#cognitive process automation tools for business
Explore tagged Tumblr posts
Text
Unlock the Power of MDM with AIOps
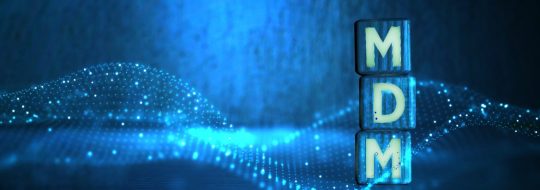
Master Data Management (MDM) is a process used by telecom companies to ensure the accuracy, consistency and completeness of data across all business channels. It enables telecom companies to make informed decisions about their products and services, through digital transformation services and solutions as well as provide better customer experience.
MDM in telecom industry involves collecting, organizing, managing and maintaining all master data related to customers, products, services and other entities. This data is then used to create reports which help telecom companies understand their current market position and make informed decisions about their future strategies. Furthermore, MDM ensures that the data is up-to-date so that it can be used for analytics purposes.
Role of AIOps in Building and Improving MDM Systems
AIOps is becoming increasingly important in the modern world of data management. It helps organizations to build and improve their master data management systems by automating processes, analysing large amounts of data, and providing insights into the performance of their systems.
AIOps can be used to automate and streamline the process of collecting, cleaning, and organizing data from multiple sources. This can help organizations save time and resources by eliminating manual tasks that are often associated with master data management. Additionally, AIOps can analyse large amounts of data quickly and accurately to provide insights into system performance that would otherwise take a long time to gather manually.
In conclusion, AIOps is an invaluable tool for building and improving master data management systems. By automating processes, analysing large datasets quickly, and providing insights into system performance, it can help organizations save time and resources while also improving their overall efficiency.
AIOps is revolutionizing the way enterprises manage their master data. It allows organizations to implement IT automation with AI and Machine Learning (ML) algorithms, helping them to reduce costs, improve efficiency, and increase productivity.
AIOps leverages AI and ML techniques to analyze large amounts of data in real-time and identify patterns that can be used to automate repetitive tasks. This helps organizations build a more efficient Master Data Management system by automating mundane tasks such as data cleansing and validation.
Telecom companies can benefit from using the best AIOps platforms software. These solutions provide MDM facilities and help create systems and operations that will boost customer satisfaction. Through effective MDM and improvement of communications channels, telecom companies can increase their clientele and ensure better performance of applications.
Read more @ https://zif.ai/what-is-master-data-management-mdm-in-telecom-and-how-aiops-help-to-build-it/
#AIOps platform market#service availability#AIOps Platform#IT infrastructure#digital service desk#predictive analytics models#digitaltransformations#Digital Transformation#cognitive process automation tools for business#predictive analytics using ai applications#predictive analytics business forecasting#aiops solutions#AIOPS
0 notes
Text
Introduction to AI Platforms
AI Platforms are powerful tools that allow businesses to automate complex tasks, provide real-time insights, and improve customer experiences. With their ability to process massive amounts of data, AI platforms can help organizations make more informed decisions, enhance productivity, and reduce costs.
These platforms incorporate advanced algorithms such as machine learning, natural language processing (NLP), and computer vision to analyze data through neural networks and predictive models. They offer a broad range of capabilities such as chatbots, image recognition, sentiment analysis, and recommendation engines.
Choosing the right AI platform is imperative for businesses that wish to stay ahead of the competition. Each platform has its strengths and weaknesses which must be assessed when deciding on a vendor. Moreover, an AI platform’s ability to integrate with existing systems is critical in effectively streamlining operations.
The history of AI platforms dates back to the 1950s, with the development of early artificial intelligence research. However, over time these technologies have evolved considerably – thanks to advancements in computing power and big data analytics. While still in their infancy stages just a few years ago – today’s AI platforms have matured into complex and feature-rich solutions designed specifically for business use cases.
Ready to have your mind blown and your workload lightened? Check out the best AI platforms for businesses and say goodbye to manual tasks:
Popular Commercial AI Platforms
To explore popular the top AI platforms and make informed decisions, you need to know the benefits each platform offers. With IBM Watson, Google Cloud AI Platform, Microsoft Azure AI Platform, and Amazon SageMaker in focus, this section shows the unique advantages each platform provides for various industries and cognitive services.
IBM Watson
The Innovative AI Platform by IBM:
Transform your business with the dynamic cognitive computing technology of IBM Watson. Enhance decision-making, automate operations, and accelerate the growth of your organization with this powerful tool.
Additional unique details about the platform:
IBM Watson’s Artificial intelligence streamlines workflows and personalizes experiences while enhancing predictive capabilities. The open-source ecosystem allows developers and businesses alike to integrate their innovative applications seamlessly.
Suggested implementation strategies:
1) Leverage Watson’s data visualization tools to clearly understand complex data sets and analyze them. 2) Utilize Watson’s Natural Language processing capabilities for sentiment analysis, identifying keywords, or contextual understanding.
By incorporating IBM Watson’s versatile machine learning functions into your operations, you can gain valuable insights into customer behavior patterns, track industry trends, improve decision-making abilities, and eventually boost revenue. Google’s AI platform is so powerful, it knows what you’re searching for before you do.
Google Cloud AI Platform
The AI platform provided by Google Cloud is an exceptional tool for businesses that major in delivering machine learning services. It provides a broad array of functionalities tailored to meet the diverse demands of clients all over the world.
The following table summarizes the features and capabilities offered by the Google Cloud AI Platform:FeaturesCapabilitiesData Management & Pre-processing
– Large-scale data processing
– Data Integration and Analysis tools
– Deep Learning Frameworks
– Data versioning tools
Model Training
– Scalable training
– AutoML tools
– Advanced tuning configurations
– Distributed Training on CPU/GPU/TPU
Prediction
– High-performance responses within seconds
– Accurate predictions resulting from models trained using large-scale datasets.
Monitoring
– Real-time model supervision and adjustment
– Comprehensive monitoring, management, and optimization of models across various stages including deployment.
One unique aspect of the Google Cloud AI platform is its prominent role in enabling any developer, regardless of their prior experience with machine learning, to build sophisticated models. This ease of use accelerates experimentation and fosters innovation.
Finally, it is worth noting that according to a study conducted by International Business Machines Corporation (IBM), brands that adopted AI for customer support purposes experienced 40% cost savings while improving customer satisfaction rates by 90%.
Continue Reading
2 notes
·
View notes
Text
RPA vs. AI: Choosing the Right Intelligent Automation Solution
Businesses today are under constant pressure to operate faster, more efficiently, and at a lower cost. Automation has become the cornerstone of this transformation, helping companies eliminate manual processes, reduce errors, and scale their operations. Among the many automation tools available, Robotic Process Automation (RPA) and Artificial Intelligence (AI) stand out as two of the most popular solutions.
Though both technologies fall under the umbrella of intelligent automation, they are fundamentally different in their capabilities, applications, and benefits. For organizations considering automation, understanding the nuances of RPA and AI is critical to making the right investment.
This blog takes a deep dive into RPA, AI, and their use cases, while also guiding you on how to choose the best solution for your business needs.
What is Robotic Process Automation (RPA)?
RPAÂ refers to software technology that mimics human actions to perform routine, repetitive, and rule-based tasks. These software bots are programmed to interact with existing applications, such as CRM systems, ERP software, or email platforms, much like a human worker would.
Unlike traditional automation tools that require deep system integrations, RPA is lightweight and non-invasive, meaning it works with your current systems without major changes.
Key Features of RPA
Rule-Based Automation: RPA excels in scenarios where processes follow strict, defined rules, such as data entry or invoice processing.
User-Friendly Design: Many RPA platforms offer low-code or no-code interfaces, allowing business users to design automation workflows with minimal technical expertise.
System Independence: Bots can work across various software platforms without requiring API-based integrations.
Advantages of RPA
Ease of Implementation One of the biggest advantages of RPA is its simplicity. Businesses can deploy RPA bots quickly without needing to overhaul their IT systems. This makes it a low-risk option for companies new to automation.
Cost Savings By automating mundane tasks, companies can reduce the need for manual intervention, cutting down labor costs and reallocating resources to higher-value tasks.
Accuracy and Reliability Unlike humans, RPA bots don’t get tired or make mistakes when performing repetitive tasks. This ensures high accuracy, which is particularly valuable in data-sensitive industries like finance and healthcare.
Scalability RPA is highly scalable, enabling businesses to deploy more bots as the workload increases. This is particularly useful during seasonal peaks or in industries with fluctuating demands.
Use Cases of RPA
RPA is highly effective in automating tasks that involve repetitive, structured workflows. Common examples include:
Accounts Payable: Automating invoice data entry, payment approvals, and reconciliations.
Human Resources: Streamlining tasks like employee onboarding, updating employee records, and payroll management.
IT Support: Automating ticket generation, password resets, and system monitoring.
Customer Support: Automating routine responses, such as order status updates or refund requests.
While RPA offers immense value, it is inherently limited to structured data and predefined rules. When processes require cognitive decision-making, RPA alone isn’t enough.
What is Artificial Intelligence (AI)?
Artificial Intelligence is a branch of computer science that focuses on building systems capable of performing tasks that would normally require human intelligence. This includes learning from data, understanding natural language, recognizing images, and making decisions based on patterns.
Unlike RPA, which follows rigid rules, AI can learn and adapt. This makes it a powerful tool for businesses looking to process unstructured data or gain actionable insights from large datasets.
Key Features of AI
Learning and Adaptation: AI systems use techniques like machine learning to improve their performance over time.
Versatility: AI handles complex tasks such as natural language processing (NLP), image recognition, and predictive analytics.
Unstructured Data Handling: Unlike RPA, AI can process unstructured data, such as emails, social media posts, or audio recordings.
Advantages of AI
Cognitive Capabilities AI enables systems to think, reason, and make decisions, opening the door to automating complex processes that require judgment or problem-solving.
Improvement Over Time AI algorithms learn from data and improve as more information becomes available. For example, a recommendation engine becomes more accurate the longer it’s in use.
Data-Driven Insights AI helps organizations analyze large datasets to uncover patterns, predict outcomes, and support decision-making. This is particularly valuable for industries like healthcare, retail, and finance.
Personalization By analyzing user behavior, AI can deliver personalized customer experiences, such as recommending products or tailoring marketing campaigns.
Use Cases of AI
AI is versatile and can be applied across a wide range of business scenarios, including:
Customer Support: AI-powered chatbots and virtual assistants can handle customer inquiries in real-time, reducing the workload on human agents.
Fraud Detection: AI algorithms analyze transaction patterns to identify suspicious activity in real-time.
Predictive Maintenance: In manufacturing, AI can predict equipment failures based on sensor data, reducing downtime.
Healthcare: AI-powered systems assist in diagnostics, drug discovery, and patient care personalization.
How RPA and AI Work Together
Although RPA and AI are distinct technologies, they complement each other beautifully when combined. Together, they create a comprehensive automation framework known as Hyperautomation.
Why Combine RPA and AI?
End-to-End Automation: RPA handles structured, rule-based processes, while AI deals with unstructured data and decision-making.
Enhanced Capabilities: AI adds cognitive intelligence to RPA, allowing bots to handle complex tasks like sentiment analysis or document interpretation.
Future-Proofing: Combining RPA and AI creates a scalable, future-ready automation ecosystem.
An Example of Combined Use Case
Invoice Processing: AI extracts key information from invoices (even with varying formats) using OCR and NLP. RPA then inputs this data into the accounting system and triggers payment workflows.
How to Choose the Right Automation Solution
Selecting between RPA, AI, or a hybrid approach depends on your business needs. Consider the following factors:
Choose RPA If:
Your processes are rule-based and repetitive.
You deal primarily with structured data.
You’re looking for a quick, cost-effective automation solution.
Choose AI If:
Your processes involve decision-making or unstructured data.
You need insights from data analysis or predictive analytics.
You aim to create adaptive, intelligent workflows.
Combine Both If:
You require automation across diverse processes involving both structured and unstructured data.
You want to future-proof your business with intelligent, scalable automation.
Conclusion
The choice between RPA and AI depends on the specific challenges and goals of your organization. While RPA is an excellent starting point for automating rule-based tasks, AI brings intelligence, adaptability, and innovation to your operations. For many businesses, a combination of RPA and AI delivers the best results, enabling end-to-end process automation.
If you’re ready to explore intelligent automation solutions, contact Charter Global to discuss how we can tailor an automation strategy to fit your unique needs.
Book a Consultation.
Or email us at [email protected] or call +1 770–326–9933.
0 notes
Text
The Future of Robotic Process Automation: Trends to Watch in 2025Â
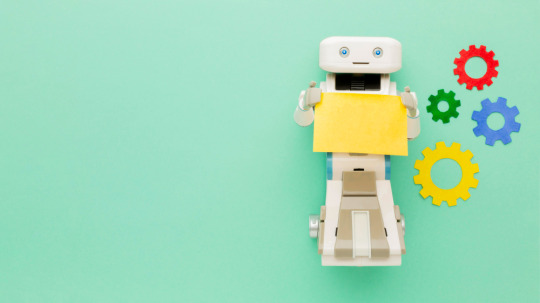
As we enter 2025, Robotic Process Automation (RPA) continues to evolve rapidly, with its transformative potential reaching new heights across industries. The integration of robotic automation tools is already reshaping businesses by streamlining operations, reducing costs, and improving efficiency. But what’s next? In the coming years, businesses can expect to see RPA take on more sophisticated roles, powered by advanced technologies like AI, machine learning, and natural language processing. Let’s explore the key trends that will define the future of Robotic Process Automation in 2025 and beyond.Â
1. AI-Powered Automation: The Next FrontierÂ
The most significant trend shaping the future of RPA is the integration of artificial intelligence (AI). While traditional RPA focused primarily on automating rule-based tasks, AI-powered RPA is designed to handle more complex, cognitive processes. This shift enables RPA systems to learn from data, adapt to new scenarios, and even make decisions autonomously. AI-enhanced bots will significantly expand the scope of tasks that can be automated, from customer service to data analysis, and help businesses tackle more intricate workflows that require human-like judgment.Â
For example, AI-powered RPA could be used to automate customer support, where bots are not only performing repetitive tasks but also understanding and responding to queries in natural language. As AI continues to evolve, businesses can expect even greater efficiency and intelligence in their RPA solutions.Â
2. Increased Adoption of Cloud-Based RPAÂ
Another trend to watch is the growing shift toward cloud-based RPA solutions. Cloud technologies offer several advantages over traditional on-premise systems, including scalability, flexibility, and ease of access. By migrating RPA deployments to the cloud, businesses can achieve faster implementation and avoid the high upfront costs associated with on-premise infrastructure.Â
Cloud-based RPA also supports hybrid environments, enabling organizations to leverage both on-premise and cloud systems. This makes it easier to integrate automation tools with other cloud applications, allowing businesses to gain a holistic view of their operations. Additionally, cloud-based RPA solutions provide seamless updates and improvements, ensuring that businesses stay on the cutting edge without worrying about maintaining complex infrastructure.Â
3. Hyperautomation: Going Beyond RPAÂ
Hyperautomation is an emerging trend that goes beyond simple RPA to incorporate a wide array of automation technologies, such as AI, machine learning, process mining, and analytics. The goal of hyperautomation is to automate end-to-end business processes, not just isolated tasks. With hyperautomation, organizations can create highly efficient, intelligent workflows that span multiple departments and functions.Â
In 2025, businesses will begin to implement hyperautomation at scale. For instance, an organization might combine RPA tools with AI to automate an entire customer journey, from initial contact to follow-up. This enables businesses to reduce manual intervention and improve customer satisfaction while driving overall process efficiency. The key to hyperautomation’s success will lie in integrating multiple automation technologies into cohesive workflows that drive maximum value.Â
4. Robotic Process Automation in the Cloud for Mobile AppsÂ
As mobile apps continue to grow in popularity, companies will increasingly turn to RPA to streamline their mobile app development and maintenance processes. For example, developers can use robotic process automation tools to handle repetitive tasks like testing and debugging, allowing them to focus on more complex aspects of app development. By automating these tedious processes, companies can significantly reduce the time it takes to bring a new app to market.Â
To further streamline mobile app development, businesses may adopt mobile app cost calculators to estimate the costs involved in building and maintaining mobile applications. By integrating robotic automation tools with these calculators, companies can automate the entire process of budgeting for mobile app projects, helping them plan more accurately and avoid costly overruns. This is an exciting intersection of RPA and mobile technology that will grow in importance as mobile apps become increasingly central to business strategies.Â
5. Enhanced Human-Robot CollaborationÂ
As automation becomes more pervasive, the role of human workers will evolve. Instead of replacing jobs, RPA will enhance human capabilities by automating mundane tasks, allowing employees to focus on more strategic and creative activities. In 2025, we can expect to see an increased emphasis on human-robot collaboration, where bots assist human workers in real-time, rather than operating in isolation.Â
This collaboration will be especially evident in sectors like healthcare, finance, and customer service. For instance, in healthcare, RPA can handle administrative tasks like scheduling and billing, allowing healthcare professionals to spend more time with patients. In finance, bots can automate data entry, freeing up financial analysts to focus on higher-value activities like forecasting and strategy.Â
If you're interested in exploring the benefits of custom mobile app development services for your business, we encourage you to book an appointment with our team of experts.Â
Book an AppointmentÂ
6. Data Security and GovernanceÂ
With the growing reliance on automation, data security and governance will be more important than ever. In 2025, businesses will need to ensure that their RPA systems are secure, compliant with regulations, and free from vulnerabilities. This is particularly critical as RPA expands into sensitive areas like healthcare, finance, and legal sectors, where the handling of personal and confidential information is regulated.Â
RPA providers are expected to focus more on building robust security features, including data encryption, access controls, and audit trails, to help businesses protect sensitive data. Additionally, organizations will need to implement governance frameworks that ensure RPA deployments comply with industry regulations and internal policies.Â
7. RPA as a ServiceÂ
The demand for RPA solutions will continue to grow, leading to the rise of RPA as a Service (RPAaaS). This model allows businesses to access RPA tools and services on a subscription basis, eliminating the need for expensive upfront investments in software and infrastructure. With RPAaaS, businesses of all sizes can easily scale their automation efforts without the complexities of managing the underlying technology.Â
As RPAaaS becomes more prevalent in 2025, businesses will have more flexibility to adopt automation at their own pace. Whether they need to automate a few processes or implement enterprise-wide automation, RPAaaS offers a cost-effective and scalable solution.Â
Conclusion: The Evolution of RPA SolutionsÂ
Looking ahead to 2025, the future of Robotic Process Automation is bright, with numerous advancements on the horizon. From AI-powered bots and cloud-based solutions to the rise of hyperautomation and human-robot collaboration, RPA is set to revolutionize how businesses operate. By integrating these cutting-edge technologies, organizations can optimize their processes, improve efficiency, and stay competitive in a rapidly evolving landscape. Now is the time to invest in RPA solutions to future-proof your business and drive lasting success.Â
0 notes
Text
Humanizing Artificial Intelligence: Bridging the Gap Between Machines and Humans
Outline:
1. Introduction
Definition of Humanizing Artificial Intelligence (AI)
Why humanizing AI matters in the modern world
Overview of the article's content and key takeaways
2. What Does It Mean to Humanize AI? humanize ai
Defining “Humanized AI” and its key principles
How humanized AI differs from traditional AI systems
Key features: Empathy, emotional intelligence, and social interaction
3. The Science Behind Humanizing AI
Cognitive computing: Making AI systems think more like humans
Natural Language Processing (NLP): How AI understands human speech
Emotion recognition and emotional AI: Reading facial expressions, tone, and sentiment
Machine learning: Improving human-like decision-making
4. Applications of Humanized AI
Healthcare: AI companions for elderly care and mental health
Customer Service: AI chatbots with empathy and context-aware conversations
Education: Personalized AI tutors and learning assistants
Social Companionship: Virtual friends and social robots for reducing loneliness
5. Ethical Considerations in Humanizing AI
Privacy concerns: Data collection and emotional vulnerability
Bias in AI: How human biases can be encoded in AI systems
Emotional dependency: Risks of over-reliance on AI for companionship and mental health
Regulation and transparency: Ensuring fairness and accountability in humanized AI systems
6. Challenges in Humanizing AI
Emulating authentic emotions vs. mimicking behaviors
Technological limitations in emotion recognition and contextual understanding
Public perception: Humanizing AI without crossing ethical boundaries
Overcoming resistance to the rise of emotionally intelligent machines
7. The Future of Humanized AI
AI-Human Collaboration: Blending AI with human creativity and decision-making
Improved Emotional Intelligence: Future advancements in AI’s ability to understand and respond to emotions
Human-AI Hybrids: Exploring brain-computer interfaces and more integrated systems
The role of AI in enhancing human potential rather than replacing it
8. Tutorials and Strategies for Engaging with Humanized AI
Using AI in Healthcare: How to interact with AI-powered healthcare companions
Developing Your Own Humanized AI Chatbot: A step-by-step guide for beginners
Integrating AI in Customer Service: How businesses can use humanized AI for better customer engagement
9. Conclusion
Summarizing the importance of humanizing AI
Final thoughts on the challenges and ethical considerations
The long-term outlook: A future where AI and humans work together harmoniously
Topic Strategy:
Clear and Concise Explanation: The article will break down the complex subject of humanized AI into understandable chunks, targeting both general readers and those with some technical background.
Balance Between Technical and Practical: While the article will cover the underlying science and technology behind humanized AI, it will also focus on how it applies to real-world scenarios like healthcare, customer service, and companionship.
Ethical and Social Awareness: Ethical concerns about humanizing AI will be addressed in a nuanced way to highlight the balance between innovation and caution.
Actionable Insights: The inclusion of tutorials and strategies offers readers a practical understanding of how they can engage with or create their own humanized AI systems.
Draft Article (Between 1,500 and 2,100 Words):
Introduction
Artificial Intelligence (AI) has evolved from simple automation systems to powerful tools capable of transforming industries and everyday life. As AI continues to grow, the concept of humanizing artificial intelligence—making AI systems capable of understanding, responding to, and interacting with humans on a deeper emotional and social level—has become a significant focus. Humanized AI involves imbuing machines with traits such as empathy, emotional intelligence, and the ability to understand context, enabling them to interact with humans in more natural and meaningful ways.
This article explores the process of humanizing AI, examining how AI can replicate or even augment human traits, its real-world applications, and the challenges it faces. We will also look at the ethical considerations involved and provide practical tutorials for engaging with this next frontier in artificial intelligence.
What Does It Mean to Humanize AI?
At its core, humanizing AI means designing machines that not only perform tasks but can also engage in meaningful, human-like interactions. Traditional AI systems are good at executing specific functions—like processing data or automating repetitive tasks—but they lack the ability to engage with humans in emotionally intelligent ways.
Humanized AI goes beyond task automation by incorporating the following features:
Empathy: The ability to understand and respond to human emotions.
Social Awareness: Recognizing context and adjusting responses to fit the situation.
Natural Interaction: Mimicking human-like behaviors such as conversation, facial expressions, and body language.
Unlike traditional AI that focuses on efficiency, humanized AI aims to create machines that understand and respond in a more human-like manner, making interactions more intuitive and personalized.
The Science Behind Humanizing AI
Several technologies and methodologies are central to making AI systems human-like:
Cognitive Computing: This involves developing AI systems that simulate human thought processes, allowing machines to understand, learn, and respond in ways that mirror human reasoning and decision-making.
Natural Language Processing (NLP): NLP enables AI to understand human language, including nuances like tone, slang, and sentiment. This allows for smoother, more intuitive communication between machines and humans.
Emotion Recognition: Through facial recognition, voice analysis, and behavioral cues, AI can detect emotions and respond accordingly. This is key to building empathy into AI systems.
Machine Learning: AI systems learn from vast amounts of data to make predictions, adapt to new situations, and improve over time—much like human learning.
By combining these technologies, AI systems can move beyond mere functionality to engage with humans on a deeper, emotional level.
Applications of Humanized AI
Humanized AI has a wide range of applications, from healthcare to customer service and social companionship. Some key areas include:
Healthcare: AI companions are being used to assist the elderly and those with disabilities, providing emotional support, reminders, and even interactive therapy. Virtual therapists are also emerging, offering mental health assistance through empathetic conversations.
Customer Service: AI-powered chatbots and virtual assistants are becoming more empathetic and context-aware. Unlike traditional bots that respond with basic, pre-programmed answers, humanized AI can engage in dynamic, nuanced conversations that mimic real human interactions.
Education: AI-powered tutoring systems are becoming increasingly personalized, adapting to the learning pace and style of individual students. Humanized AI can also simulate real-world scenarios for training, enhancing learning experiences.
Social Companionship: AI-driven virtual companions are helping to reduce feelings of loneliness, especially among elderly individuals or those living in isolation. These AI systems can engage in conversations, provide entertainment, and offer emotional support.
Ethical Considerations in Humanizing AI
As AI becomes more human-like, ethical concerns inevitably arise. Some key considerations include:
Privacy: Humanized AI systems often need access to personal data, raising concerns about how this information is collected, used, and protected.
Bias: AI systems may inherit the biases of their creators or the data they are trained on, leading to unfair or discriminatory outcomes.
Emotional Dependency: Over-reliance on AI for companionship or mental health support could create emotional dependency, potentially replacing human interaction with machines.
Regulation: As humanized AI becomes more prevalent, frameworks must be developed to ensure these systems are transparent, accountable, and used responsibly.
Challenges in Humanizing AI
While the potential for humanized AI is immense, there are still significant challenges to overcome:
Authenticity vs. Simulation: Can AI truly understand emotions, or is it just mimicking human behavior? The question of whether AI can develop true emotional intelligence remains a contentious issue.
Technological Limitations: Current AI systems still struggle with understanding the full depth of human emotions, tone, and context. Advancements in emotion recognition and NLP are still ongoing.
Public Perception: Many people remain skeptical about the idea of machines with human-like qualities. The balance between innovation and societal acceptance is delicate, and too much "humanization" might raise ethical concerns.
The Future of Humanized AI
The future of humanized AI looks promising. As technology improves, we can expect:
AI-Human Collaboration: Humanized AI will increasingly work alongside humans, augmenting human capabilities in fields like healthcare, education, and entertainment.
Better Emotional Intelligence: AI will continue to evolve, becoming more adept at reading and responding to human emotions in real-time.
Human-AI Hybrids: Brain-computer interfaces may allow humans and AI to work together more closely, blending human cognition with machine intelligence.
Tutorials and Strategies for Engaging with Humanized AI
Using AI in Healthcare: If you are using an AI-powered healthcare companion, engage with it as you would with a human assistant—ask questions, provide feedback, and monitor its emotional
0 notes
Text
Humanizing Artificial Intelligence: Bridging the Gap Between Machines and Humans
Outline:
1. Introduction
Definition of Humanizing Artificial Intelligence (AI)
Why humanizing AI matters in the modern world
Overview of the article's content and key takeaways
2. What Does It Mean to Humanize AI? humanize ai
Defining “Humanized AI” and its key principles
How humanized AI differs from traditional AI systems
Key features: Empathy, emotional intelligence, and social interaction
3. The Science Behind Humanizing AI

Cognitive computing: Making AI systems think more like humans
Natural Language Processing (NLP): How AI understands human speech
Emotion recognition and emotional AI: Reading facial expressions, tone, and sentiment
Machine learning: Improving human-like decision-making
4. Applications of Humanized AI
Healthcare: AI companions for elderly care and mental health
Customer Service: AI chatbots with empathy and context-aware conversations
Education: Personalized AI tutors and learning assistants
Social Companionship: Virtual friends and social robots for reducing loneliness
5. Ethical Considerations in Humanizing AI
Privacy concerns: Data collection and emotional vulnerability
Bias in AI: How human biases can be encoded in AI systems
Emotional dependency: Risks of over-reliance on AI for companionship and mental health
Regulation and transparency: Ensuring fairness and accountability in humanized AI systems
6. Challenges in Humanizing AI
Emulating authentic emotions vs. mimicking behaviors
Technological limitations in emotion recognition and contextual understanding
Public perception: Humanizing AI without crossing ethical boundaries
Overcoming resistance to the rise of emotionally intelligent machines
7. The Future of Humanized AI
AI-Human Collaboration: Blending AI with human creativity and decision-making
Improved Emotional Intelligence: Future advancements in AI’s ability to understand and respond to emotions
Human-AI Hybrids: Exploring brain-computer interfaces and more integrated systems
The role of AI in enhancing human potential rather than replacing it
8. Tutorials and Strategies for Engaging with Humanized AI
Using AI in Healthcare: How to interact with AI-powered healthcare companions
Developing Your Own Humanized AI Chatbot: A step-by-step guide for beginners
Integrating AI in Customer Service: How businesses can use humanized AI for better customer engagement
9. Conclusion
Summarizing the importance of humanizing AI
Final thoughts on the challenges and ethical considerations
The long-term outlook: A future where AI and humans work together harmoniously
Topic Strategy:
Clear and Concise Explanation: The article will break down the complex subject of humanized AI into understandable chunks, targeting both general readers and those with some technical background.
Balance Between Technical and Practical: While the article will cover the underlying science and technology behind humanized AI, it will also focus on how it applies to real-world scenarios like healthcare, customer service, and companionship.
Ethical and Social Awareness: Ethical concerns about humanizing AI will be addressed in a nuanced way to highlight the balance between innovation and caution.
Actionable Insights: The inclusion of tutorials and strategies offers readers a practical understanding of how they can engage with or create their own humanized AI systems.
Draft Article (Between 1,500 and 2,100 Words):
Introduction
Artificial Intelligence (AI) has evolved from simple automation systems to powerful tools capable of transforming industries and everyday life. As AI continues to grow, the concept of humanizing artificial intelligence—making AI systems capable of understanding, responding to, and interacting with humans on a deeper emotional and social level—has become a significant focus. Humanized AI involves imbuing machines with traits such as empathy, emotional intelligence, and the ability to understand context, enabling them to interact with humans in more natural and meaningful ways.
This article explores the process of humanizing AI, examining how AI can replicate or even augment human traits, its real-world applications, and the challenges it faces. We will also look at the ethical considerations involved and provide practical tutorials for engaging with this next frontier in artificial intelligence.
What Does It Mean to Humanize AI?
At its core, humanizing AI means designing machines that not only perform tasks but can also engage in meaningful, human-like interactions. Traditional AI systems are good at executing specific functions—like processing data or automating repetitive tasks—but they lack the ability to engage with humans in emotionally intelligent ways.
Humanized AI goes beyond task automation by incorporating the following features:
Empathy: The ability to understand and respond to human emotions.
Social Awareness: Recognizing context and adjusting responses to fit the situation.
Natural Interaction: Mimicking human-like behaviors such as conversation, facial expressions, and body language.
Unlike traditional AI that focuses on efficiency, humanized AI aims to create machines that understand and respond in a more human-like manner, making interactions more intuitive and personalized.
The Science Behind Humanizing AI
Several technologies and methodologies are central to making AI systems human-like:
Cognitive Computing: This involves developing AI systems that simulate human thought processes, allowing machines to understand, learn, and respond in ways that mirror human reasoning and decision-making.
Natural Language Processing (NLP): NLP enables AI to understand human language, including nuances like tone, slang, and sentiment. This allows for smoother, more intuitive communication between machines and humans.
Emotion Recognition: Through facial recognition, voice analysis, and behavioral cues, AI can detect emotions and respond accordingly. This is key to building empathy into AI systems.
Machine Learning: AI systems learn from vast amounts of data to make predictions, adapt to new situations, and improve over time—much like human learning.
By combining these technologies, AI systems can move beyond mere functionality to engage with humans on a deeper, emotional level.
Applications of Humanized AI
Humanized AI has a wide range of applications, from healthcare to customer service and social companionship. Some key areas include:
Healthcare: AI companions are being used to assist the elderly and those with disabilities, providing emotional support, reminders, and even interactive therapy. Virtual therapists are also emerging, offering mental health assistance through empathetic conversations.
Customer Service: AI-powered chatbots and virtual assistants are becoming more empathetic and context-aware. Unlike traditional bots that respond with basic, pre-programmed answers, humanized AI can engage in dynamic, nuanced conversations that mimic real human interactions.
Education: AI-powered tutoring systems are becoming increasingly personalized, adapting to the learning pace and style of individual students. Humanized AI can also simulate real-world scenarios for training, enhancing learning experiences.
Social Companionship: AI-driven virtual companions are helping to reduce feelings of loneliness, especially among elderly individuals or those living in isolation. These AI systems can engage in conversations, provide entertainment, and offer emotional support.
Ethical Considerations in Humanizing AI
As AI becomes more human-like, ethical concerns inevitably arise. Some key considerations include:
Privacy: Humanized AI systems often need access to personal data, raising concerns about how this information is collected, used, and protected.
Bias: AI systems may inherit the biases of their creators or the data they are trained on, leading to unfair or discriminatory outcomes.
Emotional Dependency: Over-reliance on AI for companionship or mental health support could create emotional dependency, potentially replacing human interaction with machines.
Regulation: As humanized AI becomes more prevalent, frameworks must be developed to ensure these systems are transparent, accountable, and used responsibly.
Challenges in Humanizing AI
While the potential for humanized AI is immense, there are still significant challenges to overcome:
Authenticity vs. Simulation: Can AI truly understand emotions, or is it just mimicking human behavior? The question of whether AI can develop true emotional intelligence remains a contentious issue.
Technological Limitations: Current AI systems still struggle with understanding the full depth of human emotions, tone, and context. Advancements in emotion recognition and NLP are still ongoing.
Public Perception: Many people remain skeptical about the idea of machines with human-like qualities. The balance between innovation and societal acceptance is delicate, and too much "humanization" might raise ethical concerns.
The Future of Humanized AI
The future of humanized AI looks promising. As technology improves, we can expect:
AI-Human Collaboration: Humanized AI will increasingly work alongside humans, augmenting human capabilities in fields like healthcare, education, and entertainment.
Better Emotional Intelligence: AI will continue to evolve, becoming more adept at reading and responding to human emotions in real-time.
Human-AI Hybrids: Brain-computer interfaces may allow humans and AI to work together more closely, blending human cognition with machine intelligence.
Tutorials and Strategies for Engaging with Humanized AI
Using AI in Healthcare: If you are using an AI-powered healthcare companion, engage with it as you would with a human assistant—ask questions, provide feedback, and monitor its emotional
0 notes
Text
Revolutionizing Technology: The Impact of Artificial Intelligence Software Development

Artificial Intelligence Software Development reshapes industries. IdeyaLabs leads this revolution. We explore how AI redefines technology.
Discovering the Power of AI
AI transforms routine tasks. It mimics human cognition. Automated processes elevate efficiency. IdeyaLabs applies AI to drive innovation. Tasks become streamlined. Accuracy enhances decision-making.
Understanding AI Software Development
AI Software Development encompasses creating intelligent applications. Machine learning and deep learning algorithms play critical roles. These algorithms analyze data patterns. IdeyaLabs crafts superior AI solutions.
Key Elements in AI Software Development
Several components constitute AI Software Development:
Algorithms: Basic building blocks. They process data efficiently.
Data: Fuel for AI systems. Quality data powers accurate results.
Computing Power: Enhanced hardware accelerates operations.
Human Expertise: Professionals guide AI development.
IdeyaLabs excels in all these areas.
Benefits of Implementing AI Solutions
AI solutions offer numerous advantages:
Efficiency: Accelerates task completion.
Accuracy: Reduces errors in processes.
Scalability: Grows with the business needs.
Cost-Effectiveness: Saves resources in the long run.
Businesses leverage these benefits with IdeyaLabs' expertise.
Exploring the Role of Machine Learning
Machine Learning stands as a subset of AI. It allows systems to learn from data. Predictions improve over time. IdeyaLabs integrates machine learning into applications. Insightful data analysis becomes possible.
Deep Learning: Taking AI to the Next Level
Deep Learning, a branch of machine learning, uses neural networks. These networks simulate human brain processes. They handle complex tasks like image recognition. IdeyaLabs harnesses deep learning for robust solutions.
Natural Language Processing: Bridging Human-Computer Interaction
NLP enables machines to understand human language. Text and speech recognition improve interaction. IdeyaLabs enhances user experiences with NLP technologies.
Computer Vision: Enabling Machines to See
Computer Vision allows machines to interpret visual input. It analyzes images and videos. IdeyaLabs develops applications that utilize computer vision. Industries such as healthcare benefit immensely.
AI in Business Applications
AI transforms business processes. Customer service becomes proactive with chatbots. Data analysis gains depth. IdeyaLabs integrates AI into business solutions. Operations enhance and competitiveness improves.
Security Enhanced by AI
AI strengthens cybersecurity. It detects threats in real-time. IdeyaLabs offers AI-driven security solutions. Businesses protect sensitive information effectively.
Healthcare Revolutionized by AI
AI improves patient diagnosis. Predictive analytics aid in treatment planning. IdeyaLabs pioneers healthcare solutions. Efficiency and accuracy in diagnostics see improvement.
AI in Financial Services
Financial services harness AI for fraud detection. Automated trading becomes possible. IdeyaLabs develops AI solutions for financial institutions. Risks diminish and returns optimize.
Retail Industry Benefits from AI
AI personalizes shopping experiences. Inventory management becomes efficient. IdeyaLabs enhances retail solutions with AI implementations.
AI in Manufacturing
AI predicts machinery maintenance needs. Production processes optimize. IdeyaLabs shapes the manufacturing sector with AI. Productivity and uptime see significant improvement.
Education Transformed by AI
AI customizes learning experiences. It identifies student needs efficiently. IdeyaLabs develops AI tools for educational institutions. Learning becomes more effective.
AI-Powered Marketing Strategies
Marketing gains precision with AI. Consumer behavior analysis refines campaigns. IdeyaLabs creates AI-driven marketing solutions. Conversion rates improve.
Challenges in AI Software Development
AI Software Development faces obstacles:
Data Privacy: Ensuring ethical use of data.
Bias in Algorithms: Maintaining fairness and accuracy.
Integration: Seamlessly incorporating AI into existing systems.
IdeyaLabs addresses these challenges effectively.
Future Prospects of AI
The future holds immense potential. AI continues to evolve. IdeyaLabs stays ahead with cutting-edge solutions. The technology landscape witnesses constant innovation.
IdeyaLabs: Your Partner in AI Development
IdeyaLabs' expertise ensures superior AI solutions. Our team excels in delivering customized applications. Businesses achieve growth and efficiency with our support. Embrace the future of technology with IdeyaLabs.
Conclusion
Artificial Intelligence Software Development stands as a key driver of innovation. It enhances various sectors. IdeyaLabs leads this technological race with superior solutions. Explore the future of AI with us.
0 notes
Text
How Sarath Maddineni is Redefining Artificial Intelligence and Machine Learning

Artificial Intelligence (AI) and Machine Learning (ML) have emerged as transformative technologies reshaping industries worldwide. Among the visionaries leading this revolution is Sarath Maddineni, a tech pioneer whose contributions are redefining the way AI and ML are understood and implemented. His work has not only pushed the boundaries of innovation but also paved the way for ethical, efficient, and scalable AI solutions across diverse industries.
A Visionary Approach to Artificial Intelligence
Sarath Maddineni’s approach to AI transcends conventional methodologies. He emphasizes creating systems that not only learn from data but also adapt intelligently to dynamic environments. By combining deep learning algorithms with advanced neural network architectures, Maddineni has developed solutions that mirror human cognition in decision-making, problem-solving, and pattern recognition.
A notable area of Maddineni’s expertise lies in natural language processing (NLP), where his innovations have led to smarter, context-aware systems capable of understanding and responding to human language with near-human accuracy. These advancements have been pivotal in enhancing virtual assistants, chatbots, and real-time translation tools.
Revolutionizing Machine Learning with Innovation
Machine Learning thrives on data, and Maddineni has been instrumental in creating models that are not only data-efficient but also interpretable and transparent. He advocates for explainable AI (XAI), ensuring that ML models offer insights into their decision-making processes, making them trustworthy and reliable for critical applications such as healthcare, finance, and autonomous vehicles.
One of Maddineni’s hallmark contributions is the development of transfer learning techniques. These techniques allow ML models to leverage pre-trained knowledge, significantly reducing training time and computational costs. This breakthrough has democratized access to machine learning, enabling smaller businesses and organizations to adopt ML technologies without extensive resources.
Transforming Industries Through AI and ML
Sarath Maddineni’s innovative work has had a profound impact on various industries. His AI-driven solutions are enabling businesses to optimize operations, improve customer experiences, and achieve unprecedented levels of efficiency.
Healthcare: Maddineni has been a driving force in applying AI to medical diagnostics and treatment planning. By integrating computer vision algorithms with medical imaging, his solutions can detect diseases such as cancer and diabetic retinopathy at early stages, potentially saving countless lives.
Finance: In the financial sector, his predictive analytics models are helping institutions identify fraud, assess risks, and automate decision-making. Maddineni’s emphasis on bias-free AI ensures ethical and fair practices, especially in areas like loan approvals and credit scoring.
Retail: Maddineni’s contributions to AI-powered personalization are revolutionizing the retail industry. Through advanced recommendation engines, retailers can offer tailored shopping experiences, increasing customer satisfaction and loyalty.
Autonomous Systems: His work in autonomous vehicles and robotics has advanced the development of self-driving cars, drones, and industrial robots, making these technologies safer, more reliable, and adaptable to complex environments.
Ethical AI: A Core Principle
In a rapidly evolving AI landscape, ethical considerations are paramount. Sarath Maddineni is a staunch advocate for responsible AI development, ensuring that technology is used for the betterment of humanity. His frameworks for fairness, accountability, and transparency are setting new standards for the industry.
By addressing issues such as data privacy, algorithmic bias, and the environmental impact of AI systems, Maddineni ensures that his solutions align with societal values and global sustainability goals. His work highlights the importance of balancing innovation with responsibility, ensuring that AI serves as a force for good.
The Road Ahead
As technology continues to evolve, Sarath Maddineni remains at the forefront of AI and ML innovation. His focus on next-generation technologies, such as quantum computing and edge AI, promises to unlock new possibilities for real-time analytics, decentralized systems, and faster computation.
Maddineni’s dedication to bridging the gap between theoretical research and practical applications positions him as a trailblazer in the tech world. By fostering collaboration across academia, industry, and government, he is driving the adoption of AI and ML on a global scale.
Sarath Maddineni’s groundbreaking contributions to AI and ML are not just reshaping industries but also redefining the possibilities of technology. From advancing NLP and transfer learning to championing ethical AI practices, his work serves as a blueprint for the future of artificial intelligence. As we move forward into an AI-driven era, Maddineni’s innovations stand as a testament to the transformative power of technology when guided by vision, integrity, and a commitment to societal progress.
0 notes
Text
Cognition AI: The New Frontier in Human-Like Intelligence
In the era of technological discoveries, Cognition AI, as a novel area of research, is changing what is possible with artificial intelligence today. It combines cognitive science with very advanced AI techniques to make machines imitate human-like reasoning and learning and decision-making abilities.

What is Cognition AI?
Cognition AI refers to systems of artificial intelligence designed to mimic human cognitive processes like thinking, reasoning, problem-solving, and understanding. Unlike traditional AI, which is based on pre-defined rules and data-driven patterns, Cognition AI is concerned with learning and adapting in real-time, just like human process information, draw inferences, and make decisions.
The approach of AI draws from cognition science, neuroscience, and psychology to build systems with understanding of context, interpretation of intent, and subtle response mechanisms. Cognition AI advances beyond simple automation by supporting systems that can interact, reason, and evolve.
Key Features of Cognition AI
1. Contextual Understanding:Â Understands the context of user queries, ensuring relevant and accurate responses.
2. Real-Time Adaptability:Â Learns from ongoing interactions and updates its understanding dynamically.
3. Human-Like Interaction: Uses NLP to communicate effectively and empathetically.
4. Problem-Solving:Â Analyzes complex datasets to find patterns and propose innovative solutions.
5. Decision Support:Â Provides data-driven recommendations tailored to specific scenarios.
How Does Cognition AI Work?
Cognition AI operates at the intersection of cognitive science and advanced AI technologies. The process involves:
1. Data gathering:Â Inclusion of various kinds of data coming from both structured and unstructured forms of text, images, audio, etc.
2. Pattern Recognition:Â Machine learning algorithms identifying trends, correlation, or anomalies in data.
3. Reasoning and Inference:Â Logical frameworks that interpret ambiguity or incompleteness in data.
4. Natural Language Understanding:Â NLPÂ is used for comprehension and conversational response in language.
5. Feedback Integration:Â Improvement with feedback loops and adaptation based on new inputs.
Cognition AI vs. Cognitive AI
While the terms “Cognition AI” and “Cognitive AI” are often used interchangeably, they have subtle distinctions:
1. Cognition AI
Generalized term:Â All types of AI inspired by the working of the human mind come under cognition AI.
Holistic Approach:Â It integrates diverse fields like cognitive science, neuroscience, and psychology to mimic comprehensive human intelligence, including reasoning, problem-solving, learning, and adaptation.
Applications: It’s used in various industries solving complex problems that have both human-like understanding and interactivity.
2. Cognitive AI
Focused Area:Â Cognitive AI is a subset of Cognition AI that focuses on duplicating specific cognitive functions, including decision-making, reasoning, and learning.
Targeted Solutions:Â This focuses on specific tasks or use cases, such as chatbots, recommendation systems, or decision-support tools.
Applications:Â Often deployed in customer service, healthcare diagnostics, and business intelligence, where specific cognitive abilities are critical.
Introducing Devon: A Catalyst in Cognition AI
Devon is one of the critical breakthroughs related to Cognition AI as a next-generation platform to speed up the incorporation of cognitive intelligence into real-world applications, combining robust machine learning models with cognitive computing principles to realize human-like interactions and decision-making capabilities.
Key Features of Devon:
1. Advanced NLP Capabilities: Devon excels in natural language understanding, allowing for smooth interaction with users.
2. Dynamic Adaptability:Â It learns continuously from user interactions, making sure that the responses are always up-to-date and contextually relevant.
3. Data Synthesis:Â Devon integrates and processes data from multiple sources to provide comprehensive insights.
4. User-Friendly Interface: It simplifies the deployment of Cognition AI solutions for businesses and developers.
Applications of Devon:
1. Customer Support:Â Enabling smart virtual assistants that provide human-like support experiences.
2. Healthcare:Â Aiding in diagnosis and treatment plans through data analysis in the context of real-time.
3. Retail:Â Customer engagement enhanced through personal recommendations and chatbots.
4. Finance:Â Assisting in risk assessment and fraud detection through real-time data analysis.
Devon exemplifies how platforms can bridge the gap between theoretical AI principles and practical, impactful solutions, making Cognition AI accessible to a broader audience.
Applications of Cognition AI
Cognition AI is revolutionizing industries by offering advanced solutions that were previously unattainable. Here are some of its transformative applications:
1. Health Care
Diagnostics:Â Analyzes medical records and imaging data to detect early onsets.
Personalized Medicine:Â Creates treatment plans based on individual patient profiles .
2. Finance
Fraud Detection: Anomalies are identified in real-time to prevent fraud risks.
Advisory on Investment:Â Uses predictive analytics to give personalized financial advice.
3. Retail
Customer Experience:Â AI-based chatbots and recommendations improve customer experience while shopping.
Inventory Management:Â Optimization of stock by demand forecasting.
4. Education
Adaptive Learning:Â Educational material that adapts to the needs of students.
Administrative Efficiency:Â Automates routine tasks, allowing educators to focus on teaching.
5. Customer Support
Virtual Assistants:Â Delivers human-like support through intelligent conversational agents.
Issue Resolution:Â Analyzes user complaints to propose effective solutions.
Benefits of Cognition AI
1. Enhanced Decision-Making:Â Actionable insights derived from comprehensive data analysis.
2. Increased Efficiency:Â Automates complex tasks, saving time and resources.
3. Personalization: Offers solutions that are tailored to the user’s preferences and behaviors.
4. Scalability:Â Adapts to growing data volumes and evolving business needs.
5. Innovation: Drives creative problem-solving across industries.
Challenges and Ethical Considerations
While Cognition AI offers great promise, it comes with challenges:
Bias in Data:Â AI systems might inherit biases from training datasets, leading to unfair results.
Privacy Concerns: Handling sensitive user data demands robust safeguards to ensure security.
Transparency:Â Ensuring AI systems provide explainable and accountable decision-making processes.
Dependence on Technology:Â Over-reliance on AI may diminish critical thinking skills in humans.
The Future of Cognition AI
With advancements in machine learning, NLP, and neuroscience, the potential of Cognition AI is endless. It will tackle the world’s most urgent problems: climate change, healthcare inequities, and sustainable urban development. It will become an essential component of smart cities, autonomous systems, and next-generation decision-making platforms.
Conclusion
Cognitive AI is the new paradigm that defines how human interaction with technology happens. By closing the gap between artificial intelligence and human cognition, it opens doors to smarter and more intuitive systems for industry transformation and improving lives. Platforms like Devon further speed up this change, exemplifying how theory can actually be used to create impacts in real-world applications. As businesses and researchers explore its possibilities, Cognition AI will undoubtedly shape the future of technology, ushering in an era of unprecedented innovation and collaboration.
If you are looking to build cutting-edge software or leverage Cognition AI for your business needs, we at Raybit Technologies are the perfect partners for your software development requirements. With our expertise in delivering innovative and reliable solutions, we ensure your projects are a step ahead in the digital age.
0 notes
Text
Operational Efficiency
Operational efficiency is a critical goal for any organization aiming to optimize performance, reduce costs, and improve productivity. Data Science plays a pivotal role in achieving this by leveraging data to streamline operations, automate processes, and enhance decision-making. This section explores how Data Science contributes to operational efficiency through various applications and methodologies.
Automation
Automation is a significant driver of operational efficiency, and Data Science is at the forefront of this revolution. By automating repetitive and mundane tasks, organizations can free up human resources for more strategic activities.
Robotic Process Automation (RPA)
Robotic Process Automation involves the use of software robots to automate routine tasks such as data entry, invoicing, and customer onboarding. RPA not only reduces the time required to perform these tasks but also minimizes human error, ensuring higher accuracy and efficiency.
Data Entry: Automating data entry processes reduces manual errors and speeds up data processing. For example, banks use RPA to process loan applications, extracting and inputting data from forms automatically.
Invoice Processing: RPA can handle invoice processing by extracting information from invoices, matching it with purchase orders, and entering it into accounting systems. This streamlines the accounts payable process and reduces processing time.
Customer Onboarding: In industries like finance and telecom, RPA helps in automating the customer onboarding process by verifying documents, conducting background checks, and setting up accounts, thereby enhancing the customer experience.
Intelligent Automation
Intelligent Automation combines RPA with Artificial Intelligence (AI) and Machine Learning (ML) to handle more complex tasks that require cognitive capabilities. This advanced form of automation is capable of making decisions, learning from data, and adapting to new situations.
Customer Service: AI-driven chatbots and virtual assistants provide 24/7 customer support, handling inquiries and resolving issues without human intervention. This not only improves customer satisfaction but also reduces the workload on human agents.
Fraud Detection: Intelligent automation systems analyze transaction patterns to detect fraudulent activities in real-time. For example, credit card companies use AI models to identify and block suspicious transactions automatically.
Supply Chain Optimization: Intelligent automation optimizes supply chain operations by predicting demand, managing inventory, and coordinating logistics. AI models can suggest optimal routes for delivery trucks, reducing fuel costs and delivery times.
Workflow Optimization
Data Science helps organizations optimize their workflows by identifying inefficiencies and bottlenecks in business processes. By analyzing process data, companies can streamline operations and improve productivity.
Employee Performance: Analyzing employee performance data helps in identifying areas for improvement and optimizing workforce allocation. For example, call centers use performance analytics to schedule shifts based on call volume predictions.
Production Processes: In manufacturing, data science analyzes production line data to identify inefficiencies and optimize workflows. For instance, predictive maintenance models can schedule maintenance activities during non-peak hours, reducing downtime.
Document Management: Automating document management processes, such as filing, retrieving, and sharing documents, improves efficiency and reduces the risk of errors. This is particularly important in sectors like healthcare and legal services where document handling is critical.
Resource Management
Efficient resource management is essential for maximizing productivity and minimizing waste. Data Science provides the tools needed to achieve optimal resource allocation and utilization.
Human Resources Management
Data Science plays a crucial role in managing human resources by analyzing employee data to optimize recruitment, performance, and retention strategies.
Recruitment: Predictive analytics models analyze historical hiring data to identify the characteristics of successful employees. This helps in screening and selecting candidates who are more likely to perform well and stay with the company.
Performance Management: Data-driven performance management systems track employee performance metrics and provide insights into areas for improvement. This helps in setting realistic goals, providing targeted training, and recognizing top performers.
Retention Strategies: Predictive models identify employees at risk of leaving the organization by analyzing factors such as job satisfaction, engagement levels, and career progression. This enables HR teams to implement proactive retention strategies, such as personalized career development plans.
Asset Management
Effective management of physical assets is crucial for reducing operational costs and maximizing their lifespan. Data Science helps in monitoring and maintaining assets more efficiently.
Predictive Maintenance: Predictive maintenance models analyze sensor data from equipment to predict potential failures before they occur. This allows for scheduled maintenance activities, reducing downtime and maintenance costs.
Inventory Optimization: Data Science optimizes inventory management by predicting demand and adjusting stock levels accordingly. For example, retailers use demand forecasting models to ensure they have the right products in stock without overstocking.
Asset Utilization: By analyzing usage patterns, organizations can optimize the utilization of their assets. For instance, transportation companies use telematics data to optimize fleet usage and reduce fuel consumption.
Energy Management
Data Science is instrumental in optimizing energy consumption, leading to cost savings and environmental benefits.
Smart Grids: Smart grids use data analytics to monitor and manage electricity distribution in real-time. This ensures efficient energy use, reduces losses, and integrates renewable energy sources more effectively.
Building Management: Data science optimizes energy use in buildings by analyzing data from HVAC systems, lighting, and other utilities. Smart building systems adjust energy consumption based on occupancy patterns and external weather conditions.
Industrial Processes: In industrial settings, data analytics optimize energy use in production processes. For example, predictive models can adjust machinery settings to minimize energy consumption while maintaining production efficiency.
Risk Management Managing risk is crucial for the stability and growth of any organization. Data Science enhances risk management through predictive analytics and real-time monitoring.
Financial Risk Management
Financial institutions rely on Data Science to assess and mitigate various types of financial risk.
Credit Risk: Predictive models analyze credit history, transaction data, and other factors to assess the creditworthiness of loan applicants. This helps banks make informed lending decisions and reduce default rates.
Market Risk: Data Science models analyze market trends and economic indicators to predict potential market risks. This allows financial institutions to adjust their investment strategies and minimize losses.
Operational Risk: Analyzing operational data helps in identifying and mitigating risks associated with internal processes, systems, and human errors. For example, banks use data analytics to monitor compliance with regulatory requirements and prevent operational failures.
Operational Risk Management
Data Science helps organizations identify and mitigate operational risks across various domains.
Supply Chain Risks: Predictive analytics models forecast potential supply chain disruptions due to factors like natural disasters, geopolitical events, or supplier issues. This enables companies to develop contingency plans and ensure business continuity.
Equipment Failure: Predictive maintenance models analyze sensor data from machinery to predict equipment failures. This allows for proactive maintenance, reducing downtime and preventing costly breakdowns.
Compliance and Safety: Data Science helps organizations monitor compliance with safety regulations and standards. For example, in the construction industry, analytics models analyze safety data to identify potential hazards and implement preventive measures.
Cybersecurity
In the digital age, cybersecurity is a critical concern for organizations. Data Science plays a key role in enhancing cybersecurity through anomaly detection and threat prediction.
Anomaly Detection: Machine learning models analyze network traffic to detect unusual patterns that may indicate a cyber attack. For example, sudden spikes in data transfer or unusual login activities can trigger alerts for further investigation.
Threat Prediction: Predictive analytics models identify potential threats by analyzing historical attack data and emerging threat patterns. This helps organizations implement preventive measures and strengthen their security posture.
Incident Response: Data Science aids in the incident response process by analyzing attack patterns and determining the source and impact of a breach. This enables quicker resolution and minimizes damage.
Cost Reduction
Reducing operational costs is a primary objective for businesses aiming to improve their bottom line. Data Science helps organizations identify cost-saving opportunities and implement efficient practices.
Supply Chain Optimization
Optimizing supply chain operations through data analytics leads to significant cost savings.
Demand Forecasting: Predictive models forecast demand for products, helping businesses maintain optimal inventory levels. This prevents overstocking and stockouts, reducing inventory carrying costs and lost sales.
Logistics Efficiency: Data Science optimizes logistics by analyzing transportation data to identify the most efficient routes and modes of delivery. This reduces fuel consumption, delivery times, and transportation costs.
Procurement Strategies: Analyzing procurement data helps organizations identify cost-saving opportunities in supplier selection, contract negotiation, and purchasing practices. For example, companies can use spend analysis to consolidate purchases and negotiate better terms with suppliers.
Operational Efficiency
Streamlining operations through data-driven insights leads to cost savings and improved productivity.
Process Automation: Automating routine tasks reduces labor costs and increases efficiency. For example, automating invoice processing reduces the need for manual data entry, speeding up the accounts payable process.
Workflow Optimization: Analyzing workflow data helps identify inefficiencies and optimize business processes. For instance, lean manufacturing principles combined with data analytics can reduce waste and improve production efficiency.
Resource Allocation: Data Science optimizes resource allocation by predicting demand and adjusting staffing levels accordingly. For example, call centers use predictive models to schedule shifts based on call volume forecasts, reducing labor costs.
Energy Savings
Optimizing energy consumption leads to significant cost savings and environmental benefits.
Smart Energy Management: Data Science enables smart energy management systems that monitor and control energy use in real-time. For example, smart thermostats adjust heating and cooling settings based on occupancy and weather conditions, reducing energy costs.
Industrial Energy Efficiency: Analyzing energy consumption data in industrial processes helps identify inefficiencies and implement energy-saving measures. For instance, optimizing machine settings can reduce energy use without compromising production output.
Renewable Energy Integration: Data Science helps integrate renewable energy sources into the grid more effectively. Predictive models forecast energy production from solar and wind sources, allowing for better planning and utilization.
Quality Control
Maintaining high-quality standards is essential for customer satisfaction and business success. Data Science enhances quality control through advanced analytics and real-time monitoring.
Manufacturing Quality
In manufacturing, maintaining product quality is crucial for customer satisfaction and regulatory compliance. Data Science helps in monitoring and controlling quality throughout the production process.
Defect Detection: Machine learning models analyze production data to detect defects and anomalies in real-time. This allows for immediate corrective actions, reducing the number of defective products and ensuring high-quality standards.
Process Control: Statistical process control (SPC) techniques combined with data analytics monitor production processes to ensure they stay within predefined quality limits. For example, analyzing temperature and pressure data in real-time helps maintain optimal conditions for product manufacturing.
Supplier Quality Management: Data Science helps in monitoring the quality of raw materials supplied by vendors. By analyzing supplier performance data, companies can identify reliable suppliers and negotiate better terms.
Service Quality
In service industries, maintaining high service quality is critical for customer retention and competitive advantage. Data Science provides insights into service performance and customer satisfaction.
Customer Feedback Analysis: Analyzing customer feedback and reviews helps in identifying areas for improvement. For example, sentiment analysis of social media comments provides insights into customer perceptions and experiences.
Service Performance Metrics: Monitoring key performance indicators (KPIs) such as response time, resolution time, and customer satisfaction scores helps in maintaining high service standards. Data-driven insights enable service managers to address issues promptly and improve service quality.
Personalized Service: Data Science enables personalized service experiences by analyzing customer data and preferences. For instance, recommendation systems in online platforms suggest products and services based on individual customer profiles.
Compliance
Ensuring compliance with regulations and standards is critical for avoiding penalties and maintaining reputation. Data Science helps organizations monitor compliance and identify potential issues.
Regulatory Compliance: Analyzing compliance data helps organizations ensure they meet regulatory requirements. For example, financial institutions use data analytics to monitor transactions and detect potential money laundering activities.
Safety Standards: In industries such as healthcare and manufacturing, maintaining safety standards is crucial. Data Science helps in monitoring safety data and identifying potential hazards, ensuring compliance with safety regulations.
Audit and Reporting: Data-driven audit and reporting systems provide accurate and timely information for regulatory audits. This reduces the risk of non-compliance and ensures transparency in business operations.
Inventory Management
Effective inventory management is essential for minimizing costs and meeting customer demand. Data Science optimizes inventory levels through predictive analytics and real-time monitoring.
Demand Forecasting
Accurate demand forecasting is crucial for maintaining optimal inventory levels. Data Science models analyze historical sales data, market trends, and other factors to predict future demand.
Seasonal Trends: Predictive models account for seasonal variations in demand, helping businesses stock up on popular items during peak seasons and reduce inventory during off-peak periods.
Market Conditions: Analyzing market conditions and consumer behavior provides insights into potential demand shifts. For example, economic indicators and competitor activities can influence demand for certain products.
Promotional Impact: Data Science helps in predicting the impact of promotional activities on demand. This enables businesses to adjust inventory levels and avoid stockouts or overstock situations.
Inventory Optimization
Optimizing inventory levels involves balancing the cost of holding inventory with the need to meet customer demand. Data Science provides the tools needed to achieve this balance.
Safety Stock: Predictive models calculate optimal safety stock levels to buffer against uncertainties in demand and supply. This ensures that businesses can meet customer demand without incurring excessive holding costs.
Reorder Points: Data Science determines optimal reorder points based on demand forecasts and lead times. This helps in maintaining inventory levels and preventing stockouts.
Inventory Turnover: Analyzing inventory turnover ratios helps in identifying slow-moving and fast-moving items. This enables businesses to make informed decisions about purchasing, stocking, and pricing strategies.
Supply Chain Coordination
Effective supply chain coordination is essential for optimizing inventory management. Data Science enhances visibility and collaboration across the supply chain.
Real-Time Monitoring: Real-time monitoring systems provide visibility into inventory levels and movement across the supply chain. This enables businesses to respond quickly to changes in demand and supply conditions.
Supplier Collaboration: Data-driven insights enhance collaboration with suppliers, ensuring timely deliveries and reducing lead times. For example, sharing demand forecasts with suppliers helps in synchronizing production and delivery schedules.
Logistics Optimization: Data Science optimizes logistics operations by analyzing transportation data to identify the most efficient routes and modes of delivery. This reduces transportation costs and improves delivery times.
Warehouse Management
Efficient warehouse management is crucial for optimizing inventory storage and retrieval processes. Data Science provides insights into warehouse operations and helps in improving efficiency.
Layout Optimization: Analyzing warehouse layout data helps in optimizing storage space and reducing retrieval times. For example, frequently picked items can be stored closer to the shipping area to speed up order fulfillment.
Inventory Tracking: Real-time inventory tracking systems provide accurate information about inventory levels and locations within the warehouse. This reduces the risk of stockouts and overstock situations.
Order Fulfillment: Data Science optimizes order fulfillment processes by analyzing order data and warehouse operations. For example, batch picking strategies can reduce the time required to pick and pack orders, improving overall efficiency.
In conclusion, Data Science is a powerful tool for enhancing operational efficiency across various domains. By leveraging data-driven insights, organizations can automate processes, optimize resource management, manage risks effectively, reduce costs, maintain high-quality standards, and manage inventory efficiently. As data continues to grow in volume and complexity, the role of Data Science in driving operational efficiency will only become more critical, shaping the future of business and industry.
Read More on Strategic Leap
0 notes
Text
Master the Machine: AI Certifications Leading Innovation in Tech
The age of artificial intelligence (AI) is upon us, transforming industries and redefining the skills professionals need to succeed. From self-driving cars to personalized healthcare, AI-powered technologies are driving innovation like never before. To stay competitive, tech professionals must adapt — and the fastest way to demonstrate expertise is through industry-recognized certifications.
This article explores the top AI certifications that empower professionals to master the machine and lead innovation in tech. Whether you’re an AI novice or an experienced engineer, these certifications offer a pathway to excellence in one of the most transformative fields of our time.

Why AI Certifications Are Essential
The rapid adoption of AI has created a massive demand for skilled professionals. Certifications provide the perfect solution for bridging the skills gap, offering:
Validated Expertise: Certifications are proof of your AI knowledge and skills.
Career Advancement: They open doors to high-paying roles in AI development, data science, and more.
Networking Opportunities: Certification programs often connect you with like-minded professionals and industry leaders.
Future-Proofing: AI skills are relevant across industries, from finance to healthcare.
Top AI Certifications Leading Innovation in Tech
Here are the certifications that are shaping the future of AI, offering cutting-edge knowledge and practical skills.
1. AI+ Robotics™ by AI Certs
Designed to prepare professionals for the intersection of AI and robotics, the AI+ Robotics™ certification by AI Certs is perfect for those looking to specialize in automated systems and intelligent machines.
Key Features:
Covers robotic process automation (RPA), deep learning, and advanced AI applications in robotics.
Includes hands-on projects that simulate real-world robotics challenges.
Focuses on ethical considerations in deploying AI in robotics.
Who It’s For:
Engineers, roboticists, and tech professionals keen on automating processes with AI.
Why It Matters:
As robotics and AI converge, professionals with expertise in both fields are in high demand. The AI+ Robotics™ certification equips you with the knowledge and tools to lead innovation in this exciting domain.
Use the coupon code NEWCOURSE25 to get 25% OFF on AI CERTS’ certifications. Don’t miss out on this limited-time offer! Visit this link to explore the courses and enroll today.
2. Microsoft Certified: Azure AI Engineer Associate
Microsoft’s Azure AI certification focuses on creating AI solutions using the Azure platform.
Key Features:
Comprehensive training in Azure AI tools, including Azure Cognitive Services.
Covers AI integration with cloud-based applications.
Hands-on labs to apply skills in real-world scenarios.
Who It’s For:
Professionals looking to specialize in AI cloud services.
Engineers using Microsoft Azure in their projects.
Why It Matters:
With cloud-based AI services becoming a standard in the tech industry, expertise in platforms like Azure is crucial for staying competitive.
3. Certified Artificial Intelligence Practitioner (CAIP)
Offered by CertNexus, the CAIP certification provides foundational AI knowledge with a focus on business applications.
Key Features:
Covers machine learning, natural language processing, and computer vision.
Focuses on AI integration into business strategies.
Includes practical exercises to implement AI solutions.
Who It’s For:
Professionals aiming to leverage AI for strategic decision-making.
Tech leads and business analysts working on AI projects.
Why It Matters:
The CAIP certification bridges the gap between technical expertise and business acumen, making it ideal for professionals aiming to lead AI initiatives in corporate settings.
Choosing the Right AI Certification
Selecting the best certification depends on your career goals, skill level, and area of interest.
1. Align with Career Objectives
Engineering and Robotics: Opt for AI+ Robotics™ to master AI in automation and robotics.
Cloud-Based AI: Choose Microsoft’s Azure certification for cloud-specific expertise.
Business Strategy: Go for the CAIP certification to integrate AI into business frameworks.
2. Consider Industry Relevance
Research job postings in your field to identify which certifications are most in-demand.
3. Evaluate the Curriculum
Look for certifications that balance theory with practical application to ensure you gain actionable skills.
The Impact of AI Certifications
AI certifications are more than just credentials — they’re a gateway to professional growth.
1. Enhanced Earning Potential
AI-certified professionals often command higher salaries. For instance, AI engineers earn an average of $150,000 per year in the U.S.
2. Diverse Career Opportunities
AI certifications are versatile, opening roles in data science, machine learning, robotics, and more.
3. Leadership Roles
With certifications, you’re better positioned to lead AI projects and drive innovation within your organization.
4. Global Recognition
Many AI certifications, like those from AI Certs and Microsoft, are recognized worldwide, making you a valuable asset in any job market.
Future Trends in AI Certifications
As AI continues to evolve, certification programs are adapting to meet emerging needs:
Specialized Certifications: Expect more niche programs in areas like AI ethics, quantum computing, and edge AI.
Cross-Disciplinary Skills: Certifications will increasingly combine AI with fields like cybersecurity and healthcare.
Flexible Learning: Online, self-paced courses are becoming the norm, accommodating busy professionals.

Conclusion
AI certifications are the key to mastering the machine and leading innovation in tech. Programs like AI+ Robotics™ by AI Certs, Microsoft’s Azure AI certification, and the CAIP certification stand out for their real-world relevance and practical focus.
By investing in the right certification, you can unlock new career opportunities, enhance your earning potential, and position yourself as a leader in one of the most exciting fields of our time. Whether you’re just starting or looking to specialize, these certifications will equip you with the skills needed to thrive in an AI-driven future.
Now is the time to embrace the AI revolution. Choose your certification, master the machine, and become a driving force in tech innovation.
0 notes
Text
Enhance Efficiency with Comprehensive Manufacturing Technology Solutions
In today's rapidly evolving industrial landscape, manufacturers are constantly seeking innovative ways to optimize their operations, increase productivity, and gain a competitive edge. This is where comprehensive manufacturing technology solutions come into play. By leveraging cutting-edge technologies, manufacturers can streamline their processes, improve quality control, and enhance overall efficiency.
Industry 4.0 Manufacturing: The Future of Production
Industry 4.0, also known as the Fourth Industrial Revolution, is characterized by the integration of cyber-physical systems, the Internet of Things (IoT), cloud computing, and cognitive computing. These technologies are transforming the manufacturing sector by enabling real-time data collection, analysis, and decision-making. Â
Key Manufacturing Technology Solutions
Several key manufacturing technology solutions are driving this transformation:
Digital Manufacturing Solutions: These solutions leverage digital technologies such as 3D printing, computer-aided design (CAD), and computer-aided manufacturing (CAM) to accelerate product development, improve design accuracy, and reduce production costs.
Microsoft Cloud for Manufacturing: Microsoft's cloud-based solutions offer a range of tools and services that can help manufacturers optimize their operations, improve supply chain management, and enhance customer relationships.
Manufacturing Solutions: A wide range of manufacturing solutions are available, from enterprise resource planning (ERP) systems to supply chain management (SCM) software, all designed to improve efficiency and productivity.
Benefits of Implementing Manufacturing Technology Solutions
By implementing these solutions, manufacturers can experience a range of benefits, including:
Increased Efficiency: Automation and data-driven decision-making can significantly improve operational efficiency and reduce production times.
Improved Quality: Real-time data analysis and quality control systems can help identify and address quality issues proactively.
Enhanced Flexibility: Flexible manufacturing systems can quickly adapt to changing market demands and customer requirements.
Reduced Costs: Automation and streamlined processes can help reduce labor costs, material waste, and energy consumption.
Increased Innovation: Access to real-time data and advanced analytics can drive innovation and new product development.
Conclusion
In conclusion, comprehensive manufacturing technology solutions are essential for businesses looking to thrive in today's competitive market. By embracing Industry 4.0 technologies and implementing the right solutions, manufacturers can enhance efficiency, improve quality, and gain a sustainable competitive advantage.
Ready to transform your manufacturing operations?
Contact Celebal Technologies today to learn more about our comprehensive manufacturing technology solutions and how we can help you achieve your business goals.
0 notes
Text
Must-Have Process Automation Tools for Non-Tech Teams: Boost Efficiency with Ease
Is your team bogged down with repetitive tasks and laborious manual processes? Sometimes there’s no alternate way of doing things manually. But, advances in machine learning and artificial intelligence (AI) have made it easier to automate corporate processes than ever before. QKS Group Reveals that Robotic Process Automation Projected to Register a CAGR of above 32.30% by 2028. 
You don’t need to know how to code or build machine learning models. Business process automation tools make it a cinch to introduce automation to non-technical team members—provided you choose the correct solution.
Choosing a process automation tool is no small task, so think carefully before committing. In this article, we'll discuss what you look for in process automation technologies and recommend the finest robotic platforms for 2024.
Download the sample report of Market Share: https://qksgroup.com/download-sample-form/market-share-robotic-process-automation-rpa-2023-worldwide-2418 Â
What Should You Look for in Robotic Process Automation Tools?
There are several process automation tools on the market, but at a minimum, your workflow automation software should offer the following features:
Robotic process automation (RPA): Robotic Process Automation is a unique way of conveying the software that operates bots to do tasks for you. Some platforms offer only trigger-based automation, which is incredible, but RPA takes even more tedious tasks off your plate.
Integrations: We aren’t just discussing about Zapier links here. Glance for solutions that incorporate natively Excel, Gmail, Slack, social media, and any other platforms you use
Scalability: You should be capable of using the automation tool for multiple use cases. For instance, you might start utilizing it just for data entry, but over time, you extend it to analyze and enhance processes for accounting, marketing, and more.
User-friendly interface: The more instinctive a solution, the faster you’ll see the importance of it. Go for process automation software that is either no-code or low-code. A drag-and-drop interface is also a must-have.
By seeing all these points, everyone knows that RPA is increasingly popular amongst vendors and end-users due to obvious benefits including overall cost reduction, end-to-end process streamlining, and driving high-value customer experience. Additionally, end-to-end RPA implementation doesn’t require organizations to undertake any significant changes in their existing IT infrastructure, or their IT Team to spend time and energy on acclimatizing and implementing the new tools after thorough research.
The Process Automation Market Share, 2023, Worldwide study and Market Forecast: Process Automation, 2024-2028, Worldwide report are essential for gaining a thorough grasp of these rising threats. Thus, the overall RPA usage is exponentially increasing owing to the RPA systems’ robust integrations with the centralized IT governance, in addition to the modern RPA systems being strengthened by AI, NLP, and machine vision for ably mitigating breakage or system inconsistencies if any. Ultimately, the majority RPA vendors are moving towards providing robust intelligent process automation (IPA) solutions, and further evolving their cognitive automation capabilities for the provision of a hyper-automation suite. Â
Download the sample report of Market Forecast: https://qksgroup.com/download-sample-form/market-forecast-robotic-process-automation-rpa-2024-2028-worldwide-2222Â
Top Robotic Process Automation Tools for 2024
Microsoft Power Automate
This process automation software allows you to focus on critical business operations while automating the rest. All repetitious procedures may be simplified to save money and improve efficiency. This BPA platform enables businesses to securely automate their operations at scale by leveraging low-code, AI technology. The multiple connectors that come with the software allow users to integrate with existing applications and systems easily.
Nintex Workflow Cloud
Nintex provides easy, powerful process automation that can manage all of your company operations at a minimal cost. You may create sophisticated workflows in minutes without coding. This program allows you to automate, manage, and optimize all operations without having to write any code. Nintex is a cloud-based software that is simple to use and highly visual, allowing for easy digital transformation.
TrackVia
This is a well-designed low-code workflow automation software for managing processes. The drag-and-drop interface makes it simple for users to create apps and automated procedures. The primary focus is on mobile processes, including Zapier integration and responsive dashboards. Because of predefined layouts, modification is limited. TrackVia allows you to design and configure, integrate and fill, monitor, and optimize.
Appian RPA
Appian is a low-code automation platform that helps businesses automate processes, create apps, and manage workflows. Appian's user-friendly interface and sophisticated automation features enable enterprises to accelerate digital transformation and process improvement.
Talk To Analyst:Â https://qksgroup.com/become-clientÂ
Conclusion
Choosing the finest Robotic Process Automation (RPA) software for your company is a significant choice that must be made after thorough evaluation of several aspects. By examining your company requirements, reviewing critical criteria, researching available solutions, and performing rigorous assessments, you can select an RPA software solution that matches with your objectives and allows you to optimize your operations, increase efficiency, and drive growth. Remember to consider usability, integration capabilities, scalability, security features, and support services to guarantee a successful installation and optimize the benefits of automation for your firm.
0 notes
Text
Key Technologies and Tools to Build AI Agents Effectively

The development of AI agents has revolutionized how businesses operate, offering automation, enhanced customer interactions, and data-driven insights. Building an effective AI agent requires a combination of the right technologies and tools. This blog delves into the key technologies and tools essential for creating intelligent and responsive AI agents that can drive business success.
1. Machine Learning Frameworks
Machine learning frameworks provide the foundational tools needed to develop, train, and deploy AI models.
TensorFlow: An open-source framework developed by Google, TensorFlow is widely used for building deep learning models. It offers flexibility and scalability, making it suitable for both research and production environments.
PyTorch: Developed by Facebook, PyTorch is known for its ease of use and dynamic computational graph, which makes it ideal for rapid prototyping and research.
Scikit-learn: A versatile library for machine learning in Python, Scikit-learn is perfect for developing traditional machine learning models, including classification, regression, and clustering.
2. Natural Language Processing (NLP) Tools
NLP tools are crucial for enabling AI agents to understand and interact using human language.
spaCy: An open-source library for advanced NLP in Python, spaCy offers robust support for tokenization, parsing, and named entity recognition, making it ideal for building conversational AI agents.
NLTK (Natural Language Toolkit): A comprehensive library for building NLP applications, NLTK provides tools for text processing, classification, and sentiment analysis.
Transformers by Hugging Face: This library offers state-of-the-art transformer models like BERT, GPT-4, and others, enabling powerful language understanding and generation capabilities for AI agents.
3. AI Development Platforms
AI development platforms streamline the process of building, training, and deploying AI agents by providing integrated tools and services.
Dialogflow: Developed by Google, Dialogflow is a versatile platform for building conversational agents and chatbots. It offers natural language understanding, multi-platform integration, and customizable responses.
Microsoft Bot Framework: This framework provides a comprehensive set of tools for building intelligent bots that can interact across various channels, including websites, messaging apps, and voice assistants.
Rasa: An open-source framework for building contextual AI assistants, Rasa offers flexibility and control over your AI agent’s conversational capabilities, making it suitable for customized and complex applications.
4. Cloud Computing Services
Cloud computing services provide the necessary infrastructure and scalability for developing and deploying AI agents.
AWS (Amazon Web Services): AWS offers a suite of AI and machine learning services, including SageMaker for model building and deployment, and Lex for building conversational interfaces.
Google Cloud Platform (GCP): GCP provides tools like AI Platform for machine learning, Dialogflow for conversational agents, and AutoML for automated model training.
Microsoft Azure: Azure’s AI services include Azure Machine Learning for model development, Azure Bot Service for building intelligent bots, and Cognitive Services for adding pre-built AI capabilities.
5. Data Management and Processing Tools
Effective data management and processing are essential for training accurate and reliable AI agents.
Pandas: A powerful data manipulation library in Python, Pandas is essential for cleaning, transforming, and analyzing data before feeding it into AI models.
Apache Spark: An open-source unified analytics engine, Spark is ideal for large-scale data processing and real-time analytics, enabling efficient handling of big data for AI training.
Data Lakes and Warehouses: Solutions like Amazon S3, Google BigQuery, and Snowflake provide scalable storage and efficient querying capabilities for managing vast amounts of data.
6. Development and Collaboration Tools
Collaboration and efficient development practices are crucial for successful AI agent projects.
GitHub: A platform for version control and collaboration, GitHub allows multiple developers to work together on AI projects, manage code repositories, and track changes.
Jupyter Notebooks: An interactive development environment, Jupyter Notebooks are widely used for exploratory data analysis, model prototyping, and sharing insights.
Docker: Containerization with Docker ensures that your AI agent’s environment is consistent across development, testing, and production, facilitating smoother deployments.
7. Testing and Deployment Tools
Ensuring the reliability and performance of AI agents is critical before deploying them to production.
CI/CD Pipelines: Continuous Integration and Continuous Deployment (CI/CD) tools like Jenkins, GitLab CI, and GitHub Actions automate the testing and deployment process, ensuring that updates are seamlessly integrated.
Monitoring Tools: Tools like Prometheus, Grafana, and AWS CloudWatch provide real-time monitoring and alerting, helping you maintain the performance and reliability of your AI agents post-deployment.
A/B Testing Platforms: Platforms like Optimizely and Google Optimize enable you to conduct A/B tests, allowing you to evaluate different versions of your AI agent and optimize its performance based on user interactions.
Best Practices for Building AI Agents
Start with Clear Objectives: Define the specific tasks and goals your AI agent should achieve to guide the development process.
Ensure Data Quality: Use high-quality, relevant data for training your AI models to enhance accuracy and reliability.
Prioritize User Experience: Design your AI agent with the end-user in mind, ensuring intuitive interactions and valuable responses.
Maintain Security and Privacy: Implement robust security measures to protect user data and comply with relevant regulations.
Iterate and Improve: Continuously monitor your AI agent’s performance and make iterative improvements based on feedback and data insights.
Conclusion
Building an effective AI agent involves a strategic blend of the right technologies, tools, and best practices. By leveraging machine learning frameworks, NLP tools, AI development platforms, cloud services, and robust data management systems, businesses can create intelligent and responsive AI agents that drive operational efficiency and enhance customer experiences. Embracing these technologies not only streamlines the development process but also ensures that your AI agents are scalable, reliable, and aligned with your business objectives.
Whether you’re looking to build a customer service chatbot, a virtual assistant, or an advanced data analysis tool, following a structured approach and utilizing the best available tools will set you on the path to success. Start building your AI agent today and unlock the transformative potential of artificial intelligence for your business.
0 notes
Text

Seven Most In-Demand Job Skills for 2025
Here’s a detailed description for each of the seven most in-demand job skills for 2025:
1. Data Analysis and Interpretation
As data continues to drive decision-making across industries, professionals skilled in analyzing and interpreting data are invaluable. This skill involves collecting, organizing, and analyzing large datasets to identify trends, patterns, and insights that inform business strategies and operational improvements. Proficiency in tools like Excel, SQL, Python, and visualization platforms such as Tableau or Power BI is critical.
2. Digital Literacy and Tech Savviness
The digital transformation of workplaces requires employees to be proficient with various digital tools, platforms, and software. Digital literacy includes the ability to navigate emerging technologies, understand cybersecurity basics, and utilize digital communication tools effectively. Tech-savvy individuals can easily adapt to new software and workflows, increasing productivity and efficiency in dynamic work environments.
3. Artificial Intelligence (AI) and Machine Learning (ML)
AI and ML are revolutionizing industries, from automation to personalized user experiences. Understanding AI tools, algorithms, and machine learning concepts allows professionals to automate processes, analyze complex data, and create intelligent solutions. Skills in AI model development, coding (Python, R), and familiarity with platforms like TensorFlow and PyTorch are becoming increasingly essential.
4. Creativity and Innovation
In a rapidly changing world, businesses need individuals who can think outside the box, generate fresh ideas, and drive innovation. Creativity involves conceptualizing new products, processes, or solutions, while innovation focuses on implementing these ideas effectively. This skill is particularly vital in competitive industries like marketing, design, technology, and entrepreneurship.
5. Critical Thinking and Problem-Solving
Employers value professionals who can analyze problems, evaluate information, and develop logical, effective solutions. Critical thinking involves assessing situations objectively, questioning assumptions, and making decisions based on evidence and reasoning. It is a universal skill applicable across roles, from strategic planning to troubleshooting technical challenges.
6. Adaptability and Flexibility
The modern workplace demands agility as industries evolve and technologies advance. Adaptability involves embracing change, learning new skills, and adjusting to shifting priorities or roles. Employees who demonstrate flexibility thrive in uncertain environments, easily handling challenges like remote work, organizational restructuring, or emerging technologies.
7. Emotional Intelligence and Interpersonal Skills
Success in any role depends on the ability to understand and manage emotions—both one’s own and others’. Emotional intelligence includes empathy, self-awareness, and effective communication. Interpersonal skills such as teamwork, conflict resolution, and leadership foster strong workplace relationships and enhance collaboration, critical for team success in diverse and hybrid work environments.
This combination of technical, cognitive, and emotional skills will empower professionals to navigate the evolving job market and thrive in 2025 and beyond.
Source -Â
1 note
·
View note