#dnn development
Explore tagged Tumblr posts
Text
1 note
·
View note
Link
DNN is an ideal solution for creating wide range of commercial applications and websites that allows using third-party products and add-ons or custom tools. This is highly recommended by C# software development companies USA. DNN eases the work of developers, designers, administrators, editors, and other users of the system or website. As DNN’s skinning engine is extremely flexible, it is easy to get better and as per user requirement attractive UI. DNN has the ability for the administrations tools to fully integrate into the websites which makes it easy to modify things. It is used to make professional websites, social intranets, community portals, etc. and mostly preferred for medium sized eCommerce.
0 notes
Text
#dotnetnuke web development#dnn services#dotnetnuke development services#dnn developer#dnn development
0 notes
Text
2 notes
·
View notes
Text
Our experience as a DNN developer has given the DyNNamite team unique insight and capabilities in DNN custom module development.
0 notes
Text

Image denoising using a diffractive material
While image denoising algorithms have undergone extensive research and advancements in the past decades, classical denoising techniques often necessitate numerous iterations for their inference, making them less suitable for real-time applications. The advent of deep neural networks (DNNs) has ushered in a paradigm shift, enabling the development of non-iterative, feed-forward digital image denoising approaches. These DNN-based methods exhibit remarkable efficacy, achieving real-time performance while maintaining high denoising accuracy. However, these deep learning-based digital denoisers incur a trade-off, demanding high-cost, resource- and power-intensive graphics processing units (GPUs) for operation.
Read more.
12 notes
·
View notes
Text
rules rambling: stats and intention
i think if i had played it safer and kept rules about georgenap-centric dream exclusion in dnn and the infidelity resulting from that it would have done better and the punishment scene with dream after the christmas streams was just too far and made a lot of people give up on it. like thats kinda what i expect happened based on what ive seen in the stats especially compared to similar yet different but comparable works of mine (home security no angst with smut ratio vs best friend eater heavy angst and no smut ratio)
but at the same time from my perspective as a writer... that's where it all started. my original idea for rules was this one passed around screenshot from the christmas streams of dnf at one end of the kitchen and sapnap at the other and everyone was sharing it gassing up dnf and i was like what if it was secretly georgenap/dnn and george got mad at dream for being extra pda-y on camera with him and 'excluding' sapnap like the fans say. that was the whole premise of the fic it came from this idea that george would have those feelings and the way his and dream's characters developped it lead pretty naturally to an unhealthy punishment scene
and then of course there's the ridiculous fact that i was just going to write that general idea and suddenly georgenap were having the UK trip. and i had to get to christmas 2022. it got a little out of my hands.
rules isnt porn with plot the porn IS the plot all of the gritty shit that gives it substance and makes it interesting happens mostly within the smut scenes because thats kind of how my brain works as a smut writer. also its only natural that george, someone who doesnt communicate properly in his relationships, would rely heavily on physical touch and intimacy to articulate his love (and anger in dream's case) and that's the whole issue, right? i touched on love languages a little bit in another rules post so i wont get distracted.
dream's punishment was fucked. and he was way more effected by it in the moment than i intended but while i was writing the scene it just kept getting darker and darker and i had to keep an eye on sapanap's reactions kind of like a timer. so since it took sapnap so long to speak up the punishment just gets so fucking cruel. and dream as noted in the fic is such an under-experienced sub who relies heavily on praise to keep his head in-scene... it was fucked. it was fucked up.
but he does get to chew george out for it! completely tears him to shreds and give him a taste of how it feels to be miserable like that, and then later in a better headspace they explore that vulnerability of subbing/being penetrated too. the ending of rules is so fucking good. sure georgenap is slightly unresolved but dnf really have an incredible dynamic that i thought came out really good. it's a shame not everyone who had their heart muscles strained from the nonstop 60k of angst got the tiger balm soothing of resolution, but maybe i didn't tag the happy ending soon enough.
15 notes
·
View notes
Note
i’ve been having dnkn thoughts that i was thinking of somehow incorporating into a fic but probably not so i am now unleashing them onto your inbox. really in love with the idea of karl being the “missing piece” so to speak that breaks down their walls and makes them finally start acting on their feelings for each other. like dnn all have individually thought about their friendship being something more, but they don’t want to mess up what they already have by pushing on invisible boundaries, so for the most part they keep it to themselves and just carry on as normal. but then in swoops karl, who so openly and easily gives affection that it becomes more comfortable for all of them. it starts with sapnap, and then george, and finally dream, and just as the three of them are starting to actually navigate their feelings for each other they start to realize their developing feelings for karl as well, which is briefly terrifying for both parties (dteam because despite the fact that they’re now happy with each other, they’re all now in love with another one of their friends who’s also partially the reason they got together in the first place, and poor karl who slowly falls in love with not one but three of his best friends who he’s also convinced are perfect for each other already) but then they all end up confessing to each other and it turns out they had nothing to worry about bc they all love each other anyways. thats really all i got to say dnkn in gay love yippeee🎉🏳️🌈🫶
dnkn in gay love yippeee !!!
oh anon :') ugh that's so so cute. gonna be honest i LOVE the concept of Karl swooping in on an established relationship and the relationship having a gay panic bc kind, funny, and physically affectionate Karl just meshes so well with them? like they thought they truly found what they've been looking for but then Karl comes along and suddenly their relationship + Karl becomes what they were looking for and it's scary but it's so easy for them to talk about bc Karl is so blatant that they can cast it off as a joke:
"did you see the way Karl was hanging off of Sapnap today?"
"yeah. kinda hot ngl"
"oh. you too huh"
dnn realize they're all in love with Karl over a late night conversation and they just have to laugh bc they were getting all worried but wow there's something to say about the fact that they all have similar tastes
one of my favorite things to think about is cuddly dnkn all squished in bed together :') Sapnap and George getting competitive about where they are in the pile. Karl and George giggling and messing around between dreamnap. George accidentally kicking Dream while rolling around, trying to muffle his laughter. Dream hoisting George on the other side of him to separate them. sometimes Sapnap joins in on the shenanigans and Dream just gives up bc there's no way he can control all three of them (he can barely control George and Sapnap when they get going)
i could rant about it all day honestly :')
#dnkn#sigh ty anon for sharing !! if you ever do get around to making a fic please send it our way i'd love to read it :') <3#speaking of i really need to write a dnkn fic at some point#order up#🍟
13 notes
·
View notes
Text
hey, tell me that you want me to stay
Happy (late) Valentine’s Day, @dreamnotnapss! I hope you two have had an amazing and full of love month, enjoying (hopefully? haha) this gift for you. For now it's in Spanish (my first language), but in two or three days the translation should already be done:)
5 times where George unexpectedly popped in Dream/Sapnap’s streams, 1 time where the same thing happened, but in a different way. You’ll see why.
5 ocasiones en donde George apareció de improviso en las transmisiones de Dream y Sapnap, 1 ocasión en donde ocurrió lo mismo, pero de manera diferente.
Rating: T Relationships: DNN (Dream/Sapnap/George) Main tags: Polyamory, Developing Relationship, Chatting & Messaging, Domestic, 5+1 Things Language: Spanish - Español, in a few days the translation should be ok!
[Leer en AO3]
#dnn#dnn fic#dnn ao3#dreamnotnap#dreamnotnap fic#dreamnotnap ao3#dsmp fic#fanfic#ao3#español#soon translation#nahuel writes
4 notes
·
View notes
Text
PyTorch has developed over the past few years into a well-liked and often used framework for deep neural networks (DNN) training. about Pytorch 2.0 PyTorch’s popularity is credited to its ease of use, first-rate Python integration, and imperative programming approach.To know more about PyTorch 2.0, check out the Python training course.
2 notes
·
View notes
Text
New study on improving MRI image quality based on deep learning technology
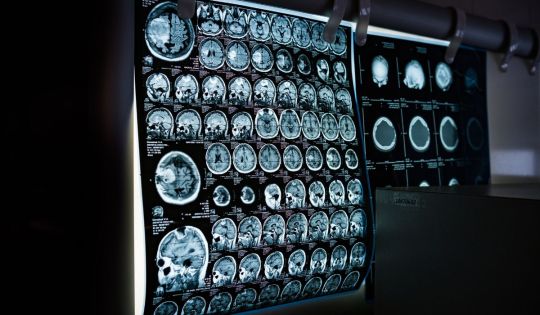
New study on improving MRI image quality based on deep learning technology published in European Radiology. SwiftMR™, an AI-powered MRI reconstruction solution from AIRS Medical proved its performance for enhancing the image quality of 3D high-resolution MRI. SEOUL, South Korea, Nov. 25, 2022 - A recent study published in European Radiology demonstrated that SwiftMR, an AI-powered MRI reconstruction solution from AIRS Medical, successfully denoises 3D MR images and improves its image quality by using routine clinical scans only. The study aimed to develop a deep neural network (DNN)–based noise reduction and image quality improvement by only using routine clinical scans and evaluating its performance in 3D high-resolution MRI. The study was conducted by AIRS Medical and Dr. Jinhee Jang, MD, Ph.D. of Seoul St. Mary's Hospital. The retrospective study included T1-weighted magnetization-prepared rapid gradient-echo (MP-RAGE) images from 185 clinical scans. Qualitative evaluation between conventional MP-RAGE and DNN-based MP-RAGE was performed by two radiologists in image quality, fine structure delineation, and lesion conspicuity. Quantitative evaluation was performed with full sampled data as a reference by measuring quantitative error metrics and volumetry at seven different simulated noise levels. DNN application on VWI was evaluated by two radiologists in image quality. The study shows that DNN-based MP-RAGE outperformed conventional MP-RAGE in all image quality parameters (average scores = 3.7 vs. 4.9, p < 0.001). In the quantitative evaluation, DNN showed better error metrics (p < 0.001) and comparable (p > 0.09) or better (p < 0.02) volumetry results than conventional MP-RAGE. DNN application to VWI also revealed improved image quality (3.5 vs. 4.6, p < 0.001). "We are very pleased that we have proved SwiftMR™ contributes to not only reducing time but also helps make images good to great, eventually contribute radiologists could read images with confidence." remarked Hyeseong Lee, MD, CEO of AIRS Medical. "We are expecting more adaptions and collaborations with radiologists around the world thus we could grow together." AIRS Medical recently announced its participation in the 108th Scientific Assembly and Annual Meetings of the RSNA 2022, held from November 27 to 30th in Chicago. During the event, AIRS Medical showcases its award-winning MRI reconstruction solution SwiftMR™and also delivering two oral presentations at scientific session. "We are proud of ourselves since it is quite unusual for a startup to deliver oral presentations at RSNA scientific session. Being adopted as oral presentation means recognition of academically important achievements." he added Read the full article
4 notes
·
View notes
Link
DotNetNuke is fairly easy to use CMS that requires less to nominal technical knowledge. It is also extensible to any third party apps for extra features and functionality as per requirement and it is highly recommended by DotNetNuke software companies in USA. It is designed for all types of websites and businesses.
One Stop Solution for Dot Net Development - Enquire Today
#dot ne#dnn#dotnetnuke#hire dot net developers#dot net development company#ASP.Net Software Development#Custom Software Development#hire software development agency
0 notes
Text
Introduction
In the digital age, data-driven decisions have become the cornerstone of successful businesses. Predictive analytics, powered by deep learning, offers unprecedented insights, enabling companies to anticipate trends and make informed choices. Our project, "Predictive Analytics on Business License Data Using Deep Learning Project," serves as a comprehensive introduction to deep neural networks (DNNs) and their application in real-world scenarios. By analyzing data from 86,000 businesses across various sectors, this project not only demystifies deep learning concepts but also demonstrates how they can be effectively utilized for predictive analytics.
The Importance of Predictive Analytics in Business
Predictive analytics uses historical data to forecast future events, helping businesses anticipate market changes, optimize operations, and enhance decision-making processes. In this project, we focus on business license data to predict the status of licenses, offering valuable insights into compliance trends, potential risks, and operational benchmarks.
Project Overview
Our project is designed to teach participants the fundamentals of deep neural networks (DNNs) through a hands-on approach. Using a dataset of business licenses, participants will learn essential steps such as Exploratory Data Analysis (EDA), data cleaning, and preparation. The project introduces key deep learning concepts like activation functions, feedforward, backpropagation, and dropout regularization, all within the context of building and evaluating DNN models.
Methodology
The project is structured into several key phases:
Data Exploration and Preparation:
Participants begin by exploring the dataset, identifying key features, and understanding the distribution of license statuses.
Data cleaning involves handling missing values, standardizing categorical variables, and transforming the data into a format suitable for modeling.
Building Baseline Models:
Before diving into deep learning, we create baseline models using the H2O framework. This step helps participants understand the importance of model comparison and sets the stage for more complex DNN models.
Deep Neural Networks (DNN) Development:
The core of the project involves building and training DNN models using TensorFlow. Participants learn how to design a neural network architecture, choose activation functions, implement dropout regularization, and fine-tune hyperparameters.
The model is trained to predict the status of business licenses based on various features, such as application type, license code, and business type.
Model Evaluation:
After training, the DNN model is evaluated on a test dataset to assess its performance. Participants learn to interpret metrics like accuracy, loss, and confusion matrices, gaining insights into the model's predictive power.
Results and Impact
The DNN model developed in this project demonstrates strong predictive capabilities, accurately classifying business license statuses. This model serves as a valuable tool for businesses and regulators, enabling them to anticipate compliance issues, streamline operations, and make data-driven decisions. Beyond the immediate application, participants gain a solid foundation in deep learning, preparing them for more advanced projects in the field of AI and machine learning.
Conclusion
The "Predictive Analytics on Business License Data Using Deep Learning" project offers a practical and educational journey into the world of deep learning. By engaging with real-world data and building predictive models, participants not only enhance their technical skills but also contribute to the broader field of AI-driven business analytics. This project underscores the transformative potential of deep learning in unlocking valuable insights from complex datasets, paving the way for more informed and strategic business decisions. You can download "Predictive Analytics on Business License Data Using Deep Learning Project (https://www.aionlinecourse.com/ai-projects/playground/predictive-analytics-on-business-license-data-using-deep-learningund/complete-cnn-image-classification-models-for-real-time-prediction)" from Aionlinecourse. Also you will get a live practice session on this playground.
0 notes
Text
New Tools and Technology Development will drive ENT Devices Market in coming years
ENT Devices Industry Overview
The global ENT devices market size was estimated at USD 25.93 billion in 2023 and is expected to grow at a compound annual growth rate (CAGR) of 5.54% from 2024 to 2030. This growth can be attributed to several factors, such as high prevalence of ENT-related disorders, an increase in the usage of minimally invasive ENT procedures, and rising geriatric population. Technological advancements also play a crucial role in driving the market growth.
The demand for advanced ENT devices, like robot-assisted endoscopes, is more in developed countries such as the U.S. while it is less in developing countries due to their high cost. The ENT devices market penetration is anticipated to grow significantly due to increasing healthcare spending by other governments and a rise in per capita income. Sales are expected to increase rapidly in developing economies due to high occurrences of ENT diseases such as hearing loss and sinusitis. Additionally, there is an increase in efforts to provide better access to healthcare facilities in these regions.
Gather more insights about the market drivers, restrains and growth of the ENT Devices Market
Hearing loss or impairment is a common condition among patients, particularly in industrialized countries. The World Health Organization reported, more than 430 million people worldwide, approximately 5% of the world's population, have a disabling hearing loss. This number is projected to rise to over 700 million, or one in every ten people, by 2050. The main reasons for this increase are growing life expectancy and noise pollution, leading to more age-related hearing loss cases. In low-income countries, infections such as middle ear infections, measles, or meningitis are the common causes of hearing loss. Moreover, vascular disorders, noise exposure, chronic inflammation, genetic susceptibility, physiological aging of the ear contributing to hearing impairment.
Rising technological advancements such as the adoption of AI and ML and innovations in auditory products are propelling industry growth. For instance, in September 2023, ELEHEAR Inc., an AI-powered hearing aids and audio solutions provider, introduced ELEHEAR Alpha Pro and ELEHEAR Alpha hearing aid devices. It is incorporated with AI noise reduction and extraction, which predicts daily users and their actions to minimize the effect of noise in typical audio environments such as public transit, offices, restaurants, homes, and busy streets. In March 2023, Oticon Medical A/S introduced new features in the processing chip Polaris R, which uses an onboard Deep Neural Network (DNN) for an entirely new method of sound processing. The updated processing chip features include sudden sound stabilizer and Wind & Handling Stabilizer.
Browse through Grand View Research's Medical Devices Industry Research Reports.
• The global intrauterine devices market size was estimated at USD 6.25 billion in 2023 and is projected to grow at a CAGR of 3.66% from 2024 to 2030.
• The global dual chamber prefilled syringes market size was valued at USD 167.3 million in 2023 and is projected to grow at a CAGR of 5.8% from 2024 to 2030.
Key ENT Devices Company Insights
Some of the key market players include, Cochlear Ltd., Demant A/S, Stryker, and KARL STORZ.
Cochlear Ltd. (Cochlear) engages in developing and commercializing cochlear implants, bone conduction implants, & acoustic implants to treat hearing-impaired individuals. Cochlear Ltd. is a global company with major manufacturing facilities in Sweden and Australia.It has a global presence in more than 180 countries.
Demant A/S (Demant) is a global company that develops, manufactures, and commercializes hearing implants, traditional hearing instruments, personal communication devices, & diagnostic instruments. The group operates in over 30 countries and sells its products in over 130 countries.
Nemera., Nico Corporation, and Rion Co., Ltd. are the emerging market participants.
Nemera, founded in 2003, is a medical equipment manufacturer specializing in a diverse product portfolio, including Ear, Nose, Throat, Nasal spray pumps, drug delivery devices, ophthalmic, and others. In 2021, Nemera established an operational base in Brazil and expanded its product and service offerings throughout Latin America.
NICO Corporation is a medical technology company that specializes in developing minimally invasive surgical solutions, particularly in the fields of neurosurgery and otolaryngology (ear, nose, and throat or ENT)
Key ENT Devices Companies:
The following are the leading companies in the ENT devices market. These companies collectively hold the largest market share and dictate industry trends. Financials, strategy maps & products of these ENT devices companies are analyzed to map the supply network.
Ambu A/S
Cochlear Ltd.
Demant A/S
GN Store Nord A/S
Karl Storz SE & Co.
Olympus Corporation
Pentax of America, Inc.
Richard Wolf GmbH
Rion Co., Ltd.
Smith & Nephew plc
Sonova
Starkey Laboratories, Inc.
Stryker
Nico Corporation
Nemera
Recent Developments
In April 2023, Unitron, a brand of Sonova launched Vivante, a platform aimed to enhance listener’s experience through personalized hearing control. This platform offers improved sound performance and new designs to enhance the hearing experience, integrating experience innovations and the remote plus app to offer a customized hearing experience.
In February 2023, Cochlear Ltd. announced a partnership with Amazon.com, Inc. to expand audio streaming for hearing aids for people with Cochlear's hearing implants to provide comfortable entertainment.
In November 2022, Cochlear Ltd. announced the expansion of its manufacturing facility plant in Kuala Lumpur, Malaysia. The expansion involved an asset of more than USD 6.28 million (RM 30 million) to help the growing demand for acoustic and cochlear hearing implants
Order a free sample PDF of the ENT Devices Market Intelligence Study, published by Grand View Research.
0 notes
Text
AI Voice Cloning: Innovations and Implications for the Tech Industry
Artificial intelligence (AI) has advanced fast over the last decade, pushing the limits of what technology is capable of. One of the most intriguing advancements in this field is AI voice cloning. This technology enables the creation of very realistic and customizable synthetic voices, revolutionizing industries ranging from entertainment to customer service. In this blog article, we'll look at the advances driving AI voice cloning, the potential ramifications for the IT industry, and the growing trend of free AI voice cloning tools. Understanding AI Voice Cloning AI voice cloning uses deep learning algorithms to analyze and reproduce a person's voice. By processing large datasets of recorded speech, AI systems may develop synthetic voices that imitate the actual speaker's tone, pitch, and intonation with fantastic accuracy. This approach includes several critical technologies: Deep Neural Networks (DNNs): DNNs model the complexity of human speech, allowing AI to generate real-sounding voices. Natural Language Processing (NLP): NLP aids in comprehending and generating human language, enabling AI to produce coherent and contextually relevant speech. Generative Adversarial Networks (GANs): GANs enhance synthetic voices, increasing authenticity and minimizing artificial undertones. Innovations in AI Voice Cloning Improved Realism and Accuracy The increased realism and accuracy of synthetic voices is one of the most significant advances in AI voice cloning. Early attempts at voice synthesis frequently produced monotone, artificial speech. However, with improvements in machine learning, today's AI-generated sounds are nearly identical to human voices. Google, Amazon, and Microsoft have created voice cloning technology that can duplicate minor differences in speech, such as emotional tones and accents. Customisation and Personalisation AI voice cloning offers an excellent level of customization. Users can customize synthetic voices to reflect unique features, making them more personalized and engaging. This is especially beneficial in applications like virtual assistants, where a personalized voice may significantly improve the user experience. Businesses can also build brand-specific voices that correspond with their identity, guaranteeing consistency across all customer interactions. Real-time Voice Cloning Another ground-breaking breakthrough is real-time voice copying. This technique allows for real-time speech generation by creating synthetic voices on the fly. Real-time voice cloning has essential implications for live broadcasts, video games, and interactive applications, where immediate speech synthesis can improve the immersion experience. Free AI Voice Cloning Tools The democratization of AI technology has resulted in the creation of free AI voice cloning tools. These solutions give individuals and small organizations access to advanced voice copying capabilities without requiring a significant financial investment. Open-source programs and platforms such as Resemble AI, Descript, and iSpeech provide free or freemium approaches, allowing users to experiment with and integrate voice cloning into their projects. Applications of AI Voice Cloning in the Tech Industry Entertainment and Media AI voice cloning is revolutionizing the entertainment industry by allowing the generation of synthetic voices for animated characters, dubbing, and voiceovers. This technology enables the seamless integration of voices from multiple languages and places, making material more accessible worldwide. Furthermore, voice cloning can resurrect the voices of deceased actors, ensuring continuity in long-running series or posthumously released works. Customer Service In customer service, AI voice cloning can improve the capabilities of virtual assistants and chatbots. These AI-powered systems can provide better customer experiences by using a more human-like voice and responding to requests with greater empathy and efficiency. Personalized voices can also strengthen consumer relationships, increasing satisfaction and loyalty. Healthcare AI voice cloning has potential applications in healthcare, especially for individuals with speech disability. Patients can regain functional communication skills by developing synthetic voices that resemble their native voices. Additionally, telemedicine can use voice cloning to add a personal touch to remote consultations. Education AI voice cloning can help educators develop interactive and engaging learning experiences. Synthetic voices can narrate educational information, provide feedback, and aid in language learning by producing consistent and precise pronunciations. This technology can also create personalized learning aids tailored to each student's needs. Implications of AI Voice Cloning Ethical Consideration The rise of AI voice cloning raises numerous ethical concerns. One of the main issues is the possibility of abuse, such as making deepfake audio clips that can be used to deceive or manipulate. Robust legislative frameworks and explicit permission and data privacy norms must be in place to ensure this technology is used ethically. Intellectual Property AI voice cloning creates issues about intellectual property rights. Who owns the rights to a synthetic voice, especially if it sounds like a natural voice? Establishing legal protections and procedures will be critical in dealing with these challenges and avoiding the unauthorized use of cloned voices. Impact on Employment The broad deployment of AI voice cloning could impact voice acting and customer service jobs. While AI can complement human capabilities, job displacement is risky. Examining strategies for reskilling and upskilling employees to adapt to the changing landscape is critical. Future of AI Voice Cloning As AI voice cloning technology advances, we should expect more improvements in realism, customization, and accessibility. More sophisticated algorithms and processing power will enable progressively more convincing synthetic voices to be fabricated. Furthermore, the trend towards open AI voice cloning tools will increase access, allowing for more experimentation and innovation. The future of AI voice cloning holds enormous promise for improving human-computer interactions and generating more immersive and personalized experiences. We may use this technology to create beneficial change in various industries by addressing ethical and legal issues. Conclusion AI voice cloning is a revolutionary discovery that is altering the IT sector. This technology has numerous applications, from improving customer service to revolutionizing entertainment and media. With the introduction of free AI voice cloning tools, more people and organizations may investigate and benefit from this technology. However, careful consideration of the ethical and legal ramifications is required to ensure responsible and equitable use. As we look to the future, AI voice cloning promises to open up new possibilities and change how we engage with technology. Read the full article
0 notes
Link
Honey Trap Exposed: Discover the latest developments in the naval espionage case where Pakistan's ISI allegedly honey-trapped Indian
0 notes