amazing visuals that help to put everything into context.
Disliked:
The presentation is only a short conference solution and not a comprehensive view of machine learning.
Also, a passive form of learning.
Presenter:
Martin Görner:
Developer Relations, Google (since 2011)
Started Mobipocket, a startup that later became the software part of the Amazon Kindle and its mobile variants
Links:
Part 1 - https://www.youtube.com/watch?v=u4alGiomYP4
Part 2 - https://www.youtube.com/watch?v=fTUwdXUFfI8
Tips on Watching:
Google any concepts you're unfamiliar with.
Take your time with this one; 2 hours of screen time doesn't count all of the Googling and processing time for this one.
Caltech Machine Learning iTunes ↓
Need to Know: If math is your thing, this course does a stellar job of building the mathematic intuition behind many machine learning models. Dr. Abu-Mostafa is a raconteur, includes useful visualizations, relevant real-world examples, and compelling analogies.
Loved:
First and foremost, this is a real Caltech course, meaning it's not a watered-down version and contains fundamental concepts that are vital to understanding the mechanics of machine learning.
On iTunes, audio downloads are available, which can be useful for on-the-go learning.
Dr. Abu-Mostafa is a skilled speaker, making the 27 hours spent listening much easier!
Dr. Abu-Mostafa offers up some strong real-world examples and analogies which makes the content more relatable.
As an example, he asks students: "Why do I give you practice exams and not just give you the final exam?" as an illustration of why a testing set is useful. If he were to just give students the final, they would just memorize the answers (i.e., they would overfit to the data) and not genuinely learn the material. The final is a test to show how much students learn.
The last 1/2 hour of the class is always a Q&A, where students can ask questions. Their questions were useful to understanding the topic more in-depth.
The video and audio quality was strong throughout. There were a few times when I couldn't understand a question in the Q&A, but overall very strong.
This course is designed to build mathematical intuition of what's going on under the hood of specific machine learning models.
Caution: Dr. Abu-Mostafa uses mathematical notation, but it's different from Andrew Ng's (e.g., theta = w).
The final lecture was the most useful, as it pulled a lot of the conceptual puzzle pieces together. The course on neural networks was a close second!
Disliked:
Although it contains mostly evergreen content, being released in 2012, it could use a refresh.
Very passive form of learning, as it wasn't immediately actionable.
Lecturer:
Dr. Yaser S. Abu-Mostafa:
Professor of Electrical Engineering and Computer Science at the California Institute of Technology
Chairman of Machine Learning Consultants LLC
Serves on a number of scientific advisory boards
Has served as a technical consultant on machine learning for several companies (including Citibank).
Multiple articles in Scientific American
Links:
https://work.caltech.edu/telecourse.html
https://itunes.apple.com/us/course/machine-learning/id515364596
Tips on Watching:
Consider listening to the last lesson first, as it pulls together the course overall conceptually. The map of the course, below, was particularly useful to organizing the information taught in the courses.
Image source: http://work.caltech.edu/slides/slides18.pdf
"Pattern Recognition & Machine Learning" by Christopher Bishop ↓
Need to Know: This is a very popular college-level machine learning textbook. I've heard it likened to a bible for machine learning. However, after spending a month trying to tackle the first few chapters, I gave up. It was too much math and pre-requisites to tackle (even with a multitude of Google sessions).
Loved:
The text of choice for many major universities, so if you can make it through this text and understand all of the concepts, you're probably in a very good position.
I appreciated the history aside sections, where Bishop talked about influential people and their career accomplishments in statistics and machine learning.
Despite being a highly mathematically text, the textbook actually has some pretty visually intuitive imagery.
Disliked:
I couldn't make it through the text, which was a bit frustrating. The statistics and mathematical notation (which is probably very benign for a student in this topic) were too much for me.
The sunk cost was pretty high here (~$75).
Writer:
Christopher Bishop:
Laboratory Director at Microsoft Research Cambridge
Professor of Computer Science at the University of Edinburgh
Fellow of Darwin College, Cambridge
PhD in Theoretical Physics from the University of Edinburgh
Links:
https://www.amazon.com/Pattern-Recognition-Learning-Information-Statistics/dp/0387310738/ref=sr_1_2?ie=UTF8&qid=1516839475&sr=8-2&keywords=Pattern+Recognition+%26+Machine+Learning
Tips on Reading:
Don't start your machine learning journey with this book.
Get a friend in statistics to walk you through anything complicated (my plan is to get a mentor in statistics).
Consider taking a (free) online statistics course (Khan Academy and Udacity both have some great content on statistics, calculus, math, and data analysis).
Machine Learning: Hands-on for Developers and Technical Professionals ↓
Need to Know: A fun, non-intimidating end-to-end launching pad/whistle stop for machine learning in action.
Loved:
Talks about practical issues that many other sources didn't really address (e.g. data-cleansing).
Covered the basics of machine learning in a non-intimidating way.
Offers abridged, consolidated versions of the content.
Added fun anecdotes that makes it easier to read.
Overall the writer has a great sense of humor.
Writer talks to the reader as if they're a real human being (i.e., doesn't expect you to go out and do proofs; acknowledges the challenge of certain concepts).
Covers a wide variety of topics.
Because it was well-written, I flew through the book (even though it's about ~300 pages).
Disliked:
N/A
Writer:
Jason Bell:
Technical architect, lecturer, and startup consultant
Data Engineer at MastodonC
Former section editor for Java Developer's Journal
Former writer on IBM DeveloperWorks
Links:
https://www.amazon.com/Machine-Learning-Hands-Developers-Professionals/dp/1118889061
https://www.wiley.com/en-us/Machine+Learning%3A+Hands+On+for+Developers+and+Technical+Professionals-p-9781118889060
Jason's Blog: https://dataissexy.wordpress.com/
Tips on Reading:
Download and explore Weka's interface beforehand.
Give some of the exercises a shot.
Introduction to Machine Learning with Python: A Guide for Data Scientists ↓
Need to Know: This was a was a well-written piece on machine learning, making it a quick read.
Loved:
Quick, smooth read.
Easy-to-follow code examples.
The first few chapters served as a stellar introduction to the basics of machine learning.
Contain subtle jokes that add a bit of fun.
Tip to use the Python package manager Anaconda with Jupyter Notebooks was helpful.
Disliked:
Once again, installation was a challenge.
The "mglearn" utility library threw me for a loop. I had to reread the first few chapters before I figured out it was support for the book.
Although I liked the book, I didn't love it. Overall it just missed the "empowering" mark.
Writers:
Andreas C. Müller:
PhD in Computer Science
Lecturer at the Data Science Institute at Columbia University
Worked at the NYU Center for Data Science on open source and open science
Former Machine Learning Scientist at Amazon
Speaks often on Machine Learning and scikit-learn (a popular machine learning library)
And he makes some pretty incredibly useful graphics, such as this scikit-learn cheat sheet:
Image source: http://peekaboo-vision.blogspot.com/2013/01/machin...
Sarah Guido:
Former senior data scientist at Mashable
Lead data scientist at Bitly
2018 SciPy Conference Data Science track co-chair
Links:
https://www.amazon.com/Introduction-Machine-Learning-Python-Scientists/dp/1449369413/ref=sr_1_7?s=books&ie=UTF8&qid=1516734322&sr=1-7&keywords=python+machine+learning
http://shop.oreilly.com/product/0636920030515.do
Tips on Reading:
Type out code examples.
Beware of the "mglearn" utility library.
Udacity: Machine Learning by Georgia Tech ↓
Need to Know: A mix between an online learning experience and a university machine learning teaching approach. The lecturers are fun, but the course still fell a bit short in terms of active learning.
Loved:
This class is offered as CS7641 at Georgia Tech, where it is a part of the Online Masters Degree. Although taking this course here will not earn credit towards the OMS degree, it's still a non-watered-down college teaching philosophy approach.
Covers a wide variety of topics, many of which reminded me of the Caltech course (including: VC Dimension versus Bayesian, Occam's razor, etc.)
Discusses Markov Decision Chains, which is something that didn't really come up in many other introductory machine learning course, but they are referenced within Google patents.
The lecturers have a great dynamic, are wicked smart, and displayed a great sense of (nerd) humor, which make the topics less intimidating.
The course has quizzes, which give the course a slight amount of interaction.
Disliked:
Some videos were very long, which made the content a bit harder to digest.
The course overall was very time consuming.
Despite the quizzes, the course was a very passive form of learning with no assignments and little coding.
Many videos started with a bunch of content already written out. Having the content written out was probably a big time-saver, but it was also a bit jarring for a viewer to see so much information all at once, while also trying to listen.
It's vital to pay very close attention to notation, which compounds in complexity quickly.
Tablet version didn't function flawlessly: some was missing content (which I had to mark down and review on a desktop), the app would crash randomly on the tablet, and sometimes the audio wouldn't start.
There were no subtitles available on tablet, which I found not only to be a major accessibility blunder, but also made it harder for me to process (since I'm not an audio learner).
Lecturer:
Michael Littman:
Professor of Computer Science at Brown University.
Was granted a patent for one of the earliest systems for Cross-language information retrieval
Perhaps the most interesting man in the world:
Been in two TEDx talks
How I Learned to Stop Worrying and Be Realistic About AI
A Cooperative Path to Artificial Intelligence
During his time at Duke, he worked on an automated crossword solver (PROVERB)
Has a Family Quartet
He has appeared in a TurboTax commercial
Charles Isbell:
Professor and Executive Associate Dean at School of Interactive Computing at Georgia Tech
Focus on statistical machine learning and "interactive" artificial intelligence.
Links:
https://www.udacity.com/course/machine-learning--ud262
Tips on Watching:
Pick specific topics of interest and focusing on those lessons.
Andrew Ng's Stanford's Machine Learning iTunes ↓
Need to Know: A non-watered-down Stanford course. It's outdated (filmed in 2008), video/audio are a bit poor, and most links online now point towards the Coursera course. Although the idea of watching a Stanford course was energizing for the first few courses, it became dreadfully boring. I made it to course six before calling it.
Loved:
Designed for students, so you know you're not missing out on anything.
This course provides a deeper study into the mathematical and theoretical foundation behind machine learning to the point that the students could create their own machine learning algorithms. This isn't necessarily very practical for the everyday machine learning user.
Has some powerful real-world examples (although they're outdated).
There is something about the kinesthetic nature of watching someone write information out. The blackboard writing helped me to process certain ideas.
Disliked:
Video and audio quality were pain to watch.
Many questions asked by students were hard to hear.
On-screen visuals range from hard to impossible to see.
Found myself counting minutes.
Dr. Ng mentions TA classes, supplementary learning, but these are not available online.
Sometimes the video showed students, which I felt was invasive.
Lecturer:
Andrew Ng (see above)
Links:
https://itunes.apple.com/us/course/machine-learning/id495053006
https://www.youtube.com/watch?v=UzxYlbK2c7E
Tips on Watching:
Only watch if you're looking to gain a deeper understanding of the math presented in the Coursera course.
Skip the first half of the first lecture, since it's mostly class logistics.
Additional Resources
Fast.ai (part 2) - free access to materials, cost for AWS EC2 instance
Deeplearning.ai - $50/month
Udacity Machine Learning Engineer Nanodegree - $1K
https://machinelearningmastery.com/
Motivations and inspiration
If you're wondering why I spent a year doing this, then I'm with you. I'm genuinely not sure why I set my sights on this project, much less why I followed through with it. I saw Mike King give a session on Machine Learning. I was caught off guard, since I knew nothing on the topic. It gave me a pesky, insatiable curiosity itch. It started with one course and then spiraled out of control. Eventually it transformed into an idea: a review guide on the most affordable and popular machine learning resources on the web (through the lens of a complete beginner). Hopefully you found it useful, or at least somewhat interesting. Be sure to share your thoughts or questions in the comments!
Sign up for The Moz Top 10, a semimonthly mailer updating you on the top ten hottest pieces of SEO news, tips, and rad links uncovered by the Moz team. Think of it as your exclusive digest of stuff you don't have time to hunt down but want to read!
xem them tai https://ift.tt/2o9GYfe A Machine Learning Guide for Average Humans xem thêm tại: https://ift.tt/2mb4VST để biết thêm về địa chỉ bán tai nghe không dây giá rẻ A Machine Learning Guide for Average Humans https://ift.tt/2rFyAUY xem thêm tại: https://ift.tt/2mb4VST để biết thêm về địa chỉ bán tai nghe không dây giá rẻ A Machine Learning Guide for Average Humans https://ift.tt/2rFyAUY xem thêm tại: https://ift.tt/2mb4VST để biết thêm về địa chỉ bán tai nghe không dây giá rẻ A Machine Learning Guide for Average Humans https://ift.tt/2rFyAUY xem thêm tại: https://ift.tt/2mb4VST để biết thêm về địa chỉ bán tai nghe không dây giá rẻ A Machine Learning Guide for Average Humans https://ift.tt/2rFyAUY xem thêm tại: https://ift.tt/2mb4VST để biết thêm về địa chỉ bán tai nghe không dây giá rẻ A Machine Learning Guide for Average Humans https://ift.tt/2rFyAUY xem thêm tại: https://ift.tt/2mb4VST để biết thêm về địa chỉ bán tai nghe không dây giá rẻ A Machine Learning Guide for Average Humans https://ift.tt/2rFyAUY xem thêm tại: https://ift.tt/2mb4VST để biết thêm về địa chỉ bán tai nghe không dây giá rẻ A Machine Learning Guide for Average Humans https://ift.tt/2rFyAUY xem thêm tại: https://ift.tt/2mb4VST để biết thêm về địa chỉ bán tai nghe không dây giá rẻ A Machine Learning Guide for Average Humans https://ift.tt/2rFyAUY xem thêm tại: https://ift.tt/2mb4VST để biết thêm về địa chỉ bán tai nghe không dây giá rẻ A Machine Learning Guide for Average Humans https://ift.tt/2rFyAUY xem thêm tại: https://ift.tt/2mb4VST để biết thêm về địa chỉ bán tai nghe không dây giá rẻ A Machine Learning Guide for Average Humans https://ift.tt/2rFyAUY xem thêm tại: https://ift.tt/2mb4VST để biết thêm về địa chỉ bán tai nghe không dây giá rẻ A Machine Learning Guide for Average Humans https://ift.tt/2rFyAUY xem thêm tại: https://ift.tt/2mb4VST để biết thêm về địa chỉ bán tai nghe không dây giá rẻ A Machine Learning Guide for Average Humans https://ift.tt/2rFyAUY xem thêm tại: https://ift.tt/2mb4VST để biết thêm về địa chỉ bán tai nghe không dây giá rẻ A Machine Learning Guide for Average Humans https://ift.tt/2rFyAUY xem thêm tại: https://ift.tt/2mb4VST để biết thêm về địa chỉ bán tai nghe không dây giá rẻ A Machine Learning Guide for Average Humans https://ift.tt/2rFyAUY xem thêm tại: https://ift.tt/2mb4VST để biết thêm về địa chỉ bán tai nghe không dây giá rẻ A Machine Learning Guide for Average Humans https://ift.tt/2rFyAUY xem thêm tại: https://ift.tt/2mb4VST để biết thêm về địa chỉ bán tai nghe không dây giá rẻ A Machine Learning Guide for Average Humans https://ift.tt/2rFyAUY xem thêm tại: https://ift.tt/2mb4VST để biết thêm về địa chỉ bán tai nghe không dây giá rẻ A Machine Learning Guide for Average Humans https://ift.tt/2rFyAUY xem thêm tại: https://ift.tt/2mb4VST để biết thêm về địa chỉ bán tai nghe không dây giá rẻ A Machine Learning Guide for Average Humans https://ift.tt/2rFyAUY xem thêm tại: https://ift.tt/2mb4VST để biết thêm về địa chỉ bán tai nghe không dây giá rẻ A Machine Learning Guide for Average Humans https://ift.tt/2rFyAUY xem thêm tại: https://ift.tt/2mb4VST để biết thêm về địa chỉ bán tai nghe không dây giá rẻ A Machine Learning Guide for Average Humans https://ift.tt/2rFyAUY xem thêm tại: https://ift.tt/2mb4VST để biết thêm về địa chỉ bán tai nghe không dây giá rẻ A Machine Learning Guide for Average Humans https://ift.tt/2rFyAUY xem thêm tại: https://ift.tt/2mb4VST để biết thêm về địa chỉ bán tai nghe không dây giá rẻ A Machine Learning Guide for Average Humans https://ift.tt/2rFyAUY xem thêm tại: https://ift.tt/2mb4VST để biết thêm về địa chỉ bán tai nghe không dây giá rẻ A Machine Learning Guide for Average Humans https://ift.tt/2rFyAUY xem thêm tại: https://ift.tt/2mb4VST để biết thêm về địa chỉ bán tai nghe không dây giá rẻ A Machine Learning Guide for Average Humans https://ift.tt/2rFyAUY xem thêm tại: https://ift.tt/2mb4VST để biết thêm về địa chỉ bán tai nghe không dây giá rẻ A Machine Learning Guide for Average Humans https://ift.tt/2rFyAUY xem thêm tại: https://ift.tt/2mb4VST để biết thêm về địa chỉ bán tai nghe không dây giá rẻ A Machine Learning Guide for Average Humans https://ift.tt/2rFyAUY xem thêm tại: https://ift.tt/2mb4VST để biết thêm về địa chỉ bán tai nghe không dây giá rẻ A Machine Learning Guide for Average Humans https://ift.tt/2rFyAUY xem thêm tại: https://ift.tt/2mb4VST để biết thêm về địa chỉ bán tai nghe không dây giá rẻ A Machine Learning Guide for Average Humans https://ift.tt/2rFyAUY xem thêm tại: https://ift.tt/2mb4VST để biết thêm về địa chỉ bán tai nghe không dây giá rẻ A Machine Learning Guide for Average Humans https://ift.tt/2rFyAUY xem thêm tại: https://ift.tt/2mb4VST để biết thêm về địa chỉ bán tai nghe không dây giá rẻ A Machine Learning Guide for Average Humans https://ift.tt/2rFyAUY xem thêm tại: https://ift.tt/2mb4VST để biết thêm về địa chỉ bán tai nghe không dây giá rẻ A Machine Learning Guide for Average Humans https://ift.tt/2rFyAUY xem thêm tại: https://ift.tt/2mb4VST để biết thêm về địa chỉ bán tai nghe không dây giá rẻ A Machine Learning Guide for Average Humans https://ift.tt/2rFyAUY xem thêm tại: https://ift.tt/2mb4VST để biết thêm về địa chỉ bán tai nghe không dây giá rẻ A Machine Learning Guide for Average Humans https://ift.tt/2rFyAUY xem thêm tại: https://ift.tt/2mb4VST để biết thêm về địa chỉ bán tai nghe không dây giá rẻ A Machine Learning Guide for Average Humans https://ift.tt/2rFyAUY xem thêm tại: https://ift.tt/2mb4VST để biết thêm về địa chỉ bán tai nghe không dây giá rẻ A Machine Learning Guide for Average Humans https://ift.tt/2rFyAUY xem thêm tại: https://ift.tt/2mb4VST để biết thêm về địa chỉ bán tai nghe không dây giá rẻ A Machine Learning Guide for Average Humans https://ift.tt/2rFyAUY xem thêm tại: https://ift.tt/2mb4VST để biết thêm về địa chỉ bán tai nghe không dây giá rẻ A Machine Learning Guide for Average Humans https://ift.tt/2rFyAUY xem thêm tại: https://ift.tt/2mb4VST để biết thêm về địa chỉ bán tai nghe không dây giá rẻ A Machine Learning Guide for Average Humans https://ift.tt/2rFyAUY xem thêm tại: https://ift.tt/2mb4VST để biết thêm về địa chỉ bán tai nghe không dây giá rẻ A Machine Learning Guide for Average Humans https://ift.tt/2rFyAUY xem thêm tại: https://ift.tt/2mb4VST để biết thêm về địa chỉ bán tai nghe không dây giá rẻ A Machine Learning Guide for Average Humans https://ift.tt/2rFyAUY xem thêm tại: https://ift.tt/2mb4VST để biết thêm về địa chỉ bán tai nghe không dây giá rẻ A Machine Learning Guide for Average Humans https://ift.tt/2rFyAUY xem thêm tại: https://ift.tt/2mb4VST để biết thêm về địa chỉ bán tai nghe không dây giá rẻ A Machine Learning Guide for Average Humans https://ift.tt/2rFyAUY xem thêm tại: https://ift.tt/2mb4VST để biết thêm về địa chỉ bán tai nghe không dây giá rẻ A Machine Learning Guide for Average Humans https://ift.tt/2rFyAUY xem thêm tại: https://ift.tt/2mb4VST để biết thêm về địa chỉ bán tai nghe không dây giá rẻ A Machine Learning Guide for Average Humans https://ift.tt/2rFyAUY xem thêm tại: https://ift.tt/2mb4VST để biết thêm về địa chỉ bán tai nghe không dây giá rẻ A Machine Learning Guide for Average Humans https://ift.tt/2rFyAUY xem thêm tại: https://ift.tt/2mb4VST để biết thêm về địa chỉ bán tai nghe không dây giá rẻ A Machine Learning Guide for Average Humans https://ift.tt/2rFyAUY xem thêm tại: https://ift.tt/2mb4VST để biết thêm về địa chỉ bán tai nghe không dây giá rẻ A Machine Learning Guide for Average Humans https://ift.tt/2rFyAUY xem thêm tại: https://ift.tt/2mb4VST để biết thêm về địa chỉ bán tai nghe không dây giá rẻ A Machine Learning Guide for Average Humans https://ift.tt/2rFyAUY xem thêm tại: https://ift.tt/2mb4VST để biết thêm về địa chỉ bán tai nghe không dây giá rẻ A Machine Learning Guide for Average Humans https://ift.tt/2rFyAUY xem thêm tại: https://ift.tt/2mb4VST để biết thêm về địa chỉ bán tai nghe không dây giá rẻ A Machine Learning Guide for Average Humans https://ift.tt/2rFyAUY xem thêm tại: https://ift.tt/2mb4VST để biết thêm về địa chỉ bán tai nghe không dây giá rẻ A Machine Learning Guide for Average Humans https://ift.tt/2rFyAUY xem thêm tại: https://ift.tt/2mb4VST để biết thêm về địa chỉ bán tai nghe không dây giá rẻ A Machine Learning Guide for Average Humans https://ift.tt/2rFyAUY xem thêm tại: https://ift.tt/2mb4VST để biết thêm về địa chỉ bán tai nghe không dây giá rẻ A Machine Learning Guide for Average Humans https://ift.tt/2rFyAUY xem thêm tại: https://ift.tt/2mb4VST để biết thêm về địa chỉ bán tai nghe không dây giá rẻ A Machine Learning Guide for Average Humans https://ift.tt/2rFyAUY xem thêm tại: https://ift.tt/2mb4VST để biết thêm về địa chỉ bán tai nghe không dây giá rẻ A Machine Learning Guide for Average Humans https://ift.tt/2rFyAUY xem thêm tại: https://ift.tt/2mb4VST để biết thêm về địa chỉ bán tai nghe không dây giá rẻ A Machine Learning Guide for Average Humans https://ift.tt/2rFyAUY xem thêm tại: https://ift.tt/2mb4VST để biết thêm về địa chỉ bán tai nghe không dây giá rẻ A Machine Learning Guide for Average Humans https://ift.tt/2rFyAUY xem thêm tại: https://ift.tt/2mb4VST để biết thêm về địa chỉ bán tai nghe không dây giá rẻ A Machine Learning Guide for Average Humans https://ift.tt/2rFyAUY xem thêm tại: https://ift.tt/2mb4VST để biết thêm về địa chỉ bán tai nghe không dây giá rẻ A Machine Learning Guide for Average Humans https://ift.tt/2rFyAUY xem thêm tại: https://ift.tt/2mb4VST để biết thêm về địa chỉ bán tai nghe không dây giá rẻ A Machine Learning Guide for Average Humans https://ift.tt/2rFyAUY xem thêm tại: https://ift.tt/2mb4VST để biết thêm về địa chỉ bán tai nghe không dây giá rẻ A Machine Learning Guide for Average Humans https://ift.tt/2rFyAUY xem thêm tại: https://ift.tt/2mb4VST để biết thêm về địa chỉ bán tai nghe không dây giá rẻ A Machine Learning Guide for Average Humans https://ift.tt/2rFyAUY xem thêm tại: https://ift.tt/2mb4VST để biết thêm về địa chỉ bán tai nghe không dây giá rẻ A Machine Learning Guide for Average Humans https://ift.tt/2rFyAUY xem thêm tại: https://ift.tt/2mb4VST để biết thêm về địa chỉ bán tai nghe không dây giá rẻ A Machine Learning Guide for Average Humans https://ift.tt/2rFyAUY xem thêm tại: https://ift.tt/2mb4VST để biết thêm về địa chỉ bán tai nghe không dây giá rẻ A Machine Learning Guide for Average Humans https://ift.tt/2rFyAUY xem thêm tại: https://ift.tt/2mb4VST để biết thêm về địa chỉ bán tai nghe không dây giá rẻ A Machine Learning Guide for Average Humans https://ift.tt/2rFyAUY xem thêm tại: https://ift.tt/2mb4VST để biết thêm về địa chỉ bán tai nghe không dây giá rẻ A Machine Learning Guide for Average Humans https://ift.tt/2rFyAUY xem thêm tại: https://ift.tt/2mb4VST để biết thêm về địa chỉ bán tai nghe không dây giá rẻ A Machine Learning Guide for Average Humans https://ift.tt/2rFyAUY xem thêm tại: https://ift.tt/2mb4VST để biết thêm về địa chỉ bán tai nghe không dây giá rẻ A Machine Learning Guide for Average Humans https://ift.tt/2rFyAUY xem thêm tại: https://ift.tt/2mb4VST để biết thêm về địa chỉ bán tai nghe không dây giá rẻ A Machine Learning Guide for Average Humans https://ift.tt/2rFyAUY xem thêm tại: https://ift.tt/2mb4VST để biết thêm về địa chỉ bán tai nghe không dây giá rẻ A Machine Learning Guide for Average Humans https://ift.tt/2rFyAUY xem thêm tại: https://ift.tt/2mb4VST để biết thêm về địa chỉ bán tai nghe không dây giá rẻ A Machine Learning Guide for Average Humans https://ift.tt/2rFyAUY xem thêm tại: https://ift.tt/2mb4VST để biết thêm về địa chỉ bán tai nghe không dây giá rẻ A Machine Learning Guide for Average Humans https://ift.tt/2rFyAUY xem thêm tại: https://ift.tt/2mb4VST để biết thêm về địa chỉ bán tai nghe không dây giá rẻ A Machine Learning Guide for Average Humans https://ift.tt/2rFyAUY xem thêm tại: https://ift.tt/2mb4VST để biết thêm về địa chỉ bán tai nghe không dây giá rẻ A Machine Learning Guide for Average Humans https://ift.tt/2rFyAUY xem thêm tại: https://ift.tt/2mb4VST để biết thêm về địa chỉ bán tai nghe không dây giá rẻ A Machine Learning Guide for Average Humans https://ift.tt/2rFyAUY xem thêm tại: https://ift.tt/2mb4VST để biết thêm về địa chỉ bán tai nghe không dây giá rẻ A Machine Learning Guide for Average Humans https://ift.tt/2rFyAUY xem thêm tại: https://ift.tt/2mb4VST để biết thêm về địa chỉ bán tai nghe không dây giá rẻ A Machine Learning Guide for Average Humans https://ift.tt/2rFyAUY xem thêm tại: https://ift.tt/2mb4VST để biết thêm về địa chỉ bán tai nghe không dây giá rẻ A Machine Learning Guide for Average Humans https://ift.tt/2rFyAUY xem thêm tại: https://ift.tt/2mb4VST để biết thêm về địa chỉ bán tai nghe không dây giá rẻ A Machine Learning Guide for Average Humans https://ift.tt/2rFyAUY xem thêm tại: https://ift.tt/2mb4VST để biết thêm về địa chỉ bán tai nghe không dây giá rẻ A Machine Learning Guide for Average Humans https://ift.tt/2rFyAUY xem thêm tại: https://ift.tt/2mb4VST để biết thêm về địa chỉ bán tai nghe không dây giá rẻ A Machine Learning Guide for Average Humans https://ift.tt/2rFyAUY xem thêm tại: https://ift.tt/2mb4VST để biết thêm về địa chỉ bán tai nghe không dây giá rẻ A Machine Learning Guide for Average Humans https://ift.tt/2rFyAUY xem thêm tại: https://ift.tt/2mb4VST để biết thêm về địa chỉ bán tai nghe không dây giá rẻ A Machine Learning Guide for Average Humans https://ift.tt/2rFyAUY xem thêm tại: https://ift.tt/2mb4VST để biết thêm về địa chỉ bán tai nghe không dây giá rẻ A Machine Learning Guide for Average Humans https://ift.tt/2rFyAUY Bạn có thể xem thêm địa chỉ mua tai nghe không dây tại đây https://ift.tt/2mb4VST
0 notes
Text
A Machine Learning Guide for Average Humans
A Machine Learning Guide for Average Humans
Posted by alexis-sanders
//<![CDATA[ (function($) { // code using $ as alias to jQuery $(function() { // Hide the hypotext content. $('.hypotext-content').hide(); // When a hypotext link is clicked. $('a.hypotext.closed').click(function (e) { // custom handling here e.preventDefault(); // Create the class reference from the rel value. var id = '.' + $(this).attr('rel'); // If the content is hidden, show it now. if ( $(id).css('display') == 'none' ) { $(id).show('slow'); if (jQuery.ui) { // UI loaded $(id).effect("highlight", {}, 1000); } } // If the content is shown, hide it now. else { $(id).hide('slow'); } }); // If we have a hash value in the url. if (window.location.hash) { // If the anchor is within a hypotext block, expand it, by clicking the // relevant link. console.log(window.location.hash); var anchor = $(window.location.hash); var hypotextLink = $('#' + anchor.parents('.hypotext-content').attr('rel')); console.log(hypotextLink); hypotextLink.click(); // Wait until the content has expanded before jumping to anchor. //$.delay(1000); setTimeout(function(){ scrollToAnchor(window.location.hash); }, 1000); } }); function scrollToAnchor(id) { var anchor = $(id); $('html,body').animate({scrollTop: anchor.offset().top},'slow'); } })(jQuery); //]]>
Machine learning (ML) has grown consistently in worldwide prevalence. Its implications have stretched from small, seemingly inconsequential victories to groundbreaking discoveries. The SEO community is no exception. An understanding and intuition of machine learning can support our understanding of the challenges and solutions Google's engineers are facing, while also opening our minds to ML's broader implications.
The advantages of gaining an general understanding of machine learning include:
Gaining empathy for engineers, who are ultimately trying to establish the best results for users
Understanding what problems machines are solving for, their current capabilities and scientists' goals
Understanding the competitive ecosystem and how companies are using machine learning to drive results
Preparing oneself for for what many industry leaders call a major shift in our society (Andrew Ng refers to AI as a "new electricity")
Understanding basic concepts that often appear within research (it's helped me with understanding certain concepts that appear within Google Brain's Research)
Growing as an individual and expanding your horizons (you might really enjoy machine learning!)
When code works and data is produced, it's a very fulfilling, empowering feeling (even if it's a very humble result)
I spent a year taking online courses, reading books, and learning about learning (...as a machine). This post is the fruit borne of that labor -- it covers 17 machine learning resources (including online courses, books, guides, conference presentations, etc.) comprising the most affordable and popular machine learning resources on the web (through the lens of a complete beginner). I've also added a summary of "If I were to start over again, how I would approach it."
This article isn't about credit or degrees. It's about regular Joes and Joannas with an interest in machine learning, and who want to spend their learning time efficiently. Most of these resources will consume over 50 hours of commitment. Ain't nobody got time for a painful waste of a work week (especially when this is probably completed during your personal time). The goal here is for you to find the resource that best suits your learning style. I genuinely hope you find this research useful, and I encourage comments on which materials prove most helpful (especially ones not included)! #HumanLearningMachineLearning
Executive summary:
Here's everything you need to know in a chart:
Machine Learning Resource
Time (hours)
Cost ($)
Year
Credibility
Code
Math
Enjoyability
Jason Maye's Machine Learning 101 slidedeck: 2 years of headbanging, so you don't have to
2
$0
'17
{ML} Recipes with Josh Gordon Playlist
2
$0
'16
Machine Learning Crash Course
15
$0
'18
OCDevel Machine Learning Guide Podcast
30
$0
'17-
Kaggle's Machine Learning Track (part 1)
6
$0
'17
Fast.ai (part 1)
70
$70*
'16
Hands-On Machine Learning with Scikit-Learn and TensorFlow: Concepts, Tools, and Techniques to Build Intelligent Systems
20
$25
'17
Udacity's Intro to Machine Learning (Kate/Sebastian)
60
$0
'15
Andrew Ng's Coursera Machine Learning
55
$0
'11
iPullRank Machine Learning Guide
3
$0
'17
Review Google PhD
2
$0
'17
Caltech Machine Learning on iTunes
27
$0
'12
Pattern Recognition & Machine Learning by Christopher Bishop
150
$75
'06
N/A
Machine Learning: Hands-on for Developers and Technical Professionals
15
$50
'15
Introduction to Machine Learning with Python: A Guide for Data Scientists
15
$25
'16
Udacity's Machine Learning by Georgia Tech
96
$0
'15
Machine Learning Stanford iTunes by Andrew Ng
25
$0
'08
N/A
*Free, but there is the cost of running an AWS EC2 instance (~$70 when I finished, but I did tinker a ton and made a Rick and Morty script generator, which I ran many epochs [rounds] of...)
Here's my suggested program:
1. Starting out (estimated 60 hours)
Start with shorter content targeting beginners. This will allow you to get the gist of what's going on with minimal time commitment.
Commit three hours to Jason Maye's Machine Learning 101 slidedeck: 2 years of headbanging, so you don't have to.
Commit two hours to watch Google's {ML} Recipes with Josh Gordon YouTube Playlist.
Sign up for Sam DeBrule's Machine Learnings newsletter.
Work through Google's Machine Learning Crash Course.
Start listening to OCDevel's Machine Learning Guide Podcast (skip episodes 1, 3, 16, 21, and 26) in your car, working out, and/or when using hands and eyes for other activities.
Commit two days to working through Kaggle's Machine Learning Track part 1.
2. Ready to commit (estimated 80 hours)
By this point, learners would understand their interest levels. Continue with content focused on applying relevant knowledge as fast as possible.
Commit to Fast.ai 10 hours per week, for 7 weeks. If you have a friend/mentor that can help you work through AWS setup, definitely lean on any support in installation (it's 100% the worst part of ML).
Acquire Hands-On Machine Learning with Scikit-Learn and TensorFlow: Concepts, Tools, and Techniques to Build Intelligent Systems, and read the first two chapters immediately. Then use this as supplemental to the Fast.ai course.
3. Broadening your horizons (estimated 115 hours)
If you've made it through the last section and are still hungry for more knowledge, move on to broadening your horizons. Read content focused on teaching the breadth of machine learning -- building an intuition for what the algorithms are trying to accomplish (whether visual or mathematically).
Start watching videos and participating in Udacity's Intro to Machine Learning (by Sebastian Thrun and Katie Malone).
Work through Andrew Ng's Coursera Machine Learning course.
Your next steps
By this point, you will already have AWS running instances, a mathematical foundation, and an overarching view of machine learning. This is your jumping-off point to determine what you want to do.
You should be able to determine your next step based on your interest, whether it's entering Kaggle competitions; doing Fast.ai part two; diving deep into the mathematics with Pattern Recognition & Machine Learning by Christopher Bishop; giving Andrew Ng's newer Deeplearning.ai course on Coursera; learning more about specific tech stacks (TensorFlow, Scikit-Learn, Keras, Pandas, Numpy, etc.); or applying machine learning to your own problems.
Why am I recommending these steps and resources?
I am not qualified to write an article on machine learning. I don't have a PhD. I took one statistics class in college, which marked the first moment I truly understood "fight or flight" reactions. And to top it off, my coding skills are lackluster (at their best, they're chunks of reverse-engineered code from Stack Overflow). Despite my many shortcomings, this piece had to be written by someone like me, an average person.
Statistically speaking, most of us are average (ah, the bell curve/Gaussian distribution always catches up to us). Since I'm not tied to any elitist sentiments, I can be real with you. Below contains a high-level summary of my reviews on all of the classes I took, along with a plan for how I would approach learning machine learning if I could start over. Click to expand each course for the full version with notes.
In-depth reviews of machine learning courses:
Starting out
Jason Maye's Machine Learning 101 slidedeck: 2 years of head-banging, so you don't have to ↓
Need to Know: A stellar high-level overview of machine learning fundamentals in an engaging and visually stimulating format.
Loved:
Very user-friendly, engaging, and playful slidedeck.
Has the potential to take some of the pain out of the process, through introducing core concepts.
Breaks up content by beginner/need-to-know (green), and intermediate/less-useful noise (specifically for individuals starting out) (blue).
Provides resources to dive deeper into machine learning.
Provides some top people to follow in machine learning.
Disliked:
That there is not more! Jason's creativity, visual-based teaching approach, and quirky sense of humor all support the absorption of the material.
Lecturer:
Jason Mayes:
Senior Creative Technologist and Research Engineer at Google
Masters in Computer Science from University of Bristols
Personal Note: He's also kind on Twitter! :)
Links:
Machine Learning 101 slide deck
Tips on Watching:
Set aside 2-4 hours to work through the deck once.
Since there is a wealth of knowledge, refer back as needed (or as a grounding source).
Identify areas of interest and explore the resources provided.
{ML} Recipes with Josh Gordon ↓
Need to Know: This mini-series YouTube-hosted playlist covers the very fundamentals of machine learning with opportunities to complete exercises.
Loved:
It is genuinely beginner-focused.
They make no assumption of any prior knowledge.
Gloss over potentially complex topics that may serve as noise.
Playlist ~2 hours
Very high-quality filming, audio, and presentation, almost to the point where it had its own aesthetic.
Covers some examples in scikit-learn and TensorFlow, which felt modern and practical.
Josh Gordon was an engaging speaker.
Disliked:
I could not get Dockers on Windows (suggested package manager). This wasn't a huge deal, since I already had my AWS setup by this point; however, a bit of a bummer since it made it impossible to follow certain steps exactly.
Issue: Every time I tried to download (over the course of two weeks), the .exe file would recursively start and keep spinning until either my memory ran out, computer crashed, or I shut my computer down. I sent this to Docker's Twitter account to no avail.
Lecturer:
Josh Gordon:
Developer Advocate for at TensorFlow at Google
Leads Machine Learning advocacy at Google
Member of the Udacity AI & Data Industry Advisory Board
Masters in Computer Science from Columbia University
Links:
Hello World - Machine Learning Recipes #1 (YouTube)
GitHub: Machine Learning Recipes with Josh Gordon
Tips on Watching:
The playlist is short (only ~1.5 hours screen time). However, it can be a bit fast-paced at times (especially if you like mimicking the examples), so set aside 3-4 hours to play around with examples and allow time for installation, pausing, and following along.
Take time to explore code labs.
Google's Machine Learning Crash Course with TensorFlow APIs ↓
Need to Know: A Google researcher-made crash course on machine learning that is interactive and offers its own built-in coding system!
Loved:
Different formats of learning: high-quality video (with ability to adjust speed, closed captioning), readings, quizzes (with explanations), visuals (including whiteboarding), interactive components/ playgrounds, code lab exercises (run directly in your browser (no setup required!))
Non-intimidating
One of my favorite quotes: "You don't need to understand the math to be able to take a look at the graphical interpretation."
Broken down into digestible sections
Introduces key terms
Disliked:
N/A
Lecturers:
Multiple Google researchers participated in this course, including:
Peter Norvig
Director of Research at Google Inc.
Previously he directed Google's core search algorithms group.
He is co-author of Artificial Intelligence: A Modern Approach
D. Sculley
Senior Staff Software Engineer at Google
KDD award-winning papers
Works on massive-scale ML systems for online advertising
Was part of a research ML paper on optimizing chocolate chip cookies
According to his personal website, he prefers to go by "D."
Cassandra Xia
Programmer, Software Engineer at Google
She has some really cool (and cute) projects based on learning statistics concepts interactively
Maya Gupta
Leads Glassbox Machine Learning R&D team at Google
Associate Professor of Electrical Engineering at the University of Washington (2003-2012)
In 2007, Gupta received the PECASE award from President George Bush for her work in classifying uncertain (e.g. random) signals
Gupta also runs Artifact Puzzles, the second-largest US maker of wooden jigsaw puzzles
Sally Goldman
Research Scientist at Google
Co-author of A Practical Guide to Data Structures and Algorithms Using Java
Numerous journals, classes taught at Washington University, and contributions to the ML community
Links:
Machine Learning Crash Course
Tips on Doing:
Actively work through playground and coding exercises
OCDevel's Machine Learning Guide Podcast ↓
Need to Know: This podcast focuses on the high-level fundamentals of machine learning, including basic intuition, algorithms, math, languages, and frameworks. It also includes references to learn more on each episode's topic.
Loved:
Great for trips (when traveling a ton, it was an easy listen).
The podcast makes machine learning fun with interesting and compelling analogies.
Tyler is a big fan of Andrew Ng's Coursera course and reviews concepts in Coursera course very well, such that both pair together nicely.
Covers the canonical resources for learning more on a particular topic.
Disliked:
Certain courses were more theory-based; all are interesting, yet impractical.
Due to limited funding the project is a bit slow to update and has less than 30 episodes.
Podcaster:
Tyler Renelle:
Machine learning engineer focused on time series and reinforcement
Background in full-stack JavaScript, 10 years web and mobile
Creator of HabitRPG, an app that treats habits as an RPG game
Links:
Machine Learning Guide podcast
Machine Learning Guide podcast (iTunes)
Tips on Listening:
Listen along your journey to help solidify understanding of topics.
Skip episodes 1, 3, 16, 21, and 26 (unless their topics interest and inspire you!).
Kaggle Machine Learning Track (Lesson 1) ↓
Need to Know: A simple code lab that covers the very basics of machine learning with scikit-learn and Panda through the application of the examples onto another set of data.
Loved:
A more active form of learning.
An engaging code lab that encourages participants to apply knowledge.
This track offers has a built-in Python notebook on Kaggle with all input files included. This removed any and all setup/installation issues.
Side note: It's a bit different than Jupyter notebook (e.g., have to click into a cell to add another cell).
Each lesson is short, which made the entire lesson go by very fast.
Disliked:
The writing in the first lesson didn't initially make it clear that one would need to apply the knowledge in the lesson to their workbook.
It wasn't a big deal, but when I started referencing files in the lesson, I had to dive into the files in my workbook to find they didn't exist, only to realize that the knowledge was supposed to be applied and not transcribed.
Lecturer:
Dan Becker:
Data Scientist at Kaggle
Undergrad in Computer Science, PhD in Econometrics
Supervised data science consultant for six Fortune 100 companies
Contributed to the Keras and Tensorflow libraries
Finished 2nd (out of 1353 teams) in $3 million Heritage Health Prize data mining competition
Speaks at deep learning workshops at events and conferences
Links:
https://www.kaggle.com/learn/machine-learning
Tips on Doing:
Read the exercises and apply to your dataset as you go.
Try lesson 2, which covers more complex/abstract topics (note: this second took a bit longer to work through).
Ready to commit
Fast.ai (part 1 of 2) ↓
Need to Know: Hands-down the most engaging and active form of learning ML. The source I would most recommend for anyone (although the training plan does help to build up to this course). This course is about learning through coding. This is the only course that I started to truly see the practical mechanics start to come together. It involves applying the most practical solutions to the most common problems (while also building an intuition for those solutions).
Loved:
Course Philosophy:
Active learning approach
"Go out into the world and understand underlying mechanics (of machine learning by doing)."
Counter-culture to the exclusivity of the machine learning field, focusing on inclusion.
"Let's do shit that matters to people as quickly as possible."
Highly pragmatic approach with tools that are currently being used (Jupyter Notebooks, scikit-learn, Keras, AWS, etc.).
Show an end-to-end process that you get to complete and play with in a development environment.
Math is involved, but is not prohibitive. Excel files helped to consolidate information/interact with information in a different way, and Jeremy spends a lot of time recapping confusing concepts.
Amazing set of learning resources that allow for all different styles of learning, including:
Video Lessons
Notes
Jupyter Notebooks
Assignments
Highly active forums
Resources on Stackoverflow
Readings/resources
Jeremy often references popular academic texts
Jeremy's TEDx talk in Brussels
Jeremy really pushes one to do extra and put in the effort by teaching interesting problems and engaging one in solving them.
It's a huge time commitment; however, it's worth it.
All of the course's profits are donated.
Disliked:
Overview covers their approach to learning (obviously I'm a fan!). If you're already drinking the Kool-aid, skip past.
I struggled through the AWS setup (13-minute video) for about five hours (however, it felt so good when it was up and running!).
Because of its practicality and concentration on solutions used today to solve popular problem types (image recognition, text generation, etc.), it lacks breadth of machine learning topics.
Lecturers:
Jeremy Howard:
Distinguished Research Scientist at the University of San Francisco
Faculty member at Singularity University
Young Global Leader with the World Economic Forum
Founder of Enlitic (the first company to apply deep learning to medicine)
Former President and Chief Scientist of the data science platform Kaggle
Rachel Thomas:
PhD in Math from Duke
One of Forbes' "20 Incredible Women Advancing AI Research"
Researcher-in-residence at the University of San Francisco Data Institute
Teaches in the Masters in Data Science program
Links:
http://course.fast.ai/start.html
http://wiki.fast.ai/index.php/Main_Page
https://github.com/fastai/courses/tree/master/deeplearning1/nbs
Tips on Doing:
Set expectations with yourself that installation is going to probably take a few hours.
Prepare to spend about ~70 hours for this course (it's worth it).
Don't forget to shut off your AWS instance.
Balance out machine learning knowledge with a course with more breadth.
Consider giving part two of the Fast.ai program a shot!
Hands-On Machine Learning with Scikit-Learn and TensorFlow: Concepts, Tools, and Techniques to Build Intelligent Systems ↓
Need to Know: This book is an Amazon best seller for a reason. It covers a lot of ground quickly, empowers readers to walk through a machine learning problem by chapter two, and contains practical up-to-date machine learning skills.
Loved:
Book contains an amazing introduction to machine learning that briskly provides an overarching quick view of the machine learning ecosystem.
Chapter 2 immediately walks the reader through an end-to-end machine learning problem.
Immediately afterwards, Aurélien pushes a user to attempt to apply this solution to another problem, which was very empowering.
There are review questions at the end of each chapter to ensure on has grasped the content within the chapter and to push the reader to explore more.
Once installation was completed, it was easy to follow and all code is available on GitHub.
Chapters 11-14 were very tough reading; however, they were a great reference when working through Fast.ai.
Contains some powerful analogies.
Each chapter's introductions were very useful and put everything into context. This general-to-specifics learning was very useful.
Disliked:
Installation was a common source of issues during the beginning of my journey; the text glided over this. I felt the frustration that most people experience from installation should have been addressed with more resources.
Writer:
Aurélien Géron:
Led the YouTube video classification team from 2013 to 2016
Currently a machine Learning consultant
Founder and CTO of Wifirst and Polyconseil
Published technical books (on C++, Wi-Fi, and Internet architectures)
Links:
https://www.amazon.com/_/dp/1491962291?tag=oreilly20-20
http://shop.oreilly.com/product/0636920052289.do
https://github.com/ageron/handson-ml
Tips on Using:
Get a friend with Python experience to help with installation.
Read the introductions to each chapter thoroughly, read the chapter (pay careful attention to code), review the questions at the end (highlight any in-text answer), make a copy of Aurélien's GitHub and make sure everything works on your setup, re-type the notebooks, go to Kaggle and try on other datasets.
Broadening your horizons
Udacity: Intro to Machine Learning (Kate/Sebastian) ↓
Need to Know: A course that covers a range of machine learning topics, supports building of intuition via visualization and simple examples, offers coding challenges, and a certificate (upon completion of a final project). The biggest challenge with this course is bridging the gap between the hand-holding lectures and the coding exercises.
Loved:
Focus on developing a visual intuition on what each model is trying to accomplish.
This visual learning mathematics approach is very useful.
Cover a vast variety and breadth of models and machine learning basics.
In terms of presenting the concept, there was a lot of hand-holding (which I completely appreciated!).
Many people have done this training, so their GitHub accounts can be used as reference for the mini-projects.
Katie actively notes documentation and suggests where viewers can learn more/reference material.
Disliked:
All of the conceptual hand-holding in the lessons is a stark contrast to the challenges of installation, coding exercises, and mini-projects.
This is the first course started and the limited instructions on setting up the environment and many failed attempts caused me to break down crying at least a handful of times.
The mini-projects are intimidating.
There is extra code added to support the viewers; however, it's done so with little acknowledgement as to what it's actually doing. This made learning a bit harder.
Lecturer:
Caitlin (Katie) Malone:
Director of Data Science Research and Development at Civis Analytics
Stanford PhD in Experimental Particle Physics
Intern at Udacity in summer 2014
Graduate Researcher at the SLAC National Accelerator Laboratory
https://www6.slac.stanford.edu/
Podcaster with Ben Jaffe (currently Facebook UI Engineer and a music aficionado) on a machine learning podcast Linear Digressions (100+ episodes)
Sebastian Thrun:
CEO of the Kitty Hawk Corporation
Chairman and co-founder of Udacity
One of my favorite Sebastian quotes: "It occurred to me, I could be at Google and build a self-driving car, or I can teach 10,000 students how to build self-driving cars."
Former Google VP
Founded Google X
Led development of the robotic vehicle Stanley
Professor of Computer Science at Stanford University
Formerly a professor at Carnegie Mellon University.
Links:
https://www.udacity.com/course/intro-to-machine-learning--ud120
Udacity also offers a next step, the Machine Learning Engineer Nanodegree, which will set one back about $1K.
Tips on Watching:
Get a friend to help you set up your environment.
Print mini-project instructions to check off each step.
Andrew Ng's Coursera Machine Learning Course ↓
Need to Know: The Andrew Ng Coursera course is the most referenced online machine learning course. It covers a broad set of fundamental, evergreen topics with a strong focus in building mathematical intuition behind machine learning models. Also, one can submit assignments and earn a grade for free. If you want to earn a certificate, one can subscribe or apply for financial aid.
Loved:
This course has a high level of credibility.
Introduces all necessary machine learning terminology and jargon.
Contains a very classic machine learning education approach with a high level of math focus.
Quizzes interspersed in courses and after each lesson support understanding and overall learning.
The sessions for the course are flexible, the option to switch into a different section is always available.
Disliked:
The mathematic notation was hard to process at times.
The content felt a bit dated and non-pragmatic. For example, the main concentration was MATLAB and Octave versus more modern languages and resources.
Video quality was less than average and could use a refresh.
Lecturer:
Andrew Ng:
Adjunct Professor, Stanford University (focusing on AI, Machine Learning, and Deep Learning)
Co-founder of Coursera
Former head of Baidu AI Group
Founder and previous head of Google Brain (deep learning) project
Former Director of the Stanford AI Lab
Chairman of the board of Woebot (a machine learning bot that focuses on Cognitive Behavior Therapy)
Links:
https://www.coursera.org/learn/machine-learning/
Andrew Ng recently launched a new course (August 2017) called DeepLearning.ai, a ~15 week course containing five mini-courses ($49 USD per month to continue learning after trial period of 7 days ends).
Course: https://www.coursera.org/specializations/deep-learning
Course 1: Neural Networks and Deep Learning
Course 2: Improving Deep Neural Networks: Hyperparameter tuning, Regularization and Optimization
Course 3: Structuring Machine Learning Projects
Course 4: Convolutional Neural Networks
Course 5: Sequence Models
Tips on Watching:
Be disciplined with setting aside timing (even if it's only 15 minutes a day) to help power through some of the more boring concepts.
Don't do this course first, because it's intimidating, requires a large time commitment, and isn't a very energizing experience.
Additional machine learning opportunities
iPullRank Machine Learning Guide ↓
Need to Know: A machine learning e-book targeted at marketers.
Loved:
Targeted at marketers and applied to organic search.
Covers a variety of machine learning topics.
Some good examples, including real-world blunders.
Gives some practical tools for non-data scientists (including: MonkeyLearn and Orange)
I found Orange to be a lot of fun. It struggled with larger datasets; however, it has a very visual interface that was more user-friendly and offers potential to show some pretty compelling stories.
Example: World Happiness Dataset by:
X-axis: Happiness Score
Y-axis: Economy
Color: Health
Disliked:
Potential to break up content more with relevant imagery -- the content was very dense.
Writers:
iPullRank Team (including Mike King):
Mike King has a few slide decks on the basics of machine learnings and AI
iPullRank has a few data scientists on staff
Links:
http://ipullrank.com/machine-learning-guide/
Tips on Reading:
Read chapters 1-6 and the rest depending upon personal interest.
Review Google PhD ↓
Need to Know: A two-hour presentation from Google's 2017 IO conference that walks through getting 99% accuracy on the MNIST dataset (a famous dataset containing a bunch of handwritten numbers, which the machine must learn to identify the numbers).
Loved:
This talk struck me as very modern, covering the cutting edge.
Found this to be very complementary to Fast.ai, as it covered similar topics (e.g. ReLu, CNNs, RNNs, etc.)
Amazing visuals that help to put everything into context.
Disliked:
The presentation is only a short conference solution and not a comprehensive view of machine learning.
Also, a passive form of learning.
Presenter:
Martin Görner:
Developer Relations, Google (since 2011)
Started Mobipocket, a startup that later became the software part of the Amazon Kindle and its mobile variants
Links:
Part 1 - https://www.youtube.com/watch?v=u4alGiomYP4
Part 2 - https://www.youtube.com/watch?v=fTUwdXUFfI8
Tips on Watching:
Google any concepts you're unfamiliar with.
Take your time with this one; 2 hours of screen time doesn't count all of the Googling and processing time for this one.
Caltech Machine Learning iTunes ↓
Need to Know: If math is your thing, this course does a stellar job of building the mathematic intuition behind many machine learning models. Dr. Abu-Mostafa is a raconteur, includes useful visualizations, relevant real-world examples, and compelling analogies.
Loved:
First and foremost, this is a real Caltech course, meaning it's not a watered-down version and contains fundamental concepts that are vital to understanding the mechanics of machine learning.
On iTunes, audio downloads are available, which can be useful for on-the-go learning.
Dr. Abu-Mostafa is a skilled speaker, making the 27 hours spent listening much easier!
Dr. Abu-Mostafa offers up some strong real-world examples and analogies which makes the content more relatable.
As an example, he asks students: "Why do I give you practice exams and not just give you the final exam?" as an illustration of why a testing set is useful. If he were to just give students the final, they would just memorize the answers (i.e., they would overfit to the data) and not genuinely learn the material. The final is a test to show how much students learn.
The last 1/2 hour of the class is always a Q&A, where students can ask questions. Their questions were useful to understanding the topic more in-depth.
The video and audio quality was strong throughout. There were a few times when I couldn't understand a question in the Q&A, but overall very strong.
This course is designed to build mathematical intuition of what's going on under the hood of specific machine learning models.
Caution: Dr. Abu-Mostafa uses mathematical notation, but it's different from Andrew Ng's (e.g., theta = w).
The final lecture was the most useful, as it pulled a lot of the conceptual puzzle pieces together. The course on neural networks was a close second!
Disliked:
Although it contains mostly evergreen content, being released in 2012, it could use a refresh.
Very passive form of learning, as it wasn't immediately actionable.
Lecturer:
Dr. Yaser S. Abu-Mostafa:
Professor of Electrical Engineering and Computer Science at the California Institute of Technology
Chairman of Machine Learning Consultants LLC
Serves on a number of scientific advisory boards
Has served as a technical consultant on machine learning for several companies (including Citibank).
Multiple articles in Scientific American
Links:
https://work.caltech.edu/telecourse.html
https://itunes.apple.com/us/course/machine-learning/id515364596
Tips on Watching:
Consider listening to the last lesson first, as it pulls together the course overall conceptually. The map of the course, below, was particularly useful to organizing the information taught in the courses.
Image source: http://work.caltech.edu/slides/slides18.pdf
"Pattern Recognition & Machine Learning" by Christopher Bishop ↓
Need to Know: This is a very popular college-level machine learning textbook. I've heard it likened to a bible for machine learning. However, after spending a month trying to tackle the first few chapters, I gave up. It was too much math and pre-requisites to tackle (even with a multitude of Google sessions).
Loved:
The text of choice for many major universities, so if you can make it through this text and understand all of the concepts, you're probably in a very good position.
I appreciated the history aside sections, where Bishop talked about influential people and their career accomplishments in statistics and machine learning.
Despite being a highly mathematically text, the textbook actually has some pretty visually intuitive imagery.
Disliked:
I couldn't make it through the text, which was a bit frustrating. The statistics and mathematical notation (which is probably very benign for a student in this topic) were too much for me.
The sunk cost was pretty high here (~$75).
Writer:
Christopher Bishop:
Laboratory Director at Microsoft Research Cambridge
Professor of Computer Science at the University of Edinburgh
Fellow of Darwin College, Cambridge
PhD in Theoretical Physics from the University of Edinburgh
Links:
https://www.amazon.com/Pattern-Recognition-Learning-Information-Statistics/dp/0387310738/ref=sr_1_2?ie=UTF8&qid=1516839475&sr=8-2&keywords=Pattern+Recognition+%26+Machine+Learning
Tips on Reading:
Don't start your machine learning journey with this book.
Get a friend in statistics to walk you through anything complicated (my plan is to get a mentor in statistics).
Consider taking a (free) online statistics course (Khan Academy and Udacity both have some great content on statistics, calculus, math, and data analysis).
Machine Learning: Hands-on for Developers and Technical Professionals ↓
Need to Know: A fun, non-intimidating end-to-end launching pad/whistle stop for machine learning in action.
Loved:
Talks about practical issues that many other sources didn't really address (e.g. data-cleansing).
Covered the basics of machine learning in a non-intimidating way.
Offers abridged, consolidated versions of the content.
Added fun anecdotes that makes it easier to read.
Overall the writer has a great sense of humor.
Writer talks to the reader as if they're a real human being (i.e., doesn't expect you to go out and do proofs; acknowledges the challenge of certain concepts).
Covers a wide variety of topics.
Because it was well-written, I flew through the book (even though it's about ~300 pages).
Disliked:
N/A
Writer:
Jason Bell:
Technical architect, lecturer, and startup consultant
Data Engineer at MastodonC
Former section editor for Java Developer's Journal
Former writer on IBM DeveloperWorks
Links:
https://www.amazon.com/Machine-Learning-Hands-Developers-Professionals/dp/1118889061
https://www.wiley.com/en-us/Machine+Learning%3A+Hands+On+for+Developers+and+Technical+Professionals-p-9781118889060
Jason's Blog: https://dataissexy.wordpress.com/
Tips on Reading:
Download and explore Weka's interface beforehand.
Give some of the exercises a shot.
Introduction to Machine Learning with Python: A Guide for Data Scientists ↓
Need to Know: This was a was a well-written piece on machine learning, making it a quick read.
Loved:
Quick, smooth read.
Easy-to-follow code examples.
The first few chapters served as a stellar introduction to the basics of machine learning.
Contain subtle jokes that add a bit of fun.
Tip to use the Python package manager Anaconda with Jupyter Notebooks was helpful.
Disliked:
Once again, installation was a challenge.
The "mglearn" utility library threw me for a loop. I had to reread the first few chapters before I figured out it was support for the book.
Although I liked the book, I didn't love it. Overall it just missed the "empowering" mark.
Writers:
Andreas C. Müller:
PhD in Computer Science
Lecturer at the Data Science Institute at Columbia University
Worked at the NYU Center for Data Science on open source and open science
Former Machine Learning Scientist at Amazon
Speaks often on Machine Learning and scikit-learn (a popular machine learning library)
And he makes some pretty incredibly useful graphics, such as this scikit-learn cheat sheet:
Image source: http://peekaboo-vision.blogspot.com/2013/01/machin...
Sarah Guido:
Former senior data scientist at Mashable
Lead data scientist at Bitly
2018 SciPy Conference Data Science track co-chair
Links:
https://www.amazon.com/Introduction-Machine-Learning-Python-Scientists/dp/1449369413/ref=sr_1_7?s=books&ie=UTF8&qid=1516734322&sr=1-7&keywords=python+machine+learning
http://shop.oreilly.com/product/0636920030515.do
Tips on Reading:
Type out code examples.
Beware of the "mglearn" utility library.
Udacity: Machine Learning by Georgia Tech ↓
Need to Know: A mix between an online learning experience and a university machine learning teaching approach. The lecturers are fun, but the course still fell a bit short in terms of active learning.
Loved:
This class is offered as CS7641 at Georgia Tech, where it is a part of the Online Masters Degree. Although taking this course here will not earn credit towards the OMS degree, it's still a non-watered-down college teaching philosophy approach.
Covers a wide variety of topics, many of which reminded me of the Caltech course (including: VC Dimension versus Bayesian, Occam's razor, etc.)
Discusses Markov Decision Chains, which is something that didn't really come up in many other introductory machine learning course, but they are referenced within Google patents.
The lecturers have a great dynamic, are wicked smart, and displayed a great sense of (nerd) humor, which make the topics less intimidating.
The course has quizzes, which give the course a slight amount of interaction.
Disliked:
Some videos were very long, which made the content a bit harder to digest.
The course overall was very time consuming.
Despite the quizzes, the course was a very passive form of learning with no assignments and little coding.
Many videos started with a bunch of content already written out. Having the content written out was probably a big time-saver, but it was also a bit jarring for a viewer to see so much information all at once, while also trying to listen.
It's vital to pay very close attention to notation, which compounds in complexity quickly.
Tablet version didn't function flawlessly: some was missing content (which I had to mark down and review on a desktop), the app would crash randomly on the tablet, and sometimes the audio wouldn't start.
There were no subtitles available on tablet, which I found not only to be a major accessibility blunder, but also made it harder for me to process (since I'm not an audio learner).
Lecturer:
Michael Littman:
Professor of Computer Science at Brown University.
Was granted a patent for one of the earliest systems for Cross-language information retrieval
Perhaps the most interesting man in the world:
Been in two TEDx talks
How I Learned to Stop Worrying and Be Realistic About AI
A Cooperative Path to Artificial Intelligence
During his time at Duke, he worked on an automated crossword solver (PROVERB)
Has a Family Quartet
He has appeared in a TurboTax commercial
Charles Isbell:
Professor and Executive Associate Dean at School of Interactive Computing at Georgia Tech
Focus on statistical machine learning and "interactive" artificial intelligence.
Links:
https://www.udacity.com/course/machine-learning--ud262
Tips on Watching:
Pick specific topics of interest and focusing on those lessons.
Andrew Ng's Stanford's Machine Learning iTunes ↓
Need to Know: A non-watered-down Stanford course. It's outdated (filmed in 2008), video/audio are a bit poor, and most links online now point towards the Coursera course. Although the idea of watching a Stanford course was energizing for the first few courses, it became dreadfully boring. I made it to course six before calling it.
Loved:
Designed for students, so you know you're not missing out on anything.
This course provides a deeper study into the mathematical and theoretical foundation behind machine learning to the point that the students could create their own machine learning algorithms. This isn't necessarily very practical for the everyday machine learning user.
Has some powerful real-world examples (although they're outdated).
There is something about the kinesthetic nature of watching someone write information out. The blackboard writing helped me to process certain ideas.
Disliked:
Video and audio quality were pain to watch.
Many questions asked by students were hard to hear.
On-screen visuals range from hard to impossible to see.
Found myself counting minutes.
Dr. Ng mentions TA classes, supplementary learning, but these are not available online.
Sometimes the video showed students, which I felt was invasive.
Lecturer:
Andrew Ng (see above)
Links:
https://itunes.apple.com/us/course/machine-learning/id495053006
https://www.youtube.com/watch?v=UzxYlbK2c7E
Tips on Watching:
Only watch if you're looking to gain a deeper understanding of the math presented in the Coursera course.
Skip the first half of the first lecture, since it's mostly class logistics.
Additional Resources
Fast.ai (part 2) - free access to materials, cost for AWS EC2 instance
Deeplearning.ai - $50/month
Udacity Machine Learning Engineer Nanodegree - $1K
https://machinelearningmastery.com/
Motivations and inspiration
If you're wondering why I spent a year doing this, then I'm with you. I'm genuinely not sure why I set my sights on this project, much less why I followed through with it. I saw Mike King give a session on Machine Learning. I was caught off guard, since I knew nothing on the topic. It gave me a pesky, insatiable curiosity itch. It started with one course and then spiraled out of control. Eventually it transformed into an idea: a review guide on the most affordable and popular machine learning resources on the web (through the lens of a complete beginner). Hopefully you found it useful, or at least somewhat interesting. Be sure to share your thoughts or questions in the comments!
Sign up for The Moz Top 10, a semimonthly mailer updating you on the top ten hottest pieces of SEO news, tips, and rad links uncovered by the Moz team. Think of it as your exclusive digest of stuff you don't have time to hunt down but want to read!
xem them tai https://ift.tt/2o9GYfe A Machine Learning Guide for Average Humans xem thêm tại: https://ift.tt/2mb4VST để biết thêm về địa chỉ bán tai nghe không dây giá rẻ A Machine Learning Guide for Average Humans https://ift.tt/2rFyAUY xem thêm tại: https://ift.tt/2mb4VST để biết thêm về địa chỉ bán tai nghe không dây giá rẻ A Machine Learning Guide for Average Humans https://ift.tt/2rFyAUY xem thêm tại: https://ift.tt/2mb4VST để biết thêm về địa chỉ bán tai nghe không dây giá rẻ A Machine Learning Guide for Average Humans https://ift.tt/2rFyAUY xem thêm tại: https://ift.tt/2mb4VST để biết thêm về địa chỉ bán tai nghe không dây giá rẻ A Machine Learning Guide for Average Humans https://ift.tt/2rFyAUY xem thêm tại: https://ift.tt/2mb4VST để biết thêm về địa chỉ bán tai nghe không dây giá rẻ A Machine Learning Guide for Average Humans https://ift.tt/2rFyAUY xem thêm tại: https://ift.tt/2mb4VST để biết thêm về địa chỉ bán tai nghe không dây giá rẻ A Machine Learning Guide for Average Humans https://ift.tt/2rFyAUY xem thêm tại: https://ift.tt/2mb4VST để biết thêm về địa chỉ bán tai nghe không dây giá rẻ A Machine Learning Guide for Average Humans https://ift.tt/2rFyAUY xem thêm tại: https://ift.tt/2mb4VST để biết thêm về địa chỉ bán tai nghe không dây giá rẻ A Machine Learning Guide for Average Humans https://ift.tt/2rFyAUY xem thêm tại: https://ift.tt/2mb4VST để biết thêm về địa chỉ bán tai nghe không dây giá rẻ A Machine Learning Guide for Average Humans https://ift.tt/2rFyAUY xem thêm tại: https://ift.tt/2mb4VST để biết thêm về địa chỉ bán tai nghe không dây giá rẻ A Machine Learning Guide for Average Humans https://ift.tt/2rFyAUY xem thêm tại: https://ift.tt/2mb4VST để biết thêm về địa chỉ bán tai nghe không dây giá rẻ A Machine Learning Guide for Average Humans https://ift.tt/2rFyAUY xem thêm tại: https://ift.tt/2mb4VST để biết thêm về địa chỉ bán tai nghe không dây giá rẻ A Machine Learning Guide for Average Humans https://ift.tt/2rFyAUY xem thêm tại: https://ift.tt/2mb4VST để biết thêm về địa chỉ bán tai nghe không dây giá rẻ A Machine Learning Guide for Average Humans https://ift.tt/2rFyAUY xem thêm tại: https://ift.tt/2mb4VST để biết thêm về địa chỉ bán tai nghe không dây giá rẻ A Machine Learning Guide for Average Humans https://ift.tt/2rFyAUY xem thêm tại: https://ift.tt/2mb4VST để biết thêm về địa chỉ bán tai nghe không dây giá rẻ A Machine Learning Guide for Average Humans https://ift.tt/2rFyAUY xem thêm tại: https://ift.tt/2mb4VST để biết thêm về địa chỉ bán tai nghe không dây giá rẻ A Machine Learning Guide for Average Humans https://ift.tt/2rFyAUY xem thêm tại: https://ift.tt/2mb4VST để biết thêm về địa chỉ bán tai nghe không dây giá rẻ A Machine Learning Guide for Average Humans https://ift.tt/2rFyAUY xem thêm tại: https://ift.tt/2mb4VST để biết thêm về địa chỉ bán tai nghe không dây giá rẻ A Machine Learning Guide for Average Humans https://ift.tt/2rFyAUY xem thêm tại: https://ift.tt/2mb4VST để biết thêm về địa chỉ bán tai nghe không dây giá rẻ A Machine Learning Guide for Average Humans https://ift.tt/2rFyAUY xem thêm tại: https://ift.tt/2mb4VST để biết thêm về địa chỉ bán tai nghe không dây giá rẻ A Machine Learning Guide for Average Humans https://ift.tt/2rFyAUY xem thêm tại: https://ift.tt/2mb4VST để biết thêm về địa chỉ bán tai nghe không dây giá rẻ A Machine Learning Guide for Average Humans https://ift.tt/2rFyAUY xem thêm tại: https://ift.tt/2mb4VST để biết thêm về địa chỉ bán tai nghe không dây giá rẻ A Machine Learning Guide for Average Humans https://ift.tt/2rFyAUY xem thêm tại: https://ift.tt/2mb4VST để biết thêm về địa chỉ bán tai nghe không dây giá rẻ A Machine Learning Guide for Average Humans https://ift.tt/2rFyAUY xem thêm tại: https://ift.tt/2mb4VST để biết thêm về địa chỉ bán tai nghe không dây giá rẻ A Machine Learning Guide for Average Humans https://ift.tt/2rFyAUY xem thêm tại: https://ift.tt/2mb4VST để biết thêm về địa chỉ bán tai nghe không dây giá rẻ A Machine Learning Guide for Average Humans https://ift.tt/2rFyAUY xem thêm tại: https://ift.tt/2mb4VST để biết thêm về địa chỉ bán tai nghe không dây giá rẻ A Machine Learning Guide for Average Humans https://ift.tt/2rFyAUY xem thêm tại: https://ift.tt/2mb4VST để biết thêm về địa chỉ bán tai nghe không dây giá rẻ A Machine Learning Guide for Average Humans https://ift.tt/2rFyAUY xem thêm tại: https://ift.tt/2mb4VST để biết thêm về địa chỉ bán tai nghe không dây giá rẻ A Machine Learning Guide for Average Humans https://ift.tt/2rFyAUY xem thêm tại: https://ift.tt/2mb4VST để biết thêm về địa chỉ bán tai nghe không dây giá rẻ A Machine Learning Guide for Average Humans https://ift.tt/2rFyAUY xem thêm tại: https://ift.tt/2mb4VST để biết thêm về địa chỉ bán tai nghe không dây giá rẻ A Machine Learning Guide for Average Humans https://ift.tt/2rFyAUY xem thêm tại: https://ift.tt/2mb4VST để biết thêm về địa chỉ bán tai nghe không dây giá rẻ A Machine Learning Guide for Average Humans https://ift.tt/2rFyAUY xem thêm tại: https://ift.tt/2mb4VST để biết thêm về địa chỉ bán tai nghe không dây giá rẻ A Machine Learning Guide for Average Humans https://ift.tt/2rFyAUY xem thêm tại: https://ift.tt/2mb4VST để biết thêm về địa chỉ bán tai nghe không dây giá rẻ A Machine Learning Guide for Average Humans https://ift.tt/2rFyAUY xem thêm tại: https://ift.tt/2mb4VST để biết thêm về địa chỉ bán tai nghe không dây giá rẻ A Machine Learning Guide for Average Humans https://ift.tt/2rFyAUY xem thêm tại: https://ift.tt/2mb4VST để biết thêm về địa chỉ bán tai nghe không dây giá rẻ A Machine Learning Guide for Average Humans https://ift.tt/2rFyAUY xem thêm tại: https://ift.tt/2mb4VST để biết thêm về địa chỉ bán tai nghe không dây giá rẻ A Machine Learning Guide for Average Humans https://ift.tt/2rFyAUY xem thêm tại: https://ift.tt/2mb4VST để biết thêm về địa chỉ bán tai nghe không dây giá rẻ A Machine Learning Guide for Average Humans https://ift.tt/2rFyAUY xem thêm tại: https://ift.tt/2mb4VST để biết thêm về địa chỉ bán tai nghe không dây giá rẻ A Machine Learning Guide for Average Humans https://ift.tt/2rFyAUY xem thêm tại: https://ift.tt/2mb4VST để biết thêm về địa chỉ bán tai nghe không dây giá rẻ A Machine Learning Guide for Average Humans https://ift.tt/2rFyAUY xem thêm tại: https://ift.tt/2mb4VST để biết thêm về địa chỉ bán tai nghe không dây giá rẻ A Machine Learning Guide for Average Humans https://ift.tt/2rFyAUY xem thêm tại: https://ift.tt/2mb4VST để biết thêm về địa chỉ bán tai nghe không dây giá rẻ A Machine Learning Guide for Average Humans https://ift.tt/2rFyAUY xem thêm tại: https://ift.tt/2mb4VST để biết thêm về địa chỉ bán tai nghe không dây giá rẻ A Machine Learning Guide for Average Humans https://ift.tt/2rFyAUY xem thêm tại: https://ift.tt/2mb4VST để biết thêm về địa chỉ bán tai nghe không dây giá rẻ A Machine Learning Guide for Average Humans https://ift.tt/2rFyAUY xem thêm tại: https://ift.tt/2mb4VST để biết thêm về địa chỉ bán tai nghe không dây giá rẻ A Machine Learning Guide for Average Humans https://ift.tt/2rFyAUY xem thêm tại: https://ift.tt/2mb4VST để biết thêm về địa chỉ bán tai nghe không dây giá rẻ A Machine Learning Guide for Average Humans https://ift.tt/2rFyAUY xem thêm tại: https://ift.tt/2mb4VST để biết thêm về địa chỉ bán tai nghe không dây giá rẻ A Machine Learning Guide for Average Humans https://ift.tt/2rFyAUY xem thêm tại: https://ift.tt/2mb4VST để biết thêm về địa chỉ bán tai nghe không dây giá rẻ A Machine Learning Guide for Average Humans https://ift.tt/2rFyAUY xem thêm tại: https://ift.tt/2mb4VST để biết thêm về địa chỉ bán tai nghe không dây giá rẻ A Machine Learning Guide for Average Humans https://ift.tt/2rFyAUY xem thêm tại: https://ift.tt/2mb4VST để biết thêm về địa chỉ bán tai nghe không dây giá rẻ A Machine Learning Guide for Average Humans https://ift.tt/2rFyAUY xem thêm tại: https://ift.tt/2mb4VST để biết thêm về địa chỉ bán tai nghe không dây giá rẻ A Machine Learning Guide for Average Humans https://ift.tt/2rFyAUY xem thêm tại: https://ift.tt/2mb4VST để biết thêm về địa chỉ bán tai nghe không dây giá rẻ A Machine Learning Guide for Average Humans https://ift.tt/2rFyAUY xem thêm tại: https://ift.tt/2mb4VST để biết thêm về địa chỉ bán tai nghe không dây giá rẻ A Machine Learning Guide for Average Humans https://ift.tt/2rFyAUY xem thêm tại: https://ift.tt/2mb4VST để biết thêm về địa chỉ bán tai nghe không dây giá rẻ A Machine Learning Guide for Average Humans https://ift.tt/2rFyAUY xem thêm tại: https://ift.tt/2mb4VST để biết thêm về địa chỉ bán tai nghe không dây giá rẻ A Machine Learning Guide for Average Humans https://ift.tt/2rFyAUY xem thêm tại: https://ift.tt/2mb4VST để biết thêm về địa chỉ bán tai nghe không dây giá rẻ A Machine Learning Guide for Average Humans https://ift.tt/2rFyAUY xem thêm tại: https://ift.tt/2mb4VST để biết thêm về địa chỉ bán tai nghe không dây giá rẻ A Machine Learning Guide for Average Humans https://ift.tt/2rFyAUY xem thêm tại: https://ift.tt/2mb4VST để biết thêm về địa chỉ bán tai nghe không dây giá rẻ A Machine Learning Guide for Average Humans https://ift.tt/2rFyAUY xem thêm tại: https://ift.tt/2mb4VST để biết thêm về địa chỉ bán tai nghe không dây giá rẻ A Machine Learning Guide for Average Humans https://ift.tt/2rFyAUY xem thêm tại: https://ift.tt/2mb4VST để biết thêm về địa chỉ bán tai nghe không dây giá rẻ A Machine Learning Guide for Average Humans https://ift.tt/2rFyAUY xem thêm tại: https://ift.tt/2mb4VST để biết thêm về địa chỉ bán tai nghe không dây giá rẻ A Machine Learning Guide for Average Humans https://ift.tt/2rFyAUY xem thêm tại: https://ift.tt/2mb4VST để biết thêm về địa chỉ bán tai nghe không dây giá rẻ A Machine Learning Guide for Average Humans https://ift.tt/2rFyAUY xem thêm tại: https://ift.tt/2mb4VST để biết thêm về địa chỉ bán tai nghe không dây giá rẻ A Machine Learning Guide for Average Humans https://ift.tt/2rFyAUY xem thêm tại: https://ift.tt/2mb4VST để biết thêm về địa chỉ bán tai nghe không dây giá rẻ A Machine Learning Guide for Average Humans https://ift.tt/2rFyAUY xem thêm tại: https://ift.tt/2mb4VST để biết thêm về địa chỉ bán tai nghe không dây giá rẻ A Machine Learning Guide for Average Humans https://ift.tt/2rFyAUY xem thêm tại: https://ift.tt/2mb4VST để biết thêm về địa chỉ bán tai nghe không dây giá rẻ A Machine Learning Guide for Average Humans https://ift.tt/2rFyAUY xem thêm tại: https://ift.tt/2mb4VST để biết thêm về địa chỉ bán tai nghe không dây giá rẻ A Machine Learning Guide for Average Humans https://ift.tt/2rFyAUY xem thêm tại: https://ift.tt/2mb4VST để biết thêm về địa chỉ bán tai nghe không dây giá rẻ A Machine Learning Guide for Average Humans https://ift.tt/2rFyAUY xem thêm tại: https://ift.tt/2mb4VST để biết thêm về địa chỉ bán tai nghe không dây giá rẻ A Machine Learning Guide for Average Humans https://ift.tt/2rFyAUY xem thêm tại: https://ift.tt/2mb4VST để biết thêm về địa chỉ bán tai nghe không dây giá rẻ A Machine Learning Guide for Average Humans https://ift.tt/2rFyAUY xem thêm tại: https://ift.tt/2mb4VST để biết thêm về địa chỉ bán tai nghe không dây giá rẻ A Machine Learning Guide for Average Humans https://ift.tt/2rFyAUY xem thêm tại: https://ift.tt/2mb4VST để biết thêm về địa chỉ bán tai nghe không dây giá rẻ A Machine Learning Guide for Average Humans https://ift.tt/2rFyAUY xem thêm tại: https://ift.tt/2mb4VST để biết thêm về địa chỉ bán tai nghe không dây giá rẻ A Machine Learning Guide for Average Humans https://ift.tt/2rFyAUY xem thêm tại: https://ift.tt/2mb4VST để biết thêm về địa chỉ bán tai nghe không dây giá rẻ A Machine Learning Guide for Average Humans https://ift.tt/2rFyAUY xem thêm tại: https://ift.tt/2mb4VST để biết thêm về địa chỉ bán tai nghe không dây giá rẻ A Machine Learning Guide for Average Humans https://ift.tt/2rFyAUY xem thêm tại: https://ift.tt/2mb4VST để biết thêm về địa chỉ bán tai nghe không dây giá rẻ A Machine Learning Guide for Average Humans https://ift.tt/2rFyAUY xem thêm tại: https://ift.tt/2mb4VST để biết thêm về địa chỉ bán tai nghe không dây giá rẻ A Machine Learning Guide for Average Humans https://ift.tt/2rFyAUY xem thêm tại: https://ift.tt/2mb4VST để biết thêm về địa chỉ bán tai nghe không dây giá rẻ A Machine Learning Guide for Average Humans https://ift.tt/2rFyAUY xem thêm tại: https://ift.tt/2mb4VST để biết thêm về địa chỉ bán tai nghe không dây giá rẻ A Machine Learning Guide for Average Humans https://ift.tt/2rFyAUY xem thêm tại: https://ift.tt/2mb4VST để biết thêm về địa chỉ bán tai nghe không dây giá rẻ A Machine Learning Guide for Average Humans https://ift.tt/2rFyAUY xem thêm tại: https://ift.tt/2mb4VST để biết thêm về địa chỉ bán tai nghe không dây giá rẻ A Machine Learning Guide for Average Humans https://ift.tt/2rFyAUY xem thêm tại: https://ift.tt/2mb4VST để biết thêm về địa chỉ bán tai nghe không dây giá rẻ A Machine Learning Guide for Average Humans https://ift.tt/2rFyAUY xem thêm tại: https://ift.tt/2mb4VST để biết thêm về địa chỉ bán tai nghe không dây giá rẻ A Machine Learning Guide for Average Humans https://ift.tt/2rFyAUY xem thêm tại: https://ift.tt/2mb4VST để biết thêm về địa chỉ bán tai nghe không dây giá rẻ A Machine Learning Guide for Average Humans https://ift.tt/2rFyAUY xem thêm tại: https://ift.tt/2mb4VST để biết thêm về địa chỉ bán tai nghe không dây giá rẻ A Machine Learning Guide for Average Humans https://ift.tt/2rFyAUY xem thêm tại: https://ift.tt/2mb4VST để biết thêm về địa chỉ bán tai nghe không dây giá rẻ A Machine Learning Guide for Average Humans https://ift.tt/2rFyAUY Bạn có thể xem thêm địa chỉ mua tai nghe không dây tại đây https://ift.tt/2mb4VST
0 notes
Text
A Machine Learning Guide for Average Humans
A Machine Learning Guide for Average Humans
Posted by alexis-sanders
//<![CDATA[ (function($) { // code using $ as alias to jQuery $(function() { // Hide the hypotext content. $('.hypotext-content').hide(); // When a hypotext link is clicked. $('a.hypotext.closed').click(function (e) { // custom handling here e.preventDefault(); // Create the class reference from the rel value. var id = '.' + $(this).attr('rel'); // If the content is hidden, show it now. if ( $(id).css('display') == 'none' ) { $(id).show('slow'); if (jQuery.ui) { // UI loaded $(id).effect("highlight", {}, 1000); } } // If the content is shown, hide it now. else { $(id).hide('slow'); } }); // If we have a hash value in the url. if (window.location.hash) { // If the anchor is within a hypotext block, expand it, by clicking the // relevant link. console.log(window.location.hash); var anchor = $(window.location.hash); var hypotextLink = $('#' + anchor.parents('.hypotext-content').attr('rel')); console.log(hypotextLink); hypotextLink.click(); // Wait until the content has expanded before jumping to anchor. //$.delay(1000); setTimeout(function(){ scrollToAnchor(window.location.hash); }, 1000); } }); function scrollToAnchor(id) { var anchor = $(id); $('html,body').animate({scrollTop: anchor.offset().top},'slow'); } })(jQuery); //]]>
Machine learning (ML) has grown consistently in worldwide prevalence. Its implications have stretched from small, seemingly inconsequential victories to groundbreaking discoveries. The SEO community is no exception. An understanding and intuition of machine learning can support our understanding of the challenges and solutions Google's engineers are facing, while also opening our minds to ML's broader implications.
The advantages of gaining an general understanding of machine learning include:
Gaining empathy for engineers, who are ultimately trying to establish the best results for users
Understanding what problems machines are solving for, their current capabilities and scientists' goals
Understanding the competitive ecosystem and how companies are using machine learning to drive results
Preparing oneself for for what many industry leaders call a major shift in our society (Andrew Ng refers to AI as a "new electricity")
Understanding basic concepts that often appear within research (it's helped me with understanding certain concepts that appear within Google Brain's Research)
Growing as an individual and expanding your horizons (you might really enjoy machine learning!)
When code works and data is produced, it's a very fulfilling, empowering feeling (even if it's a very humble result)
I spent a year taking online courses, reading books, and learning about learning (...as a machine). This post is the fruit borne of that labor -- it covers 17 machine learning resources (including online courses, books, guides, conference presentations, etc.) comprising the most affordable and popular machine learning resources on the web (through the lens of a complete beginner). I've also added a summary of "If I were to start over again, how I would approach it."
This article isn't about credit or degrees. It's about regular Joes and Joannas with an interest in machine learning, and who want to spend their learning time efficiently. Most of these resources will consume over 50 hours of commitment. Ain't nobody got time for a painful waste of a work week (especially when this is probably completed during your personal time). The goal here is for you to find the resource that best suits your learning style. I genuinely hope you find this research useful, and I encourage comments on which materials prove most helpful (especially ones not included)! #HumanLearningMachineLearning
Executive summary:
Here's everything you need to know in a chart:
Machine Learning Resource
Time (hours)
Cost ($)
Year
Credibility
Code
Math
Enjoyability
Jason Maye's Machine Learning 101 slidedeck: 2 years of headbanging, so you don't have to
2
$0
'17
{ML} Recipes with Josh Gordon Playlist
2
$0
'16
Machine Learning Crash Course
15
$0
'18
OCDevel Machine Learning Guide Podcast
30
$0
'17-
Kaggle's Machine Learning Track (part 1)
6
$0
'17
Fast.ai (part 1)
70
$70*
'16
Hands-On Machine Learning with Scikit-Learn and TensorFlow: Concepts, Tools, and Techniques to Build Intelligent Systems
20
$25
'17
Udacity's Intro to Machine Learning (Kate/Sebastian)
60
$0
'15
Andrew Ng's Coursera Machine Learning
55
$0
'11
iPullRank Machine Learning Guide
3
$0
'17
Review Google PhD
2
$0
'17
Caltech Machine Learning on iTunes
27
$0
'12
Pattern Recognition & Machine Learning by Christopher Bishop
150
$75
'06
N/A
Machine Learning: Hands-on for Developers and Technical Professionals
15
$50
'15
Introduction to Machine Learning with Python: A Guide for Data Scientists
15
$25
'16
Udacity's Machine Learning by Georgia Tech
96
$0
'15
Machine Learning Stanford iTunes by Andrew Ng
25
$0
'08
N/A
*Free, but there is the cost of running an AWS EC2 instance (~$70 when I finished, but I did tinker a ton and made a Rick and Morty script generator, which I ran many epochs [rounds] of...)
Here's my suggested program:
1. Starting out (estimated 60 hours)
Start with shorter content targeting beginners. This will allow you to get the gist of what's going on with minimal time commitment.
Commit three hours to Jason Maye's Machine Learning 101 slidedeck: 2 years of headbanging, so you don't have to.
Commit two hours to watch Google's {ML} Recipes with Josh Gordon YouTube Playlist.
Sign up for Sam DeBrule's Machine Learnings newsletter.
Work through Google's Machine Learning Crash Course.
Start listening to OCDevel's Machine Learning Guide Podcast (skip episodes 1, 3, 16, 21, and 26) in your car, working out, and/or when using hands and eyes for other activities.
Commit two days to working through Kaggle's Machine Learning Track part 1.
2. Ready to commit (estimated 80 hours)
By this point, learners would understand their interest levels. Continue with content focused on applying relevant knowledge as fast as possible.
Commit to Fast.ai 10 hours per week, for 7 weeks. If you have a friend/mentor that can help you work through AWS setup, definitely lean on any support in installation (it's 100% the worst part of ML).
Acquire Hands-On Machine Learning with Scikit-Learn and TensorFlow: Concepts, Tools, and Techniques to Build Intelligent Systems, and read the first two chapters immediately. Then use this as supplemental to the Fast.ai course.
3. Broadening your horizons (estimated 115 hours)
If you've made it through the last section and are still hungry for more knowledge, move on to broadening your horizons. Read content focused on teaching the breadth of machine learning -- building an intuition for what the algorithms are trying to accomplish (whether visual or mathematically).
Start watching videos and participating in Udacity's Intro to Machine Learning (by Sebastian Thrun and Katie Malone).
Work through Andrew Ng's Coursera Machine Learning course.
Your next steps
By this point, you will already have AWS running instances, a mathematical foundation, and an overarching view of machine learning. This is your jumping-off point to determine what you want to do.
You should be able to determine your next step based on your interest, whether it's entering Kaggle competitions; doing Fast.ai part two; diving deep into the mathematics with Pattern Recognition & Machine Learning by Christopher Bishop; giving Andrew Ng's newer Deeplearning.ai course on Coursera; learning more about specific tech stacks (TensorFlow, Scikit-Learn, Keras, Pandas, Numpy, etc.); or applying machine learning to your own problems.
Why am I recommending these steps and resources?
I am not qualified to write an article on machine learning. I don't have a PhD. I took one statistics class in college, which marked the first moment I truly understood "fight or flight" reactions. And to top it off, my coding skills are lackluster (at their best, they're chunks of reverse-engineered code from Stack Overflow). Despite my many shortcomings, this piece had to be written by someone like me, an average person.
Statistically speaking, most of us are average (ah, the bell curve/Gaussian distribution always catches up to us). Since I'm not tied to any elitist sentiments, I can be real with you. Below contains a high-level summary of my reviews on all of the classes I took, along with a plan for how I would approach learning machine learning if I could start over. Click to expand each course for the full version with notes.
In-depth reviews of machine learning courses:
Starting out
Jason Maye's Machine Learning 101 slidedeck: 2 years of head-banging, so you don't have to ↓
Need to Know: A stellar high-level overview of machine learning fundamentals in an engaging and visually stimulating format.
Loved:
Very user-friendly, engaging, and playful slidedeck.
Has the potential to take some of the pain out of the process, through introducing core concepts.
Breaks up content by beginner/need-to-know (green), and intermediate/less-useful noise (specifically for individuals starting out) (blue).
Provides resources to dive deeper into machine learning.
Provides some top people to follow in machine learning.
Disliked:
That there is not more! Jason's creativity, visual-based teaching approach, and quirky sense of humor all support the absorption of the material.
Lecturer:
Jason Mayes:
Senior Creative Technologist and Research Engineer at Google
Masters in Computer Science from University of Bristols
Personal Note: He's also kind on Twitter! :)
Links:
Machine Learning 101 slide deck
Tips on Watching:
Set aside 2-4 hours to work through the deck once.
Since there is a wealth of knowledge, refer back as needed (or as a grounding source).
Identify areas of interest and explore the resources provided.
{ML} Recipes with Josh Gordon ↓
Need to Know: This mini-series YouTube-hosted playlist covers the very fundamentals of machine learning with opportunities to complete exercises.
Loved:
It is genuinely beginner-focused.
They make no assumption of any prior knowledge.
Gloss over potentially complex topics that may serve as noise.
Playlist ~2 hours
Very high-quality filming, audio, and presentation, almost to the point where it had its own aesthetic.
Covers some examples in scikit-learn and TensorFlow, which felt modern and practical.
Josh Gordon was an engaging speaker.
Disliked:
I could not get Dockers on Windows (suggested package manager). This wasn't a huge deal, since I already had my AWS setup by this point; however, a bit of a bummer since it made it impossible to follow certain steps exactly.
Issue: Every time I tried to download (over the course of two weeks), the .exe file would recursively start and keep spinning until either my memory ran out, computer crashed, or I shut my computer down. I sent this to Docker's Twitter account to no avail.
Lecturer:
Josh Gordon:
Developer Advocate for at TensorFlow at Google
Leads Machine Learning advocacy at Google
Member of the Udacity AI & Data Industry Advisory Board
Masters in Computer Science from Columbia University
Links:
Hello World - Machine Learning Recipes #1 (YouTube)
GitHub: Machine Learning Recipes with Josh Gordon
Tips on Watching:
The playlist is short (only ~1.5 hours screen time). However, it can be a bit fast-paced at times (especially if you like mimicking the examples), so set aside 3-4 hours to play around with examples and allow time for installation, pausing, and following along.
Take time to explore code labs.
Google's Machine Learning Crash Course with TensorFlow APIs ↓
Need to Know: A Google researcher-made crash course on machine learning that is interactive and offers its own built-in coding system!
Loved:
Different formats of learning: high-quality video (with ability to adjust speed, closed captioning), readings, quizzes (with explanations), visuals (including whiteboarding), interactive components/ playgrounds, code lab exercises (run directly in your browser (no setup required!))
Non-intimidating
One of my favorite quotes: "You don't need to understand the math to be able to take a look at the graphical interpretation."
Broken down into digestible sections
Introduces key terms
Disliked:
N/A
Lecturers:
Multiple Google researchers participated in this course, including:
Peter Norvig
Director of Research at Google Inc.
Previously he directed Google's core search algorithms group.
He is co-author of Artificial Intelligence: A Modern Approach
D. Sculley
Senior Staff Software Engineer at Google
KDD award-winning papers
Works on massive-scale ML systems for online advertising
Was part of a research ML paper on optimizing chocolate chip cookies
According to his personal website, he prefers to go by "D."
Cassandra Xia
Programmer, Software Engineer at Google
She has some really cool (and cute) projects based on learning statistics concepts interactively
Maya Gupta
Leads Glassbox Machine Learning R&D team at Google
Associate Professor of Electrical Engineering at the University of Washington (2003-2012)
In 2007, Gupta received the PECASE award from President George Bush for her work in classifying uncertain (e.g. random) signals
Gupta also runs Artifact Puzzles, the second-largest US maker of wooden jigsaw puzzles
Sally Goldman
Research Scientist at Google
Co-author of A Practical Guide to Data Structures and Algorithms Using Java
Numerous journals, classes taught at Washington University, and contributions to the ML community
Links:
Machine Learning Crash Course
Tips on Doing:
Actively work through playground and coding exercises
OCDevel's Machine Learning Guide Podcast ↓
Need to Know: This podcast focuses on the high-level fundamentals of machine learning, including basic intuition, algorithms, math, languages, and frameworks. It also includes references to learn more on each episode's topic.
Loved:
Great for trips (when traveling a ton, it was an easy listen).
The podcast makes machine learning fun with interesting and compelling analogies.
Tyler is a big fan of Andrew Ng's Coursera course and reviews concepts in Coursera course very well, such that both pair together nicely.
Covers the canonical resources for learning more on a particular topic.
Disliked:
Certain courses were more theory-based; all are interesting, yet impractical.
Due to limited funding the project is a bit slow to update and has less than 30 episodes.
Podcaster:
Tyler Renelle:
Machine learning engineer focused on time series and reinforcement
Background in full-stack JavaScript, 10 years web and mobile
Creator of HabitRPG, an app that treats habits as an RPG game
Links:
Machine Learning Guide podcast
Machine Learning Guide podcast (iTunes)
Tips on Listening:
Listen along your journey to help solidify understanding of topics.
Skip episodes 1, 3, 16, 21, and 26 (unless their topics interest and inspire you!).
Kaggle Machine Learning Track (Lesson 1) ↓
Need to Know: A simple code lab that covers the very basics of machine learning with scikit-learn and Panda through the application of the examples onto another set of data.
Loved:
A more active form of learning.
An engaging code lab that encourages participants to apply knowledge.
This track offers has a built-in Python notebook on Kaggle with all input files included. This removed any and all setup/installation issues.
Side note: It's a bit different than Jupyter notebook (e.g., have to click into a cell to add another cell).
Each lesson is short, which made the entire lesson go by very fast.
Disliked:
The writing in the first lesson didn't initially make it clear that one would need to apply the knowledge in the lesson to their workbook.
It wasn't a big deal, but when I started referencing files in the lesson, I had to dive into the files in my workbook to find they didn't exist, only to realize that the knowledge was supposed to be applied and not transcribed.
Lecturer:
Dan Becker:
Data Scientist at Kaggle
Undergrad in Computer Science, PhD in Econometrics
Supervised data science consultant for six Fortune 100 companies
Contributed to the Keras and Tensorflow libraries
Finished 2nd (out of 1353 teams) in $3 million Heritage Health Prize data mining competition
Speaks at deep learning workshops at events and conferences
Links:
https://www.kaggle.com/learn/machine-learning
Tips on Doing:
Read the exercises and apply to your dataset as you go.
Try lesson 2, which covers more complex/abstract topics (note: this second took a bit longer to work through).
Ready to commit
Fast.ai (part 1 of 2) ↓
Need to Know: Hands-down the most engaging and active form of learning ML. The source I would most recommend for anyone (although the training plan does help to build up to this course). This course is about learning through coding. This is the only course that I started to truly see the practical mechanics start to come together. It involves applying the most practical solutions to the most common problems (while also building an intuition for those solutions).
Loved:
Course Philosophy:
Active learning approach
"Go out into the world and understand underlying mechanics (of machine learning by doing)."
Counter-culture to the exclusivity of the machine learning field, focusing on inclusion.
"Let's do shit that matters to people as quickly as possible."
Highly pragmatic approach with tools that are currently being used (Jupyter Notebooks, scikit-learn, Keras, AWS, etc.).
Show an end-to-end process that you get to complete and play with in a development environment.
Math is involved, but is not prohibitive. Excel files helped to consolidate information/interact with information in a different way, and Jeremy spends a lot of time recapping confusing concepts.
Amazing set of learning resources that allow for all different styles of learning, including:
Video Lessons
Notes
Jupyter Notebooks
Assignments
Highly active forums
Resources on Stackoverflow
Readings/resources
Jeremy often references popular academic texts
Jeremy's TEDx talk in Brussels
Jeremy really pushes one to do extra and put in the effort by teaching interesting problems and engaging one in solving them.
It's a huge time commitment; however, it's worth it.
All of the course's profits are donated.
Disliked:
Overview covers their approach to learning (obviously I'm a fan!). If you're already drinking the Kool-aid, skip past.
I struggled through the AWS setup (13-minute video) for about five hours (however, it felt so good when it was up and running!).
Because of its practicality and concentration on solutions used today to solve popular problem types (image recognition, text generation, etc.), it lacks breadth of machine learning topics.
Lecturers:
Jeremy Howard:
Distinguished Research Scientist at the University of San Francisco
Faculty member at Singularity University
Young Global Leader with the World Economic Forum
Founder of Enlitic (the first company to apply deep learning to medicine)
Former President and Chief Scientist of the data science platform Kaggle
Rachel Thomas:
PhD in Math from Duke
One of Forbes' "20 Incredible Women Advancing AI Research"
Researcher-in-residence at the University of San Francisco Data Institute
Teaches in the Masters in Data Science program
Links:
http://course.fast.ai/start.html
http://wiki.fast.ai/index.php/Main_Page
https://github.com/fastai/courses/tree/master/deeplearning1/nbs
Tips on Doing:
Set expectations with yourself that installation is going to probably take a few hours.
Prepare to spend about ~70 hours for this course (it's worth it).
Don't forget to shut off your AWS instance.
Balance out machine learning knowledge with a course with more breadth.
Consider giving part two of the Fast.ai program a shot!
Hands-On Machine Learning with Scikit-Learn and TensorFlow: Concepts, Tools, and Techniques to Build Intelligent Systems ↓
Need to Know: This book is an Amazon best seller for a reason. It covers a lot of ground quickly, empowers readers to walk through a machine learning problem by chapter two, and contains practical up-to-date machine learning skills.
Loved:
Book contains an amazing introduction to machine learning that briskly provides an overarching quick view of the machine learning ecosystem.
Chapter 2 immediately walks the reader through an end-to-end machine learning problem.
Immediately afterwards, Aurélien pushes a user to attempt to apply this solution to another problem, which was very empowering.
There are review questions at the end of each chapter to ensure on has grasped the content within the chapter and to push the reader to explore more.
Once installation was completed, it was easy to follow and all code is available on GitHub.
Chapters 11-14 were very tough reading; however, they were a great reference when working through Fast.ai.
Contains some powerful analogies.
Each chapter's introductions were very useful and put everything into context. This general-to-specifics learning was very useful.
Disliked:
Installation was a common source of issues during the beginning of my journey; the text glided over this. I felt the frustration that most people experience from installation should have been addressed with more resources.
Writer:
Aurélien Géron:
Led the YouTube video classification team from 2013 to 2016
Currently a machine Learning consultant
Founder and CTO of Wifirst and Polyconseil
Published technical books (on C++, Wi-Fi, and Internet architectures)
Links:
https://www.amazon.com/_/dp/1491962291?tag=oreilly20-20
http://shop.oreilly.com/product/0636920052289.do
https://github.com/ageron/handson-ml
Tips on Using:
Get a friend with Python experience to help with installation.
Read the introductions to each chapter thoroughly, read the chapter (pay careful attention to code), review the questions at the end (highlight any in-text answer), make a copy of Aurélien's GitHub and make sure everything works on your setup, re-type the notebooks, go to Kaggle and try on other datasets.
Broadening your horizons
Udacity: Intro to Machine Learning (Kate/Sebastian) ↓
Need to Know: A course that covers a range of machine learning topics, supports building of intuition via visualization and simple examples, offers coding challenges, and a certificate (upon completion of a final project). The biggest challenge with this course is bridging the gap between the hand-holding lectures and the coding exercises.
Loved:
Focus on developing a visual intuition on what each model is trying to accomplish.
This visual learning mathematics approach is very useful.
Cover a vast variety and breadth of models and machine learning basics.
In terms of presenting the concept, there was a lot of hand-holding (which I completely appreciated!).
Many people have done this training, so their GitHub accounts can be used as reference for the mini-projects.
Katie actively notes documentation and suggests where viewers can learn more/reference material.
Disliked:
All of the conceptual hand-holding in the lessons is a stark contrast to the challenges of installation, coding exercises, and mini-projects.
This is the first course started and the limited instructions on setting up the environment and many failed attempts caused me to break down crying at least a handful of times.
The mini-projects are intimidating.
There is extra code added to support the viewers; however, it's done so with little acknowledgement as to what it's actually doing. This made learning a bit harder.
Lecturer:
Caitlin (Katie) Malone:
Director of Data Science Research and Development at Civis Analytics
Stanford PhD in Experimental Particle Physics
Intern at Udacity in summer 2014
Graduate Researcher at the SLAC National Accelerator Laboratory
https://www6.slac.stanford.edu/
Podcaster with Ben Jaffe (currently Facebook UI Engineer and a music aficionado) on a machine learning podcast Linear Digressions (100+ episodes)
Sebastian Thrun:
CEO of the Kitty Hawk Corporation
Chairman and co-founder of Udacity
One of my favorite Sebastian quotes: "It occurred to me, I could be at Google and build a self-driving car, or I can teach 10,000 students how to build self-driving cars."
Former Google VP
Founded Google X
Led development of the robotic vehicle Stanley
Professor of Computer Science at Stanford University
Formerly a professor at Carnegie Mellon University.
Links:
https://www.udacity.com/course/intro-to-machine-learning--ud120
Udacity also offers a next step, the Machine Learning Engineer Nanodegree, which will set one back about $1K.
Tips on Watching:
Get a friend to help you set up your environment.
Print mini-project instructions to check off each step.
Andrew Ng's Coursera Machine Learning Course ↓
Need to Know: The Andrew Ng Coursera course is the most referenced online machine learning course. It covers a broad set of fundamental, evergreen topics with a strong focus in building mathematical intuition behind machine learning models. Also, one can submit assignments and earn a grade for free. If you want to earn a certificate, one can subscribe or apply for financial aid.
Loved:
This course has a high level of credibility.
Introduces all necessary machine learning terminology and jargon.
Contains a very classic machine learning education approach with a high level of math focus.
Quizzes interspersed in courses and after each lesson support understanding and overall learning.
The sessions for the course are flexible, the option to switch into a different section is always available.
Disliked:
The mathematic notation was hard to process at times.
The content felt a bit dated and non-pragmatic. For example, the main concentration was MATLAB and Octave versus more modern languages and resources.
Video quality was less than average and could use a refresh.
Lecturer:
Andrew Ng:
Adjunct Professor, Stanford University (focusing on AI, Machine Learning, and Deep Learning)
Co-founder of Coursera
Former head of Baidu AI Group
Founder and previous head of Google Brain (deep learning) project
Former Director of the Stanford AI Lab
Chairman of the board of Woebot (a machine learning bot that focuses on Cognitive Behavior Therapy)
Links:
https://www.coursera.org/learn/machine-learning/
Andrew Ng recently launched a new course (August 2017) called DeepLearning.ai, a ~15 week course containing five mini-courses ($49 USD per month to continue learning after trial period of 7 days ends).
Course: https://www.coursera.org/specializations/deep-learning
Course 1: Neural Networks and Deep Learning
Course 2: Improving Deep Neural Networks: Hyperparameter tuning, Regularization and Optimization
Course 3: Structuring Machine Learning Projects
Course 4: Convolutional Neural Networks
Course 5: Sequence Models
Tips on Watching:
Be disciplined with setting aside timing (even if it's only 15 minutes a day) to help power through some of the more boring concepts.
Don't do this course first, because it's intimidating, requires a large time commitment, and isn't a very energizing experience.
Additional machine learning opportunities
iPullRank Machine Learning Guide ↓
Need to Know: A machine learning e-book targeted at marketers.
Loved:
Targeted at marketers and applied to organic search.
Covers a variety of machine learning topics.
Some good examples, including real-world blunders.
Gives some practical tools for non-data scientists (including: MonkeyLearn and Orange)
I found Orange to be a lot of fun. It struggled with larger datasets; however, it has a very visual interface that was more user-friendly and offers potential to show some pretty compelling stories.
Example: World Happiness Dataset by:
X-axis: Happiness Score
Y-axis: Economy
Color: Health
Disliked:
Potential to break up content more with relevant imagery -- the content was very dense.
Writers:
iPullRank Team (including Mike King):
Mike King has a few slide decks on the basics of machine learnings and AI
iPullRank has a few data scientists on staff
Links:
http://ipullrank.com/machine-learning-guide/
Tips on Reading:
Read chapters 1-6 and the rest depending upon personal interest.
Review Google PhD ↓
Need to Know: A two-hour presentation from Google's 2017 IO conference that walks through getting 99% accuracy on the MNIST dataset (a famous dataset containing a bunch of handwritten numbers, which the machine must learn to identify the numbers).
Loved:
This talk struck me as very modern, covering the cutting edge.
Found this to be very complementary to Fast.ai, as it covered similar topics (e.g. ReLu, CNNs, RNNs, etc.)
Amazing visuals that help to put everything into context.
Disliked:
The presentation is only a short conference solution and not a comprehensive view of machine learning.
Also, a passive form of learning.
Presenter:
Martin Görner:
Developer Relations, Google (since 2011)
Started Mobipocket, a startup that later became the software part of the Amazon Kindle and its mobile variants
Links:
Part 1 - https://www.youtube.com/watch?v=u4alGiomYP4
Part 2 - https://www.youtube.com/watch?v=fTUwdXUFfI8
Tips on Watching:
Google any concepts you're unfamiliar with.
Take your time with this one; 2 hours of screen time doesn't count all of the Googling and processing time for this one.
Caltech Machine Learning iTunes ↓
Need to Know: If math is your thing, this course does a stellar job of building the mathematic intuition behind many machine learning models. Dr. Abu-Mostafa is a raconteur, includes useful visualizations, relevant real-world examples, and compelling analogies.
Loved:
First and foremost, this is a real Caltech course, meaning it's not a watered-down version and contains fundamental concepts that are vital to understanding the mechanics of machine learning.
On iTunes, audio downloads are available, which can be useful for on-the-go learning.
Dr. Abu-Mostafa is a skilled speaker, making the 27 hours spent listening much easier!
Dr. Abu-Mostafa offers up some strong real-world examples and analogies which makes the content more relatable.
As an example, he asks students: "Why do I give you practice exams and not just give you the final exam?" as an illustration of why a testing set is useful. If he were to just give students the final, they would just memorize the answers (i.e., they would overfit to the data) and not genuinely learn the material. The final is a test to show how much students learn.
The last 1/2 hour of the class is always a Q&A, where students can ask questions. Their questions were useful to understanding the topic more in-depth.
The video and audio quality was strong throughout. There were a few times when I couldn't understand a question in the Q&A, but overall very strong.
This course is designed to build mathematical intuition of what's going on under the hood of specific machine learning models.
Caution: Dr. Abu-Mostafa uses mathematical notation, but it's different from Andrew Ng's (e.g., theta = w).
The final lecture was the most useful, as it pulled a lot of the conceptual puzzle pieces together. The course on neural networks was a close second!
Disliked:
Although it contains mostly evergreen content, being released in 2012, it could use a refresh.
Very passive form of learning, as it wasn't immediately actionable.
Lecturer:
Dr. Yaser S. Abu-Mostafa:
Professor of Electrical Engineering and Computer Science at the California Institute of Technology
Chairman of Machine Learning Consultants LLC
Serves on a number of scientific advisory boards
Has served as a technical consultant on machine learning for several companies (including Citibank).
Multiple articles in Scientific American
Links:
https://work.caltech.edu/telecourse.html
https://itunes.apple.com/us/course/machine-learning/id515364596
Tips on Watching:
Consider listening to the last lesson first, as it pulls together the course overall conceptually. The map of the course, below, was particularly useful to organizing the information taught in the courses.
Image source: http://work.caltech.edu/slides/slides18.pdf
"Pattern Recognition & Machine Learning" by Christopher Bishop ↓
Need to Know: This is a very popular college-level machine learning textbook. I've heard it likened to a bible for machine learning. However, after spending a month trying to tackle the first few chapters, I gave up. It was too much math and pre-requisites to tackle (even with a multitude of Google sessions).
Loved:
The text of choice for many major universities, so if you can make it through this text and understand all of the concepts, you're probably in a very good position.
I appreciated the history aside sections, where Bishop talked about influential people and their career accomplishments in statistics and machine learning.
Despite being a highly mathematically text, the textbook actually has some pretty visually intuitive imagery.
Disliked:
I couldn't make it through the text, which was a bit frustrating. The statistics and mathematical notation (which is probably very benign for a student in this topic) were too much for me.
The sunk cost was pretty high here (~$75).
Writer:
Christopher Bishop:
Laboratory Director at Microsoft Research Cambridge
Professor of Computer Science at the University of Edinburgh
Fellow of Darwin College, Cambridge
PhD in Theoretical Physics from the University of Edinburgh
Links:
https://www.amazon.com/Pattern-Recognition-Learning-Information-Statistics/dp/0387310738/ref=sr_1_2?ie=UTF8&qid=1516839475&sr=8-2&keywords=Pattern+Recognition+%26+Machine+Learning
Tips on Reading:
Don't start your machine learning journey with this book.
Get a friend in statistics to walk you through anything complicated (my plan is to get a mentor in statistics).
Consider taking a (free) online statistics course (Khan Academy and Udacity both have some great content on statistics, calculus, math, and data analysis).
Machine Learning: Hands-on for Developers and Technical Professionals ↓
Need to Know: A fun, non-intimidating end-to-end launching pad/whistle stop for machine learning in action.
Loved:
Talks about practical issues that many other sources didn't really address (e.g. data-cleansing).
Covered the basics of machine learning in a non-intimidating way.
Offers abridged, consolidated versions of the content.
Added fun anecdotes that makes it easier to read.
Overall the writer has a great sense of humor.
Writer talks to the reader as if they're a real human being (i.e., doesn't expect you to go out and do proofs; acknowledges the challenge of certain concepts).
Covers a wide variety of topics.
Because it was well-written, I flew through the book (even though it's about ~300 pages).
Disliked:
N/A
Writer:
Jason Bell:
Technical architect, lecturer, and startup consultant
Data Engineer at MastodonC
Former section editor for Java Developer's Journal
Former writer on IBM DeveloperWorks
Links:
https://www.amazon.com/Machine-Learning-Hands-Developers-Professionals/dp/1118889061
https://www.wiley.com/en-us/Machine+Learning%3A+Hands+On+for+Developers+and+Technical+Professionals-p-9781118889060
Jason's Blog: https://dataissexy.wordpress.com/
Tips on Reading:
Download and explore Weka's interface beforehand.
Give some of the exercises a shot.
Introduction to Machine Learning with Python: A Guide for Data Scientists ↓
Need to Know: This was a was a well-written piece on machine learning, making it a quick read.
Loved:
Quick, smooth read.
Easy-to-follow code examples.
The first few chapters served as a stellar introduction to the basics of machine learning.
Contain subtle jokes that add a bit of fun.
Tip to use the Python package manager Anaconda with Jupyter Notebooks was helpful.
Disliked:
Once again, installation was a challenge.
The "mglearn" utility library threw me for a loop. I had to reread the first few chapters before I figured out it was support for the book.
Although I liked the book, I didn't love it. Overall it just missed the "empowering" mark.
Writers:
Andreas C. Müller:
PhD in Computer Science
Lecturer at the Data Science Institute at Columbia University
Worked at the NYU Center for Data Science on open source and open science
Former Machine Learning Scientist at Amazon
Speaks often on Machine Learning and scikit-learn (a popular machine learning library)
And he makes some pretty incredibly useful graphics, such as this scikit-learn cheat sheet:
Image source: http://peekaboo-vision.blogspot.com/2013/01/machin...
Sarah Guido:
Former senior data scientist at Mashable
Lead data scientist at Bitly
2018 SciPy Conference Data Science track co-chair
Links:
https://www.amazon.com/Introduction-Machine-Learning-Python-Scientists/dp/1449369413/ref=sr_1_7?s=books&ie=UTF8&qid=1516734322&sr=1-7&keywords=python+machine+learning
http://shop.oreilly.com/product/0636920030515.do
Tips on Reading:
Type out code examples.
Beware of the "mglearn" utility library.
Udacity: Machine Learning by Georgia Tech ↓
Need to Know: A mix between an online learning experience and a university machine learning teaching approach. The lecturers are fun, but the course still fell a bit short in terms of active learning.
Loved:
This class is offered as CS7641 at Georgia Tech, where it is a part of the Online Masters Degree. Although taking this course here will not earn credit towards the OMS degree, it's still a non-watered-down college teaching philosophy approach.
Covers a wide variety of topics, many of which reminded me of the Caltech course (including: VC Dimension versus Bayesian, Occam's razor, etc.)
Discusses Markov Decision Chains, which is something that didn't really come up in many other introductory machine learning course, but they are referenced within Google patents.
The lecturers have a great dynamic, are wicked smart, and displayed a great sense of (nerd) humor, which make the topics less intimidating.
The course has quizzes, which give the course a slight amount of interaction.
Disliked:
Some videos were very long, which made the content a bit harder to digest.
The course overall was very time consuming.
Despite the quizzes, the course was a very passive form of learning with no assignments and little coding.
Many videos started with a bunch of content already written out. Having the content written out was probably a big time-saver, but it was also a bit jarring for a viewer to see so much information all at once, while also trying to listen.
It's vital to pay very close attention to notation, which compounds in complexity quickly.
Tablet version didn't function flawlessly: some was missing content (which I had to mark down and review on a desktop), the app would crash randomly on the tablet, and sometimes the audio wouldn't start.
There were no subtitles available on tablet, which I found not only to be a major accessibility blunder, but also made it harder for me to process (since I'm not an audio learner).
Lecturer:
Michael Littman:
Professor of Computer Science at Brown University.
Was granted a patent for one of the earliest systems for Cross-language information retrieval
Perhaps the most interesting man in the world:
Been in two TEDx talks
How I Learned to Stop Worrying and Be Realistic About AI
A Cooperative Path to Artificial Intelligence
During his time at Duke, he worked on an automated crossword solver (PROVERB)
Has a Family Quartet
He has appeared in a TurboTax commercial
Charles Isbell:
Professor and Executive Associate Dean at School of Interactive Computing at Georgia Tech
Focus on statistical machine learning and "interactive" artificial intelligence.
Links:
https://www.udacity.com/course/machine-learning--ud262
Tips on Watching:
Pick specific topics of interest and focusing on those lessons.
Andrew Ng's Stanford's Machine Learning iTunes ↓
Need to Know: A non-watered-down Stanford course. It's outdated (filmed in 2008), video/audio are a bit poor, and most links online now point towards the Coursera course. Although the idea of watching a Stanford course was energizing for the first few courses, it became dreadfully boring. I made it to course six before calling it.
Loved:
Designed for students, so you know you're not missing out on anything.
This course provides a deeper study into the mathematical and theoretical foundation behind machine learning to the point that the students could create their own machine learning algorithms. This isn't necessarily very practical for the everyday machine learning user.
Has some powerful real-world examples (although they're outdated).
There is something about the kinesthetic nature of watching someone write information out. The blackboard writing helped me to process certain ideas.
Disliked:
Video and audio quality were pain to watch.
Many questions asked by students were hard to hear.
On-screen visuals range from hard to impossible to see.
Found myself counting minutes.
Dr. Ng mentions TA classes, supplementary learning, but these are not available online.
Sometimes the video showed students, which I felt was invasive.
Lecturer:
Andrew Ng (see above)
Links:
https://itunes.apple.com/us/course/machine-learning/id495053006
https://www.youtube.com/watch?v=UzxYlbK2c7E
Tips on Watching:
Only watch if you're looking to gain a deeper understanding of the math presented in the Coursera course.
Skip the first half of the first lecture, since it's mostly class logistics.
Additional Resources
Fast.ai (part 2) - free access to materials, cost for AWS EC2 instance
Deeplearning.ai - $50/month
Udacity Machine Learning Engineer Nanodegree - $1K
https://machinelearningmastery.com/
Motivations and inspiration
If you're wondering why I spent a year doing this, then I'm with you. I'm genuinely not sure why I set my sights on this project, much less why I followed through with it. I saw Mike King give a session on Machine Learning. I was caught off guard, since I knew nothing on the topic. It gave me a pesky, insatiable curiosity itch. It started with one course and then spiraled out of control. Eventually it transformed into an idea: a review guide on the most affordable and popular machine learning resources on the web (through the lens of a complete beginner). Hopefully you found it useful, or at least somewhat interesting. Be sure to share your thoughts or questions in the comments!
Sign up for The Moz Top 10, a semimonthly mailer updating you on the top ten hottest pieces of SEO news, tips, and rad links uncovered by the Moz team. Think of it as your exclusive digest of stuff you don't have time to hunt down but want to read!
xem them tai https://ift.tt/2o9GYfe A Machine Learning Guide for Average Humans xem thêm tại: https://ift.tt/2mb4VST để biết thêm về địa chỉ bán tai nghe không dây giá rẻ A Machine Learning Guide for Average Humans https://ift.tt/2rFyAUY xem thêm tại: https://ift.tt/2mb4VST để biết thêm về địa chỉ bán tai nghe không dây giá rẻ A Machine Learning Guide for Average Humans https://ift.tt/2rFyAUY xem thêm tại: https://ift.tt/2mb4VST để biết thêm về địa chỉ bán tai nghe không dây giá rẻ A Machine Learning Guide for Average Humans https://ift.tt/2rFyAUY xem thêm tại: https://ift.tt/2mb4VST để biết thêm về địa chỉ bán tai nghe không dây giá rẻ A Machine Learning Guide for Average Humans https://ift.tt/2rFyAUY xem thêm tại: https://ift.tt/2mb4VST để biết thêm về địa chỉ bán tai nghe không dây giá rẻ A Machine Learning Guide for Average Humans https://ift.tt/2rFyAUY xem thêm tại: https://ift.tt/2mb4VST để biết thêm về địa chỉ bán tai nghe không dây giá rẻ A Machine Learning Guide for Average Humans https://ift.tt/2rFyAUY xem thêm tại: https://ift.tt/2mb4VST để biết thêm về địa chỉ bán tai nghe không dây giá rẻ A Machine Learning Guide for Average Humans https://ift.tt/2rFyAUY xem thêm tại: https://ift.tt/2mb4VST để biết thêm về địa chỉ bán tai nghe không dây giá rẻ A Machine Learning Guide for Average Humans https://ift.tt/2rFyAUY xem thêm tại: https://ift.tt/2mb4VST để biết thêm về địa chỉ bán tai nghe không dây giá rẻ A Machine Learning Guide for Average Humans https://ift.tt/2rFyAUY xem thêm tại: https://ift.tt/2mb4VST để biết thêm về địa chỉ bán tai nghe không dây giá rẻ A Machine Learning Guide for Average Humans https://ift.tt/2rFyAUY xem thêm tại: https://ift.tt/2mb4VST để biết thêm về địa chỉ bán tai nghe không dây giá rẻ A Machine Learning Guide for Average Humans https://ift.tt/2rFyAUY xem thêm tại: https://ift.tt/2mb4VST để biết thêm về địa chỉ bán tai nghe không dây giá rẻ A Machine Learning Guide for Average Humans https://ift.tt/2rFyAUY xem thêm tại: https://ift.tt/2mb4VST để biết thêm về địa chỉ bán tai nghe không dây giá rẻ A Machine Learning Guide for Average Humans https://ift.tt/2rFyAUY xem thêm tại: https://ift.tt/2mb4VST để biết thêm về địa chỉ bán tai nghe không dây giá rẻ A Machine Learning Guide for Average Humans https://ift.tt/2rFyAUY xem thêm tại: https://ift.tt/2mb4VST để biết thêm về địa chỉ bán tai nghe không dây giá rẻ A Machine Learning Guide for Average Humans https://ift.tt/2rFyAUY xem thêm tại: https://ift.tt/2mb4VST để biết thêm về địa chỉ bán tai nghe không dây giá rẻ A Machine Learning Guide for Average Humans https://ift.tt/2rFyAUY xem thêm tại: https://ift.tt/2mb4VST để biết thêm về địa chỉ bán tai nghe không dây giá rẻ A Machine Learning Guide for Average Humans https://ift.tt/2rFyAUY xem thêm tại: https://ift.tt/2mb4VST để biết thêm về địa chỉ bán tai nghe không dây giá rẻ A Machine Learning Guide for Average Humans https://ift.tt/2rFyAUY xem thêm tại: https://ift.tt/2mb4VST để biết thêm về địa chỉ bán tai nghe không dây giá rẻ A Machine Learning Guide for Average Humans https://ift.tt/2rFyAUY xem thêm tại: https://ift.tt/2mb4VST để biết thêm về địa chỉ bán tai nghe không dây giá rẻ A Machine Learning Guide for Average Humans https://ift.tt/2rFyAUY xem thêm tại: https://ift.tt/2mb4VST để biết thêm về địa chỉ bán tai nghe không dây giá rẻ A Machine Learning Guide for Average Humans https://ift.tt/2rFyAUY xem thêm tại: https://ift.tt/2mb4VST để biết thêm về địa chỉ bán tai nghe không dây giá rẻ A Machine Learning Guide for Average Humans https://ift.tt/2rFyAUY xem thêm tại: https://ift.tt/2mb4VST để biết thêm về địa chỉ bán tai nghe không dây giá rẻ A Machine Learning Guide for Average Humans https://ift.tt/2rFyAUY xem thêm tại: https://ift.tt/2mb4VST để biết thêm về địa chỉ bán tai nghe không dây giá rẻ A Machine Learning Guide for Average Humans https://ift.tt/2rFyAUY xem thêm tại: https://ift.tt/2mb4VST để biết thêm về địa chỉ bán tai nghe không dây giá rẻ A Machine Learning Guide for Average Humans https://ift.tt/2rFyAUY xem thêm tại: https://ift.tt/2mb4VST để biết thêm về địa chỉ bán tai nghe không dây giá rẻ A Machine Learning Guide for Average Humans https://ift.tt/2rFyAUY xem thêm tại: https://ift.tt/2mb4VST để biết thêm về địa chỉ bán tai nghe không dây giá rẻ A Machine Learning Guide for Average Humans https://ift.tt/2rFyAUY xem thêm tại: https://ift.tt/2mb4VST để biết thêm về địa chỉ bán tai nghe không dây giá rẻ A Machine Learning Guide for Average Humans https://ift.tt/2rFyAUY xem thêm tại: https://ift.tt/2mb4VST để biết thêm về địa chỉ bán tai nghe không dây giá rẻ A Machine Learning Guide for Average Humans https://ift.tt/2rFyAUY xem thêm tại: https://ift.tt/2mb4VST để biết thêm về địa chỉ bán tai nghe không dây giá rẻ A Machine Learning Guide for Average Humans https://ift.tt/2rFyAUY xem thêm tại: https://ift.tt/2mb4VST để biết thêm về địa chỉ bán tai nghe không dây giá rẻ A Machine Learning Guide for Average Humans https://ift.tt/2rFyAUY xem thêm tại: https://ift.tt/2mb4VST để biết thêm về địa chỉ bán tai nghe không dây giá rẻ A Machine Learning Guide for Average Humans https://ift.tt/2rFyAUY xem thêm tại: https://ift.tt/2mb4VST để biết thêm về địa chỉ bán tai nghe không dây giá rẻ A Machine Learning Guide for Average Humans https://ift.tt/2rFyAUY xem thêm tại: https://ift.tt/2mb4VST để biết thêm về địa chỉ bán tai nghe không dây giá rẻ A Machine Learning Guide for Average Humans https://ift.tt/2rFyAUY xem thêm tại: https://ift.tt/2mb4VST để biết thêm về địa chỉ bán tai nghe không dây giá rẻ A Machine Learning Guide for Average Humans https://ift.tt/2rFyAUY xem thêm tại: https://ift.tt/2mb4VST để biết thêm về địa chỉ bán tai nghe không dây giá rẻ A Machine Learning Guide for Average Humans https://ift.tt/2rFyAUY xem thêm tại: https://ift.tt/2mb4VST để biết thêm về địa chỉ bán tai nghe không dây giá rẻ A Machine Learning Guide for Average Humans https://ift.tt/2rFyAUY xem thêm tại: https://ift.tt/2mb4VST để biết thêm về địa chỉ bán tai nghe không dây giá rẻ A Machine Learning Guide for Average Humans https://ift.tt/2rFyAUY xem thêm tại: https://ift.tt/2mb4VST để biết thêm về địa chỉ bán tai nghe không dây giá rẻ A Machine Learning Guide for Average Humans https://ift.tt/2rFyAUY xem thêm tại: https://ift.tt/2mb4VST để biết thêm về địa chỉ bán tai nghe không dây giá rẻ A Machine Learning Guide for Average Humans https://ift.tt/2rFyAUY xem thêm tại: https://ift.tt/2mb4VST để biết thêm về địa chỉ bán tai nghe không dây giá rẻ A Machine Learning Guide for Average Humans https://ift.tt/2rFyAUY xem thêm tại: https://ift.tt/2mb4VST để biết thêm về địa chỉ bán tai nghe không dây giá rẻ A Machine Learning Guide for Average Humans https://ift.tt/2rFyAUY xem thêm tại: https://ift.tt/2mb4VST để biết thêm về địa chỉ bán tai nghe không dây giá rẻ A Machine Learning Guide for Average Humans https://ift.tt/2rFyAUY xem thêm tại: https://ift.tt/2mb4VST để biết thêm về địa chỉ bán tai nghe không dây giá rẻ A Machine Learning Guide for Average Humans https://ift.tt/2rFyAUY xem thêm tại: https://ift.tt/2mb4VST để biết thêm về địa chỉ bán tai nghe không dây giá rẻ A Machine Learning Guide for Average Humans https://ift.tt/2rFyAUY xem thêm tại: https://ift.tt/2mb4VST để biết thêm về địa chỉ bán tai nghe không dây giá rẻ A Machine Learning Guide for Average Humans https://ift.tt/2rFyAUY xem thêm tại: https://ift.tt/2mb4VST để biết thêm về địa chỉ bán tai nghe không dây giá rẻ A Machine Learning Guide for Average Humans https://ift.tt/2rFyAUY xem thêm tại: https://ift.tt/2mb4VST để biết thêm về địa chỉ bán tai nghe không dây giá rẻ A Machine Learning Guide for Average Humans https://ift.tt/2rFyAUY xem thêm tại: https://ift.tt/2mb4VST để biết thêm về địa chỉ bán tai nghe không dây giá rẻ A Machine Learning Guide for Average Humans https://ift.tt/2rFyAUY xem thêm tại: https://ift.tt/2mb4VST để biết thêm về địa chỉ bán tai nghe không dây giá rẻ A Machine Learning Guide for Average Humans https://ift.tt/2rFyAUY xem thêm tại: https://ift.tt/2mb4VST để biết thêm về địa chỉ bán tai nghe không dây giá rẻ A Machine Learning Guide for Average Humans https://ift.tt/2rFyAUY xem thêm tại: https://ift.tt/2mb4VST để biết thêm về địa chỉ bán tai nghe không dây giá rẻ A Machine Learning Guide for Average Humans https://ift.tt/2rFyAUY xem thêm tại: https://ift.tt/2mb4VST để biết thêm về địa chỉ bán tai nghe không dây giá rẻ A Machine Learning Guide for Average Humans https://ift.tt/2rFyAUY xem thêm tại: https://ift.tt/2mb4VST để biết thêm về địa chỉ bán tai nghe không dây giá rẻ A Machine Learning Guide for Average Humans https://ift.tt/2rFyAUY xem thêm tại: https://ift.tt/2mb4VST để biết thêm về địa chỉ bán tai nghe không dây giá rẻ A Machine Learning Guide for Average Humans https://ift.tt/2rFyAUY xem thêm tại: https://ift.tt/2mb4VST để biết thêm về địa chỉ bán tai nghe không dây giá rẻ A Machine Learning Guide for Average Humans https://ift.tt/2rFyAUY xem thêm tại: https://ift.tt/2mb4VST để biết thêm về địa chỉ bán tai nghe không dây giá rẻ A Machine Learning Guide for Average Humans https://ift.tt/2rFyAUY xem thêm tại: https://ift.tt/2mb4VST để biết thêm về địa chỉ bán tai nghe không dây giá rẻ A Machine Learning Guide for Average Humans https://ift.tt/2rFyAUY xem thêm tại: https://ift.tt/2mb4VST để biết thêm về địa chỉ bán tai nghe không dây giá rẻ A Machine Learning Guide for Average Humans https://ift.tt/2rFyAUY xem thêm tại: https://ift.tt/2mb4VST để biết thêm về địa chỉ bán tai nghe không dây giá rẻ A Machine Learning Guide for Average Humans https://ift.tt/2rFyAUY xem thêm tại: https://ift.tt/2mb4VST để biết thêm về địa chỉ bán tai nghe không dây giá rẻ A Machine Learning Guide for Average Humans https://ift.tt/2rFyAUY xem thêm tại: https://ift.tt/2mb4VST để biết thêm về địa chỉ bán tai nghe không dây giá rẻ A Machine Learning Guide for Average Humans https://ift.tt/2rFyAUY xem thêm tại: https://ift.tt/2mb4VST để biết thêm về địa chỉ bán tai nghe không dây giá rẻ A Machine Learning Guide for Average Humans https://ift.tt/2rFyAUY xem thêm tại: https://ift.tt/2mb4VST để biết thêm về địa chỉ bán tai nghe không dây giá rẻ A Machine Learning Guide for Average Humans https://ift.tt/2rFyAUY xem thêm tại: https://ift.tt/2mb4VST để biết thêm về địa chỉ bán tai nghe không dây giá rẻ A Machine Learning Guide for Average Humans https://ift.tt/2rFyAUY xem thêm tại: https://ift.tt/2mb4VST để biết thêm về địa chỉ bán tai nghe không dây giá rẻ A Machine Learning Guide for Average Humans https://ift.tt/2rFyAUY xem thêm tại: https://ift.tt/2mb4VST để biết thêm về địa chỉ bán tai nghe không dây giá rẻ A Machine Learning Guide for Average Humans https://ift.tt/2rFyAUY xem thêm tại: https://ift.tt/2mb4VST để biết thêm về địa chỉ bán tai nghe không dây giá rẻ A Machine Learning Guide for Average Humans https://ift.tt/2rFyAUY xem thêm tại: https://ift.tt/2mb4VST để biết thêm về địa chỉ bán tai nghe không dây giá rẻ A Machine Learning Guide for Average Humans https://ift.tt/2rFyAUY xem thêm tại: https://ift.tt/2mb4VST để biết thêm về địa chỉ bán tai nghe không dây giá rẻ A Machine Learning Guide for Average Humans https://ift.tt/2rFyAUY xem thêm tại: https://ift.tt/2mb4VST để biết thêm về địa chỉ bán tai nghe không dây giá rẻ A Machine Learning Guide for Average Humans https://ift.tt/2rFyAUY xem thêm tại: https://ift.tt/2mb4VST để biết thêm về địa chỉ bán tai nghe không dây giá rẻ A Machine Learning Guide for Average Humans https://ift.tt/2rFyAUY xem thêm tại: https://ift.tt/2mb4VST để biết thêm về địa chỉ bán tai nghe không dây giá rẻ A Machine Learning Guide for Average Humans https://ift.tt/2rFyAUY xem thêm tại: https://ift.tt/2mb4VST để biết thêm về địa chỉ bán tai nghe không dây giá rẻ A Machine Learning Guide for Average Humans https://ift.tt/2rFyAUY xem thêm tại: https://ift.tt/2mb4VST để biết thêm về địa chỉ bán tai nghe không dây giá rẻ A Machine Learning Guide for Average Humans https://ift.tt/2rFyAUY xem thêm tại: https://ift.tt/2mb4VST để biết thêm về địa chỉ bán tai nghe không dây giá rẻ A Machine Learning Guide for Average Humans https://ift.tt/2rFyAUY xem thêm tại: https://ift.tt/2mb4VST để biết thêm về địa chỉ bán tai nghe không dây giá rẻ A Machine Learning Guide for Average Humans https://ift.tt/2rFyAUY xem thêm tại: https://ift.tt/2mb4VST để biết thêm về địa chỉ bán tai nghe không dây giá rẻ A Machine Learning Guide for Average Humans https://ift.tt/2rFyAUY xem thêm tại: https://ift.tt/2mb4VST để biết thêm về địa chỉ bán tai nghe không dây giá rẻ A Machine Learning Guide for Average Humans https://ift.tt/2rFyAUY xem thêm tại: https://ift.tt/2mb4VST để biết thêm về địa chỉ bán tai nghe không dây giá rẻ A Machine Learning Guide for Average Humans https://ift.tt/2rFyAUY xem thêm tại: https://ift.tt/2mb4VST để biết thêm về địa chỉ bán tai nghe không dây giá rẻ A Machine Learning Guide for Average Humans https://ift.tt/2rFyAUY xem thêm tại: https://ift.tt/2mb4VST để biết thêm về địa chỉ bán tai nghe không dây giá rẻ A Machine Learning Guide for Average Humans https://ift.tt/2rFyAUY xem thêm tại: https://ift.tt/2mb4VST để biết thêm về địa chỉ bán tai nghe không dây giá rẻ A Machine Learning Guide for Average Humans https://ift.tt/2rFyAUY xem thêm tại: https://ift.tt/2mb4VST để biết thêm về địa chỉ bán tai nghe không dây giá rẻ A Machine Learning Guide for Average Humans https://ift.tt/2rFyAUY Bạn có thể xem thêm địa chỉ mua tai nghe không dây tại đây https://ift.tt/2mb4VST
0 notes
Text
A Machine Learning Guide for Average Humans
A Machine Learning Guide for Average Humans
Posted by alexis-sanders
//<![CDATA[ (function($) { // code using $ as alias to jQuery $(function() { // Hide the hypotext content. $('.hypotext-content').hide(); // When a hypotext link is clicked. $('a.hypotext.closed').click(function (e) { // custom handling here e.preventDefault(); // Create the class reference from the rel value. var id = '.' + $(this).attr('rel'); // If the content is hidden, show it now. if ( $(id).css('display') == 'none' ) { $(id).show('slow'); if (jQuery.ui) { // UI loaded $(id).effect("highlight", {}, 1000); } } // If the content is shown, hide it now. else { $(id).hide('slow'); } }); // If we have a hash value in the url. if (window.location.hash) { // If the anchor is within a hypotext block, expand it, by clicking the // relevant link. console.log(window.location.hash); var anchor = $(window.location.hash); var hypotextLink = $('#' + anchor.parents('.hypotext-content').attr('rel')); console.log(hypotextLink); hypotextLink.click(); // Wait until the content has expanded before jumping to anchor. //$.delay(1000); setTimeout(function(){ scrollToAnchor(window.location.hash); }, 1000); } }); function scrollToAnchor(id) { var anchor = $(id); $('html,body').animate({scrollTop: anchor.offset().top},'slow'); } })(jQuery); //]]>
Machine learning (ML) has grown consistently in worldwide prevalence. Its implications have stretched from small, seemingly inconsequential victories to groundbreaking discoveries. The SEO community is no exception. An understanding and intuition of machine learning can support our understanding of the challenges and solutions Google's engineers are facing, while also opening our minds to ML's broader implications.
The advantages of gaining an general understanding of machine learning include:
Gaining empathy for engineers, who are ultimately trying to establish the best results for users
Understanding what problems machines are solving for, their current capabilities and scientists' goals
Understanding the competitive ecosystem and how companies are using machine learning to drive results
Preparing oneself for for what many industry leaders call a major shift in our society (Andrew Ng refers to AI as a "new electricity")
Understanding basic concepts that often appear within research (it's helped me with understanding certain concepts that appear within Google Brain's Research)
Growing as an individual and expanding your horizons (you might really enjoy machine learning!)
When code works and data is produced, it's a very fulfilling, empowering feeling (even if it's a very humble result)
I spent a year taking online courses, reading books, and learning about learning (...as a machine). This post is the fruit borne of that labor -- it covers 17 machine learning resources (including online courses, books, guides, conference presentations, etc.) comprising the most affordable and popular machine learning resources on the web (through the lens of a complete beginner). I've also added a summary of "If I were to start over again, how I would approach it."
This article isn't about credit or degrees. It's about regular Joes and Joannas with an interest in machine learning, and who want to spend their learning time efficiently. Most of these resources will consume over 50 hours of commitment. Ain't nobody got time for a painful waste of a work week (especially when this is probably completed during your personal time). The goal here is for you to find the resource that best suits your learning style. I genuinely hope you find this research useful, and I encourage comments on which materials prove most helpful (especially ones not included)! #HumanLearningMachineLearning
Executive summary:
Here's everything you need to know in a chart:
Machine Learning Resource
Time (hours)
Cost ($)
Year
Credibility
Code
Math
Enjoyability
Jason Maye's Machine Learning 101 slidedeck: 2 years of headbanging, so you don't have to
2
$0
'17
{ML} Recipes with Josh Gordon Playlist
2
$0
'16
Machine Learning Crash Course
15
$0
'18
OCDevel Machine Learning Guide Podcast
30
$0
'17-
Kaggle's Machine Learning Track (part 1)
6
$0
'17
Fast.ai (part 1)
70
$70*
'16
Hands-On Machine Learning with Scikit-Learn and TensorFlow: Concepts, Tools, and Techniques to Build Intelligent Systems
20
$25
'17
Udacity's Intro to Machine Learning (Kate/Sebastian)
60
$0
'15
Andrew Ng's Coursera Machine Learning
55
$0
'11
iPullRank Machine Learning Guide
3
$0
'17
Review Google PhD
2
$0
'17
Caltech Machine Learning on iTunes
27
$0
'12
Pattern Recognition & Machine Learning by Christopher Bishop
150
$75
'06
N/A
Machine Learning: Hands-on for Developers and Technical Professionals
15
$50
'15
Introduction to Machine Learning with Python: A Guide for Data Scientists
15
$25
'16
Udacity's Machine Learning by Georgia Tech
96
$0
'15
Machine Learning Stanford iTunes by Andrew Ng
25
$0
'08
N/A
*Free, but there is the cost of running an AWS EC2 instance (~$70 when I finished, but I did tinker a ton and made a Rick and Morty script generator, which I ran many epochs [rounds] of...)
Here's my suggested program:
1. Starting out (estimated 60 hours)
Start with shorter content targeting beginners. This will allow you to get the gist of what's going on with minimal time commitment.
Commit three hours to Jason Maye's Machine Learning 101 slidedeck: 2 years of headbanging, so you don't have to.
Commit two hours to watch Google's {ML} Recipes with Josh Gordon YouTube Playlist.
Sign up for Sam DeBrule's Machine Learnings newsletter.
Work through Google's Machine Learning Crash Course.
Start listening to OCDevel's Machine Learning Guide Podcast (skip episodes 1, 3, 16, 21, and 26) in your car, working out, and/or when using hands and eyes for other activities.
Commit two days to working through Kaggle's Machine Learning Track part 1.
2. Ready to commit (estimated 80 hours)
By this point, learners would understand their interest levels. Continue with content focused on applying relevant knowledge as fast as possible.
Commit to Fast.ai 10 hours per week, for 7 weeks. If you have a friend/mentor that can help you work through AWS setup, definitely lean on any support in installation (it's 100% the worst part of ML).
Acquire Hands-On Machine Learning with Scikit-Learn and TensorFlow: Concepts, Tools, and Techniques to Build Intelligent Systems, and read the first two chapters immediately. Then use this as supplemental to the Fast.ai course.
3. Broadening your horizons (estimated 115 hours)
If you've made it through the last section and are still hungry for more knowledge, move on to broadening your horizons. Read content focused on teaching the breadth of machine learning -- building an intuition for what the algorithms are trying to accomplish (whether visual or mathematically).
Start watching videos and participating in Udacity's Intro to Machine Learning (by Sebastian Thrun and Katie Malone).
Work through Andrew Ng's Coursera Machine Learning course.
Your next steps
By this point, you will already have AWS running instances, a mathematical foundation, and an overarching view of machine learning. This is your jumping-off point to determine what you want to do.
You should be able to determine your next step based on your interest, whether it's entering Kaggle competitions; doing Fast.ai part two; diving deep into the mathematics with Pattern Recognition & Machine Learning by Christopher Bishop; giving Andrew Ng's newer Deeplearning.ai course on Coursera; learning more about specific tech stacks (TensorFlow, Scikit-Learn, Keras, Pandas, Numpy, etc.); or applying machine learning to your own problems.
Why am I recommending these steps and resources?
I am not qualified to write an article on machine learning. I don't have a PhD. I took one statistics class in college, which marked the first moment I truly understood "fight or flight" reactions. And to top it off, my coding skills are lackluster (at their best, they're chunks of reverse-engineered code from Stack Overflow). Despite my many shortcomings, this piece had to be written by someone like me, an average person.
Statistically speaking, most of us are average (ah, the bell curve/Gaussian distribution always catches up to us). Since I'm not tied to any elitist sentiments, I can be real with you. Below contains a high-level summary of my reviews on all of the classes I took, along with a plan for how I would approach learning machine learning if I could start over. Click to expand each course for the full version with notes.
In-depth reviews of machine learning courses:
Starting out
Jason Maye's Machine Learning 101 slidedeck: 2 years of head-banging, so you don't have to ↓
Need to Know: A stellar high-level overview of machine learning fundamentals in an engaging and visually stimulating format.
Loved:
Very user-friendly, engaging, and playful slidedeck.
Has the potential to take some of the pain out of the process, through introducing core concepts.
Breaks up content by beginner/need-to-know (green), and intermediate/less-useful noise (specifically for individuals starting out) (blue).
Provides resources to dive deeper into machine learning.
Provides some top people to follow in machine learning.
Disliked:
That there is not more! Jason's creativity, visual-based teaching approach, and quirky sense of humor all support the absorption of the material.
Lecturer:
Jason Mayes:
Senior Creative Technologist and Research Engineer at Google
Masters in Computer Science from University of Bristols
Personal Note: He's also kind on Twitter! :)
Links:
Machine Learning 101 slide deck
Tips on Watching:
Set aside 2-4 hours to work through the deck once.
Since there is a wealth of knowledge, refer back as needed (or as a grounding source).
Identify areas of interest and explore the resources provided.
{ML} Recipes with Josh Gordon ↓
Need to Know: This mini-series YouTube-hosted playlist covers the very fundamentals of machine learning with opportunities to complete exercises.
Loved:
It is genuinely beginner-focused.
They make no assumption of any prior knowledge.
Gloss over potentially complex topics that may serve as noise.
Playlist ~2 hours
Very high-quality filming, audio, and presentation, almost to the point where it had its own aesthetic.
Covers some examples in scikit-learn and TensorFlow, which felt modern and practical.
Josh Gordon was an engaging speaker.
Disliked:
I could not get Dockers on Windows (suggested package manager). This wasn't a huge deal, since I already had my AWS setup by this point; however, a bit of a bummer since it made it impossible to follow certain steps exactly.
Issue: Every time I tried to download (over the course of two weeks), the .exe file would recursively start and keep spinning until either my memory ran out, computer crashed, or I shut my computer down. I sent this to Docker's Twitter account to no avail.
Lecturer:
Josh Gordon:
Developer Advocate for at TensorFlow at Google
Leads Machine Learning advocacy at Google
Member of the Udacity AI & Data Industry Advisory Board
Masters in Computer Science from Columbia University
Links:
Hello World - Machine Learning Recipes #1 (YouTube)
GitHub: Machine Learning Recipes with Josh Gordon
Tips on Watching:
The playlist is short (only ~1.5 hours screen time). However, it can be a bit fast-paced at times (especially if you like mimicking the examples), so set aside 3-4 hours to play around with examples and allow time for installation, pausing, and following along.
Take time to explore code labs.
Google's Machine Learning Crash Course with TensorFlow APIs ↓
Need to Know: A Google researcher-made crash course on machine learning that is interactive and offers its own built-in coding system!
Loved:
Different formats of learning: high-quality video (with ability to adjust speed, closed captioning), readings, quizzes (with explanations), visuals (including whiteboarding), interactive components/ playgrounds, code lab exercises (run directly in your browser (no setup required!))
Non-intimidating
One of my favorite quotes: "You don't need to understand the math to be able to take a look at the graphical interpretation."
Broken down into digestible sections
Introduces key terms
Disliked:
N/A
Lecturers:
Multiple Google researchers participated in this course, including:
Peter Norvig
Director of Research at Google Inc.
Previously he directed Google's core search algorithms group.
He is co-author of Artificial Intelligence: A Modern Approach
D. Sculley
Senior Staff Software Engineer at Google
KDD award-winning papers
Works on massive-scale ML systems for online advertising
Was part of a research ML paper on optimizing chocolate chip cookies
According to his personal website, he prefers to go by "D."
Cassandra Xia
Programmer, Software Engineer at Google
She has some really cool (and cute) projects based on learning statistics concepts interactively
Maya Gupta
Leads Glassbox Machine Learning R&D team at Google
Associate Professor of Electrical Engineering at the University of Washington (2003-2012)
In 2007, Gupta received the PECASE award from President George Bush for her work in classifying uncertain (e.g. random) signals
Gupta also runs Artifact Puzzles, the second-largest US maker of wooden jigsaw puzzles
Sally Goldman
Research Scientist at Google
Co-author of A Practical Guide to Data Structures and Algorithms Using Java
Numerous journals, classes taught at Washington University, and contributions to the ML community
Links:
Machine Learning Crash Course
Tips on Doing:
Actively work through playground and coding exercises
OCDevel's Machine Learning Guide Podcast ↓
Need to Know: This podcast focuses on the high-level fundamentals of machine learning, including basic intuition, algorithms, math, languages, and frameworks. It also includes references to learn more on each episode's topic.
Loved:
Great for trips (when traveling a ton, it was an easy listen).
The podcast makes machine learning fun with interesting and compelling analogies.
Tyler is a big fan of Andrew Ng's Coursera course and reviews concepts in Coursera course very well, such that both pair together nicely.
Covers the canonical resources for learning more on a particular topic.
Disliked:
Certain courses were more theory-based; all are interesting, yet impractical.
Due to limited funding the project is a bit slow to update and has less than 30 episodes.
Podcaster:
Tyler Renelle:
Machine learning engineer focused on time series and reinforcement
Background in full-stack JavaScript, 10 years web and mobile
Creator of HabitRPG, an app that treats habits as an RPG game
Links:
Machine Learning Guide podcast
Machine Learning Guide podcast (iTunes)
Tips on Listening:
Listen along your journey to help solidify understanding of topics.
Skip episodes 1, 3, 16, 21, and 26 (unless their topics interest and inspire you!).
Kaggle Machine Learning Track (Lesson 1) ↓
Need to Know: A simple code lab that covers the very basics of machine learning with scikit-learn and Panda through the application of the examples onto another set of data.
Loved:
A more active form of learning.
An engaging code lab that encourages participants to apply knowledge.
This track offers has a built-in Python notebook on Kaggle with all input files included. This removed any and all setup/installation issues.
Side note: It's a bit different than Jupyter notebook (e.g., have to click into a cell to add another cell).
Each lesson is short, which made the entire lesson go by very fast.
Disliked:
The writing in the first lesson didn't initially make it clear that one would need to apply the knowledge in the lesson to their workbook.
It wasn't a big deal, but when I started referencing files in the lesson, I had to dive into the files in my workbook to find they didn't exist, only to realize that the knowledge was supposed to be applied and not transcribed.
Lecturer:
Dan Becker:
Data Scientist at Kaggle
Undergrad in Computer Science, PhD in Econometrics
Supervised data science consultant for six Fortune 100 companies
Contributed to the Keras and Tensorflow libraries
Finished 2nd (out of 1353 teams) in $3 million Heritage Health Prize data mining competition
Speaks at deep learning workshops at events and conferences
Links:
https://www.kaggle.com/learn/machine-learning
Tips on Doing:
Read the exercises and apply to your dataset as you go.
Try lesson 2, which covers more complex/abstract topics (note: this second took a bit longer to work through).
Ready to commit
Fast.ai (part 1 of 2) ↓
Need to Know: Hands-down the most engaging and active form of learning ML. The source I would most recommend for anyone (although the training plan does help to build up to this course). This course is about learning through coding. This is the only course that I started to truly see the practical mechanics start to come together. It involves applying the most practical solutions to the most common problems (while also building an intuition for those solutions).
Loved:
Course Philosophy:
Active learning approach
"Go out into the world and understand underlying mechanics (of machine learning by doing)."
Counter-culture to the exclusivity of the machine learning field, focusing on inclusion.
"Let's do shit that matters to people as quickly as possible."
Highly pragmatic approach with tools that are currently being used (Jupyter Notebooks, scikit-learn, Keras, AWS, etc.).
Show an end-to-end process that you get to complete and play with in a development environment.
Math is involved, but is not prohibitive. Excel files helped to consolidate information/interact with information in a different way, and Jeremy spends a lot of time recapping confusing concepts.
Amazing set of learning resources that allow for all different styles of learning, including:
Video Lessons
Notes
Jupyter Notebooks
Assignments
Highly active forums
Resources on Stackoverflow
Readings/resources
Jeremy often references popular academic texts
Jeremy's TEDx talk in Brussels
Jeremy really pushes one to do extra and put in the effort by teaching interesting problems and engaging one in solving them.
It's a huge time commitment; however, it's worth it.
All of the course's profits are donated.
Disliked:
Overview covers their approach to learning (obviously I'm a fan!). If you're already drinking the Kool-aid, skip past.
I struggled through the AWS setup (13-minute video) for about five hours (however, it felt so good when it was up and running!).
Because of its practicality and concentration on solutions used today to solve popular problem types (image recognition, text generation, etc.), it lacks breadth of machine learning topics.
Lecturers:
Jeremy Howard:
Distinguished Research Scientist at the University of San Francisco
Faculty member at Singularity University
Young Global Leader with the World Economic Forum
Founder of Enlitic (the first company to apply deep learning to medicine)
Former President and Chief Scientist of the data science platform Kaggle
Rachel Thomas:
PhD in Math from Duke
One of Forbes' "20 Incredible Women Advancing AI Research"
Researcher-in-residence at the University of San Francisco Data Institute
Teaches in the Masters in Data Science program
Links:
http://course.fast.ai/start.html
http://wiki.fast.ai/index.php/Main_Page
https://github.com/fastai/courses/tree/master/deeplearning1/nbs
Tips on Doing:
Set expectations with yourself that installation is going to probably take a few hours.
Prepare to spend about ~70 hours for this course (it's worth it).
Don't forget to shut off your AWS instance.
Balance out machine learning knowledge with a course with more breadth.
Consider giving part two of the Fast.ai program a shot!
Hands-On Machine Learning with Scikit-Learn and TensorFlow: Concepts, Tools, and Techniques to Build Intelligent Systems ↓
Need to Know: This book is an Amazon best seller for a reason. It covers a lot of ground quickly, empowers readers to walk through a machine learning problem by chapter two, and contains practical up-to-date machine learning skills.
Loved:
Book contains an amazing introduction to machine learning that briskly provides an overarching quick view of the machine learning ecosystem.
Chapter 2 immediately walks the reader through an end-to-end machine learning problem.
Immediately afterwards, Aurélien pushes a user to attempt to apply this solution to another problem, which was very empowering.
There are review questions at the end of each chapter to ensure on has grasped the content within the chapter and to push the reader to explore more.
Once installation was completed, it was easy to follow and all code is available on GitHub.
Chapters 11-14 were very tough reading; however, they were a great reference when working through Fast.ai.
Contains some powerful analogies.
Each chapter's introductions were very useful and put everything into context. This general-to-specifics learning was very useful.
Disliked:
Installation was a common source of issues during the beginning of my journey; the text glided over this. I felt the frustration that most people experience from installation should have been addressed with more resources.
Writer:
Aurélien Géron:
Led the YouTube video classification team from 2013 to 2016
Currently a machine Learning consultant
Founder and CTO of Wifirst and Polyconseil
Published technical books (on C++, Wi-Fi, and Internet architectures)
Links:
https://www.amazon.com/_/dp/1491962291?tag=oreilly20-20
http://shop.oreilly.com/product/0636920052289.do
https://github.com/ageron/handson-ml
Tips on Using:
Get a friend with Python experience to help with installation.
Read the introductions to each chapter thoroughly, read the chapter (pay careful attention to code), review the questions at the end (highlight any in-text answer), make a copy of Aurélien's GitHub and make sure everything works on your setup, re-type the notebooks, go to Kaggle and try on other datasets.
Broadening your horizons
Udacity: Intro to Machine Learning (Kate/Sebastian) ↓
Need to Know: A course that covers a range of machine learning topics, supports building of intuition via visualization and simple examples, offers coding challenges, and a certificate (upon completion of a final project). The biggest challenge with this course is bridging the gap between the hand-holding lectures and the coding exercises.
Loved:
Focus on developing a visual intuition on what each model is trying to accomplish.
This visual learning mathematics approach is very useful.
Cover a vast variety and breadth of models and machine learning basics.
In terms of presenting the concept, there was a lot of hand-holding (which I completely appreciated!).
Many people have done this training, so their GitHub accounts can be used as reference for the mini-projects.
Katie actively notes documentation and suggests where viewers can learn more/reference material.
Disliked:
All of the conceptual hand-holding in the lessons is a stark contrast to the challenges of installation, coding exercises, and mini-projects.
This is the first course started and the limited instructions on setting up the environment and many failed attempts caused me to break down crying at least a handful of times.
The mini-projects are intimidating.
There is extra code added to support the viewers; however, it's done so with little acknowledgement as to what it's actually doing. This made learning a bit harder.
Lecturer:
Caitlin (Katie) Malone:
Director of Data Science Research and Development at Civis Analytics
Stanford PhD in Experimental Particle Physics
Intern at Udacity in summer 2014
Graduate Researcher at the SLAC National Accelerator Laboratory
https://www6.slac.stanford.edu/
Podcaster with Ben Jaffe (currently Facebook UI Engineer and a music aficionado) on a machine learning podcast Linear Digressions (100+ episodes)
Sebastian Thrun:
CEO of the Kitty Hawk Corporation
Chairman and co-founder of Udacity
One of my favorite Sebastian quotes: "It occurred to me, I could be at Google and build a self-driving car, or I can teach 10,000 students how to build self-driving cars."
Former Google VP
Founded Google X
Led development of the robotic vehicle Stanley
Professor of Computer Science at Stanford University
Formerly a professor at Carnegie Mellon University.
Links:
https://www.udacity.com/course/intro-to-machine-learning--ud120
Udacity also offers a next step, the Machine Learning Engineer Nanodegree, which will set one back about $1K.
Tips on Watching:
Get a friend to help you set up your environment.
Print mini-project instructions to check off each step.
Andrew Ng's Coursera Machine Learning Course ↓
Need to Know: The Andrew Ng Coursera course is the most referenced online machine learning course. It covers a broad set of fundamental, evergreen topics with a strong focus in building mathematical intuition behind machine learning models. Also, one can submit assignments and earn a grade for free. If you want to earn a certificate, one can subscribe or apply for financial aid.
Loved:
This course has a high level of credibility.
Introduces all necessary machine learning terminology and jargon.
Contains a very classic machine learning education approach with a high level of math focus.
Quizzes interspersed in courses and after each lesson support understanding and overall learning.
The sessions for the course are flexible, the option to switch into a different section is always available.
Disliked:
The mathematic notation was hard to process at times.
The content felt a bit dated and non-pragmatic. For example, the main concentration was MATLAB and Octave versus more modern languages and resources.
Video quality was less than average and could use a refresh.
Lecturer:
Andrew Ng:
Adjunct Professor, Stanford University (focusing on AI, Machine Learning, and Deep Learning)
Co-founder of Coursera
Former head of Baidu AI Group
Founder and previous head of Google Brain (deep learning) project
Former Director of the Stanford AI Lab
Chairman of the board of Woebot (a machine learning bot that focuses on Cognitive Behavior Therapy)
Links:
https://www.coursera.org/learn/machine-learning/
Andrew Ng recently launched a new course (August 2017) called DeepLearning.ai, a ~15 week course containing five mini-courses ($49 USD per month to continue learning after trial period of 7 days ends).
Course: https://www.coursera.org/specializations/deep-learning
Course 1: Neural Networks and Deep Learning
Course 2: Improving Deep Neural Networks: Hyperparameter tuning, Regularization and Optimization
Course 3: Structuring Machine Learning Projects
Course 4: Convolutional Neural Networks
Course 5: Sequence Models
Tips on Watching:
Be disciplined with setting aside timing (even if it's only 15 minutes a day) to help power through some of the more boring concepts.
Don't do this course first, because it's intimidating, requires a large time commitment, and isn't a very energizing experience.
Additional machine learning opportunities
iPullRank Machine Learning Guide ↓
Need to Know: A machine learning e-book targeted at marketers.
Loved:
Targeted at marketers and applied to organic search.
Covers a variety of machine learning topics.
Some good examples, including real-world blunders.
Gives some practical tools for non-data scientists (including: MonkeyLearn and Orange)
I found Orange to be a lot of fun. It struggled with larger datasets; however, it has a very visual interface that was more user-friendly and offers potential to show some pretty compelling stories.
Example: World Happiness Dataset by:
X-axis: Happiness Score
Y-axis: Economy
Color: Health
Disliked:
Potential to break up content more with relevant imagery -- the content was very dense.
Writers:
iPullRank Team (including Mike King):
Mike King has a few slide decks on the basics of machine learnings and AI
iPullRank has a few data scientists on staff
Links:
http://ipullrank.com/machine-learning-guide/
Tips on Reading:
Read chapters 1-6 and the rest depending upon personal interest.
Review Google PhD ↓
Need to Know: A two-hour presentation from Google's 2017 IO conference that walks through getting 99% accuracy on the MNIST dataset (a famous dataset containing a bunch of handwritten numbers, which the machine must learn to identify the numbers).
Loved:
This talk struck me as very modern, covering the cutting edge.
Found this to be very complementary to Fast.ai, as it covered similar topics (e.g. ReLu, CNNs, RNNs, etc.)
Amazing visuals that help to put everything into context.
Disliked:
The presentation is only a short conference solution and not a comprehensive view of machine learning.
Also, a passive form of learning.
Presenter:
Martin Görner:
Developer Relations, Google (since 2011)
Started Mobipocket, a startup that later became the software part of the Amazon Kindle and its mobile variants
Links:
Part 1 - https://www.youtube.com/watch?v=u4alGiomYP4
Part 2 - https://www.youtube.com/watch?v=fTUwdXUFfI8
Tips on Watching:
Google any concepts you're unfamiliar with.
Take your time with this one; 2 hours of screen time doesn't count all of the Googling and processing time for this one.
Caltech Machine Learning iTunes ↓
Need to Know: If math is your thing, this course does a stellar job of building the mathematic intuition behind many machine learning models. Dr. Abu-Mostafa is a raconteur, includes useful visualizations, relevant real-world examples, and compelling analogies.
Loved:
First and foremost, this is a real Caltech course, meaning it's not a watered-down version and contains fundamental concepts that are vital to understanding the mechanics of machine learning.
On iTunes, audio downloads are available, which can be useful for on-the-go learning.
Dr. Abu-Mostafa is a skilled speaker, making the 27 hours spent listening much easier!
Dr. Abu-Mostafa offers up some strong real-world examples and analogies which makes the content more relatable.
As an example, he asks students: "Why do I give you practice exams and not just give you the final exam?" as an illustration of why a testing set is useful. If he were to just give students the final, they would just memorize the answers (i.e., they would overfit to the data) and not genuinely learn the material. The final is a test to show how much students learn.
The last 1/2 hour of the class is always a Q&A, where students can ask questions. Their questions were useful to understanding the topic more in-depth.
The video and audio quality was strong throughout. There were a few times when I couldn't understand a question in the Q&A, but overall very strong.
This course is designed to build mathematical intuition of what's going on under the hood of specific machine learning models.
Caution: Dr. Abu-Mostafa uses mathematical notation, but it's different from Andrew Ng's (e.g., theta = w).
The final lecture was the most useful, as it pulled a lot of the conceptual puzzle pieces together. The course on neural networks was a close second!
Disliked:
Although it contains mostly evergreen content, being released in 2012, it could use a refresh.
Very passive form of learning, as it wasn't immediately actionable.
Lecturer:
Dr. Yaser S. Abu-Mostafa:
Professor of Electrical Engineering and Computer Science at the California Institute of Technology
Chairman of Machine Learning Consultants LLC
Serves on a number of scientific advisory boards
Has served as a technical consultant on machine learning for several companies (including Citibank).
Multiple articles in Scientific American
Links:
https://work.caltech.edu/telecourse.html
https://itunes.apple.com/us/course/machine-learning/id515364596
Tips on Watching:
Consider listening to the last lesson first, as it pulls together the course overall conceptually. The map of the course, below, was particularly useful to organizing the information taught in the courses.
Image source: http://work.caltech.edu/slides/slides18.pdf
"Pattern Recognition & Machine Learning" by Christopher Bishop ↓
Need to Know: This is a very popular college-level machine learning textbook. I've heard it likened to a bible for machine learning. However, after spending a month trying to tackle the first few chapters, I gave up. It was too much math and pre-requisites to tackle (even with a multitude of Google sessions).
Loved:
The text of choice for many major universities, so if you can make it through this text and understand all of the concepts, you're probably in a very good position.
I appreciated the history aside sections, where Bishop talked about influential people and their career accomplishments in statistics and machine learning.
Despite being a highly mathematically text, the textbook actually has some pretty visually intuitive imagery.
Disliked:
I couldn't make it through the text, which was a bit frustrating. The statistics and mathematical notation (which is probably very benign for a student in this topic) were too much for me.
The sunk cost was pretty high here (~$75).
Writer:
Christopher Bishop:
Laboratory Director at Microsoft Research Cambridge
Professor of Computer Science at the University of Edinburgh
Fellow of Darwin College, Cambridge
PhD in Theoretical Physics from the University of Edinburgh
Links:
https://www.amazon.com/Pattern-Recognition-Learning-Information-Statistics/dp/0387310738/ref=sr_1_2?ie=UTF8&qid=1516839475&sr=8-2&keywords=Pattern+Recognition+%26+Machine+Learning
Tips on Reading:
Don't start your machine learning journey with this book.
Get a friend in statistics to walk you through anything complicated (my plan is to get a mentor in statistics).
Consider taking a (free) online statistics course (Khan Academy and Udacity both have some great content on statistics, calculus, math, and data analysis).
Machine Learning: Hands-on for Developers and Technical Professionals ↓
Need to Know: A fun, non-intimidating end-to-end launching pad/whistle stop for machine learning in action.
Loved:
Talks about practical issues that many other sources didn't really address (e.g. data-cleansing).
Covered the basics of machine learning in a non-intimidating way.
Offers abridged, consolidated versions of the content.
Added fun anecdotes that makes it easier to read.
Overall the writer has a great sense of humor.
Writer talks to the reader as if they're a real human being (i.e., doesn't expect you to go out and do proofs; acknowledges the challenge of certain concepts).
Covers a wide variety of topics.
Because it was well-written, I flew through the book (even though it's about ~300 pages).
Disliked:
N/A
Writer:
Jason Bell:
Technical architect, lecturer, and startup consultant
Data Engineer at MastodonC
Former section editor for Java Developer's Journal
Former writer on IBM DeveloperWorks
Links:
https://www.amazon.com/Machine-Learning-Hands-Developers-Professionals/dp/1118889061
https://www.wiley.com/en-us/Machine+Learning%3A+Hands+On+for+Developers+and+Technical+Professionals-p-9781118889060
Jason's Blog: https://dataissexy.wordpress.com/
Tips on Reading:
Download and explore Weka's interface beforehand.
Give some of the exercises a shot.
Introduction to Machine Learning with Python: A Guide for Data Scientists ↓
Need to Know: This was a was a well-written piece on machine learning, making it a quick read.
Loved:
Quick, smooth read.
Easy-to-follow code examples.
The first few chapters served as a stellar introduction to the basics of machine learning.
Contain subtle jokes that add a bit of fun.
Tip to use the Python package manager Anaconda with Jupyter Notebooks was helpful.
Disliked:
Once again, installation was a challenge.
The "mglearn" utility library threw me for a loop. I had to reread the first few chapters before I figured out it was support for the book.
Although I liked the book, I didn't love it. Overall it just missed the "empowering" mark.
Writers:
Andreas C. Müller:
PhD in Computer Science
Lecturer at the Data Science Institute at Columbia University
Worked at the NYU Center for Data Science on open source and open science
Former Machine Learning Scientist at Amazon
Speaks often on Machine Learning and scikit-learn (a popular machine learning library)
And he makes some pretty incredibly useful graphics, such as this scikit-learn cheat sheet:
Image source: http://peekaboo-vision.blogspot.com/2013/01/machin...
Sarah Guido:
Former senior data scientist at Mashable
Lead data scientist at Bitly
2018 SciPy Conference Data Science track co-chair
Links:
https://www.amazon.com/Introduction-Machine-Learning-Python-Scientists/dp/1449369413/ref=sr_1_7?s=books&ie=UTF8&qid=1516734322&sr=1-7&keywords=python+machine+learning
http://shop.oreilly.com/product/0636920030515.do
Tips on Reading:
Type out code examples.
Beware of the "mglearn" utility library.
Udacity: Machine Learning by Georgia Tech ↓
Need to Know: A mix between an online learning experience and a university machine learning teaching approach. The lecturers are fun, but the course still fell a bit short in terms of active learning.
Loved:
This class is offered as CS7641 at Georgia Tech, where it is a part of the Online Masters Degree. Although taking this course here will not earn credit towards the OMS degree, it's still a non-watered-down college teaching philosophy approach.
Covers a wide variety of topics, many of which reminded me of the Caltech course (including: VC Dimension versus Bayesian, Occam's razor, etc.)
Discusses Markov Decision Chains, which is something that didn't really come up in many other introductory machine learning course, but they are referenced within Google patents.
The lecturers have a great dynamic, are wicked smart, and displayed a great sense of (nerd) humor, which make the topics less intimidating.
The course has quizzes, which give the course a slight amount of interaction.
Disliked:
Some videos were very long, which made the content a bit harder to digest.
The course overall was very time consuming.
Despite the quizzes, the course was a very passive form of learning with no assignments and little coding.
Many videos started with a bunch of content already written out. Having the content written out was probably a big time-saver, but it was also a bit jarring for a viewer to see so much information all at once, while also trying to listen.
It's vital to pay very close attention to notation, which compounds in complexity quickly.
Tablet version didn't function flawlessly: some was missing content (which I had to mark down and review on a desktop), the app would crash randomly on the tablet, and sometimes the audio wouldn't start.
There were no subtitles available on tablet, which I found not only to be a major accessibility blunder, but also made it harder for me to process (since I'm not an audio learner).
Lecturer:
Michael Littman:
Professor of Computer Science at Brown University.
Was granted a patent for one of the earliest systems for Cross-language information retrieval
Perhaps the most interesting man in the world:
Been in two TEDx talks
How I Learned to Stop Worrying and Be Realistic About AI
A Cooperative Path to Artificial Intelligence
During his time at Duke, he worked on an automated crossword solver (PROVERB)
Has a Family Quartet
He has appeared in a TurboTax commercial
Charles Isbell:
Professor and Executive Associate Dean at School of Interactive Computing at Georgia Tech
Focus on statistical machine learning and "interactive" artificial intelligence.
Links:
https://www.udacity.com/course/machine-learning--ud262
Tips on Watching:
Pick specific topics of interest and focusing on those lessons.
Andrew Ng's Stanford's Machine Learning iTunes ↓
Need to Know: A non-watered-down Stanford course. It's outdated (filmed in 2008), video/audio are a bit poor, and most links online now point towards the Coursera course. Although the idea of watching a Stanford course was energizing for the first few courses, it became dreadfully boring. I made it to course six before calling it.
Loved:
Designed for students, so you know you're not missing out on anything.
This course provides a deeper study into the mathematical and theoretical foundation behind machine learning to the point that the students could create their own machine learning algorithms. This isn't necessarily very practical for the everyday machine learning user.
Has some powerful real-world examples (although they're outdated).
There is something about the kinesthetic nature of watching someone write information out. The blackboard writing helped me to process certain ideas.
Disliked:
Video and audio quality were pain to watch.
Many questions asked by students were hard to hear.
On-screen visuals range from hard to impossible to see.
Found myself counting minutes.
Dr. Ng mentions TA classes, supplementary learning, but these are not available online.
Sometimes the video showed students, which I felt was invasive.
Lecturer:
Andrew Ng (see above)
Links:
https://itunes.apple.com/us/course/machine-learning/id495053006
https://www.youtube.com/watch?v=UzxYlbK2c7E
Tips on Watching:
Only watch if you're looking to gain a deeper understanding of the math presented in the Coursera course.
Skip the first half of the first lecture, since it's mostly class logistics.
Additional Resources
Fast.ai (part 2) - free access to materials, cost for AWS EC2 instance
Deeplearning.ai - $50/month
Udacity Machine Learning Engineer Nanodegree - $1K
https://machinelearningmastery.com/
Motivations and inspiration
If you're wondering why I spent a year doing this, then I'm with you. I'm genuinely not sure why I set my sights on this project, much less why I followed through with it. I saw Mike King give a session on Machine Learning. I was caught off guard, since I knew nothing on the topic. It gave me a pesky, insatiable curiosity itch. It started with one course and then spiraled out of control. Eventually it transformed into an idea: a review guide on the most affordable and popular machine learning resources on the web (through the lens of a complete beginner). Hopefully you found it useful, or at least somewhat interesting. Be sure to share your thoughts or questions in the comments!
Sign up for The Moz Top 10, a semimonthly mailer updating you on the top ten hottest pieces of SEO news, tips, and rad links uncovered by the Moz team. Think of it as your exclusive digest of stuff you don't have time to hunt down but want to read!
xem them tai https://ift.tt/2o9GYfe A Machine Learning Guide for Average Humans xem thêm tại: https://ift.tt/2mb4VST để biết thêm về địa chỉ bán tai nghe không dây giá rẻ A Machine Learning Guide for Average Humans https://ift.tt/2rFyAUY xem thêm tại: https://ift.tt/2mb4VST để biết thêm về địa chỉ bán tai nghe không dây giá rẻ A Machine Learning Guide for Average Humans https://ift.tt/2rFyAUY xem thêm tại: https://ift.tt/2mb4VST để biết thêm về địa chỉ bán tai nghe không dây giá rẻ A Machine Learning Guide for Average Humans https://ift.tt/2rFyAUY xem thêm tại: https://ift.tt/2mb4VST để biết thêm về địa chỉ bán tai nghe không dây giá rẻ A Machine Learning Guide for Average Humans https://ift.tt/2rFyAUY xem thêm tại: https://ift.tt/2mb4VST để biết thêm về địa chỉ bán tai nghe không dây giá rẻ A Machine Learning Guide for Average Humans https://ift.tt/2rFyAUY xem thêm tại: https://ift.tt/2mb4VST để biết thêm về địa chỉ bán tai nghe không dây giá rẻ A Machine Learning Guide for Average Humans https://ift.tt/2rFyAUY xem thêm tại: https://ift.tt/2mb4VST để biết thêm về địa chỉ bán tai nghe không dây giá rẻ A Machine Learning Guide for Average Humans https://ift.tt/2rFyAUY xem thêm tại: https://ift.tt/2mb4VST để biết thêm về địa chỉ bán tai nghe không dây giá rẻ A Machine Learning Guide for Average Humans https://ift.tt/2rFyAUY xem thêm tại: https://ift.tt/2mb4VST để biết thêm về địa chỉ bán tai nghe không dây giá rẻ A Machine Learning Guide for Average Humans https://ift.tt/2rFyAUY xem thêm tại: https://ift.tt/2mb4VST để biết thêm về địa chỉ bán tai nghe không dây giá rẻ A Machine Learning Guide for Average Humans https://ift.tt/2rFyAUY xem thêm tại: https://ift.tt/2mb4VST để biết thêm về địa chỉ bán tai nghe không dây giá rẻ A Machine Learning Guide for Average Humans https://ift.tt/2rFyAUY xem thêm tại: https://ift.tt/2mb4VST để biết thêm về địa chỉ bán tai nghe không dây giá rẻ A Machine Learning Guide for Average Humans https://ift.tt/2rFyAUY xem thêm tại: https://ift.tt/2mb4VST để biết thêm về địa chỉ bán tai nghe không dây giá rẻ A Machine Learning Guide for Average Humans https://ift.tt/2rFyAUY xem thêm tại: https://ift.tt/2mb4VST để biết thêm về địa chỉ bán tai nghe không dây giá rẻ A Machine Learning Guide for Average Humans https://ift.tt/2rFyAUY xem thêm tại: https://ift.tt/2mb4VST để biết thêm về địa chỉ bán tai nghe không dây giá rẻ A Machine Learning Guide for Average Humans https://ift.tt/2rFyAUY xem thêm tại: https://ift.tt/2mb4VST để biết thêm về địa chỉ bán tai nghe không dây giá rẻ A Machine Learning Guide for Average Humans https://ift.tt/2rFyAUY xem thêm tại: https://ift.tt/2mb4VST để biết thêm về địa chỉ bán tai nghe không dây giá rẻ A Machine Learning Guide for Average Humans https://ift.tt/2rFyAUY xem thêm tại: https://ift.tt/2mb4VST để biết thêm về địa chỉ bán tai nghe không dây giá rẻ A Machine Learning Guide for Average Humans https://ift.tt/2rFyAUY xem thêm tại: https://ift.tt/2mb4VST để biết thêm về địa chỉ bán tai nghe không dây giá rẻ A Machine Learning Guide for Average Humans https://ift.tt/2rFyAUY xem thêm tại: https://ift.tt/2mb4VST để biết thêm về địa chỉ bán tai nghe không dây giá rẻ A Machine Learning Guide for Average Humans https://ift.tt/2rFyAUY xem thêm tại: https://ift.tt/2mb4VST để biết thêm về địa chỉ bán tai nghe không dây giá rẻ A Machine Learning Guide for Average Humans https://ift.tt/2rFyAUY xem thêm tại: https://ift.tt/2mb4VST để biết thêm về địa chỉ bán tai nghe không dây giá rẻ A Machine Learning Guide for Average Humans https://ift.tt/2rFyAUY xem thêm tại: https://ift.tt/2mb4VST để biết thêm về địa chỉ bán tai nghe không dây giá rẻ A Machine Learning Guide for Average Humans https://ift.tt/2rFyAUY xem thêm tại: https://ift.tt/2mb4VST để biết thêm về địa chỉ bán tai nghe không dây giá rẻ A Machine Learning Guide for Average Humans https://ift.tt/2rFyAUY xem thêm tại: https://ift.tt/2mb4VST để biết thêm về địa chỉ bán tai nghe không dây giá rẻ A Machine Learning Guide for Average Humans https://ift.tt/2rFyAUY xem thêm tại: https://ift.tt/2mb4VST để biết thêm về địa chỉ bán tai nghe không dây giá rẻ A Machine Learning Guide for Average Humans https://ift.tt/2rFyAUY xem thêm tại: https://ift.tt/2mb4VST để biết thêm về địa chỉ bán tai nghe không dây giá rẻ A Machine Learning Guide for Average Humans https://ift.tt/2rFyAUY xem thêm tại: https://ift.tt/2mb4VST để biết thêm về địa chỉ bán tai nghe không dây giá rẻ A Machine Learning Guide for Average Humans https://ift.tt/2rFyAUY xem thêm tại: https://ift.tt/2mb4VST để biết thêm về địa chỉ bán tai nghe không dây giá rẻ A Machine Learning Guide for Average Humans https://ift.tt/2rFyAUY xem thêm tại: https://ift.tt/2mb4VST để biết thêm về địa chỉ bán tai nghe không dây giá rẻ A Machine Learning Guide for Average Humans https://ift.tt/2rFyAUY xem thêm tại: https://ift.tt/2mb4VST để biết thêm về địa chỉ bán tai nghe không dây giá rẻ A Machine Learning Guide for Average Humans https://ift.tt/2rFyAUY xem thêm tại: https://ift.tt/2mb4VST để biết thêm về địa chỉ bán tai nghe không dây giá rẻ A Machine Learning Guide for Average Humans https://ift.tt/2rFyAUY xem thêm tại: https://ift.tt/2mb4VST để biết thêm về địa chỉ bán tai nghe không dây giá rẻ A Machine Learning Guide for Average Humans https://ift.tt/2rFyAUY xem thêm tại: https://ift.tt/2mb4VST để biết thêm về địa chỉ bán tai nghe không dây giá rẻ A Machine Learning Guide for Average Humans https://ift.tt/2rFyAUY xem thêm tại: https://ift.tt/2mb4VST để biết thêm về địa chỉ bán tai nghe không dây giá rẻ A Machine Learning Guide for Average Humans https://ift.tt/2rFyAUY xem thêm tại: https://ift.tt/2mb4VST để biết thêm về địa chỉ bán tai nghe không dây giá rẻ A Machine Learning Guide for Average Humans https://ift.tt/2rFyAUY xem thêm tại: https://ift.tt/2mb4VST để biết thêm về địa chỉ bán tai nghe không dây giá rẻ A Machine Learning Guide for Average Humans https://ift.tt/2rFyAUY xem thêm tại: https://ift.tt/2mb4VST để biết thêm về địa chỉ bán tai nghe không dây giá rẻ A Machine Learning Guide for Average Humans https://ift.tt/2rFyAUY xem thêm tại: https://ift.tt/2mb4VST để biết thêm về địa chỉ bán tai nghe không dây giá rẻ A Machine Learning Guide for Average Humans https://ift.tt/2rFyAUY xem thêm tại: https://ift.tt/2mb4VST để biết thêm về địa chỉ bán tai nghe không dây giá rẻ A Machine Learning Guide for Average Humans https://ift.tt/2rFyAUY xem thêm tại: https://ift.tt/2mb4VST để biết thêm về địa chỉ bán tai nghe không dây giá rẻ A Machine Learning Guide for Average Humans https://ift.tt/2rFyAUY xem thêm tại: https://ift.tt/2mb4VST để biết thêm về địa chỉ bán tai nghe không dây giá rẻ A Machine Learning Guide for Average Humans https://ift.tt/2rFyAUY xem thêm tại: https://ift.tt/2mb4VST để biết thêm về địa chỉ bán tai nghe không dây giá rẻ A Machine Learning Guide for Average Humans https://ift.tt/2rFyAUY xem thêm tại: https://ift.tt/2mb4VST để biết thêm về địa chỉ bán tai nghe không dây giá rẻ A Machine Learning Guide for Average Humans https://ift.tt/2rFyAUY xem thêm tại: https://ift.tt/2mb4VST để biết thêm về địa chỉ bán tai nghe không dây giá rẻ A Machine Learning Guide for Average Humans https://ift.tt/2rFyAUY xem thêm tại: https://ift.tt/2mb4VST để biết thêm về địa chỉ bán tai nghe không dây giá rẻ A Machine Learning Guide for Average Humans https://ift.tt/2rFyAUY xem thêm tại: https://ift.tt/2mb4VST để biết thêm về địa chỉ bán tai nghe không dây giá rẻ A Machine Learning Guide for Average Humans https://ift.tt/2rFyAUY xem thêm tại: https://ift.tt/2mb4VST để biết thêm về địa chỉ bán tai nghe không dây giá rẻ A Machine Learning Guide for Average Humans https://ift.tt/2rFyAUY xem thêm tại: https://ift.tt/2mb4VST để biết thêm về địa chỉ bán tai nghe không dây giá rẻ A Machine Learning Guide for Average Humans https://ift.tt/2rFyAUY xem thêm tại: https://ift.tt/2mb4VST để biết thêm về địa chỉ bán tai nghe không dây giá rẻ A Machine Learning Guide for Average Humans https://ift.tt/2rFyAUY xem thêm tại: https://ift.tt/2mb4VST để biết thêm về địa chỉ bán tai nghe không dây giá rẻ A Machine Learning Guide for Average Humans https://ift.tt/2rFyAUY xem thêm tại: https://ift.tt/2mb4VST để biết thêm về địa chỉ bán tai nghe không dây giá rẻ A Machine Learning Guide for Average Humans https://ift.tt/2rFyAUY xem thêm tại: https://ift.tt/2mb4VST để biết thêm về địa chỉ bán tai nghe không dây giá rẻ A Machine Learning Guide for Average Humans https://ift.tt/2rFyAUY xem thêm tại: https://ift.tt/2mb4VST để biết thêm về địa chỉ bán tai nghe không dây giá rẻ A Machine Learning Guide for Average Humans https://ift.tt/2rFyAUY xem thêm tại: https://ift.tt/2mb4VST để biết thêm về địa chỉ bán tai nghe không dây giá rẻ A Machine Learning Guide for Average Humans https://ift.tt/2rFyAUY xem thêm tại: https://ift.tt/2mb4VST để biết thêm về địa chỉ bán tai nghe không dây giá rẻ A Machine Learning Guide for Average Humans https://ift.tt/2rFyAUY xem thêm tại: https://ift.tt/2mb4VST để biết thêm về địa chỉ bán tai nghe không dây giá rẻ A Machine Learning Guide for Average Humans https://ift.tt/2rFyAUY xem thêm tại: https://ift.tt/2mb4VST để biết thêm về địa chỉ bán tai nghe không dây giá rẻ A Machine Learning Guide for Average Humans https://ift.tt/2rFyAUY xem thêm tại: https://ift.tt/2mb4VST để biết thêm về địa chỉ bán tai nghe không dây giá rẻ A Machine Learning Guide for Average Humans https://ift.tt/2rFyAUY xem thêm tại: https://ift.tt/2mb4VST để biết thêm về địa chỉ bán tai nghe không dây giá rẻ A Machine Learning Guide for Average Humans https://ift.tt/2rFyAUY xem thêm tại: https://ift.tt/2mb4VST để biết thêm về địa chỉ bán tai nghe không dây giá rẻ A Machine Learning Guide for Average Humans https://ift.tt/2rFyAUY xem thêm tại: https://ift.tt/2mb4VST để biết thêm về địa chỉ bán tai nghe không dây giá rẻ A Machine Learning Guide for Average Humans https://ift.tt/2rFyAUY xem thêm tại: https://ift.tt/2mb4VST để biết thêm về địa chỉ bán tai nghe không dây giá rẻ A Machine Learning Guide for Average Humans https://ift.tt/2rFyAUY xem thêm tại: https://ift.tt/2mb4VST để biết thêm về địa chỉ bán tai nghe không dây giá rẻ A Machine Learning Guide for Average Humans https://ift.tt/2rFyAUY xem thêm tại: https://ift.tt/2mb4VST để biết thêm về địa chỉ bán tai nghe không dây giá rẻ A Machine Learning Guide for Average Humans https://ift.tt/2rFyAUY xem thêm tại: https://ift.tt/2mb4VST để biết thêm về địa chỉ bán tai nghe không dây giá rẻ A Machine Learning Guide for Average Humans https://ift.tt/2rFyAUY xem thêm tại: https://ift.tt/2mb4VST để biết thêm về địa chỉ bán tai nghe không dây giá rẻ A Machine Learning Guide for Average Humans https://ift.tt/2rFyAUY xem thêm tại: https://ift.tt/2mb4VST để biết thêm về địa chỉ bán tai nghe không dây giá rẻ A Machine Learning Guide for Average Humans https://ift.tt/2rFyAUY xem thêm tại: https://ift.tt/2mb4VST để biết thêm về địa chỉ bán tai nghe không dây giá rẻ A Machine Learning Guide for Average Humans https://ift.tt/2rFyAUY xem thêm tại: https://ift.tt/2mb4VST để biết thêm về địa chỉ bán tai nghe không dây giá rẻ A Machine Learning Guide for Average Humans https://ift.tt/2rFyAUY xem thêm tại: https://ift.tt/2mb4VST để biết thêm về địa chỉ bán tai nghe không dây giá rẻ A Machine Learning Guide for Average Humans https://ift.tt/2rFyAUY xem thêm tại: https://ift.tt/2mb4VST để biết thêm về địa chỉ bán tai nghe không dây giá rẻ A Machine Learning Guide for Average Humans https://ift.tt/2rFyAUY xem thêm tại: https://ift.tt/2mb4VST để biết thêm về địa chỉ bán tai nghe không dây giá rẻ A Machine Learning Guide for Average Humans https://ift.tt/2rFyAUY xem thêm tại: https://ift.tt/2mb4VST để biết thêm về địa chỉ bán tai nghe không dây giá rẻ A Machine Learning Guide for Average Humans https://ift.tt/2rFyAUY xem thêm tại: https://ift.tt/2mb4VST để biết thêm về địa chỉ bán tai nghe không dây giá rẻ A Machine Learning Guide for Average Humans https://ift.tt/2rFyAUY xem thêm tại: https://ift.tt/2mb4VST để biết thêm về địa chỉ bán tai nghe không dây giá rẻ A Machine Learning Guide for Average Humans https://ift.tt/2rFyAUY xem thêm tại: https://ift.tt/2mb4VST để biết thêm về địa chỉ bán tai nghe không dây giá rẻ A Machine Learning Guide for Average Humans https://ift.tt/2rFyAUY xem thêm tại: https://ift.tt/2mb4VST để biết thêm về địa chỉ bán tai nghe không dây giá rẻ A Machine Learning Guide for Average Humans https://ift.tt/2rFyAUY xem thêm tại: https://ift.tt/2mb4VST để biết thêm về địa chỉ bán tai nghe không dây giá rẻ A Machine Learning Guide for Average Humans https://ift.tt/2rFyAUY xem thêm tại: https://ift.tt/2mb4VST để biết thêm về địa chỉ bán tai nghe không dây giá rẻ A Machine Learning Guide for Average Humans https://ift.tt/2rFyAUY xem thêm tại: https://ift.tt/2mb4VST để biết thêm về địa chỉ bán tai nghe không dây giá rẻ A Machine Learning Guide for Average Humans https://ift.tt/2rFyAUY xem thêm tại: https://ift.tt/2mb4VST để biết thêm về địa chỉ bán tai nghe không dây giá rẻ A Machine Learning Guide for Average Humans https://ift.tt/2rFyAUY xem thêm tại: https://ift.tt/2mb4VST để biết thêm về địa chỉ bán tai nghe không dây giá rẻ A Machine Learning Guide for Average Humans https://ift.tt/2rFyAUY Bạn có thể xem thêm địa chỉ mua tai nghe không dây tại đây https://ift.tt/2mb4VST
0 notes
Text
A Machine Learning Guide for Average Humans
A Machine Learning Guide for Average Humans
Posted by alexis-sanders
//<![CDATA[ (function($) { // code using $ as alias to jQuery $(function() { // Hide the hypotext content. $('.hypotext-content').hide(); // When a hypotext link is clicked. $('a.hypotext.closed').click(function (e) { // custom handling here e.preventDefault(); // Create the class reference from the rel value. var id = '.' + $(this).attr('rel'); // If the content is hidden, show it now. if ( $(id).css('display') == 'none' ) { $(id).show('slow'); if (jQuery.ui) { // UI loaded $(id).effect("highlight", {}, 1000); } } // If the content is shown, hide it now. else { $(id).hide('slow'); } }); // If we have a hash value in the url. if (window.location.hash) { // If the anchor is within a hypotext block, expand it, by clicking the // relevant link. console.log(window.location.hash); var anchor = $(window.location.hash); var hypotextLink = $('#' + anchor.parents('.hypotext-content').attr('rel')); console.log(hypotextLink); hypotextLink.click(); // Wait until the content has expanded before jumping to anchor. //$.delay(1000); setTimeout(function(){ scrollToAnchor(window.location.hash); }, 1000); } }); function scrollToAnchor(id) { var anchor = $(id); $('html,body').animate({scrollTop: anchor.offset().top},'slow'); } })(jQuery); //]]>
Machine learning (ML) has grown consistently in worldwide prevalence. Its implications have stretched from small, seemingly inconsequential victories to groundbreaking discoveries. The SEO community is no exception. An understanding and intuition of machine learning can support our understanding of the challenges and solutions Google's engineers are facing, while also opening our minds to ML's broader implications.
The advantages of gaining an general understanding of machine learning include:
Gaining empathy for engineers, who are ultimately trying to establish the best results for users
Understanding what problems machines are solving for, their current capabilities and scientists' goals
Understanding the competitive ecosystem and how companies are using machine learning to drive results
Preparing oneself for for what many industry leaders call a major shift in our society (Andrew Ng refers to AI as a "new electricity")
Understanding basic concepts that often appear within research (it's helped me with understanding certain concepts that appear within Google Brain's Research)
Growing as an individual and expanding your horizons (you might really enjoy machine learning!)
When code works and data is produced, it's a very fulfilling, empowering feeling (even if it's a very humble result)
I spent a year taking online courses, reading books, and learning about learning (...as a machine). This post is the fruit borne of that labor -- it covers 17 machine learning resources (including online courses, books, guides, conference presentations, etc.) comprising the most affordable and popular machine learning resources on the web (through the lens of a complete beginner). I've also added a summary of "If I were to start over again, how I would approach it."
This article isn't about credit or degrees. It's about regular Joes and Joannas with an interest in machine learning, and who want to spend their learning time efficiently. Most of these resources will consume over 50 hours of commitment. Ain't nobody got time for a painful waste of a work week (especially when this is probably completed during your personal time). The goal here is for you to find the resource that best suits your learning style. I genuinely hope you find this research useful, and I encourage comments on which materials prove most helpful (especially ones not included)! #HumanLearningMachineLearning
Executive summary:
Here's everything you need to know in a chart:
Machine Learning Resource
Time (hours)
Cost ($)
Year
Credibility
Code
Math
Enjoyability
Jason Maye's Machine Learning 101 slidedeck: 2 years of headbanging, so you don't have to
2
$0
'17
{ML} Recipes with Josh Gordon Playlist
2
$0
'16
Machine Learning Crash Course
15
$0
'18
OCDevel Machine Learning Guide Podcast
30
$0
'17-
Kaggle's Machine Learning Track (part 1)
6
$0
'17
Fast.ai (part 1)
70
$70*
'16
Hands-On Machine Learning with Scikit-Learn and TensorFlow: Concepts, Tools, and Techniques to Build Intelligent Systems
20
$25
'17
Udacity's Intro to Machine Learning (Kate/Sebastian)
60
$0
'15
Andrew Ng's Coursera Machine Learning
55
$0
'11
iPullRank Machine Learning Guide
3
$0
'17
Review Google PhD
2
$0
'17
Caltech Machine Learning on iTunes
27
$0
'12
Pattern Recognition & Machine Learning by Christopher Bishop
150
$75
'06
N/A
Machine Learning: Hands-on for Developers and Technical Professionals
15
$50
'15
Introduction to Machine Learning with Python: A Guide for Data Scientists
15
$25
'16
Udacity's Machine Learning by Georgia Tech
96
$0
'15
Machine Learning Stanford iTunes by Andrew Ng
25
$0
'08
N/A
*Free, but there is the cost of running an AWS EC2 instance (~$70 when I finished, but I did tinker a ton and made a Rick and Morty script generator, which I ran many epochs [rounds] of...)
Here's my suggested program:
1. Starting out (estimated 60 hours)
Start with shorter content targeting beginners. This will allow you to get the gist of what's going on with minimal time commitment.
Commit three hours to Jason Maye's Machine Learning 101 slidedeck: 2 years of headbanging, so you don't have to.
Commit two hours to watch Google's {ML} Recipes with Josh Gordon YouTube Playlist.
Sign up for Sam DeBrule's Machine Learnings newsletter.
Work through Google's Machine Learning Crash Course.
Start listening to OCDevel's Machine Learning Guide Podcast (skip episodes 1, 3, 16, 21, and 26) in your car, working out, and/or when using hands and eyes for other activities.
Commit two days to working through Kaggle's Machine Learning Track part 1.
2. Ready to commit (estimated 80 hours)
By this point, learners would understand their interest levels. Continue with content focused on applying relevant knowledge as fast as possible.
Commit to Fast.ai 10 hours per week, for 7 weeks. If you have a friend/mentor that can help you work through AWS setup, definitely lean on any support in installation (it's 100% the worst part of ML).
Acquire Hands-On Machine Learning with Scikit-Learn and TensorFlow: Concepts, Tools, and Techniques to Build Intelligent Systems, and read the first two chapters immediately. Then use this as supplemental to the Fast.ai course.
3. Broadening your horizons (estimated 115 hours)
If you've made it through the last section and are still hungry for more knowledge, move on to broadening your horizons. Read content focused on teaching the breadth of machine learning -- building an intuition for what the algorithms are trying to accomplish (whether visual or mathematically).
Start watching videos and participating in Udacity's Intro to Machine Learning (by Sebastian Thrun and Katie Malone).
Work through Andrew Ng's Coursera Machine Learning course.
Your next steps
By this point, you will already have AWS running instances, a mathematical foundation, and an overarching view of machine learning. This is your jumping-off point to determine what you want to do.
You should be able to determine your next step based on your interest, whether it's entering Kaggle competitions; doing Fast.ai part two; diving deep into the mathematics with Pattern Recognition & Machine Learning by Christopher Bishop; giving Andrew Ng's newer Deeplearning.ai course on Coursera; learning more about specific tech stacks (TensorFlow, Scikit-Learn, Keras, Pandas, Numpy, etc.); or applying machine learning to your own problems.
Why am I recommending these steps and resources?
I am not qualified to write an article on machine learning. I don't have a PhD. I took one statistics class in college, which marked the first moment I truly understood "fight or flight" reactions. And to top it off, my coding skills are lackluster (at their best, they're chunks of reverse-engineered code from Stack Overflow). Despite my many shortcomings, this piece had to be written by someone like me, an average person.
Statistically speaking, most of us are average (ah, the bell curve/Gaussian distribution always catches up to us). Since I'm not tied to any elitist sentiments, I can be real with you. Below contains a high-level summary of my reviews on all of the classes I took, along with a plan for how I would approach learning machine learning if I could start over. Click to expand each course for the full version with notes.
In-depth reviews of machine learning courses:
Starting out
Jason Maye's Machine Learning 101 slidedeck: 2 years of head-banging, so you don't have to ↓
Need to Know: A stellar high-level overview of machine learning fundamentals in an engaging and visually stimulating format.
Loved:
Very user-friendly, engaging, and playful slidedeck.
Has the potential to take some of the pain out of the process, through introducing core concepts.
Breaks up content by beginner/need-to-know (green), and intermediate/less-useful noise (specifically for individuals starting out) (blue).
Provides resources to dive deeper into machine learning.
Provides some top people to follow in machine learning.
Disliked:
That there is not more! Jason's creativity, visual-based teaching approach, and quirky sense of humor all support the absorption of the material.
Lecturer:
Jason Mayes:
Senior Creative Technologist and Research Engineer at Google
Masters in Computer Science from University of Bristols
Personal Note: He's also kind on Twitter! :)
Links:
Machine Learning 101 slide deck
Tips on Watching:
Set aside 2-4 hours to work through the deck once.
Since there is a wealth of knowledge, refer back as needed (or as a grounding source).
Identify areas of interest and explore the resources provided.
{ML} Recipes with Josh Gordon ↓
Need to Know: This mini-series YouTube-hosted playlist covers the very fundamentals of machine learning with opportunities to complete exercises.
Loved:
It is genuinely beginner-focused.
They make no assumption of any prior knowledge.
Gloss over potentially complex topics that may serve as noise.
Playlist ~2 hours
Very high-quality filming, audio, and presentation, almost to the point where it had its own aesthetic.
Covers some examples in scikit-learn and TensorFlow, which felt modern and practical.
Josh Gordon was an engaging speaker.
Disliked:
I could not get Dockers on Windows (suggested package manager). This wasn't a huge deal, since I already had my AWS setup by this point; however, a bit of a bummer since it made it impossible to follow certain steps exactly.
Issue: Every time I tried to download (over the course of two weeks), the .exe file would recursively start and keep spinning until either my memory ran out, computer crashed, or I shut my computer down. I sent this to Docker's Twitter account to no avail.
Lecturer:
Josh Gordon:
Developer Advocate for at TensorFlow at Google
Leads Machine Learning advocacy at Google
Member of the Udacity AI & Data Industry Advisory Board
Masters in Computer Science from Columbia University
Links:
Hello World - Machine Learning Recipes #1 (YouTube)
GitHub: Machine Learning Recipes with Josh Gordon
Tips on Watching:
The playlist is short (only ~1.5 hours screen time). However, it can be a bit fast-paced at times (especially if you like mimicking the examples), so set aside 3-4 hours to play around with examples and allow time for installation, pausing, and following along.
Take time to explore code labs.
Google's Machine Learning Crash Course with TensorFlow APIs ↓
Need to Know: A Google researcher-made crash course on machine learning that is interactive and offers its own built-in coding system!
Loved:
Different formats of learning: high-quality video (with ability to adjust speed, closed captioning), readings, quizzes (with explanations), visuals (including whiteboarding), interactive components/ playgrounds, code lab exercises (run directly in your browser (no setup required!))
Non-intimidating
One of my favorite quotes: "You don't need to understand the math to be able to take a look at the graphical interpretation."
Broken down into digestible sections
Introduces key terms
Disliked:
N/A
Lecturers:
Multiple Google researchers participated in this course, including:
Peter Norvig
Director of Research at Google Inc.
Previously he directed Google's core search algorithms group.
He is co-author of Artificial Intelligence: A Modern Approach
D. Sculley
Senior Staff Software Engineer at Google
KDD award-winning papers
Works on massive-scale ML systems for online advertising
Was part of a research ML paper on optimizing chocolate chip cookies
According to his personal website, he prefers to go by "D."
Cassandra Xia
Programmer, Software Engineer at Google
She has some really cool (and cute) projects based on learning statistics concepts interactively
Maya Gupta
Leads Glassbox Machine Learning R&D team at Google
Associate Professor of Electrical Engineering at the University of Washington (2003-2012)
In 2007, Gupta received the PECASE award from President George Bush for her work in classifying uncertain (e.g. random) signals
Gupta also runs Artifact Puzzles, the second-largest US maker of wooden jigsaw puzzles
Sally Goldman
Research Scientist at Google
Co-author of A Practical Guide to Data Structures and Algorithms Using Java
Numerous journals, classes taught at Washington University, and contributions to the ML community
Links:
Machine Learning Crash Course
Tips on Doing:
Actively work through playground and coding exercises
OCDevel's Machine Learning Guide Podcast ↓
Need to Know: This podcast focuses on the high-level fundamentals of machine learning, including basic intuition, algorithms, math, languages, and frameworks. It also includes references to learn more on each episode's topic.
Loved:
Great for trips (when traveling a ton, it was an easy listen).
The podcast makes machine learning fun with interesting and compelling analogies.
Tyler is a big fan of Andrew Ng's Coursera course and reviews concepts in Coursera course very well, such that both pair together nicely.
Covers the canonical resources for learning more on a particular topic.
Disliked:
Certain courses were more theory-based; all are interesting, yet impractical.
Due to limited funding the project is a bit slow to update and has less than 30 episodes.
Podcaster:
Tyler Renelle:
Machine learning engineer focused on time series and reinforcement
Background in full-stack JavaScript, 10 years web and mobile
Creator of HabitRPG, an app that treats habits as an RPG game
Links:
Machine Learning Guide podcast
Machine Learning Guide podcast (iTunes)
Tips on Listening:
Listen along your journey to help solidify understanding of topics.
Skip episodes 1, 3, 16, 21, and 26 (unless their topics interest and inspire you!).
Kaggle Machine Learning Track (Lesson 1) ↓
Need to Know: A simple code lab that covers the very basics of machine learning with scikit-learn and Panda through the application of the examples onto another set of data.
Loved:
A more active form of learning.
An engaging code lab that encourages participants to apply knowledge.
This track offers has a built-in Python notebook on Kaggle with all input files included. This removed any and all setup/installation issues.
Side note: It's a bit different than Jupyter notebook (e.g., have to click into a cell to add another cell).
Each lesson is short, which made the entire lesson go by very fast.
Disliked:
The writing in the first lesson didn't initially make it clear that one would need to apply the knowledge in the lesson to their workbook.
It wasn't a big deal, but when I started referencing files in the lesson, I had to dive into the files in my workbook to find they didn't exist, only to realize that the knowledge was supposed to be applied and not transcribed.
Lecturer:
Dan Becker:
Data Scientist at Kaggle
Undergrad in Computer Science, PhD in Econometrics
Supervised data science consultant for six Fortune 100 companies
Contributed to the Keras and Tensorflow libraries
Finished 2nd (out of 1353 teams) in $3 million Heritage Health Prize data mining competition
Speaks at deep learning workshops at events and conferences
Links:
https://www.kaggle.com/learn/machine-learning
Tips on Doing:
Read the exercises and apply to your dataset as you go.
Try lesson 2, which covers more complex/abstract topics (note: this second took a bit longer to work through).
Ready to commit
Fast.ai (part 1 of 2) ↓
Need to Know: Hands-down the most engaging and active form of learning ML. The source I would most recommend for anyone (although the training plan does help to build up to this course). This course is about learning through coding. This is the only course that I started to truly see the practical mechanics start to come together. It involves applying the most practical solutions to the most common problems (while also building an intuition for those solutions).
Loved:
Course Philosophy:
Active learning approach
"Go out into the world and understand underlying mechanics (of machine learning by doing)."
Counter-culture to the exclusivity of the machine learning field, focusing on inclusion.
"Let's do shit that matters to people as quickly as possible."
Highly pragmatic approach with tools that are currently being used (Jupyter Notebooks, scikit-learn, Keras, AWS, etc.).
Show an end-to-end process that you get to complete and play with in a development environment.
Math is involved, but is not prohibitive. Excel files helped to consolidate information/interact with information in a different way, and Jeremy spends a lot of time recapping confusing concepts.
Amazing set of learning resources that allow for all different styles of learning, including:
Video Lessons
Notes
Jupyter Notebooks
Assignments
Highly active forums
Resources on Stackoverflow
Readings/resources
Jeremy often references popular academic texts
Jeremy's TEDx talk in Brussels
Jeremy really pushes one to do extra and put in the effort by teaching interesting problems and engaging one in solving them.
It's a huge time commitment; however, it's worth it.
All of the course's profits are donated.
Disliked:
Overview covers their approach to learning (obviously I'm a fan!). If you're already drinking the Kool-aid, skip past.
I struggled through the AWS setup (13-minute video) for about five hours (however, it felt so good when it was up and running!).
Because of its practicality and concentration on solutions used today to solve popular problem types (image recognition, text generation, etc.), it lacks breadth of machine learning topics.
Lecturers:
Jeremy Howard:
Distinguished Research Scientist at the University of San Francisco
Faculty member at Singularity University
Young Global Leader with the World Economic Forum
Founder of Enlitic (the first company to apply deep learning to medicine)
Former President and Chief Scientist of the data science platform Kaggle
Rachel Thomas:
PhD in Math from Duke
One of Forbes' "20 Incredible Women Advancing AI Research"
Researcher-in-residence at the University of San Francisco Data Institute
Teaches in the Masters in Data Science program
Links:
http://course.fast.ai/start.html
http://wiki.fast.ai/index.php/Main_Page
https://github.com/fastai/courses/tree/master/deeplearning1/nbs
Tips on Doing:
Set expectations with yourself that installation is going to probably take a few hours.
Prepare to spend about ~70 hours for this course (it's worth it).
Don't forget to shut off your AWS instance.
Balance out machine learning knowledge with a course with more breadth.
Consider giving part two of the Fast.ai program a shot!
Hands-On Machine Learning with Scikit-Learn and TensorFlow: Concepts, Tools, and Techniques to Build Intelligent Systems ↓
Need to Know: This book is an Amazon best seller for a reason. It covers a lot of ground quickly, empowers readers to walk through a machine learning problem by chapter two, and contains practical up-to-date machine learning skills.
Loved:
Book contains an amazing introduction to machine learning that briskly provides an overarching quick view of the machine learning ecosystem.
Chapter 2 immediately walks the reader through an end-to-end machine learning problem.
Immediately afterwards, Aurélien pushes a user to attempt to apply this solution to another problem, which was very empowering.
There are review questions at the end of each chapter to ensure on has grasped the content within the chapter and to push the reader to explore more.
Once installation was completed, it was easy to follow and all code is available on GitHub.
Chapters 11-14 were very tough reading; however, they were a great reference when working through Fast.ai.
Contains some powerful analogies.
Each chapter's introductions were very useful and put everything into context. This general-to-specifics learning was very useful.
Disliked:
Installation was a common source of issues during the beginning of my journey; the text glided over this. I felt the frustration that most people experience from installation should have been addressed with more resources.
Writer:
Aurélien Géron:
Led the YouTube video classification team from 2013 to 2016
Currently a machine Learning consultant
Founder and CTO of Wifirst and Polyconseil
Published technical books (on C++, Wi-Fi, and Internet architectures)
Links:
https://www.amazon.com/_/dp/1491962291?tag=oreilly20-20
http://shop.oreilly.com/product/0636920052289.do
https://github.com/ageron/handson-ml
Tips on Using:
Get a friend with Python experience to help with installation.
Read the introductions to each chapter thoroughly, read the chapter (pay careful attention to code), review the questions at the end (highlight any in-text answer), make a copy of Aurélien's GitHub and make sure everything works on your setup, re-type the notebooks, go to Kaggle and try on other datasets.
Broadening your horizons
Udacity: Intro to Machine Learning (Kate/Sebastian) ↓
Need to Know: A course that covers a range of machine learning topics, supports building of intuition via visualization and simple examples, offers coding challenges, and a certificate (upon completion of a final project). The biggest challenge with this course is bridging the gap between the hand-holding lectures and the coding exercises.
Loved:
Focus on developing a visual intuition on what each model is trying to accomplish.
This visual learning mathematics approach is very useful.
Cover a vast variety and breadth of models and machine learning basics.
In terms of presenting the concept, there was a lot of hand-holding (which I completely appreciated!).
Many people have done this training, so their GitHub accounts can be used as reference for the mini-projects.
Katie actively notes documentation and suggests where viewers can learn more/reference material.
Disliked:
All of the conceptual hand-holding in the lessons is a stark contrast to the challenges of installation, coding exercises, and mini-projects.
This is the first course started and the limited instructions on setting up the environment and many failed attempts caused me to break down crying at least a handful of times.
The mini-projects are intimidating.
There is extra code added to support the viewers; however, it's done so with little acknowledgement as to what it's actually doing. This made learning a bit harder.
Lecturer:
Caitlin (Katie) Malone:
Director of Data Science Research and Development at Civis Analytics
Stanford PhD in Experimental Particle Physics
Intern at Udacity in summer 2014
Graduate Researcher at the SLAC National Accelerator Laboratory
https://www6.slac.stanford.edu/
Podcaster with Ben Jaffe (currently Facebook UI Engineer and a music aficionado) on a machine learning podcast Linear Digressions (100+ episodes)
Sebastian Thrun:
CEO of the Kitty Hawk Corporation
Chairman and co-founder of Udacity
One of my favorite Sebastian quotes: "It occurred to me, I could be at Google and build a self-driving car, or I can teach 10,000 students how to build self-driving cars."
Former Google VP
Founded Google X
Led development of the robotic vehicle Stanley
Professor of Computer Science at Stanford University
Formerly a professor at Carnegie Mellon University.
Links:
https://www.udacity.com/course/intro-to-machine-learning--ud120
Udacity also offers a next step, the Machine Learning Engineer Nanodegree, which will set one back about $1K.
Tips on Watching:
Get a friend to help you set up your environment.
Print mini-project instructions to check off each step.
Andrew Ng's Coursera Machine Learning Course ↓
Need to Know: The Andrew Ng Coursera course is the most referenced online machine learning course. It covers a broad set of fundamental, evergreen topics with a strong focus in building mathematical intuition behind machine learning models. Also, one can submit assignments and earn a grade for free. If you want to earn a certificate, one can subscribe or apply for financial aid.
Loved:
This course has a high level of credibility.
Introduces all necessary machine learning terminology and jargon.
Contains a very classic machine learning education approach with a high level of math focus.
Quizzes interspersed in courses and after each lesson support understanding and overall learning.
The sessions for the course are flexible, the option to switch into a different section is always available.
Disliked:
The mathematic notation was hard to process at times.
The content felt a bit dated and non-pragmatic. For example, the main concentration was MATLAB and Octave versus more modern languages and resources.
Video quality was less than average and could use a refresh.
Lecturer:
Andrew Ng:
Adjunct Professor, Stanford University (focusing on AI, Machine Learning, and Deep Learning)
Co-founder of Coursera
Former head of Baidu AI Group
Founder and previous head of Google Brain (deep learning) project
Former Director of the Stanford AI Lab
Chairman of the board of Woebot (a machine learning bot that focuses on Cognitive Behavior Therapy)
Links:
https://www.coursera.org/learn/machine-learning/
Andrew Ng recently launched a new course (August 2017) called DeepLearning.ai, a ~15 week course containing five mini-courses ($49 USD per month to continue learning after trial period of 7 days ends).
Course: https://www.coursera.org/specializations/deep-learning
Course 1: Neural Networks and Deep Learning
Course 2: Improving Deep Neural Networks: Hyperparameter tuning, Regularization and Optimization
Course 3: Structuring Machine Learning Projects
Course 4: Convolutional Neural Networks
Course 5: Sequence Models
Tips on Watching:
Be disciplined with setting aside timing (even if it's only 15 minutes a day) to help power through some of the more boring concepts.
Don't do this course first, because it's intimidating, requires a large time commitment, and isn't a very energizing experience.
Additional machine learning opportunities
iPullRank Machine Learning Guide ↓
Need to Know: A machine learning e-book targeted at marketers.
Loved:
Targeted at marketers and applied to organic search.
Covers a variety of machine learning topics.
Some good examples, including real-world blunders.
Gives some practical tools for non-data scientists (including: MonkeyLearn and Orange)
I found Orange to be a lot of fun. It struggled with larger datasets; however, it has a very visual interface that was more user-friendly and offers potential to show some pretty compelling stories.
Example: World Happiness Dataset by:
X-axis: Happiness Score
Y-axis: Economy
Color: Health
Disliked:
Potential to break up content more with relevant imagery -- the content was very dense.
Writers:
iPullRank Team (including Mike King):
Mike King has a few slide decks on the basics of machine learnings and AI
iPullRank has a few data scientists on staff
Links:
http://ipullrank.com/machine-learning-guide/
Tips on Reading:
Read chapters 1-6 and the rest depending upon personal interest.
Review Google PhD ↓
Need to Know: A two-hour presentation from Google's 2017 IO conference that walks through getting 99% accuracy on the MNIST dataset (a famous dataset containing a bunch of handwritten numbers, which the machine must learn to identify the numbers).
Loved:
This talk struck me as very modern, covering the cutting edge.
Found this to be very complementary to Fast.ai, as it covered similar topics (e.g. ReLu, CNNs, RNNs, etc.)
Amazing visuals that help to put everything into context.
Disliked:
The presentation is only a short conference solution and not a comprehensive view of machine learning.
Also, a passive form of learning.
Presenter:
Martin Görner:
Developer Relations, Google (since 2011)
Started Mobipocket, a startup that later became the software part of the Amazon Kindle and its mobile variants
Links:
Part 1 - https://www.youtube.com/watch?v=u4alGiomYP4
Part 2 - https://www.youtube.com/watch?v=fTUwdXUFfI8
Tips on Watching:
Google any concepts you're unfamiliar with.
Take your time with this one; 2 hours of screen time doesn't count all of the Googling and processing time for this one.
Caltech Machine Learning iTunes ↓
Need to Know: If math is your thing, this course does a stellar job of building the mathematic intuition behind many machine learning models. Dr. Abu-Mostafa is a raconteur, includes useful visualizations, relevant real-world examples, and compelling analogies.
Loved:
First and foremost, this is a real Caltech course, meaning it's not a watered-down version and contains fundamental concepts that are vital to understanding the mechanics of machine learning.
On iTunes, audio downloads are available, which can be useful for on-the-go learning.
Dr. Abu-Mostafa is a skilled speaker, making the 27 hours spent listening much easier!
Dr. Abu-Mostafa offers up some strong real-world examples and analogies which makes the content more relatable.
As an example, he asks students: "Why do I give you practice exams and not just give you the final exam?" as an illustration of why a testing set is useful. If he were to just give students the final, they would just memorize the answers (i.e., they would overfit to the data) and not genuinely learn the material. The final is a test to show how much students learn.
The last 1/2 hour of the class is always a Q&A, where students can ask questions. Their questions were useful to understanding the topic more in-depth.
The video and audio quality was strong throughout. There were a few times when I couldn't understand a question in the Q&A, but overall very strong.
This course is designed to build mathematical intuition of what's going on under the hood of specific machine learning models.
Caution: Dr. Abu-Mostafa uses mathematical notation, but it's different from Andrew Ng's (e.g., theta = w).
The final lecture was the most useful, as it pulled a lot of the conceptual puzzle pieces together. The course on neural networks was a close second!
Disliked:
Although it contains mostly evergreen content, being released in 2012, it could use a refresh.
Very passive form of learning, as it wasn't immediately actionable.
Lecturer:
Dr. Yaser S. Abu-Mostafa:
Professor of Electrical Engineering and Computer Science at the California Institute of Technology
Chairman of Machine Learning Consultants LLC
Serves on a number of scientific advisory boards
Has served as a technical consultant on machine learning for several companies (including Citibank).
Multiple articles in Scientific American
Links:
https://work.caltech.edu/telecourse.html
https://itunes.apple.com/us/course/machine-learning/id515364596
Tips on Watching:
Consider listening to the last lesson first, as it pulls together the course overall conceptually. The map of the course, below, was particularly useful to organizing the information taught in the courses.
Image source: http://work.caltech.edu/slides/slides18.pdf
"Pattern Recognition & Machine Learning" by Christopher Bishop ↓
Need to Know: This is a very popular college-level machine learning textbook. I've heard it likened to a bible for machine learning. However, after spending a month trying to tackle the first few chapters, I gave up. It was too much math and pre-requisites to tackle (even with a multitude of Google sessions).
Loved:
The text of choice for many major universities, so if you can make it through this text and understand all of the concepts, you're probably in a very good position.
I appreciated the history aside sections, where Bishop talked about influential people and their career accomplishments in statistics and machine learning.
Despite being a highly mathematically text, the textbook actually has some pretty visually intuitive imagery.
Disliked:
I couldn't make it through the text, which was a bit frustrating. The statistics and mathematical notation (which is probably very benign for a student in this topic) were too much for me.
The sunk cost was pretty high here (~$75).
Writer:
Christopher Bishop:
Laboratory Director at Microsoft Research Cambridge
Professor of Computer Science at the University of Edinburgh
Fellow of Darwin College, Cambridge
PhD in Theoretical Physics from the University of Edinburgh
Links:
https://www.amazon.com/Pattern-Recognition-Learning-Information-Statistics/dp/0387310738/ref=sr_1_2?ie=UTF8&qid=1516839475&sr=8-2&keywords=Pattern+Recognition+%26+Machine+Learning
Tips on Reading:
Don't start your machine learning journey with this book.
Get a friend in statistics to walk you through anything complicated (my plan is to get a mentor in statistics).
Consider taking a (free) online statistics course (Khan Academy and Udacity both have some great content on statistics, calculus, math, and data analysis).
Machine Learning: Hands-on for Developers and Technical Professionals ↓
Need to Know: A fun, non-intimidating end-to-end launching pad/whistle stop for machine learning in action.
Loved:
Talks about practical issues that many other sources didn't really address (e.g. data-cleansing).
Covered the basics of machine learning in a non-intimidating way.
Offers abridged, consolidated versions of the content.
Added fun anecdotes that makes it easier to read.
Overall the writer has a great sense of humor.
Writer talks to the reader as if they're a real human being (i.e., doesn't expect you to go out and do proofs; acknowledges the challenge of certain concepts).
Covers a wide variety of topics.
Because it was well-written, I flew through the book (even though it's about ~300 pages).
Disliked:
N/A
Writer:
Jason Bell:
Technical architect, lecturer, and startup consultant
Data Engineer at MastodonC
Former section editor for Java Developer's Journal
Former writer on IBM DeveloperWorks
Links:
https://www.amazon.com/Machine-Learning-Hands-Developers-Professionals/dp/1118889061
https://www.wiley.com/en-us/Machine+Learning%3A+Hands+On+for+Developers+and+Technical+Professionals-p-9781118889060
Jason's Blog: https://dataissexy.wordpress.com/
Tips on Reading:
Download and explore Weka's interface beforehand.
Give some of the exercises a shot.
Introduction to Machine Learning with Python: A Guide for Data Scientists ↓
Need to Know: This was a was a well-written piece on machine learning, making it a quick read.
Loved:
Quick, smooth read.
Easy-to-follow code examples.
The first few chapters served as a stellar introduction to the basics of machine learning.
Contain subtle jokes that add a bit of fun.
Tip to use the Python package manager Anaconda with Jupyter Notebooks was helpful.
Disliked:
Once again, installation was a challenge.
The "mglearn" utility library threw me for a loop. I had to reread the first few chapters before I figured out it was support for the book.
Although I liked the book, I didn't love it. Overall it just missed the "empowering" mark.
Writers:
Andreas C. Müller:
PhD in Computer Science
Lecturer at the Data Science Institute at Columbia University
Worked at the NYU Center for Data Science on open source and open science
Former Machine Learning Scientist at Amazon
Speaks often on Machine Learning and scikit-learn (a popular machine learning library)
And he makes some pretty incredibly useful graphics, such as this scikit-learn cheat sheet:
Image source: http://peekaboo-vision.blogspot.com/2013/01/machin...
Sarah Guido:
Former senior data scientist at Mashable
Lead data scientist at Bitly
2018 SciPy Conference Data Science track co-chair
Links:
https://www.amazon.com/Introduction-Machine-Learning-Python-Scientists/dp/1449369413/ref=sr_1_7?s=books&ie=UTF8&qid=1516734322&sr=1-7&keywords=python+machine+learning
http://shop.oreilly.com/product/0636920030515.do
Tips on Reading:
Type out code examples.
Beware of the "mglearn" utility library.
Udacity: Machine Learning by Georgia Tech ↓
Need to Know: A mix between an online learning experience and a university machine learning teaching approach. The lecturers are fun, but the course still fell a bit short in terms of active learning.
Loved:
This class is offered as CS7641 at Georgia Tech, where it is a part of the Online Masters Degree. Although taking this course here will not earn credit towards the OMS degree, it's still a non-watered-down college teaching philosophy approach.
Covers a wide variety of topics, many of which reminded me of the Caltech course (including: VC Dimension versus Bayesian, Occam's razor, etc.)
Discusses Markov Decision Chains, which is something that didn't really come up in many other introductory machine learning course, but they are referenced within Google patents.
The lecturers have a great dynamic, are wicked smart, and displayed a great sense of (nerd) humor, which make the topics less intimidating.
The course has quizzes, which give the course a slight amount of interaction.
Disliked:
Some videos were very long, which made the content a bit harder to digest.
The course overall was very time consuming.
Despite the quizzes, the course was a very passive form of learning with no assignments and little coding.
Many videos started with a bunch of content already written out. Having the content written out was probably a big time-saver, but it was also a bit jarring for a viewer to see so much information all at once, while also trying to listen.
It's vital to pay very close attention to notation, which compounds in complexity quickly.
Tablet version didn't function flawlessly: some was missing content (which I had to mark down and review on a desktop), the app would crash randomly on the tablet, and sometimes the audio wouldn't start.
There were no subtitles available on tablet, which I found not only to be a major accessibility blunder, but also made it harder for me to process (since I'm not an audio learner).
Lecturer:
Michael Littman:
Professor of Computer Science at Brown University.
Was granted a patent for one of the earliest systems for Cross-language information retrieval
Perhaps the most interesting man in the world:
Been in two TEDx talks
How I Learned to Stop Worrying and Be Realistic About AI
A Cooperative Path to Artificial Intelligence
During his time at Duke, he worked on an automated crossword solver (PROVERB)
Has a Family Quartet
He has appeared in a TurboTax commercial
Charles Isbell:
Professor and Executive Associate Dean at School of Interactive Computing at Georgia Tech
Focus on statistical machine learning and "interactive" artificial intelligence.
Links:
https://www.udacity.com/course/machine-learning--ud262
Tips on Watching:
Pick specific topics of interest and focusing on those lessons.
Andrew Ng's Stanford's Machine Learning iTunes ↓
Need to Know: A non-watered-down Stanford course. It's outdated (filmed in 2008), video/audio are a bit poor, and most links online now point towards the Coursera course. Although the idea of watching a Stanford course was energizing for the first few courses, it became dreadfully boring. I made it to course six before calling it.
Loved:
Designed for students, so you know you're not missing out on anything.
This course provides a deeper study into the mathematical and theoretical foundation behind machine learning to the point that the students could create their own machine learning algorithms. This isn't necessarily very practical for the everyday machine learning user.
Has some powerful real-world examples (although they're outdated).
There is something about the kinesthetic nature of watching someone write information out. The blackboard writing helped me to process certain ideas.
Disliked:
Video and audio quality were pain to watch.
Many questions asked by students were hard to hear.
On-screen visuals range from hard to impossible to see.
Found myself counting minutes.
Dr. Ng mentions TA classes, supplementary learning, but these are not available online.
Sometimes the video showed students, which I felt was invasive.
Lecturer:
Andrew Ng (see above)
Links:
https://itunes.apple.com/us/course/machine-learning/id495053006
https://www.youtube.com/watch?v=UzxYlbK2c7E
Tips on Watching:
Only watch if you're looking to gain a deeper understanding of the math presented in the Coursera course.
Skip the first half of the first lecture, since it's mostly class logistics.
Additional Resources
Fast.ai (part 2) - free access to materials, cost for AWS EC2 instance
Deeplearning.ai - $50/month
Udacity Machine Learning Engineer Nanodegree - $1K
https://machinelearningmastery.com/
Motivations and inspiration
If you're wondering why I spent a year doing this, then I'm with you. I'm genuinely not sure why I set my sights on this project, much less why I followed through with it. I saw Mike King give a session on Machine Learning. I was caught off guard, since I knew nothing on the topic. It gave me a pesky, insatiable curiosity itch. It started with one course and then spiraled out of control. Eventually it transformed into an idea: a review guide on the most affordable and popular machine learning resources on the web (through the lens of a complete beginner). Hopefully you found it useful, or at least somewhat interesting. Be sure to share your thoughts or questions in the comments!
Sign up for The Moz Top 10, a semimonthly mailer updating you on the top ten hottest pieces of SEO news, tips, and rad links uncovered by the Moz team. Think of it as your exclusive digest of stuff you don't have time to hunt down but want to read!
xem them tai https://ift.tt/2o9GYfe A Machine Learning Guide for Average Humans xem thêm tại: https://ift.tt/2mb4VST để biết thêm về địa chỉ bán tai nghe không dây giá rẻ A Machine Learning Guide for Average Humans https://ift.tt/2rFyAUY xem thêm tại: https://ift.tt/2mb4VST để biết thêm về địa chỉ bán tai nghe không dây giá rẻ A Machine Learning Guide for Average Humans https://ift.tt/2rFyAUY xem thêm tại: https://ift.tt/2mb4VST để biết thêm về địa chỉ bán tai nghe không dây giá rẻ A Machine Learning Guide for Average Humans https://ift.tt/2rFyAUY xem thêm tại: https://ift.tt/2mb4VST để biết thêm về địa chỉ bán tai nghe không dây giá rẻ A Machine Learning Guide for Average Humans https://ift.tt/2rFyAUY xem thêm tại: https://ift.tt/2mb4VST để biết thêm về địa chỉ bán tai nghe không dây giá rẻ A Machine Learning Guide for Average Humans https://ift.tt/2rFyAUY xem thêm tại: https://ift.tt/2mb4VST để biết thêm về địa chỉ bán tai nghe không dây giá rẻ A Machine Learning Guide for Average Humans https://ift.tt/2rFyAUY xem thêm tại: https://ift.tt/2mb4VST để biết thêm về địa chỉ bán tai nghe không dây giá rẻ A Machine Learning Guide for Average Humans https://ift.tt/2rFyAUY xem thêm tại: https://ift.tt/2mb4VST để biết thêm về địa chỉ bán tai nghe không dây giá rẻ A Machine Learning Guide for Average Humans https://ift.tt/2rFyAUY xem thêm tại: https://ift.tt/2mb4VST để biết thêm về địa chỉ bán tai nghe không dây giá rẻ A Machine Learning Guide for Average Humans https://ift.tt/2rFyAUY xem thêm tại: https://ift.tt/2mb4VST để biết thêm về địa chỉ bán tai nghe không dây giá rẻ A Machine Learning Guide for Average Humans https://ift.tt/2rFyAUY xem thêm tại: https://ift.tt/2mb4VST để biết thêm về địa chỉ bán tai nghe không dây giá rẻ A Machine Learning Guide for Average Humans https://ift.tt/2rFyAUY xem thêm tại: https://ift.tt/2mb4VST để biết thêm về địa chỉ bán tai nghe không dây giá rẻ A Machine Learning Guide for Average Humans https://ift.tt/2rFyAUY xem thêm tại: https://ift.tt/2mb4VST để biết thêm về địa chỉ bán tai nghe không dây giá rẻ A Machine Learning Guide for Average Humans https://ift.tt/2rFyAUY xem thêm tại: https://ift.tt/2mb4VST để biết thêm về địa chỉ bán tai nghe không dây giá rẻ A Machine Learning Guide for Average Humans https://ift.tt/2rFyAUY xem thêm tại: https://ift.tt/2mb4VST để biết thêm về địa chỉ bán tai nghe không dây giá rẻ A Machine Learning Guide for Average Humans https://ift.tt/2rFyAUY xem thêm tại: https://ift.tt/2mb4VST để biết thêm về địa chỉ bán tai nghe không dây giá rẻ A Machine Learning Guide for Average Humans https://ift.tt/2rFyAUY xem thêm tại: https://ift.tt/2mb4VST để biết thêm về địa chỉ bán tai nghe không dây giá rẻ A Machine Learning Guide for Average Humans https://ift.tt/2rFyAUY xem thêm tại: https://ift.tt/2mb4VST để biết thêm về địa chỉ bán tai nghe không dây giá rẻ A Machine Learning Guide for Average Humans https://ift.tt/2rFyAUY xem thêm tại: https://ift.tt/2mb4VST để biết thêm về địa chỉ bán tai nghe không dây giá rẻ A Machine Learning Guide for Average Humans https://ift.tt/2rFyAUY xem thêm tại: https://ift.tt/2mb4VST để biết thêm về địa chỉ bán tai nghe không dây giá rẻ A Machine Learning Guide for Average Humans https://ift.tt/2rFyAUY xem thêm tại: https://ift.tt/2mb4VST để biết thêm về địa chỉ bán tai nghe không dây giá rẻ A Machine Learning Guide for Average Humans https://ift.tt/2rFyAUY xem thêm tại: https://ift.tt/2mb4VST để biết thêm về địa chỉ bán tai nghe không dây giá rẻ A Machine Learning Guide for Average Humans https://ift.tt/2rFyAUY xem thêm tại: https://ift.tt/2mb4VST để biết thêm về địa chỉ bán tai nghe không dây giá rẻ A Machine Learning Guide for Average Humans https://ift.tt/2rFyAUY xem thêm tại: https://ift.tt/2mb4VST để biết thêm về địa chỉ bán tai nghe không dây giá rẻ A Machine Learning Guide for Average Humans https://ift.tt/2rFyAUY xem thêm tại: https://ift.tt/2mb4VST để biết thêm về địa chỉ bán tai nghe không dây giá rẻ A Machine Learning Guide for Average Humans https://ift.tt/2rFyAUY xem thêm tại: https://ift.tt/2mb4VST để biết thêm về địa chỉ bán tai nghe không dây giá rẻ A Machine Learning Guide for Average Humans https://ift.tt/2rFyAUY xem thêm tại: https://ift.tt/2mb4VST để biết thêm về địa chỉ bán tai nghe không dây giá rẻ A Machine Learning Guide for Average Humans https://ift.tt/2rFyAUY xem thêm tại: https://ift.tt/2mb4VST để biết thêm về địa chỉ bán tai nghe không dây giá rẻ A Machine Learning Guide for Average Humans https://ift.tt/2rFyAUY xem thêm tại: https://ift.tt/2mb4VST để biết thêm về địa chỉ bán tai nghe không dây giá rẻ A Machine Learning Guide for Average Humans https://ift.tt/2rFyAUY xem thêm tại: https://ift.tt/2mb4VST để biết thêm về địa chỉ bán tai nghe không dây giá rẻ A Machine Learning Guide for Average Humans https://ift.tt/2rFyAUY xem thêm tại: https://ift.tt/2mb4VST để biết thêm về địa chỉ bán tai nghe không dây giá rẻ A Machine Learning Guide for Average Humans https://ift.tt/2rFyAUY xem thêm tại: https://ift.tt/2mb4VST để biết thêm về địa chỉ bán tai nghe không dây giá rẻ A Machine Learning Guide for Average Humans https://ift.tt/2rFyAUY xem thêm tại: https://ift.tt/2mb4VST để biết thêm về địa chỉ bán tai nghe không dây giá rẻ A Machine Learning Guide for Average Humans https://ift.tt/2rFyAUY xem thêm tại: https://ift.tt/2mb4VST để biết thêm về địa chỉ bán tai nghe không dây giá rẻ A Machine Learning Guide for Average Humans https://ift.tt/2rFyAUY xem thêm tại: https://ift.tt/2mb4VST để biết thêm về địa chỉ bán tai nghe không dây giá rẻ A Machine Learning Guide for Average Humans https://ift.tt/2rFyAUY xem thêm tại: https://ift.tt/2mb4VST để biết thêm về địa chỉ bán tai nghe không dây giá rẻ A Machine Learning Guide for Average Humans https://ift.tt/2rFyAUY xem thêm tại: https://ift.tt/2mb4VST để biết thêm về địa chỉ bán tai nghe không dây giá rẻ A Machine Learning Guide for Average Humans https://ift.tt/2rFyAUY xem thêm tại: https://ift.tt/2mb4VST để biết thêm về địa chỉ bán tai nghe không dây giá rẻ A Machine Learning Guide for Average Humans https://ift.tt/2rFyAUY xem thêm tại: https://ift.tt/2mb4VST để biết thêm về địa chỉ bán tai nghe không dây giá rẻ A Machine Learning Guide for Average Humans https://ift.tt/2rFyAUY xem thêm tại: https://ift.tt/2mb4VST để biết thêm về địa chỉ bán tai nghe không dây giá rẻ A Machine Learning Guide for Average Humans https://ift.tt/2rFyAUY xem thêm tại: https://ift.tt/2mb4VST để biết thêm về địa chỉ bán tai nghe không dây giá rẻ A Machine Learning Guide for Average Humans https://ift.tt/2rFyAUY xem thêm tại: https://ift.tt/2mb4VST để biết thêm về địa chỉ bán tai nghe không dây giá rẻ A Machine Learning Guide for Average Humans https://ift.tt/2rFyAUY xem thêm tại: https://ift.tt/2mb4VST để biết thêm về địa chỉ bán tai nghe không dây giá rẻ A Machine Learning Guide for Average Humans https://ift.tt/2rFyAUY xem thêm tại: https://ift.tt/2mb4VST để biết thêm về địa chỉ bán tai nghe không dây giá rẻ A Machine Learning Guide for Average Humans https://ift.tt/2rFyAUY xem thêm tại: https://ift.tt/2mb4VST để biết thêm về địa chỉ bán tai nghe không dây giá rẻ A Machine Learning Guide for Average Humans https://ift.tt/2rFyAUY xem thêm tại: https://ift.tt/2mb4VST để biết thêm về địa chỉ bán tai nghe không dây giá rẻ A Machine Learning Guide for Average Humans https://ift.tt/2rFyAUY xem thêm tại: https://ift.tt/2mb4VST để biết thêm về địa chỉ bán tai nghe không dây giá rẻ A Machine Learning Guide for Average Humans https://ift.tt/2rFyAUY xem thêm tại: https://ift.tt/2mb4VST để biết thêm về địa chỉ bán tai nghe không dây giá rẻ A Machine Learning Guide for Average Humans https://ift.tt/2rFyAUY xem thêm tại: https://ift.tt/2mb4VST để biết thêm về địa chỉ bán tai nghe không dây giá rẻ A Machine Learning Guide for Average Humans https://ift.tt/2rFyAUY xem thêm tại: https://ift.tt/2mb4VST để biết thêm về địa chỉ bán tai nghe không dây giá rẻ A Machine Learning Guide for Average Humans https://ift.tt/2rFyAUY xem thêm tại: https://ift.tt/2mb4VST để biết thêm về địa chỉ bán tai nghe không dây giá rẻ A Machine Learning Guide for Average Humans https://ift.tt/2rFyAUY xem thêm tại: https://ift.tt/2mb4VST để biết thêm về địa chỉ bán tai nghe không dây giá rẻ A Machine Learning Guide for Average Humans https://ift.tt/2rFyAUY xem thêm tại: https://ift.tt/2mb4VST để biết thêm về địa chỉ bán tai nghe không dây giá rẻ A Machine Learning Guide for Average Humans https://ift.tt/2rFyAUY xem thêm tại: https://ift.tt/2mb4VST để biết thêm về địa chỉ bán tai nghe không dây giá rẻ A Machine Learning Guide for Average Humans https://ift.tt/2rFyAUY xem thêm tại: https://ift.tt/2mb4VST để biết thêm về địa chỉ bán tai nghe không dây giá rẻ A Machine Learning Guide for Average Humans https://ift.tt/2rFyAUY xem thêm tại: https://ift.tt/2mb4VST để biết thêm về địa chỉ bán tai nghe không dây giá rẻ A Machine Learning Guide for Average Humans https://ift.tt/2rFyAUY xem thêm tại: https://ift.tt/2mb4VST để biết thêm về địa chỉ bán tai nghe không dây giá rẻ A Machine Learning Guide for Average Humans https://ift.tt/2rFyAUY xem thêm tại: https://ift.tt/2mb4VST để biết thêm về địa chỉ bán tai nghe không dây giá rẻ A Machine Learning Guide for Average Humans https://ift.tt/2rFyAUY xem thêm tại: https://ift.tt/2mb4VST để biết thêm về địa chỉ bán tai nghe không dây giá rẻ A Machine Learning Guide for Average Humans https://ift.tt/2rFyAUY xem thêm tại: https://ift.tt/2mb4VST để biết thêm về địa chỉ bán tai nghe không dây giá rẻ A Machine Learning Guide for Average Humans https://ift.tt/2rFyAUY xem thêm tại: https://ift.tt/2mb4VST để biết thêm về địa chỉ bán tai nghe không dây giá rẻ A Machine Learning Guide for Average Humans https://ift.tt/2rFyAUY xem thêm tại: https://ift.tt/2mb4VST để biết thêm về địa chỉ bán tai nghe không dây giá rẻ A Machine Learning Guide for Average Humans https://ift.tt/2rFyAUY xem thêm tại: https://ift.tt/2mb4VST để biết thêm về địa chỉ bán tai nghe không dây giá rẻ A Machine Learning Guide for Average Humans https://ift.tt/2rFyAUY xem thêm tại: https://ift.tt/2mb4VST để biết thêm về địa chỉ bán tai nghe không dây giá rẻ A Machine Learning Guide for Average Humans https://ift.tt/2rFyAUY xem thêm tại: https://ift.tt/2mb4VST để biết thêm về địa chỉ bán tai nghe không dây giá rẻ A Machine Learning Guide for Average Humans https://ift.tt/2rFyAUY xem thêm tại: https://ift.tt/2mb4VST để biết thêm về địa chỉ bán tai nghe không dây giá rẻ A Machine Learning Guide for Average Humans https://ift.tt/2rFyAUY xem thêm tại: https://ift.tt/2mb4VST để biết thêm về địa chỉ bán tai nghe không dây giá rẻ A Machine Learning Guide for Average Humans https://ift.tt/2rFyAUY xem thêm tại: https://ift.tt/2mb4VST để biết thêm về địa chỉ bán tai nghe không dây giá rẻ A Machine Learning Guide for Average Humans https://ift.tt/2rFyAUY xem thêm tại: https://ift.tt/2mb4VST để biết thêm về địa chỉ bán tai nghe không dây giá rẻ A Machine Learning Guide for Average Humans https://ift.tt/2rFyAUY xem thêm tại: https://ift.tt/2mb4VST để biết thêm về địa chỉ bán tai nghe không dây giá rẻ A Machine Learning Guide for Average Humans https://ift.tt/2rFyAUY xem thêm tại: https://ift.tt/2mb4VST để biết thêm về địa chỉ bán tai nghe không dây giá rẻ A Machine Learning Guide for Average Humans https://ift.tt/2rFyAUY xem thêm tại: https://ift.tt/2mb4VST để biết thêm về địa chỉ bán tai nghe không dây giá rẻ A Machine Learning Guide for Average Humans https://ift.tt/2rFyAUY xem thêm tại: https://ift.tt/2mb4VST để biết thêm về địa chỉ bán tai nghe không dây giá rẻ A Machine Learning Guide for Average Humans https://ift.tt/2rFyAUY xem thêm tại: https://ift.tt/2mb4VST để biết thêm về địa chỉ bán tai nghe không dây giá rẻ A Machine Learning Guide for Average Humans https://ift.tt/2rFyAUY xem thêm tại: https://ift.tt/2mb4VST để biết thêm về địa chỉ bán tai nghe không dây giá rẻ A Machine Learning Guide for Average Humans https://ift.tt/2rFyAUY xem thêm tại: https://ift.tt/2mb4VST để biết thêm về địa chỉ bán tai nghe không dây giá rẻ A Machine Learning Guide for Average Humans https://ift.tt/2rFyAUY xem thêm tại: https://ift.tt/2mb4VST để biết thêm về địa chỉ bán tai nghe không dây giá rẻ A Machine Learning Guide for Average Humans https://ift.tt/2rFyAUY xem thêm tại: https://ift.tt/2mb4VST để biết thêm về địa chỉ bán tai nghe không dây giá rẻ A Machine Learning Guide for Average Humans https://ift.tt/2rFyAUY xem thêm tại: https://ift.tt/2mb4VST để biết thêm về địa chỉ bán tai nghe không dây giá rẻ A Machine Learning Guide for Average Humans https://ift.tt/2rFyAUY xem thêm tại: https://ift.tt/2mb4VST để biết thêm về địa chỉ bán tai nghe không dây giá rẻ A Machine Learning Guide for Average Humans https://ift.tt/2rFyAUY xem thêm tại: https://ift.tt/2mb4VST để biết thêm về địa chỉ bán tai nghe không dây giá rẻ A Machine Learning Guide for Average Humans https://ift.tt/2rFyAUY xem thêm tại: https://ift.tt/2mb4VST để biết thêm về địa chỉ bán tai nghe không dây giá rẻ A Machine Learning Guide for Average Humans https://ift.tt/2rFyAUY xem thêm tại: https://ift.tt/2mb4VST để biết thêm về địa chỉ bán tai nghe không dây giá rẻ A Machine Learning Guide for Average Humans https://ift.tt/2rFyAUY Bạn có thể xem thêm địa chỉ mua tai nghe không dây tại đây https://ift.tt/2mb4VST
0 notes
Text
A Machine Learning Guide for Average Humans
A Machine Learning Guide for Average Humans
Posted by alexis-sanders
//<![CDATA[ (function($) { // code using $ as alias to jQuery $(function() { // Hide the hypotext content. $('.hypotext-content').hide(); // When a hypotext link is clicked. $('a.hypotext.closed').click(function (e) { // custom handling here e.preventDefault(); // Create the class reference from the rel value. var id = '.' + $(this).attr('rel'); // If the content is hidden, show it now. if ( $(id).css('display') == 'none' ) { $(id).show('slow'); if (jQuery.ui) { // UI loaded $(id).effect("highlight", {}, 1000); } } // If the content is shown, hide it now. else { $(id).hide('slow'); } }); // If we have a hash value in the url. if (window.location.hash) { // If the anchor is within a hypotext block, expand it, by clicking the // relevant link. console.log(window.location.hash); var anchor = $(window.location.hash); var hypotextLink = $('#' + anchor.parents('.hypotext-content').attr('rel')); console.log(hypotextLink); hypotextLink.click(); // Wait until the content has expanded before jumping to anchor. //$.delay(1000); setTimeout(function(){ scrollToAnchor(window.location.hash); }, 1000); } }); function scrollToAnchor(id) { var anchor = $(id); $('html,body').animate({scrollTop: anchor.offset().top},'slow'); } })(jQuery); //]]>
Machine learning (ML) has grown consistently in worldwide prevalence. Its implications have stretched from small, seemingly inconsequential victories to groundbreaking discoveries. The SEO community is no exception. An understanding and intuition of machine learning can support our understanding of the challenges and solutions Google's engineers are facing, while also opening our minds to ML's broader implications.
The advantages of gaining an general understanding of machine learning include:
Gaining empathy for engineers, who are ultimately trying to establish the best results for users
Understanding what problems machines are solving for, their current capabilities and scientists' goals
Understanding the competitive ecosystem and how companies are using machine learning to drive results
Preparing oneself for for what many industry leaders call a major shift in our society (Andrew Ng refers to AI as a "new electricity")
Understanding basic concepts that often appear within research (it's helped me with understanding certain concepts that appear within Google Brain's Research)
Growing as an individual and expanding your horizons (you might really enjoy machine learning!)
When code works and data is produced, it's a very fulfilling, empowering feeling (even if it's a very humble result)
I spent a year taking online courses, reading books, and learning about learning (...as a machine). This post is the fruit borne of that labor -- it covers 17 machine learning resources (including online courses, books, guides, conference presentations, etc.) comprising the most affordable and popular machine learning resources on the web (through the lens of a complete beginner). I've also added a summary of "If I were to start over again, how I would approach it."
This article isn't about credit or degrees. It's about regular Joes and Joannas with an interest in machine learning, and who want to spend their learning time efficiently. Most of these resources will consume over 50 hours of commitment. Ain't nobody got time for a painful waste of a work week (especially when this is probably completed during your personal time). The goal here is for you to find the resource that best suits your learning style. I genuinely hope you find this research useful, and I encourage comments on which materials prove most helpful (especially ones not included)! #HumanLearningMachineLearning
Executive summary:
Here's everything you need to know in a chart:
Machine Learning Resource
Time (hours)
Cost ($)
Year
Credibility
Code
Math
Enjoyability
Jason Maye's Machine Learning 101 slidedeck: 2 years of headbanging, so you don't have to
2
$0
'17
{ML} Recipes with Josh Gordon Playlist
2
$0
'16
Machine Learning Crash Course
15
$0
'18
OCDevel Machine Learning Guide Podcast
30
$0
'17-
Kaggle's Machine Learning Track (part 1)
6
$0
'17
Fast.ai (part 1)
70
$70*
'16
Hands-On Machine Learning with Scikit-Learn and TensorFlow: Concepts, Tools, and Techniques to Build Intelligent Systems
20
$25
'17
Udacity's Intro to Machine Learning (Kate/Sebastian)
60
$0
'15
Andrew Ng's Coursera Machine Learning
55
$0
'11
iPullRank Machine Learning Guide
3
$0
'17
Review Google PhD
2
$0
'17
Caltech Machine Learning on iTunes
27
$0
'12
Pattern Recognition & Machine Learning by Christopher Bishop
150
$75
'06
N/A
Machine Learning: Hands-on for Developers and Technical Professionals
15
$50
'15
Introduction to Machine Learning with Python: A Guide for Data Scientists
15
$25
'16
Udacity's Machine Learning by Georgia Tech
96
$0
'15
Machine Learning Stanford iTunes by Andrew Ng
25
$0
'08
N/A
*Free, but there is the cost of running an AWS EC2 instance (~$70 when I finished, but I did tinker a ton and made a Rick and Morty script generator, which I ran many epochs [rounds] of...)
Here's my suggested program:
1. Starting out (estimated 60 hours)
Start with shorter content targeting beginners. This will allow you to get the gist of what's going on with minimal time commitment.
Commit three hours to Jason Maye's Machine Learning 101 slidedeck: 2 years of headbanging, so you don't have to.
Commit two hours to watch Google's {ML} Recipes with Josh Gordon YouTube Playlist.
Sign up for Sam DeBrule's Machine Learnings newsletter.
Work through Google's Machine Learning Crash Course.
Start listening to OCDevel's Machine Learning Guide Podcast (skip episodes 1, 3, 16, 21, and 26) in your car, working out, and/or when using hands and eyes for other activities.
Commit two days to working through Kaggle's Machine Learning Track part 1.
2. Ready to commit (estimated 80 hours)
By this point, learners would understand their interest levels. Continue with content focused on applying relevant knowledge as fast as possible.
Commit to Fast.ai 10 hours per week, for 7 weeks. If you have a friend/mentor that can help you work through AWS setup, definitely lean on any support in installation (it's 100% the worst part of ML).
Acquire Hands-On Machine Learning with Scikit-Learn and TensorFlow: Concepts, Tools, and Techniques to Build Intelligent Systems, and read the first two chapters immediately. Then use this as supplemental to the Fast.ai course.
3. Broadening your horizons (estimated 115 hours)
If you've made it through the last section and are still hungry for more knowledge, move on to broadening your horizons. Read content focused on teaching the breadth of machine learning -- building an intuition for what the algorithms are trying to accomplish (whether visual or mathematically).
Start watching videos and participating in Udacity's Intro to Machine Learning (by Sebastian Thrun and Katie Malone).
Work through Andrew Ng's Coursera Machine Learning course.
Your next steps
By this point, you will already have AWS running instances, a mathematical foundation, and an overarching view of machine learning. This is your jumping-off point to determine what you want to do.
You should be able to determine your next step based on your interest, whether it's entering Kaggle competitions; doing Fast.ai part two; diving deep into the mathematics with Pattern Recognition & Machine Learning by Christopher Bishop; giving Andrew Ng's newer Deeplearning.ai course on Coursera; learning more about specific tech stacks (TensorFlow, Scikit-Learn, Keras, Pandas, Numpy, etc.); or applying machine learning to your own problems.
Why am I recommending these steps and resources?
I am not qualified to write an article on machine learning. I don't have a PhD. I took one statistics class in college, which marked the first moment I truly understood "fight or flight" reactions. And to top it off, my coding skills are lackluster (at their best, they're chunks of reverse-engineered code from Stack Overflow). Despite my many shortcomings, this piece had to be written by someone like me, an average person.
Statistically speaking, most of us are average (ah, the bell curve/Gaussian distribution always catches up to us). Since I'm not tied to any elitist sentiments, I can be real with you. Below contains a high-level summary of my reviews on all of the classes I took, along with a plan for how I would approach learning machine learning if I could start over. Click to expand each course for the full version with notes.
In-depth reviews of machine learning courses:
Starting out
Jason Maye's Machine Learning 101 slidedeck: 2 years of head-banging, so you don't have to ↓
Need to Know: A stellar high-level overview of machine learning fundamentals in an engaging and visually stimulating format.
Loved:
Very user-friendly, engaging, and playful slidedeck.
Has the potential to take some of the pain out of the process, through introducing core concepts.
Breaks up content by beginner/need-to-know (green), and intermediate/less-useful noise (specifically for individuals starting out) (blue).
Provides resources to dive deeper into machine learning.
Provides some top people to follow in machine learning.
Disliked:
That there is not more! Jason's creativity, visual-based teaching approach, and quirky sense of humor all support the absorption of the material.
Lecturer:
Jason Mayes:
Senior Creative Technologist and Research Engineer at Google
Masters in Computer Science from University of Bristols
Personal Note: He's also kind on Twitter! :)
Links:
Machine Learning 101 slide deck
Tips on Watching:
Set aside 2-4 hours to work through the deck once.
Since there is a wealth of knowledge, refer back as needed (or as a grounding source).
Identify areas of interest and explore the resources provided.
{ML} Recipes with Josh Gordon ↓
Need to Know: This mini-series YouTube-hosted playlist covers the very fundamentals of machine learning with opportunities to complete exercises.
Loved:
It is genuinely beginner-focused.
They make no assumption of any prior knowledge.
Gloss over potentially complex topics that may serve as noise.
Playlist ~2 hours
Very high-quality filming, audio, and presentation, almost to the point where it had its own aesthetic.
Covers some examples in scikit-learn and TensorFlow, which felt modern and practical.
Josh Gordon was an engaging speaker.
Disliked:
I could not get Dockers on Windows (suggested package manager). This wasn't a huge deal, since I already had my AWS setup by this point; however, a bit of a bummer since it made it impossible to follow certain steps exactly.
Issue: Every time I tried to download (over the course of two weeks), the .exe file would recursively start and keep spinning until either my memory ran out, computer crashed, or I shut my computer down. I sent this to Docker's Twitter account to no avail.
Lecturer:
Josh Gordon:
Developer Advocate for at TensorFlow at Google
Leads Machine Learning advocacy at Google
Member of the Udacity AI & Data Industry Advisory Board
Masters in Computer Science from Columbia University
Links:
Hello World - Machine Learning Recipes #1 (YouTube)
GitHub: Machine Learning Recipes with Josh Gordon
Tips on Watching:
The playlist is short (only ~1.5 hours screen time). However, it can be a bit fast-paced at times (especially if you like mimicking the examples), so set aside 3-4 hours to play around with examples and allow time for installation, pausing, and following along.
Take time to explore code labs.
Google's Machine Learning Crash Course with TensorFlow APIs ↓
Need to Know: A Google researcher-made crash course on machine learning that is interactive and offers its own built-in coding system!
Loved:
Different formats of learning: high-quality video (with ability to adjust speed, closed captioning), readings, quizzes (with explanations), visuals (including whiteboarding), interactive components/ playgrounds, code lab exercises (run directly in your browser (no setup required!))
Non-intimidating
One of my favorite quotes: "You don't need to understand the math to be able to take a look at the graphical interpretation."
Broken down into digestible sections
Introduces key terms
Disliked:
N/A
Lecturers:
Multiple Google researchers participated in this course, including:
Peter Norvig
Director of Research at Google Inc.
Previously he directed Google's core search algorithms group.
He is co-author of Artificial Intelligence: A Modern Approach
D. Sculley
Senior Staff Software Engineer at Google
KDD award-winning papers
Works on massive-scale ML systems for online advertising
Was part of a research ML paper on optimizing chocolate chip cookies
According to his personal website, he prefers to go by "D."
Cassandra Xia
Programmer, Software Engineer at Google
She has some really cool (and cute) projects based on learning statistics concepts interactively
Maya Gupta
Leads Glassbox Machine Learning R&D team at Google
Associate Professor of Electrical Engineering at the University of Washington (2003-2012)
In 2007, Gupta received the PECASE award from President George Bush for her work in classifying uncertain (e.g. random) signals
Gupta also runs Artifact Puzzles, the second-largest US maker of wooden jigsaw puzzles
Sally Goldman
Research Scientist at Google
Co-author of A Practical Guide to Data Structures and Algorithms Using Java
Numerous journals, classes taught at Washington University, and contributions to the ML community
Links:
Machine Learning Crash Course
Tips on Doing:
Actively work through playground and coding exercises
OCDevel's Machine Learning Guide Podcast ↓
Need to Know: This podcast focuses on the high-level fundamentals of machine learning, including basic intuition, algorithms, math, languages, and frameworks. It also includes references to learn more on each episode's topic.
Loved:
Great for trips (when traveling a ton, it was an easy listen).
The podcast makes machine learning fun with interesting and compelling analogies.
Tyler is a big fan of Andrew Ng's Coursera course and reviews concepts in Coursera course very well, such that both pair together nicely.
Covers the canonical resources for learning more on a particular topic.
Disliked:
Certain courses were more theory-based; all are interesting, yet impractical.
Due to limited funding the project is a bit slow to update and has less than 30 episodes.
Podcaster:
Tyler Renelle:
Machine learning engineer focused on time series and reinforcement
Background in full-stack JavaScript, 10 years web and mobile
Creator of HabitRPG, an app that treats habits as an RPG game
Links:
Machine Learning Guide podcast
Machine Learning Guide podcast (iTunes)
Tips on Listening:
Listen along your journey to help solidify understanding of topics.
Skip episodes 1, 3, 16, 21, and 26 (unless their topics interest and inspire you!).
Kaggle Machine Learning Track (Lesson 1) ↓
Need to Know: A simple code lab that covers the very basics of machine learning with scikit-learn and Panda through the application of the examples onto another set of data.
Loved:
A more active form of learning.
An engaging code lab that encourages participants to apply knowledge.
This track offers has a built-in Python notebook on Kaggle with all input files included. This removed any and all setup/installation issues.
Side note: It's a bit different than Jupyter notebook (e.g., have to click into a cell to add another cell).
Each lesson is short, which made the entire lesson go by very fast.
Disliked:
The writing in the first lesson didn't initially make it clear that one would need to apply the knowledge in the lesson to their workbook.
It wasn't a big deal, but when I started referencing files in the lesson, I had to dive into the files in my workbook to find they didn't exist, only to realize that the knowledge was supposed to be applied and not transcribed.
Lecturer:
Dan Becker:
Data Scientist at Kaggle
Undergrad in Computer Science, PhD in Econometrics
Supervised data science consultant for six Fortune 100 companies
Contributed to the Keras and Tensorflow libraries
Finished 2nd (out of 1353 teams) in $3 million Heritage Health Prize data mining competition
Speaks at deep learning workshops at events and conferences
Links:
https://www.kaggle.com/learn/machine-learning
Tips on Doing:
Read the exercises and apply to your dataset as you go.
Try lesson 2, which covers more complex/abstract topics (note: this second took a bit longer to work through).
Ready to commit
Fast.ai (part 1 of 2) ↓
Need to Know: Hands-down the most engaging and active form of learning ML. The source I would most recommend for anyone (although the training plan does help to build up to this course). This course is about learning through coding. This is the only course that I started to truly see the practical mechanics start to come together. It involves applying the most practical solutions to the most common problems (while also building an intuition for those solutions).
Loved:
Course Philosophy:
Active learning approach
"Go out into the world and understand underlying mechanics (of machine learning by doing)."
Counter-culture to the exclusivity of the machine learning field, focusing on inclusion.
"Let's do shit that matters to people as quickly as possible."
Highly pragmatic approach with tools that are currently being used (Jupyter Notebooks, scikit-learn, Keras, AWS, etc.).
Show an end-to-end process that you get to complete and play with in a development environment.
Math is involved, but is not prohibitive. Excel files helped to consolidate information/interact with information in a different way, and Jeremy spends a lot of time recapping confusing concepts.
Amazing set of learning resources that allow for all different styles of learning, including:
Video Lessons
Notes
Jupyter Notebooks
Assignments
Highly active forums
Resources on Stackoverflow
Readings/resources
Jeremy often references popular academic texts
Jeremy's TEDx talk in Brussels
Jeremy really pushes one to do extra and put in the effort by teaching interesting problems and engaging one in solving them.
It's a huge time commitment; however, it's worth it.
All of the course's profits are donated.
Disliked:
Overview covers their approach to learning (obviously I'm a fan!). If you're already drinking the Kool-aid, skip past.
I struggled through the AWS setup (13-minute video) for about five hours (however, it felt so good when it was up and running!).
Because of its practicality and concentration on solutions used today to solve popular problem types (image recognition, text generation, etc.), it lacks breadth of machine learning topics.
Lecturers:
Jeremy Howard:
Distinguished Research Scientist at the University of San Francisco
Faculty member at Singularity University
Young Global Leader with the World Economic Forum
Founder of Enlitic (the first company to apply deep learning to medicine)
Former President and Chief Scientist of the data science platform Kaggle
Rachel Thomas:
PhD in Math from Duke
One of Forbes' "20 Incredible Women Advancing AI Research"
Researcher-in-residence at the University of San Francisco Data Institute
Teaches in the Masters in Data Science program
Links:
http://course.fast.ai/start.html
http://wiki.fast.ai/index.php/Main_Page
https://github.com/fastai/courses/tree/master/deeplearning1/nbs
Tips on Doing:
Set expectations with yourself that installation is going to probably take a few hours.
Prepare to spend about ~70 hours for this course (it's worth it).
Don't forget to shut off your AWS instance.
Balance out machine learning knowledge with a course with more breadth.
Consider giving part two of the Fast.ai program a shot!
Hands-On Machine Learning with Scikit-Learn and TensorFlow: Concepts, Tools, and Techniques to Build Intelligent Systems ↓
Need to Know: This book is an Amazon best seller for a reason. It covers a lot of ground quickly, empowers readers to walk through a machine learning problem by chapter two, and contains practical up-to-date machine learning skills.
Loved:
Book contains an amazing introduction to machine learning that briskly provides an overarching quick view of the machine learning ecosystem.
Chapter 2 immediately walks the reader through an end-to-end machine learning problem.
Immediately afterwards, Aurélien pushes a user to attempt to apply this solution to another problem, which was very empowering.
There are review questions at the end of each chapter to ensure on has grasped the content within the chapter and to push the reader to explore more.
Once installation was completed, it was easy to follow and all code is available on GitHub.
Chapters 11-14 were very tough reading; however, they were a great reference when working through Fast.ai.
Contains some powerful analogies.
Each chapter's introductions were very useful and put everything into context. This general-to-specifics learning was very useful.
Disliked:
Installation was a common source of issues during the beginning of my journey; the text glided over this. I felt the frustration that most people experience from installation should have been addressed with more resources.
Writer:
Aurélien Géron:
Led the YouTube video classification team from 2013 to 2016
Currently a machine Learning consultant
Founder and CTO of Wifirst and Polyconseil
Published technical books (on C++, Wi-Fi, and Internet architectures)
Links:
https://www.amazon.com/_/dp/1491962291?tag=oreilly20-20
http://shop.oreilly.com/product/0636920052289.do
https://github.com/ageron/handson-ml
Tips on Using:
Get a friend with Python experience to help with installation.
Read the introductions to each chapter thoroughly, read the chapter (pay careful attention to code), review the questions at the end (highlight any in-text answer), make a copy of Aurélien's GitHub and make sure everything works on your setup, re-type the notebooks, go to Kaggle and try on other datasets.
Broadening your horizons
Udacity: Intro to Machine Learning (Kate/Sebastian) ↓
Need to Know: A course that covers a range of machine learning topics, supports building of intuition via visualization and simple examples, offers coding challenges, and a certificate (upon completion of a final project). The biggest challenge with this course is bridging the gap between the hand-holding lectures and the coding exercises.
Loved:
Focus on developing a visual intuition on what each model is trying to accomplish.
This visual learning mathematics approach is very useful.
Cover a vast variety and breadth of models and machine learning basics.
In terms of presenting the concept, there was a lot of hand-holding (which I completely appreciated!).
Many people have done this training, so their GitHub accounts can be used as reference for the mini-projects.
Katie actively notes documentation and suggests where viewers can learn more/reference material.
Disliked:
All of the conceptual hand-holding in the lessons is a stark contrast to the challenges of installation, coding exercises, and mini-projects.
This is the first course started and the limited instructions on setting up the environment and many failed attempts caused me to break down crying at least a handful of times.
The mini-projects are intimidating.
There is extra code added to support the viewers; however, it's done so with little acknowledgement as to what it's actually doing. This made learning a bit harder.
Lecturer:
Caitlin (Katie) Malone:
Director of Data Science Research and Development at Civis Analytics
Stanford PhD in Experimental Particle Physics
Intern at Udacity in summer 2014
Graduate Researcher at the SLAC National Accelerator Laboratory
https://www6.slac.stanford.edu/
Podcaster with Ben Jaffe (currently Facebook UI Engineer and a music aficionado) on a machine learning podcast Linear Digressions (100+ episodes)
Sebastian Thrun:
CEO of the Kitty Hawk Corporation
Chairman and co-founder of Udacity
One of my favorite Sebastian quotes: "It occurred to me, I could be at Google and build a self-driving car, or I can teach 10,000 students how to build self-driving cars."
Former Google VP
Founded Google X
Led development of the robotic vehicle Stanley
Professor of Computer Science at Stanford University
Formerly a professor at Carnegie Mellon University.
Links:
https://www.udacity.com/course/intro-to-machine-learning--ud120
Udacity also offers a next step, the Machine Learning Engineer Nanodegree, which will set one back about $1K.
Tips on Watching:
Get a friend to help you set up your environment.
Print mini-project instructions to check off each step.
Andrew Ng's Coursera Machine Learning Course ↓
Need to Know: The Andrew Ng Coursera course is the most referenced online machine learning course. It covers a broad set of fundamental, evergreen topics with a strong focus in building mathematical intuition behind machine learning models. Also, one can submit assignments and earn a grade for free. If you want to earn a certificate, one can subscribe or apply for financial aid.
Loved:
This course has a high level of credibility.
Introduces all necessary machine learning terminology and jargon.
Contains a very classic machine learning education approach with a high level of math focus.
Quizzes interspersed in courses and after each lesson support understanding and overall learning.
The sessions for the course are flexible, the option to switch into a different section is always available.
Disliked:
The mathematic notation was hard to process at times.
The content felt a bit dated and non-pragmatic. For example, the main concentration was MATLAB and Octave versus more modern languages and resources.
Video quality was less than average and could use a refresh.
Lecturer:
Andrew Ng:
Adjunct Professor, Stanford University (focusing on AI, Machine Learning, and Deep Learning)
Co-founder of Coursera
Former head of Baidu AI Group
Founder and previous head of Google Brain (deep learning) project
Former Director of the Stanford AI Lab
Chairman of the board of Woebot (a machine learning bot that focuses on Cognitive Behavior Therapy)
Links:
https://www.coursera.org/learn/machine-learning/
Andrew Ng recently launched a new course (August 2017) called DeepLearning.ai, a ~15 week course containing five mini-courses ($49 USD per month to continue learning after trial period of 7 days ends).
Course: https://www.coursera.org/specializations/deep-learning
Course 1: Neural Networks and Deep Learning
Course 2: Improving Deep Neural Networks: Hyperparameter tuning, Regularization and Optimization
Course 3: Structuring Machine Learning Projects
Course 4: Convolutional Neural Networks
Course 5: Sequence Models
Tips on Watching:
Be disciplined with setting aside timing (even if it's only 15 minutes a day) to help power through some of the more boring concepts.
Don't do this course first, because it's intimidating, requires a large time commitment, and isn't a very energizing experience.
Additional machine learning opportunities
iPullRank Machine Learning Guide ↓
Need to Know: A machine learning e-book targeted at marketers.
Loved:
Targeted at marketers and applied to organic search.
Covers a variety of machine learning topics.
Some good examples, including real-world blunders.
Gives some practical tools for non-data scientists (including: MonkeyLearn and Orange)
I found Orange to be a lot of fun. It struggled with larger datasets; however, it has a very visual interface that was more user-friendly and offers potential to show some pretty compelling stories.
Example: World Happiness Dataset by:
X-axis: Happiness Score
Y-axis: Economy
Color: Health
Disliked:
Potential to break up content more with relevant imagery -- the content was very dense.
Writers:
iPullRank Team (including Mike King):
Mike King has a few slide decks on the basics of machine learnings and AI
iPullRank has a few data scientists on staff
Links:
http://ipullrank.com/machine-learning-guide/
Tips on Reading:
Read chapters 1-6 and the rest depending upon personal interest.
Review Google PhD ↓
Need to Know: A two-hour presentation from Google's 2017 IO conference that walks through getting 99% accuracy on the MNIST dataset (a famous dataset containing a bunch of handwritten numbers, which the machine must learn to identify the numbers).
Loved:
This talk struck me as very modern, covering the cutting edge.
Found this to be very complementary to Fast.ai, as it covered similar topics (e.g. ReLu, CNNs, RNNs, etc.)
Amazing visuals that help to put everything into context.
Disliked:
The presentation is only a short conference solution and not a comprehensive view of machine learning.
Also, a passive form of learning.
Presenter:
Martin Görner:
Developer Relations, Google (since 2011)
Started Mobipocket, a startup that later became the software part of the Amazon Kindle and its mobile variants
Links:
Part 1 - https://www.youtube.com/watch?v=u4alGiomYP4
Part 2 - https://www.youtube.com/watch?v=fTUwdXUFfI8
Tips on Watching:
Google any concepts you're unfamiliar with.
Take your time with this one; 2 hours of screen time doesn't count all of the Googling and processing time for this one.
Caltech Machine Learning iTunes ↓
Need to Know: If math is your thing, this course does a stellar job of building the mathematic intuition behind many machine learning models. Dr. Abu-Mostafa is a raconteur, includes useful visualizations, relevant real-world examples, and compelling analogies.
Loved:
First and foremost, this is a real Caltech course, meaning it's not a watered-down version and contains fundamental concepts that are vital to understanding the mechanics of machine learning.
On iTunes, audio downloads are available, which can be useful for on-the-go learning.
Dr. Abu-Mostafa is a skilled speaker, making the 27 hours spent listening much easier!
Dr. Abu-Mostafa offers up some strong real-world examples and analogies which makes the content more relatable.
As an example, he asks students: "Why do I give you practice exams and not just give you the final exam?" as an illustration of why a testing set is useful. If he were to just give students the final, they would just memorize the answers (i.e., they would overfit to the data) and not genuinely learn the material. The final is a test to show how much students learn.
The last 1/2 hour of the class is always a Q&A, where students can ask questions. Their questions were useful to understanding the topic more in-depth.
The video and audio quality was strong throughout. There were a few times when I couldn't understand a question in the Q&A, but overall very strong.
This course is designed to build mathematical intuition of what's going on under the hood of specific machine learning models.
Caution: Dr. Abu-Mostafa uses mathematical notation, but it's different from Andrew Ng's (e.g., theta = w).
The final lecture was the most useful, as it pulled a lot of the conceptual puzzle pieces together. The course on neural networks was a close second!
Disliked:
Although it contains mostly evergreen content, being released in 2012, it could use a refresh.
Very passive form of learning, as it wasn't immediately actionable.
Lecturer:
Dr. Yaser S. Abu-Mostafa:
Professor of Electrical Engineering and Computer Science at the California Institute of Technology
Chairman of Machine Learning Consultants LLC
Serves on a number of scientific advisory boards
Has served as a technical consultant on machine learning for several companies (including Citibank).
Multiple articles in Scientific American
Links:
https://work.caltech.edu/telecourse.html
https://itunes.apple.com/us/course/machine-learning/id515364596
Tips on Watching:
Consider listening to the last lesson first, as it pulls together the course overall conceptually. The map of the course, below, was particularly useful to organizing the information taught in the courses.
Image source: http://work.caltech.edu/slides/slides18.pdf
"Pattern Recognition & Machine Learning" by Christopher Bishop ↓
Need to Know: This is a very popular college-level machine learning textbook. I've heard it likened to a bible for machine learning. However, after spending a month trying to tackle the first few chapters, I gave up. It was too much math and pre-requisites to tackle (even with a multitude of Google sessions).
Loved:
The text of choice for many major universities, so if you can make it through this text and understand all of the concepts, you're probably in a very good position.
I appreciated the history aside sections, where Bishop talked about influential people and their career accomplishments in statistics and machine learning.
Despite being a highly mathematically text, the textbook actually has some pretty visually intuitive imagery.
Disliked:
I couldn't make it through the text, which was a bit frustrating. The statistics and mathematical notation (which is probably very benign for a student in this topic) were too much for me.
The sunk cost was pretty high here (~$75).
Writer:
Christopher Bishop:
Laboratory Director at Microsoft Research Cambridge
Professor of Computer Science at the University of Edinburgh
Fellow of Darwin College, Cambridge
PhD in Theoretical Physics from the University of Edinburgh
Links:
https://www.amazon.com/Pattern-Recognition-Learning-Information-Statistics/dp/0387310738/ref=sr_1_2?ie=UTF8&qid=1516839475&sr=8-2&keywords=Pattern+Recognition+%26+Machine+Learning
Tips on Reading:
Don't start your machine learning journey with this book.
Get a friend in statistics to walk you through anything complicated (my plan is to get a mentor in statistics).
Consider taking a (free) online statistics course (Khan Academy and Udacity both have some great content on statistics, calculus, math, and data analysis).
Machine Learning: Hands-on for Developers and Technical Professionals ↓
Need to Know: A fun, non-intimidating end-to-end launching pad/whistle stop for machine learning in action.
Loved:
Talks about practical issues that many other sources didn't really address (e.g. data-cleansing).
Covered the basics of machine learning in a non-intimidating way.
Offers abridged, consolidated versions of the content.
Added fun anecdotes that makes it easier to read.
Overall the writer has a great sense of humor.
Writer talks to the reader as if they're a real human being (i.e., doesn't expect you to go out and do proofs; acknowledges the challenge of certain concepts).
Covers a wide variety of topics.
Because it was well-written, I flew through the book (even though it's about ~300 pages).
Disliked:
N/A
Writer:
Jason Bell:
Technical architect, lecturer, and startup consultant
Data Engineer at MastodonC
Former section editor for Java Developer's Journal
Former writer on IBM DeveloperWorks
Links:
https://www.amazon.com/Machine-Learning-Hands-Developers-Professionals/dp/1118889061
https://www.wiley.com/en-us/Machine+Learning%3A+Hands+On+for+Developers+and+Technical+Professionals-p-9781118889060
Jason's Blog: https://dataissexy.wordpress.com/
Tips on Reading:
Download and explore Weka's interface beforehand.
Give some of the exercises a shot.
Introduction to Machine Learning with Python: A Guide for Data Scientists ↓
Need to Know: This was a was a well-written piece on machine learning, making it a quick read.
Loved:
Quick, smooth read.
Easy-to-follow code examples.
The first few chapters served as a stellar introduction to the basics of machine learning.
Contain subtle jokes that add a bit of fun.
Tip to use the Python package manager Anaconda with Jupyter Notebooks was helpful.
Disliked:
Once again, installation was a challenge.
The "mglearn" utility library threw me for a loop. I had to reread the first few chapters before I figured out it was support for the book.
Although I liked the book, I didn't love it. Overall it just missed the "empowering" mark.
Writers:
Andreas C. Müller:
PhD in Computer Science
Lecturer at the Data Science Institute at Columbia University
Worked at the NYU Center for Data Science on open source and open science
Former Machine Learning Scientist at Amazon
Speaks often on Machine Learning and scikit-learn (a popular machine learning library)
And he makes some pretty incredibly useful graphics, such as this scikit-learn cheat sheet:
Image source: http://peekaboo-vision.blogspot.com/2013/01/machin...
Sarah Guido:
Former senior data scientist at Mashable
Lead data scientist at Bitly
2018 SciPy Conference Data Science track co-chair
Links:
https://www.amazon.com/Introduction-Machine-Learning-Python-Scientists/dp/1449369413/ref=sr_1_7?s=books&ie=UTF8&qid=1516734322&sr=1-7&keywords=python+machine+learning
http://shop.oreilly.com/product/0636920030515.do
Tips on Reading:
Type out code examples.
Beware of the "mglearn" utility library.
Udacity: Machine Learning by Georgia Tech ↓
Need to Know: A mix between an online learning experience and a university machine learning teaching approach. The lecturers are fun, but the course still fell a bit short in terms of active learning.
Loved:
This class is offered as CS7641 at Georgia Tech, where it is a part of the Online Masters Degree. Although taking this course here will not earn credit towards the OMS degree, it's still a non-watered-down college teaching philosophy approach.
Covers a wide variety of topics, many of which reminded me of the Caltech course (including: VC Dimension versus Bayesian, Occam's razor, etc.)
Discusses Markov Decision Chains, which is something that didn't really come up in many other introductory machine learning course, but they are referenced within Google patents.
The lecturers have a great dynamic, are wicked smart, and displayed a great sense of (nerd) humor, which make the topics less intimidating.
The course has quizzes, which give the course a slight amount of interaction.
Disliked:
Some videos were very long, which made the content a bit harder to digest.
The course overall was very time consuming.
Despite the quizzes, the course was a very passive form of learning with no assignments and little coding.
Many videos started with a bunch of content already written out. Having the content written out was probably a big time-saver, but it was also a bit jarring for a viewer to see so much information all at once, while also trying to listen.
It's vital to pay very close attention to notation, which compounds in complexity quickly.
Tablet version didn't function flawlessly: some was missing content (which I had to mark down and review on a desktop), the app would crash randomly on the tablet, and sometimes the audio wouldn't start.
There were no subtitles available on tablet, which I found not only to be a major accessibility blunder, but also made it harder for me to process (since I'm not an audio learner).
Lecturer:
Michael Littman:
Professor of Computer Science at Brown University.
Was granted a patent for one of the earliest systems for Cross-language information retrieval
Perhaps the most interesting man in the world:
Been in two TEDx talks
How I Learned to Stop Worrying and Be Realistic About AI
A Cooperative Path to Artificial Intelligence
During his time at Duke, he worked on an automated crossword solver (PROVERB)
Has a Family Quartet
He has appeared in a TurboTax commercial
Charles Isbell:
Professor and Executive Associate Dean at School of Interactive Computing at Georgia Tech
Focus on statistical machine learning and "interactive" artificial intelligence.
Links:
https://www.udacity.com/course/machine-learning--ud262
Tips on Watching:
Pick specific topics of interest and focusing on those lessons.
Andrew Ng's Stanford's Machine Learning iTunes ↓
Need to Know: A non-watered-down Stanford course. It's outdated (filmed in 2008), video/audio are a bit poor, and most links online now point towards the Coursera course. Although the idea of watching a Stanford course was energizing for the first few courses, it became dreadfully boring. I made it to course six before calling it.
Loved:
Designed for students, so you know you're not missing out on anything.
This course provides a deeper study into the mathematical and theoretical foundation behind machine learning to the point that the students could create their own machine learning algorithms. This isn't necessarily very practical for the everyday machine learning user.
Has some powerful real-world examples (although they're outdated).
There is something about the kinesthetic nature of watching someone write information out. The blackboard writing helped me to process certain ideas.
Disliked:
Video and audio quality were pain to watch.
Many questions asked by students were hard to hear.
On-screen visuals range from hard to impossible to see.
Found myself counting minutes.
Dr. Ng mentions TA classes, supplementary learning, but these are not available online.
Sometimes the video showed students, which I felt was invasive.
Lecturer:
Andrew Ng (see above)
Links:
https://itunes.apple.com/us/course/machine-learning/id495053006
https://www.youtube.com/watch?v=UzxYlbK2c7E
Tips on Watching:
Only watch if you're looking to gain a deeper understanding of the math presented in the Coursera course.
Skip the first half of the first lecture, since it's mostly class logistics.
Additional Resources
Fast.ai (part 2) - free access to materials, cost for AWS EC2 instance
Deeplearning.ai - $50/month
Udacity Machine Learning Engineer Nanodegree - $1K
https://machinelearningmastery.com/
Motivations and inspiration
If you're wondering why I spent a year doing this, then I'm with you. I'm genuinely not sure why I set my sights on this project, much less why I followed through with it. I saw Mike King give a session on Machine Learning. I was caught off guard, since I knew nothing on the topic. It gave me a pesky, insatiable curiosity itch. It started with one course and then spiraled out of control. Eventually it transformed into an idea: a review guide on the most affordable and popular machine learning resources on the web (through the lens of a complete beginner). Hopefully you found it useful, or at least somewhat interesting. Be sure to share your thoughts or questions in the comments!
Sign up for The Moz Top 10, a semimonthly mailer updating you on the top ten hottest pieces of SEO news, tips, and rad links uncovered by the Moz team. Think of it as your exclusive digest of stuff you don't have time to hunt down but want to read!
bạn xem thêm tại: https://ift.tt/2mXjlRS A Machine Learning Guide for Average Humans xem thêm tại: https://ift.tt/2mb4VST để biết thêm về địa chỉ bán tai nghe không dây giá rẻ A Machine Learning Guide for Average Humans https://ift.tt/2rFyAUY xem thêm tại: https://ift.tt/2mb4VST để biết thêm về địa chỉ bán tai nghe không dây giá rẻ A Machine Learning Guide for Average Humans https://ift.tt/2rFyAUY xem thêm tại: https://ift.tt/2mb4VST để biết thêm về địa chỉ bán tai nghe không dây giá rẻ A Machine Learning Guide for Average Humans https://ift.tt/2rFyAUY xem thêm tại: https://ift.tt/2mb4VST để biết thêm về địa chỉ bán tai nghe không dây giá rẻ A Machine Learning Guide for Average Humans https://ift.tt/2rFyAUY xem thêm tại: https://ift.tt/2mb4VST để biết thêm về địa chỉ bán tai nghe không dây giá rẻ A Machine Learning Guide for Average Humans https://ift.tt/2rFyAUY xem thêm tại: https://ift.tt/2mb4VST để biết thêm về địa chỉ bán tai nghe không dây giá rẻ A Machine Learning Guide for Average Humans https://ift.tt/2rFyAUY xem thêm tại: https://ift.tt/2mb4VST để biết thêm về địa chỉ bán tai nghe không dây giá rẻ A Machine Learning Guide for Average Humans https://ift.tt/2rFyAUY xem thêm tại: https://ift.tt/2mb4VST để biết thêm về địa chỉ bán tai nghe không dây giá rẻ A Machine Learning Guide for Average Humans https://ift.tt/2rFyAUY xem thêm tại: https://ift.tt/2mb4VST để biết thêm về địa chỉ bán tai nghe không dây giá rẻ A Machine Learning Guide for Average Humans https://ift.tt/2rFyAUY xem thêm tại: https://ift.tt/2mb4VST để biết thêm về địa chỉ bán tai nghe không dây giá rẻ A Machine Learning Guide for Average Humans https://ift.tt/2rFyAUY xem thêm tại: https://ift.tt/2mb4VST để biết thêm về địa chỉ bán tai nghe không dây giá rẻ A Machine Learning Guide for Average Humans https://ift.tt/2rFyAUY xem thêm tại: https://ift.tt/2mb4VST để biết thêm về địa chỉ bán tai nghe không dây giá rẻ A Machine Learning Guide for Average Humans https://ift.tt/2rFyAUY xem thêm tại: https://ift.tt/2mb4VST để biết thêm về địa chỉ bán tai nghe không dây giá rẻ A Machine Learning Guide for Average Humans https://ift.tt/2rFyAUY xem thêm tại: https://ift.tt/2mb4VST để biết thêm về địa chỉ bán tai nghe không dây giá rẻ A Machine Learning Guide for Average Humans https://ift.tt/2rFyAUY xem thêm tại: https://ift.tt/2mb4VST để biết thêm về địa chỉ bán tai nghe không dây giá rẻ A Machine Learning Guide for Average Humans https://ift.tt/2rFyAUY xem thêm tại: https://ift.tt/2mb4VST để biết thêm về địa chỉ bán tai nghe không dây giá rẻ A Machine Learning Guide for Average Humans https://ift.tt/2rFyAUY xem thêm tại: https://ift.tt/2mb4VST để biết thêm về địa chỉ bán tai nghe không dây giá rẻ A Machine Learning Guide for Average Humans https://ift.tt/2rFyAUY xem thêm tại: https://ift.tt/2mb4VST để biết thêm về địa chỉ bán tai nghe không dây giá rẻ A Machine Learning Guide for Average Humans https://ift.tt/2rFyAUY xem thêm tại: https://ift.tt/2mb4VST để biết thêm về địa chỉ bán tai nghe không dây giá rẻ A Machine Learning Guide for Average Humans https://ift.tt/2rFyAUY xem thêm tại: https://ift.tt/2mb4VST để biết thêm về địa chỉ bán tai nghe không dây giá rẻ A Machine Learning Guide for Average Humans https://ift.tt/2rFyAUY xem thêm tại: https://ift.tt/2mb4VST để biết thêm về địa chỉ bán tai nghe không dây giá rẻ A Machine Learning Guide for Average Humans https://ift.tt/2rFyAUY xem thêm tại: https://ift.tt/2mb4VST để biết thêm về địa chỉ bán tai nghe không dây giá rẻ A Machine Learning Guide for Average Humans https://ift.tt/2rFyAUY xem thêm tại: https://ift.tt/2mb4VST để biết thêm về địa chỉ bán tai nghe không dây giá rẻ A Machine Learning Guide for Average Humans https://ift.tt/2rFyAUY xem thêm tại: https://ift.tt/2mb4VST để biết thêm về địa chỉ bán tai nghe không dây giá rẻ A Machine Learning Guide for Average Humans https://ift.tt/2rFyAUY xem thêm tại: https://ift.tt/2mb4VST để biết thêm về địa chỉ bán tai nghe không dây giá rẻ A Machine Learning Guide for Average Humans https://ift.tt/2rFyAUY xem thêm tại: https://ift.tt/2mb4VST để biết thêm về địa chỉ bán tai nghe không dây giá rẻ A Machine Learning Guide for Average Humans https://ift.tt/2rFyAUY xem thêm tại: https://ift.tt/2mb4VST để biết thêm về địa chỉ bán tai nghe không dây giá rẻ A Machine Learning Guide for Average Humans https://ift.tt/2rFyAUY xem thêm tại: https://ift.tt/2mb4VST để biết thêm về địa chỉ bán tai nghe không dây giá rẻ A Machine Learning Guide for Average Humans https://ift.tt/2rFyAUY xem thêm tại: https://ift.tt/2mb4VST để biết thêm về địa chỉ bán tai nghe không dây giá rẻ A Machine Learning Guide for Average Humans https://ift.tt/2rFyAUY xem thêm tại: https://ift.tt/2mb4VST để biết thêm về địa chỉ bán tai nghe không dây giá rẻ A Machine Learning Guide for Average Humans https://ift.tt/2rFyAUY xem thêm tại: https://ift.tt/2mb4VST để biết thêm về địa chỉ bán tai nghe không dây giá rẻ A Machine Learning Guide for Average Humans https://ift.tt/2rFyAUY xem thêm tại: https://ift.tt/2mb4VST để biết thêm về địa chỉ bán tai nghe không dây giá rẻ A Machine Learning Guide for Average Humans https://ift.tt/2rFyAUY xem thêm tại: https://ift.tt/2mb4VST để biết thêm về địa chỉ bán tai nghe không dây giá rẻ A Machine Learning Guide for Average Humans https://ift.tt/2rFyAUY xem thêm tại: https://ift.tt/2mb4VST để biết thêm về địa chỉ bán tai nghe không dây giá rẻ A Machine Learning Guide for Average Humans https://ift.tt/2rFyAUY xem thêm tại: https://ift.tt/2mb4VST để biết thêm về địa chỉ bán tai nghe không dây giá rẻ A Machine Learning Guide for Average Humans https://ift.tt/2rFyAUY xem thêm tại: https://ift.tt/2mb4VST để biết thêm về địa chỉ bán tai nghe không dây giá rẻ A Machine Learning Guide for Average Humans https://ift.tt/2rFyAUY xem thêm tại: https://ift.tt/2mb4VST để biết thêm về địa chỉ bán tai nghe không dây giá rẻ A Machine Learning Guide for Average Humans https://ift.tt/2rFyAUY xem thêm tại: https://ift.tt/2mb4VST để biết thêm về địa chỉ bán tai nghe không dây giá rẻ A Machine Learning Guide for Average Humans https://ift.tt/2rFyAUY xem thêm tại: https://ift.tt/2mb4VST để biết thêm về địa chỉ bán tai nghe không dây giá rẻ A Machine Learning Guide for Average Humans https://ift.tt/2rFyAUY xem thêm tại: https://ift.tt/2mb4VST để biết thêm về địa chỉ bán tai nghe không dây giá rẻ A Machine Learning Guide for Average Humans https://ift.tt/2rFyAUY xem thêm tại: https://ift.tt/2mb4VST để biết thêm về địa chỉ bán tai nghe không dây giá rẻ A Machine Learning Guide for Average Humans https://ift.tt/2rFyAUY xem thêm tại: https://ift.tt/2mb4VST để biết thêm về địa chỉ bán tai nghe không dây giá rẻ A Machine Learning Guide for Average Humans https://ift.tt/2rFyAUY xem thêm tại: https://ift.tt/2mb4VST để biết thêm về địa chỉ bán tai nghe không dây giá rẻ A Machine Learning Guide for Average Humans https://ift.tt/2rFyAUY xem thêm tại: https://ift.tt/2mb4VST để biết thêm về địa chỉ bán tai nghe không dây giá rẻ A Machine Learning Guide for Average Humans https://ift.tt/2rFyAUY xem thêm tại: https://ift.tt/2mb4VST để biết thêm về địa chỉ bán tai nghe không dây giá rẻ A Machine Learning Guide for Average Humans https://ift.tt/2rFyAUY xem thêm tại: https://ift.tt/2mb4VST để biết thêm về địa chỉ bán tai nghe không dây giá rẻ A Machine Learning Guide for Average Humans https://ift.tt/2rFyAUY xem thêm tại: https://ift.tt/2mb4VST để biết thêm về địa chỉ bán tai nghe không dây giá rẻ A Machine Learning Guide for Average Humans https://ift.tt/2rFyAUY xem thêm tại: https://ift.tt/2mb4VST để biết thêm về địa chỉ bán tai nghe không dây giá rẻ A Machine Learning Guide for Average Humans https://ift.tt/2rFyAUY xem thêm tại: https://ift.tt/2mb4VST để biết thêm về địa chỉ bán tai nghe không dây giá rẻ A Machine Learning Guide for Average Humans https://ift.tt/2rFyAUY xem thêm tại: https://ift.tt/2mb4VST để biết thêm về địa chỉ bán tai nghe không dây giá rẻ A Machine Learning Guide for Average Humans https://ift.tt/2rFyAUY xem thêm tại: https://ift.tt/2mb4VST để biết thêm về địa chỉ bán tai nghe không dây giá rẻ A Machine Learning Guide for Average Humans https://ift.tt/2rFyAUY xem thêm tại: https://ift.tt/2mb4VST để biết thêm về địa chỉ bán tai nghe không dây giá rẻ A Machine Learning Guide for Average Humans https://ift.tt/2rFyAUY xem thêm tại: https://ift.tt/2mb4VST để biết thêm về địa chỉ bán tai nghe không dây giá rẻ A Machine Learning Guide for Average Humans https://ift.tt/2rFyAUY xem thêm tại: https://ift.tt/2mb4VST để biết thêm về địa chỉ bán tai nghe không dây giá rẻ A Machine Learning Guide for Average Humans https://ift.tt/2rFyAUY xem thêm tại: https://ift.tt/2mb4VST để biết thêm về địa chỉ bán tai nghe không dây giá rẻ A Machine Learning Guide for Average Humans https://ift.tt/2rFyAUY xem thêm tại: https://ift.tt/2mb4VST để biết thêm về địa chỉ bán tai nghe không dây giá rẻ A Machine Learning Guide for Average Humans https://ift.tt/2rFyAUY xem thêm tại: https://ift.tt/2mb4VST để biết thêm về địa chỉ bán tai nghe không dây giá rẻ A Machine Learning Guide for Average Humans https://ift.tt/2rFyAUY xem thêm tại: https://ift.tt/2mb4VST để biết thêm về địa chỉ bán tai nghe không dây giá rẻ A Machine Learning Guide for Average Humans https://ift.tt/2rFyAUY xem thêm tại: https://ift.tt/2mb4VST để biết thêm về địa chỉ bán tai nghe không dây giá rẻ A Machine Learning Guide for Average Humans https://ift.tt/2rFyAUY xem thêm tại: https://ift.tt/2mb4VST để biết thêm về địa chỉ bán tai nghe không dây giá rẻ A Machine Learning Guide for Average Humans https://ift.tt/2rFyAUY xem thêm tại: https://ift.tt/2mb4VST để biết thêm về địa chỉ bán tai nghe không dây giá rẻ A Machine Learning Guide for Average Humans https://ift.tt/2rFyAUY xem thêm tại: https://ift.tt/2mb4VST để biết thêm về địa chỉ bán tai nghe không dây giá rẻ A Machine Learning Guide for Average Humans https://ift.tt/2rFyAUY xem thêm tại: https://ift.tt/2mb4VST để biết thêm về địa chỉ bán tai nghe không dây giá rẻ A Machine Learning Guide for Average Humans https://ift.tt/2rFyAUY xem thêm tại: https://ift.tt/2mb4VST để biết thêm về địa chỉ bán tai nghe không dây giá rẻ A Machine Learning Guide for Average Humans https://ift.tt/2rFyAUY xem thêm tại: https://ift.tt/2mb4VST để biết thêm về địa chỉ bán tai nghe không dây giá rẻ A Machine Learning Guide for Average Humans https://ift.tt/2rFyAUY xem thêm tại: https://ift.tt/2mb4VST để biết thêm về địa chỉ bán tai nghe không dây giá rẻ A Machine Learning Guide for Average Humans https://ift.tt/2rFyAUY xem thêm tại: https://ift.tt/2mb4VST để biết thêm về địa chỉ bán tai nghe không dây giá rẻ A Machine Learning Guide for Average Humans https://ift.tt/2rFyAUY xem thêm tại: https://ift.tt/2mb4VST để biết thêm về địa chỉ bán tai nghe không dây giá rẻ A Machine Learning Guide for Average Humans https://ift.tt/2rFyAUY xem thêm tại: https://ift.tt/2mb4VST để biết thêm về địa chỉ bán tai nghe không dây giá rẻ A Machine Learning Guide for Average Humans https://ift.tt/2rFyAUY xem thêm tại: https://ift.tt/2mb4VST để biết thêm về địa chỉ bán tai nghe không dây giá rẻ A Machine Learning Guide for Average Humans https://ift.tt/2rFyAUY xem thêm tại: https://ift.tt/2mb4VST để biết thêm về địa chỉ bán tai nghe không dây giá rẻ A Machine Learning Guide for Average Humans https://ift.tt/2rFyAUY xem thêm tại: https://ift.tt/2mb4VST để biết thêm về địa chỉ bán tai nghe không dây giá rẻ A Machine Learning Guide for Average Humans https://ift.tt/2rFyAUY xem thêm tại: https://ift.tt/2mb4VST để biết thêm về địa chỉ bán tai nghe không dây giá rẻ A Machine Learning Guide for Average Humans https://ift.tt/2rFyAUY xem thêm tại: https://ift.tt/2mb4VST để biết thêm về địa chỉ bán tai nghe không dây giá rẻ A Machine Learning Guide for Average Humans https://ift.tt/2rFyAUY xem thêm tại: https://ift.tt/2mb4VST để biết thêm về địa chỉ bán tai nghe không dây giá rẻ A Machine Learning Guide for Average Humans https://ift.tt/2rFyAUY xem thêm tại: https://ift.tt/2mb4VST để biết thêm về địa chỉ bán tai nghe không dây giá rẻ A Machine Learning Guide for Average Humans https://ift.tt/2rFyAUY xem thêm tại: https://ift.tt/2mb4VST để biết thêm về địa chỉ bán tai nghe không dây giá rẻ A Machine Learning Guide for Average Humans https://ift.tt/2rFyAUY xem thêm tại: https://ift.tt/2mb4VST để biết thêm về địa chỉ bán tai nghe không dây giá rẻ A Machine Learning Guide for Average Humans https://ift.tt/2rFyAUY xem thêm tại: https://ift.tt/2mb4VST để biết thêm về địa chỉ bán tai nghe không dây giá rẻ A Machine Learning Guide for Average Humans https://ift.tt/2rFyAUY xem thêm tại: https://ift.tt/2mb4VST để biết thêm về địa chỉ bán tai nghe không dây giá rẻ A Machine Learning Guide for Average Humans https://ift.tt/2rFyAUY xem thêm tại: https://ift.tt/2mb4VST để biết thêm về địa chỉ bán tai nghe không dây giá rẻ A Machine Learning Guide for Average Humans https://ift.tt/2rFyAUY xem thêm tại: https://ift.tt/2mb4VST để biết thêm về địa chỉ bán tai nghe không dây giá rẻ A Machine Learning Guide for Average Humans https://ift.tt/2rFyAUY Bạn có thể xem thêm địa chỉ mua tai nghe không dây tại đây https://ift.tt/2mb4VST
0 notes