#approaches of ETL testing
Explore tagged Tumblr posts
Text
Approaches to ETL Testing: Ensuring Data Quality and Reliability
ETL TESTING
ETL testing (Extract, Transform, Load) is critical in data management and warehousing. It is the process of verifying the accuracy, completeness, and reliability of data as it moves through the ETL pipeline. ETL testing aims to identify any issues, inconsistencies, or errors in data, ensuring that the final data in the target system or data warehouse is of high quality and can be relied upon for decision-making.
The Importance of Different Approaches of ETL Testing
In the world of data management, where data is often the lifeblood of organizations, different approaches of ETL testing have a critical role to play. These approaches encompass a range of testing methodologies, each serving specific purposes and offering unique benefits. They are designed to scrutinize the accuracy, completeness, and consistency of data as it traverses through the ETL pipeline, ensuring that the final data in the target system or data warehouse is of the highest quality and can be trusted for making informed decisions.
Manual Testing: One of the Fundamental Approaches of ETL Testing
Manual testing is one of the fundamental approaches of ETL testing. In manual testing, human testers execute predefined test cases and meticulously validate ETL processes through visual inspection and comparisons. This approach is ideal for smaller ETL projects or the initial testing phases, where automation may not be cost-effective. Manual testing offers the flexibility to adapt to evolving ETL pipelines and is invaluable for exploratory testing, allowing testers to explore the ETL process without predefined test cases.
Automation Testing: Harnessing Automation as One of the Approaches of ETL Testing
Automation testing is another of the fundamental approaches of ETL testing. This approach involves the use of ETL testing tools and scripts to automate the execution of test cases and the validation of ETL processes. Automation testing is highly efficient for large and complex ETL pipelines, where manual testing is impractical. It saves time and reduces the risk of human error, making it ideal for repetitive testing tasks, regression testing, and ensuring that ETL processes work consistently.
Incremental Testing: A Focused Approach of ETL Testing
Incremental testing is a focused approach of ETL testing that concentrates on testing only the new or changed data within the ETL process. Instead of retesting the entire dataset, testers zero in on the data that has been added or modified since the last ETL process run. This approach is beneficial in scenarios where source data experiences frequent changes, optimizing testing efforts and speeding up the testing cycle.
Data Sampling: A Pragmatic Approach of ETL Testing
Data sampling is a pragmatic approach of ETL testing that involves selecting a subset of data from the source and target systems for testing. This approach is particularly useful when dealing with exceptionally large datasets, where testing the entire dataset is impractical. Data sampling provides a snapshot of data quality without the need for exhaustive testing.
End-to-End Testing: A Holistic Approach of ETL Testing
End-to-End testing is a holistic approach of ETL testing that validates the complete ETL pipeline. It encompasses testing data extraction, transformation, and loading. This approach ensures that all components of the ETL process work together seamlessly, verifying that data is correctly extracted, transformed, and loaded without any issues. It is critical for achieving comprehensive ETL process validation.
Data Profiling: Unearthing Insights Through Another Approach of ETL Testing
Data profiling is a highly insightful approach of ETL testing that involves analyzing data to identify patterns, anomalies, and data quality issues. It helps testers gain a deep understanding of the characteristics of the data, enabling the creation of meaningful test scenarios. Data profiling is invaluable for understanding data quality, identifying potential issues, and developing effective test cases.
Integration Testing: Ensuring Interoperability Through Another Approach of ETL Testing
Integration testing is an approach of ETL testing that verifies the integration of the ETL process with other systems, databases, and applications. In complex ETL environments where the ETL process interacts with multiple systems, integration testing ensures that data flows smoothly between systems. It is critical for identifying and addressing integration issues.
Performance Testing: Ensuring Efficiency Through Another Approach of ETL Testing
Performance testing is an approach of ETL testing that focuses on evaluating the efficiency, scalability, and response times of the ETL process. It is essential when dealing with large volumes of data, ensuring that the ETL process meets performance requirements and identifying performance bottlenecks.
Regression Testing: Safeguarding Stability Through Another Approach of ETL Testing
Regression testing is an approach of ETL testing that involves retesting the entire ETL process after making changes or enhancements. This approach ensures that new modifications do not introduce defects or disrupt existing functionality. Regression testing safeguards the stability of the ETL process as it evolves, preventing unintended issues and maintaining data quality.
conclusion
different approaches of ETL testing are the cornerstone of data quality and reliability in the realm of data management and data warehousing. By comprehending these various testing methodologies, organizations can implement effective ETL testing strategies that align with their specific needs, ensuring that their data is reliable and trustworthy for informed decision-making
0 notes
Text
Essential Predictive Analytics Techniques
With the growing usage of big data analytics, predictive analytics uses a broad and highly diverse array of approaches to assist enterprises in forecasting outcomes. Examples of predictive analytics include deep learning, neural networks, machine learning, text analysis, and artificial intelligence.
Predictive analytics trends of today reflect existing Big Data trends. There needs to be more distinction between the software tools utilized in predictive analytics and big data analytics solutions. In summary, big data and predictive analytics technologies are closely linked, if not identical.
Predictive analytics approaches are used to evaluate a person's creditworthiness, rework marketing strategies, predict the contents of text documents, forecast weather, and create safe self-driving cars with varying degrees of success.
Predictive Analytics- Meaning
By evaluating collected data, predictive analytics is the discipline of forecasting future trends. Organizations can modify their marketing and operational strategies to serve better by gaining knowledge of historical trends. In addition to the functional enhancements, businesses benefit in crucial areas like inventory control and fraud detection.
Machine learning and predictive analytics are closely related. Regardless of the precise method, a company may use, the overall procedure starts with an algorithm that learns through access to a known result (such as a customer purchase).
The training algorithms use the data to learn how to forecast outcomes, eventually creating a model that is ready for use and can take additional input variables, like the day and the weather.
Employing predictive analytics significantly increases an organization's productivity, profitability, and flexibility. Let us look at the techniques used in predictive analytics.
Techniques of Predictive Analytics
Making predictions based on existing and past data patterns requires using several statistical approaches, data mining, modeling, machine learning, and artificial intelligence. Machine learning techniques, including classification models, regression models, and neural networks, are used to make these predictions.
Data Mining
To find anomalies, trends, and correlations in massive datasets, data mining is a technique that combines statistics with machine learning. Businesses can use this method to transform raw data into business intelligence, including current data insights and forecasts that help decision-making.
Data mining is sifting through redundant, noisy, unstructured data to find patterns that reveal insightful information. A form of data mining methodology called exploratory data analysis (EDA) includes examining datasets to identify and summarize their fundamental properties, frequently using visual techniques.
EDA focuses on objectively probing the facts without any expectations; it does not entail hypothesis testing or the deliberate search for a solution. On the other hand, traditional data mining focuses on extracting insights from the data or addressing a specific business problem.
Data Warehousing
Most extensive data mining projects start with data warehousing. An example of a data management system is a data warehouse created to facilitate and assist business intelligence initiatives. This is accomplished by centralizing and combining several data sources, including transactional data from POS (point of sale) systems and application log files.
A data warehouse typically includes a relational database for storing and retrieving data, an ETL (Extract, Transfer, Load) pipeline for preparing the data for analysis, statistical analysis tools, and client analysis tools for presenting the data to clients.
Clustering
One of the most often used data mining techniques is clustering, which divides a massive dataset into smaller subsets by categorizing objects based on their similarity into groups.
When consumers are grouped together based on shared purchasing patterns or lifetime value, customer segments are created, allowing the company to scale up targeted marketing campaigns.
Hard clustering entails the categorization of data points directly. Instead of assigning a data point to a cluster, soft clustering gives it a likelihood that it belongs in one or more clusters.
Classification
A prediction approach called classification involves estimating the likelihood that a given item falls into a particular category. A multiclass classification problem has more than two classes, unlike a binary classification problem, which only has two types.
Classification models produce a serial number, usually called confidence, that reflects the likelihood that an observation belongs to a specific class. The class with the highest probability can represent a predicted probability as a class label.
Spam filters, which categorize incoming emails as "spam" or "not spam" based on predetermined criteria, and fraud detection algorithms, which highlight suspicious transactions, are the most prevalent examples of categorization in a business use case.
Regression Model
When a company needs to forecast a numerical number, such as how long a potential customer will wait to cancel an airline reservation or how much money they will spend on auto payments over time, they can use a regression method.
For instance, linear regression is a popular regression technique that searches for a correlation between two variables. Regression algorithms of this type look for patterns that foretell correlations between variables, such as the association between consumer spending and the amount of time spent browsing an online store.
Neural Networks
Neural networks are data processing methods with biological influences that use historical and present data to forecast future values. They can uncover intricate relationships buried in the data because of their design, which mimics the brain's mechanisms for pattern recognition.
They have several layers that take input (input layer), calculate predictions (hidden layer), and provide output (output layer) in the form of a single prediction. They are frequently used for applications like image recognition and patient diagnostics.
Decision Trees
A decision tree is a graphic diagram that looks like an upside-down tree. Starting at the "roots," one walks through a continuously narrowing range of alternatives, each illustrating a possible decision conclusion. Decision trees may handle various categorization issues, but they can resolve many more complicated issues when used with predictive analytics.
An airline, for instance, would be interested in learning the optimal time to travel to a new location it intends to serve weekly. Along with knowing what pricing to charge for such a flight, it might also want to know which client groups to cater to. The airline can utilize a decision tree to acquire insight into the effects of selling tickets to destination x at price point y while focusing on audience z, given these criteria.
Logistics Regression
It is used when determining the likelihood of success in terms of Yes or No, Success or Failure. We can utilize this model when the dependent variable has a binary (Yes/No) nature.
Since it uses a non-linear log to predict the odds ratio, it may handle multiple relationships without requiring a linear link between the variables, unlike a linear model. Large sample sizes are also necessary to predict future results.
Ordinal logistic regression is used when the dependent variable's value is ordinal, and multinomial logistic regression is used when the dependent variable's value is multiclass.
Time Series Model
Based on past data, time series are used to forecast the future behavior of variables. Typically, a stochastic process called Y(t), which denotes a series of random variables, are used to model these models.
A time series might have the frequency of annual (annual budgets), quarterly (sales), monthly (expenses), or daily (daily expenses) (Stock Prices). It is referred to as univariate time series forecasting if you utilize the time series' past values to predict future discounts. It is also referred to as multivariate time series forecasting if you include exogenous variables.
The most popular time series model that can be created in Python is called ARIMA, or Auto Regressive Integrated Moving Average, to anticipate future results. It's a forecasting technique based on the straightforward notion that data from time series' initial values provides valuable information.
In Conclusion-
Although predictive analytics techniques have had their fair share of critiques, including the claim that computers or algorithms cannot foretell the future, predictive analytics is now extensively employed in virtually every industry. As we gather more and more data, we can anticipate future outcomes with a certain level of accuracy. This makes it possible for institutions and enterprises to make wise judgments.
Implementing Predictive Analytics is essential for anybody searching for company growth with data analytics services since it has several use cases in every conceivable industry. Contact us at SG Analytics if you want to take full advantage of predictive analytics for your business growth.
2 notes
·
View notes
Text
Implementing the Tableau to Power BI Migration: A Strategic Approach for Transition
migrating from Tableau to Power BI offers organizations an opportunity to unlock new levels of analytics performance, cost-efficiency, and integration within the Microsoft ecosystem. However, transitioning from one BI tool to another is a complex process that requires careful planning and execution. In this guide, we explore the essential steps and strategies for a successful Tableau to Power BI migration, ensuring smooth data model conversion, optimized performance, robust security implementation, and seamless user adoption. Whether you're looking to modernize your analytics environment or improve cost management, understanding the key components of this migration is crucial to achieving long-term success.
Understanding the Tableau to Power BI migration Landscape
When planning a Tableau to Power BI migration , organizations must first understand the fundamental differences between these platforms. The process requires careful consideration of various factors, including data architecture redesign and cross-platform analytics transition. A successful Tableau to Power BI migration starts with a thorough assessment of your current environment.
Strategic Planning and Assessment
The foundation of any successful Tableau to Power BI migration lies in comprehensive planning. Organizations must conduct a thorough migration assessment framework to understand their current Tableau implementation. This involves analyzing existing reports, dashboards, and data sources while documenting specific requirements for the transition.
Technical Implementation Framework
Data Architecture and Integration
The core of Tableau to Power BI migration involves data model conversion and proper database connection transfer. Organizations must implement effective data warehouse integration strategies while ensuring proper data gateway configuration. A successful Tableau to Power BI migration requires careful attention to ETL process migration and schema migration planning.
Development and Conversion Process
During the Tableau to Power BI migration, special attention must be paid to DAX formula conversion and LOD expression transformation. The process includes careful handling of calculated field migration and implementation of proper parameter configuration transfer. Organizations should establish a robust development environment setup to ensure smooth transitions.
Performance Optimization Strategy
A critical aspect of Tableau to Power BI migration involves implementing effective performance tuning methods. This includes establishing proper query performance optimization techniques and memory usage optimization strategies. Organizations must focus on resource allocation planning and workload distribution to maintain optimal performance.
Security Implementation
Security remains paramount during Tableau to Power BI migration. Organizations must ensure proper security model transfer and implement robust access control implementation. The process includes setting up row-level security migration and establishing comprehensive data protection protocols.
User Management and Training
Successful Tableau to Power BI migration requires careful attention to user access migration and license transfer process. Organizations must implement effective group policy implementation strategies while ensuring proper user mapping strategy execution. This includes developing comprehensive user training materials and establishing clear knowledge transfer plans.
Testing and Quality Assurance
Implementing thorough migration testing protocols ensures successful outcomes. Organizations must establish comprehensive validation framework setup procedures and implement proper quality assurance methods. This includes conducting thorough user acceptance testing and implementing effective dashboard testing strategy procedures.
Maintenance and Support Planning
Post-migration success requires implementing effective post-migration monitoring systems and establishing proper system health checks. Organizations must focus on performance analytics and implement comprehensive usage monitoring setup procedures to ensure continued success.
Ensuring Long-term Success and ROI
A successful Tableau to Power BI migration requires ongoing attention to maintenance and optimization. Organizations must establish proper maintenance scheduling procedures and implement effective backup procedures while ensuring comprehensive recovery planning.
Partner with DataTerrain for Migration Excellence
At DataTerrain, we understand the complexities of Tableau to Power BI migration. Our team of certified professionals brings extensive experience in managing complex migrations, ensuring seamless transitions while maintaining business continuity. We offer:
Comprehensive Migration Services: Our expert team handles every aspect of your migration journey, from initial assessment to post-migration support.
Technical Excellence: With deep expertise in both Tableau and Power BI, we ensure optimal implementation of all technical components.
Proven Methodology: Our structured approach, refined through numerous successful migrations, ensures predictable outcomes and minimal disruption.
Transform your business intelligence capabilities with DataTerrain's expert Tableau to Power BI migration services. Contact us today for a free consultation and discover how we can guide your organization through a successful migration journey.
0 notes
Text
Challenges and Solutions in Migrating from Firebird to PostgreSQL – Ask On Data
Migrating from one database management system (DBMS) to another can be a daunting task, especially when moving from a system like Firebird to PostgreSQL. While both are powerful, open-source relational databases, they have significant differences in architecture, functionality, and performance. The Firebird to PostgreSQL Migration process involves addressing several challenges that may arise, including data integrity, schema differences, and performance optimization. In this article, we will explore some common challenges in this migration and provide practical solutions to ensure a smooth transition.
1. Schema Differences and Compatibility Issues
One of the primary challenges when migrating from Firebird to PostgreSQL is the difference in schema structures and SQL syntax. Firebird uses a slightly different approach to handling data types, constraints, and indexes compared to PostgreSQL. For example, Firebird does not support some advanced PostgreSQL data types such as JSONB and ARRAY, which could complicate the migration process.
Solution: To overcome schema compatibility issues, start by thoroughly analysing the Firebird schema. Identify any Firebird-specific data types and operations, then map them to their PostgreSQL equivalents. You may need to rewrite certain parts of the schema, particularly for custom data types or stored procedures. There are also tools available that can help with this, such as pg_loader or DBConvert, which automate many of the mapping and conversion tasks.
2. Data Migration and Integrity
Migrating large volumes of data from Firebird to PostgreSQL can be another challenge. Ensuring data integrity and avoiding data loss during the migration process is crucial, especially if the database contains sensitive information or is in production use.
Solution: To preserve data integrity, a well-planned migration strategy is essential. Begin with a backup of the Firebird database before initiating any migration tasks. Then, consider using a phased migration approach, starting with less critical data to test the migration process before handling the main data sets. You can use ETL (Extract, Transform, Load) tools to facilitate data transfer while ensuring data types and constraints are properly mapped. Additionally, validating the migrated data through comprehensive testing is critical to confirm its accuracy and consistency.
3. Stored Procedures and Triggers
Firebird and PostgreSQL handle stored procedures and triggers differently. While Firebird uses its own dialect of SQL for creating stored procedures and triggers, PostgreSQL employs PL/pgSQL, which may require substantial changes in the logic and syntax of the existing procedures.
Solution: Manual conversion of stored procedures and triggers from Firebird to PostgreSQL is often necessary. Depending on the complexity, this could be a time-consuming process. It's advisable to map the logic of Firebird stored procedures to PostgreSQL's PL/pgSQL language, ensuring that any procedural or control flow statements are appropriately translated. If the application relies heavily on stored procedures, careful testing should be done to verify that the logic remains intact post-migration.
4. Performance Optimization
Performance optimization is a key concern when migrating databases. While PostgreSQL is known for its strong performance, tuning it to perform optimally for your workload after migration may require adjustments. Firebird and PostgreSQL have different query optimization engines, indexing methods, and transaction handling mechanisms, which can affect performance.
Solution: After migrating the schema and data, conduct a thorough performance analysis of the PostgreSQL instance. Use EXPLAIN ANALYZE and VACUUM to analyse query plans and identify any slow-performing queries. Indexing strategies in PostgreSQL may differ from Firebird, so ensure that indexes are appropriately created for optimal performance. Additionally, fine-tuning PostgreSQL’s configuration settings, such as memory allocation, query cache settings, and vacuum parameters, will help optimize the overall performance of the migrated database.
5. Application Compatibility
The final challenge to address during Firebird to PostgreSQL Migration is ensuring that the applications interacting with the database continue to function properly. The application layer may contain hardcoded SQL queries or assumptions based on Firebird’s behaviour, which might not work as expected with PostgreSQL.
Solution: After migrating the database, thoroughly test all application functionalities that interact with the database. Update any application queries or functions that rely on Firebird-specific features, and ensure they are compatible with PostgreSQL’s syntax and behaviour. Tools like pgAdmin and PostgreSQL JDBC drivers can help test and optimize the connection between the application and PostgreSQL.
Conclusion
Migrating from Firebird to PostgreSQL can be a complex yet rewarding process. By understanding the potential challenges with Ask On Data—such as schema differences, data integrity issues, and performance optimization—and implementing the appropriate solutions, you can ensure a successful migration. With careful planning, testing, and the use of migration tools, you can transition smoothly to PostgreSQL and take advantage of its powerful features and scalability.
0 notes
Text
Identify Data Quality Issues with ETL Testing
Mitigate the risk of poor data quality with QASource's ETL testing. We identify and address potential issues throughout the ETL process, preventing inaccurate or incomplete data from impacting your systems and reports. This proactive approach ensures you have clean and reliable data to drive informed decision-making.
0 notes
Text
Best DBT Course in Hyderabad | Data Build Tool Training
What is DBT, and Why is it Used in Data Engineering?
DBT, short for Data Build Tool, is an open-source command-line tool that allows data analysts and engineers to transform data in their warehouses using SQL. Unlike traditional ETL (Extract, Transform, Load) processes, which manage data transformations separately, DBT focuses solely on the Transform step and operates directly within the data warehouse.
DBT enables users to define models (SQL queries) that describe how raw data should be cleaned, joined, or transformed into analytics-ready datasets. It executes these models efficiently, tracks dependencies between them, and manages the transformation process within the data warehouse. DBT Training
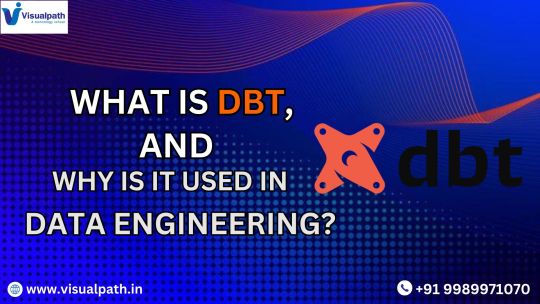
Key Features of DBT
SQL-Centric: DBT is built around SQL, making it accessible to data professionals who already have SQL expertise. No need for learning complex programming languages.
Version Control: DBT integrates seamlessly with version control systems like Git, allowing teams to collaborate effectively while maintaining an organized history of changes.
Testing and Validation: DBT provides built-in testing capabilities, enabling users to validate their data models with ease. Custom tests can also be defined to ensure data accuracy.
Documentation: With dbt, users can automatically generate documentation for their data models, providing transparency and fostering collaboration across teams.
Modularity: DBT encourages the use of modular SQL code, allowing users to break down complex transformations into manageable components that can be reused. DBT Classes Online
Why is DBT Used in Data Engineering?
DBT has become a critical tool in data engineering for several reasons:
1. Simplifies Data Transformation
Traditionally, the Transform step in ETL processes required specialized tools or complex scripts. DBT simplifies this by empowering data teams to write SQL-based transformations that run directly within their data warehouses. This eliminates the need for external tools and reduces complexity.
2. Works with Modern Data Warehouses
DBT is designed to integrate seamlessly with modern cloud-based data warehouses such as Snowflake, BigQuery, Redshift, and Databricks. By operating directly in the warehouse, it leverages the power and scalability of these platforms, ensuring fast and efficient transformations. DBT Certification Training Online
3. Encourages Collaboration and Transparency
With its integration with Git, dbt promotes collaboration among teams. Multiple team members can work on the same project, track changes, and ensure version control. The autogenerated documentation further enhances transparency by providing a clear view of the data pipeline.
4. Supports CI/CD Pipelines
DBT enables teams to adopt Continuous Integration/Continuous Deployment (CI/CD) workflows for data transformations. This ensures that changes to models are tested and validated before being deployed, reducing the risk of errors in production.
5. Focus on Analytics Engineering
DBT shifts the focus from traditional ETL to ELT (Extract, Load, Transform). With raw data already loaded into the warehouse, dbt allows teams to spend more time analyzing data rather than managing complex pipelines.
Real-World Use Cases
Data Cleaning and Enrichment: DBT is used to clean raw data, apply business logic, and create enriched datasets for analysis.
Building Data Models: Companies rely on dbt to create reusable, analytics-ready models that power dashboards and reports. DBT Online Training
Tracking Data Lineage: With its ability to visualize dependencies, dbt helps track the flow of data, ensuring transparency and accountability.
Conclusion
DBT has revolutionized the way data teams approach data transformations. By empowering analysts and engineers to use SQL for transformations, promoting collaboration, and leveraging the scalability of modern data warehouses, dbt has become a cornerstone of modern data engineering. Whether you are cleaning data, building data models, or ensuring data quality, dbt offers a robust and efficient solution that aligns with the needs of today’s data-driven organizations.
Visualpath is the Best Software Online Training Institute in Hyderabad. Avail complete Data Build Tool worldwide. You will get the best course at an affordable cost.
Attend Free Demo
Call on - +91-9989971070.
Visit: https://www.visualpath.in/online-data-build-tool-training.html
WhatsApp: https://www.whatsapp.com/catalog/919989971070/
Visit Blog: https://databuildtool1.blogspot.com/
#DBT Training#DBT Online Training#DBT Classes Online#DBT Training Courses#Best Online DBT Courses#DBT Certification Training Online#Data Build Tool Training in Hyderabad#Best DBT Course in Hyderabad#Data Build Tool Training in Ameerpet
0 notes
Text
Retrospective of 2024 - Long Time Archive
I would like to reflect on what I built last year through the lense of a software architect. This is the first installement in a series of architecture articles.
Last year, I had the opportunity to design and implement an archiving service to address GDPR requirements. In this article, I’ll share how we tackled this challenge, focusing on the architectural picture.
Introduction
This use case revolves around the Log Data Shipper, a core system component responsible for aggregating logs from multiple applications. These logs are stored in Elasticsearch, where they can be accessed and analyzed through an Angular web application. Users of the application (DPOs) can search the logs according to certain criteria, here is an example of such a search form:
The system then retrieves logs from ES so that the user can sift through them or export them if necessary, basically do what DPOs do.
As the volume of data grows over time, storing it all in Elasticsearch becomes increasingly expensive. To manage these costs, we needed a solution to archive logs older than two years without compromising the ability to retrieve necessary information later. This is where our archiving service comes into play.
Archiving Solution
Our approach involved creating buckets of data for logs older than two years and storing them on an S3 instance. Since the cost of storing compressed data is very low and the retention is very high, this was a cost-effective choice. To make retrieval efficient, we incorporated bloom filters into the design.
Bloom Filters
A bloom filter is a space-efficient probabilistic data structure used to test whether an element is part of a set. While it can produce false positives, it’s guaranteed not to produce false negatives, which made it an ideal choice for our needs.
During the archiving process, the ETL processes batches of logs and extracts essential data. For each type of data, we calculate a corresponding bloom filter - each search criteria in the form -. Both the archives and their associated bloom filters (a metadata field) are stored in S3.
When a user needs to retrieve data, the system tests each bloom filter against the search criteria. If a match is found, the corresponding archive is downloaded from S3. Although bloom filters might indicate the presence of data that isn’t actually in the archive (a false positive), this trade-off was acceptable for our use case as we are re-loading the logs in an temporary ES index. Finally we sort out all unecessary logs and the web application can read the logs in the same way current logs are consulted. We call this process "hydration".
Conclusion
This project was particularly interesting because it tackled an important topic – GDPR compliance – while allowing us to think outside the box by applying lesser-known data structures like bloom filters. Opportunities to solve challenging problems like this don’t come often, making the experience all the more rewarding.
It was also a great learning opportunity as I challenged the existing architecture, which required an additional index database. With the help of my team, we proposed an alternative solution that was both elegant and cost-effective. This project reinforced the importance of collaborative problem-solving and showed how innovative thinking can lead to efficient solutions.
0 notes
Text
How to Prepare for Data Analytics Interviews
Preparing for a data analytics interview can feel overwhelming, but with the right approach, you can ace it. Here are some practical tips to help you succeed:
1. Understand the Role
Read the job description carefully to know the skills and tools required.
Research the company’s industry, products, and services to tailor your responses.
2. Master the Fundamentals
Brush up on statistics, probability, and data visualization principles.
Be clear on concepts like correlation vs. causation, regression analysis, and hypothesis testing.
3. Get Hands-On with Tools
Practice using tools like Excel, SQL, Python, R, or Tableau.
Be ready to demonstrate your ability to manipulate and analyze data effectively.
4. Prepare for Technical Questions
Expect questions on SQL queries, data wrangling, and ETL processes.
Practice coding challenges on platforms like HackerRank or LeetCode.
5. Work on Business Acumen
Understand how data drives decision-making in businesses.
Practice interpreting data in the context of business goals and challenges.
6. Review Your Past Projects
Be prepared to discuss your past projects in detail.
Highlight the problem, your approach, tools used, and the impact of your analysis.
7. Focus on Soft Skills
Develop the ability to explain complex data insights to non-technical stakeholders.
Practice answering behavioral questions using the STAR method (Situation, Task, Action, Result).
8. Prepare for Case Studies
Practice solving case studies or real-world data problems.
Structure your response logically and explain your thought process.
9. Stay Updated
Keep up with industry trends, emerging tools, and best practices in data analytics.
Read blogs, attend webinars, or take short courses to stay current.
10. Mock Interviews
Participate in mock interviews with peers or mentors.
Get feedback to improve your responses and build confidence.
By focusing on these areas and practicing consistently, you’ll increase your chances of success in data analytics interviews. Good luck! 🚀
Would you like me to expand on any of these points?
#datascience#data analytics#python#data analysis#machinelearning#artificial intelligence#youtube#data science course#ai#digital marketing
0 notes
Text
Databricks Certified Data Engineer Professional Practice Exam For Best Preparation
Are you aspiring to become a certified data engineer with Databricks? Passing the Databricks Certified Data Engineer Professional exam is a significant step in proving your advanced data engineering skills. To simplify your preparation, the latest Databricks Certified Data Engineer Professional Practice Exam from Cert007 is an invaluable resource. Designed to mimic the real exam, it provides comprehensive practice questions that will help you master the topics and build confidence. With Cert007’s reliable preparation material, you can approach the exam with ease and increase your chances of success.
Overview of the Databricks Certified Data Engineer Professional Exam
The Databricks Certified Data Engineer Professional exam evaluates your ability to leverage the Databricks platform for advanced data engineering tasks. You will be tested on a range of skills, including:
Utilizing Apache Spark, Delta Lake, and MLflow to manage and process large datasets.
Building and optimizing ETL pipelines.
Applying data modeling principles to structure data in a Lakehouse architecture.
Using developer tools such as the Databricks CLI and REST API.
Ensuring data pipeline security, reliability, and performance through monitoring, testing, and governance.
Successful candidates will demonstrate a solid understanding of Databricks tools and the capability to design secure, efficient, and robust pipelines for data engineering.
Exam Details
Number of Questions: 60 multiple-choice questions
Duration: 120 minutes
Cost: $200 per attempt
Primary Coding Language: Python (Delta Lake functionality references are in SQL)
Certification Validity: 2 years from the date of passing
Exam Objectives and Weightage
The exam content is divided into six key objectives:
Databricks Tooling (20%) Proficiency in Databricks developer tools, including the CLI, REST API, and notebooks.
Data Processing (30%) Deep understanding of data transformation, optimization, and real-time streaming tasks using Databricks.
Data Modeling (20%) Knowledge of structuring data effectively for analysis and reporting in a Lakehouse architecture.
Security and Governance (10%) Implementation of secure practices for managing data access, encryption, and auditing.
Monitoring and Logging (10%) Ability to use tools and techniques to monitor pipeline performance and troubleshoot issues.
Testing and Deployment (10%) Knowledge of building, testing, and deploying reliable data engineering solutions.
Preparation Tips for Databricks Certified Data Engineer Professional Exam
1. Leverage Cert007 Practice Exams
The Databricks Certified Data Engineer Professional Practice Exam by Cert007 is tailored to provide a hands-on simulation of the real exam. Practicing with these questions will sharpen your understanding of the key concepts and help you identify areas where additional study is needed.
2. Understand the Databricks Ecosystem
Develop a strong understanding of the core components of the Databricks platform, including Apache Spark, Delta Lake, and MLflow. Focus on how these tools integrate to create seamless data engineering workflows.
3. Study the Official Databricks Learning Pathway
Follow the official Data Engineer learning pathway provided by Databricks. This pathway offers structured courses and materials designed to prepare candidates for the certification exam.
4. Hands-On Practice
Set up your own Databricks environment and practice creating ETL pipelines, managing data in Delta Lake, and deploying models with MLflow. This hands-on experience will enhance your skills and reinforce theoretical knowledge.
5. Review Security and Governance Best Practices
Pay attention to secure data practices, including access control, encryption, and compliance requirements. Understanding governance within the Databricks platform is essential for this exam.
6. Time Management for the Exam
Since you’ll have 120 minutes to answer 60 questions, practice pacing yourself during the exam. Aim to spend no more than 2 minutes per question, leaving time to review your answers.
Conclusion
Becoming a Databricks Certified Data Engineer Professional validates your expertise in advanced data engineering using the Databricks platform. By leveraging high-quality resources like the Cert007 practice exams and committing to hands-on practice, you can confidently approach the exam and achieve certification. Remember to stay consistent with your preparation and focus on mastering the six key objectives to ensure your success.
Good luck on your journey to becoming a certified data engineering professional!
0 notes
Text
Best Practices for a Smooth Data Warehouse Migration to Amazon Redshift
In the era of big data, many organizations find themselves outgrowing traditional on-premise data warehouses. Moving to a scalable, cloud-based solution like Amazon Redshift is an attractive solution for companies looking to improve performance, cut costs, and gain flexibility in their data operations. However, data warehouse migration to AWS, particularly to Amazon Redshift, can be complex, involving careful planning and precise execution to ensure a smooth transition. In this article, we’ll explore best practices for a seamless Redshift migration, covering essential steps from planning to optimization.
1. Establish Clear Objectives for Migration
Before diving into the technical process, it’s essential to define clear objectives for your data warehouse migration to AWS. Are you primarily looking to improve performance, reduce operational costs, or increase scalability? Understanding the ‘why’ behind your migration will help guide the entire process, from the tools you select to the migration approach.
For instance, if your main goal is to reduce costs, you’ll want to explore Amazon Redshift’s pay-as-you-go model or even Reserved Instances for predictable workloads. On the other hand, if performance is your focus, configuring the right nodes and optimizing queries will become a priority.
2. Assess and Prepare Your Data
Data assessment is a critical step in ensuring that your Redshift data warehouse can support your needs post-migration. Start by categorizing your data to determine what should be migrated and what can be archived or discarded. AWS provides tools like the AWS Schema Conversion Tool (SCT), which helps assess and convert your existing data schema for compatibility with Amazon Redshift.
For structured data that fits into Redshift’s SQL-based architecture, SCT can automatically convert schema from various sources, including Oracle and SQL Server, into a Redshift-compatible format. However, data with more complex structures might require custom ETL (Extract, Transform, Load) processes to maintain data integrity.
3. Choose the Right Migration Strategy
Amazon Redshift offers several migration strategies, each suited to different scenarios:
Lift and Shift: This approach involves migrating your data with minimal adjustments. It’s quick but may require optimization post-migration to achieve the best performance.
Re-architecting for Redshift: This strategy involves redesigning data models to leverage Redshift’s capabilities, such as columnar storage and distribution keys. Although more complex, it ensures optimal performance and scalability.
Hybrid Migration: In some cases, you may choose to keep certain workloads on-premises while migrating only specific data to Redshift. This strategy can help reduce risk and maintain critical workloads while testing Redshift’s performance.
Each strategy has its pros and cons, and selecting the best one depends on your unique business needs and resources. For a fast-tracked, low-cost migration, lift-and-shift works well, while those seeking high-performance gains should consider re-architecting.
4. Leverage Amazon’s Native Tools
Amazon Redshift provides a suite of tools that streamline and enhance the migration process:
AWS Database Migration Service (DMS): This service facilitates seamless data migration by enabling continuous data replication with minimal downtime. It’s particularly helpful for organizations that need to keep their data warehouse running during migration.
AWS Glue: Glue is a serverless data integration service that can help you prepare, transform, and load data into Redshift. It’s particularly valuable when dealing with unstructured or semi-structured data that needs to be transformed before migrating.
Using these tools allows for a smoother, more efficient migration while reducing the risk of data inconsistencies and downtime.
5. Optimize for Performance on Amazon Redshift
Once the migration is complete, it’s essential to take advantage of Redshift’s optimization features:
Use Sort and Distribution Keys: Redshift relies on distribution keys to define how data is stored across nodes. Selecting the right key can significantly improve query performance. Sort keys, on the other hand, help speed up query execution by reducing disk I/O.
Analyze and Tune Queries: Post-migration, analyze your queries to identify potential bottlenecks. Redshift’s query optimizer can help tune performance based on your specific workloads, reducing processing time for complex queries.
Compression and Encoding: Amazon Redshift offers automatic compression, reducing the size of your data and enhancing performance. Using columnar storage, Redshift efficiently compresses data, so be sure to implement optimal compression settings to save storage costs and boost query speed.
6. Plan for Security and Compliance
Data security and regulatory compliance are top priorities when migrating sensitive data to the cloud. Amazon Redshift includes various security features such as:
Data Encryption: Use encryption options, including encryption at rest using AWS Key Management Service (KMS) and encryption in transit with SSL, to protect your data during migration and beyond.
Access Control: Amazon Redshift supports AWS Identity and Access Management (IAM) roles, allowing you to define user permissions precisely, ensuring that only authorized personnel can access sensitive data.
Audit Logging: Redshift’s logging features provide transparency and traceability, allowing you to monitor all actions taken on your data warehouse. This helps meet compliance requirements and secures sensitive information.
7. Monitor and Adjust Post-Migration
Once the migration is complete, establish a monitoring routine to track the performance and health of your Redshift data warehouse. Amazon Redshift offers built-in monitoring features through Amazon CloudWatch, which can alert you to anomalies and allow for quick adjustments.
Additionally, be prepared to make adjustments as you observe user patterns and workloads. Regularly review your queries, data loads, and performance metrics, fine-tuning configurations as needed to maintain optimal performance.
Final Thoughts: Migrating to Amazon Redshift with Confidence
Migrating your data warehouse to Amazon Redshift can bring substantial advantages, but it requires careful planning, robust tools, and continuous optimization to unlock its full potential. By defining clear objectives, preparing your data, selecting the right migration strategy, and optimizing for performance, you can ensure a seamless transition to Redshift. Leveraging Amazon’s suite of tools and Redshift’s powerful features will empower your team to harness the full potential of a cloud-based data warehouse, boosting scalability, performance, and cost-efficiency.
Whether your goal is improved analytics or lower operating costs, following these best practices will help you make the most of your Amazon Redshift data warehouse, enabling your organization to thrive in a data-driven world.
#data warehouse migration to aws#redshift data warehouse#amazon redshift data warehouse#redshift migration#data warehouse to aws migration#data warehouse#aws migration
0 notes
Text
In today's rapidly evolving digital landscape, we're witnessing a significant shift in how organizations approach data projects. As a solution architect, I've observed a growing trend: the integration of DevOps practices with Business Intelligence (BI) is quickly becoming a top priority, superseding traditional siloed data projects. Let's explore why this convergence is essential for modern solutions.
The Limitations of Siloed Data Projects
Traditionally, data teams operated in isolation, focusing solely on data collection, analysis, and reporting. While this approach had its merits, it also presented several challenges:
1. Slow time-to-insight
2. Limited scalability
3. Difficulty in adapting to changing business requirements
4. Inconsistent data across departments
5. Lack of continuous improvement processes
The DevOps and BI Synergy
By bringing DevOps principles into the BI world, we're addressing these challenges head-on. Here's why this integration is crucial from a solution architecture standpoint:
1. Agile Data Pipelines: DevOps practices enable us to build flexible, automated data pipelines that can quickly adapt to new data sources or changing business needs. This flexibility is essential in today's rapidly changing business landscape.
2. Continuous Integration and Delivery of Insights: With CI/CD practices applied to BI, we can ensure that new data models, reports, and dashboards are tested, validated, and deployed rapidly and reliably.
3. Infrastructure as Code: Treating data infrastructure as code allows for version control, easy replication of environments, and quick scaling of BI systems as data volumes grow.
4. Automated Testing and Quality Assurance: Implementing automated testing for data processes, ETL jobs, and reports significantly improves data quality and reliability of insights.
5. Monitoring and Observability: DevOps principles help in setting up comprehensive monitoring for BI systems, ensuring performance, detecting anomalies, and facilitating quick troubleshooting.
6. Collaboration and Knowledge Sharing: Breaking down silos between data scientists, analysts, and IT ops teams fosters innovation and ensures that BI solutions are both powerful and practical.
Architectural Considerations
When designing solutions that integrate DevOps and BI, consider the following:
1. Modular Architecture: Design your BI system with loosely coupled components that can be independently developed, tested, and deployed.
2. API-First Approach: Implement APIs for data access and integration to enable flexibility and interoperability.
3. Containerization: Use container technologies like Docker to ensure consistency across development, testing, and production environments.
4. Orchestration: Employ orchestration tools like Kubernetes to manage and scale your BI infrastructure efficiently.
5. Version Control: Implement version control for data models, ETL processes, and dashboards, not just for code.
6. Automated Data Governance: Integrate data governance checks into your CI/CD pipeline to ensure compliance and data quality.
Overcoming Challenges
While the benefits are clear, implementing DevOps in BI is not without challenges:
1. Skill Gap: Teams need to develop new competencies spanning both DevOps and BI domains.
2. Cultural Shift: Encouraging collaboration between traditionally separate teams can be difficult.
3. Tool Integration: Ensuring seamless integration between DevOps tools and BI platforms requires careful planning.
4. Data Security: With increased automation and data flow, robust security measures become even more critical.
Conclusion
As solution architects, our role is to design systems that not only meet current needs but are also adaptable to future requirements. The integration of DevOps and BI is no longer just a nice-to-have – it's becoming essential for organizations that want to remain competitive in a data-driven world.
By embracing this convergence, we can create BI solutions that are more agile, reliable, and capable of delivering timely insights. This approach not only improves the technical aspects of data management but also aligns more closely with business objectives, enabling organizations to make data-driven decisions faster and more effectively.
The future of BI lies in breaking down silos, automating processes, and fostering a culture of continuous improvement. As solution architects, it's our responsibility to lead this transformation and help our organizations harness the full potential of their data assets.
Contact Us For More Details Or Email Us @ [email protected]
#xequalto#DevOps#BusinessIntelligence#SolutionArchitect#DataIntegration#AgileData#CI_CD#DataPipelines#InfrastructureAsCode#DataTesting#DataMonitoring#DataGovernance#DataSecurity#DataCulture#DigitalTransformation#DataDriven#ContinuousImprovement#TechInnovation#DataAnalytics#DataStrategy#customerbehavior#businessgrowth#inventorymanagement#datascience#machinelearning#datadrivendecisionmaking#customerinsights
0 notes
Text
How should I start learning Python?
Good Choice! Python is a fabulous language for Data Science, since it is very readable, versatile, and features a great many libraries.
1. Mastering the Basics of Python
First of all, learn the basics: one needs to study Variables, Data Types — numbers, strings, lists, dictionaries, Operators, Control Flow — if-else, loops, functions
Practice consistently: Learning to code is like learning a language. One has to keep practicing.
Online Resources: One can study through online platforms like Codecademy, Coursera, Lejhro, or watch YouTube Tutorials to learn in a structured format.
2. Dive into Data Structures and Algorithms
Master data structures: Know in detail about lists, tuples, sets, and dictionaries.
Understand algorithms: Know about sorting, searching, and other basic algorithms.
Problem solving: Practice problems or coding challenges on LeetCode or HackerRank.
3. Explore Data Analysis Libraries
NumPy: Introduce yourself to array manipulation, mathematical operations on arrays, and random number generation.
Pandas: Learn data manipulation, cleaning, analysis of DataFrames.
Matplotlib: Visualize your data elegantly with a variety of plot types.
Seaborn: Beautiful visualizations with a high-level interface.
4. Dive into Machine Learning
Scikit-learn: The study of supervised and unsupervised learning algorithms.
How to evaluate a model: metrics, cross-validation, hyperparameter tuning.
Practice on datasets: Solve real-world problems and build up your portfolio.
5. Deep Dive into Data Science
Statistics: probability theory, distributions, hypothesis testing, regression
Big data tools: Be familiar with PySpark for large datasets.
Data Engineering: Data pipelines, ETL processes, cloud platforms
Additional Tips
Join online communities: Participate in forums, discussions, and projects to learn from others.
Build projects: Apply the skill by making a data science project of your own.
Keep learning: The field is very dynamic; hence, keep updating your skills.
Remember
Start small: Break down complex topics into smaller, manageable chunks.
Practice consistently: To get good at coding, one needs to code regularly.
Don't be afraid to experiment: Try different approaches, learn from failures.
Look into leveraging some of the free and paid-for online resources that are available.
0 notes
Text
Top 5 Agriculture Testing Labs in Abu Dhabi: What Sets Them Apart?
In Abu Dhabi, agriculture testing labs are crucial for ensuring soil health, water quality, and overall crop productivity. As the agricultural sector in the UAE continues to evolve, selecting the right lab can significantly impact farming outcomes. This blog highlights the top five agriculture testing lab in Abu Dhabi and what sets them apart, providing insights into their unique services and strengths.
1. Dubai Central Laboratory (DCL)
Overview: The Dubai Central Laboratory (DCL) is one of the leading testing facilities in the UAE, offering a comprehensive range of agriculture testing services. With a focus on quality and accuracy, DCL is renowned for its state-of-the-art technology and expert staff.
Key Features:
Accreditation: DCL is accredited by the Emirates Authority for Standardization and Metrology (ESMA), ensuring adherence to international standards.
Services: Offers soil, water, and plant tissue analysis, with advanced testing methods for accurate results.
Technology: Utilizes the latest technology for precise testing and analysis, including high-performance liquid chromatography (HPLC) and gas chromatography (GC).
What Sets Them Apart: DCL’s commitment to quality and innovation makes it a top choice for farmers seeking reliable testing services. Their comprehensive approach and use of cutting-edge technology ensure accurate and actionable results.
2. Agricultural and Environmental Testing Laboratory (AETL)
Overview: Agricultural and Environmental Testing Laboratory (AETL) specializes in both agricultural and environmental testing. Known for its expertise in sustainable practices, AETL supports farmers in optimizing their operations while maintaining environmental responsibility.
Key Features:
Specialization: Focuses on soil health, water quality, and environmental impact assessments.
Sustainability: Provides recommendations for sustainable farming practices and resource management.
Certifications: Accredited by multiple international and local bodies, ensuring high standards of testing and analysis.
What Sets Them Apart: AETL’s emphasis on sustainability and environmental impact sets it apart. Their expertise in integrating agricultural testing with environmental considerations helps farmers adopt practices that are both productive and eco-friendly.
3. Al Barari Lab
Overview: Al Barari Lab is known for its specialized services tailored to urban and high-tech farming environments. Their focus on innovation makes them a key player in Abu Dhabi’s agricultural testing sector.
Key Features:
Urban Farming Focus: Specializes in testing for urban and vertical farming systems, addressing the unique challenges of these environments.
Advanced Techniques: Employs modern testing techniques and equipment, including nutrient management solutions and hydroponic system analysis.
Consultation Services: Offers expert advice and solutions for optimizing urban farming practices.
What Sets Them Apart: Al Barari Lab’s specialization in urban and high-tech farming sets it apart. Their advanced techniques and focus on innovative farming solutions cater to the growing trend of urban agriculture in Abu Dhabi.
4. Emirates Testing Laboratory (ETL)
Overview: Emirates Testing Laboratory (ETL) is a well-established testing facility known for its comprehensive range of services and commitment to quality. ETL provides a wide array of testing services for various agricultural needs.
Key Features:
Diverse Services: Offers soil, water, and plant tissue testing, as well as pesticide residue analysis.
Experienced Team: Features a team of experienced professionals with extensive knowledge in agriculture testing.
Quality Assurance: Adheres to rigorous quality assurance protocols to ensure reliable and accurate results.
What Sets Them Apart: ETL’s extensive experience and diverse service offerings make it a versatile choice for farmers. Their commitment to quality and reliability ensures that farmers receive precise and actionable insights.
5. METS Laboratories
Overview: METS Laboratories is recognized for its innovative approach to agriculture testing. With a focus on cutting-edge technology and customer service, METS provides comprehensive testing solutions for modern farming needs.
Key Features:
Technology: Utilizes advanced technology, including spectroscopy and mass spectrometry, for detailed and accurate analysis.
Customer Service: Offers exceptional customer support, including consultation and data interpretation services.
Research and Development: Invests in research and development to stay at the forefront of agricultural testing advancements.
What Sets Them Apart: METS Laboratories’ commitment to innovation and customer service distinguishes it from other labs. Their investment in technology and focus on providing tailored solutions ensures that farmers receive the most accurate and useful information.
Comparing the Top Labs
When choosing an agriculture testing lab in Abu Dhabi, consider the following factors:
Accreditation and Certifications: Ensure the lab is accredited by recognized bodies like ESMA to guarantee quality and reliability.
Specialization: Some labs focus on specific areas, such as urban farming or sustainability. Choose a lab that aligns with your specific needs.
Technology and Techniques: Advanced technology can provide more accurate and detailed results. Look for labs that invest in modern equipment and techniques.
Customer Service: Effective communication and support are crucial for interpreting results and implementing recommendations.
Conclusion
Selecting the right agriculture testing lab in Abu Dhabi is essential for optimizing your farming practices and ensuring high-quality outcomes. The top five labs highlighted—Dubai Central Laboratory (DCL), Agricultural and Environmental Testing Laboratory (AETL), Al Barari Lab, Emirates Testing Laboratory (ETL), and METS Laboratories—each offer unique strengths and services. By understanding what sets each lab apart, you can make an informed decision that supports your agricultural goals and contributes to the success of your farming operations.
0 notes
Text
Empowering Your Future with a Data Analytics Course in Rohini

In an era where data is often hailed as the new oil, the ability to analyze and interpret this resource has become crucial across all industries. Whether it’s for making informed business decisions, optimizing operations, or understanding customer behavior, data analytics plays a pivotal role. The demand for skilled data analysts is soaring, making it an opportune time to enter this exciting field. If you’re based in Rohini, Delhi, or nearby, a comprehensive Data Analytics course offered at H-34/1, next to Ayodhya Chowk, Sector 3, Rohini, Delhi, 110085 could be your gateway to a successful career in data analytics.
The Role of Data Analytics in Today’s World
Data Analytics Course in Rohini involves the process of examining datasets to uncover hidden patterns, correlations, and insights. These insights can help organizations across sectors make strategic decisions, predict future trends, and gain a competitive edge. The ability to derive actionable insights from data has led to a growing need for data professionals who can efficiently analyze and interpret complex datasets.
Some key applications of data analytics include:
Business Strategy: Companies leverage data analytics to devise strategic plans that align with market trends and consumer demands.
Customer Insights: Understanding customer behavior and preferences to tailor products and services.
Operational Efficiency: Streamlining operations by identifying inefficiencies and optimizing processes.
Risk Management: Anticipating risks and taking proactive measures to mitigate them.
Product Development: Innovating new products based on data-driven market research.
Overview of the Data Analytics Course in Rohini
The Data Analytics Course offered at H-34/1, next to Ayodhya Chowk, Sector 3, Rohini, Delhi, 110085 is designed to cater to both beginners and professionals looking to enhance their skills. The course covers a wide range of topics, from foundational concepts to advanced analytical techniques, providing a well-rounded education in data analytics.
Course Structure:
Introduction to Data Analytics:
Overview of data analytics and its significance in various industries.
Types of data: structured, unstructured, and semi-structured.
Data collection methods and ensuring data quality.
Introduction to key data analytics tools and software.
Statistical Analysis:
Basics of statistics relevant to data analysis.
Descriptive statistics, including measures of central tendency and variability.
Inferential statistics, including hypothesis testing, confidence intervals, and p-values.
Correlation, regression analysis, and analysis of variance (ANOVA).
Data Management and SQL:
Fundamentals of databases and data management systems.
Data modeling, normalization, and database design principles.
SQL for data querying, manipulation, and reporting.
Introduction to data warehousing and ETL (Extract, Transform, Load) processes.
Advanced Analytics Techniques:
Exploratory data analysis (EDA) and data mining.
Introduction to machine learning algorithms for predictive analytics.
Time series analysis and forecasting methods.
Big data analytics with tools like Hadoop and Spark.
Data Visualization:
Importance of data visualization in conveying insights.
Hands-on experience with tools like Tableau, Power BI, and Python libraries (Matplotlib, Seaborn).
Creating interactive dashboards and visual reports to present data findings effectively.
Capstone Project:
Application of all learned concepts in a comprehensive project.
Working with large datasets to solve real-world business problems.
Presenting findings through detailed reports and visual presentations.
Learning Approach and Methodology
The Data Analytics Course in Rohini follows a practical, hands-on approach to learning. This ensures that students not only understand theoretical concepts but also gain practical experience working with real-world data.
Instructor-Led Classes: The course is taught by experienced professionals who bring industry insights and practical knowledge to the classroom. These instructors provide personalized guidance, ensuring that each student grasps the concepts thoroughly.
Hands-On Workshops: Practical workshops are a key component of the course, where students work on real datasets to apply the techniques they’ve learned. These sessions are designed to reinforce theoretical learning and build confidence in using data analytics tools and techniques.
Project-Based Learning: The capstone project is a significant part of the course, allowing students to apply their knowledge to a real-world scenario. This project not only serves as a practical experience but also as a portfolio piece that can be showcased to potential employers.
Course Duration and Schedule
The Data Analytics Course in Rohini is designed to be flexible, accommodating the needs of different learners, whether they are full-time students or working professionals. The course typically spans 3 to 6 months, depending on the chosen batch format.
Batch Options:
Weekend Batches: Ideal for working professionals who want to learn without disrupting their work schedule.
Weekday Batches: Suitable for full-time students or those with more flexible schedules.
Fast-Track Batches: An accelerated option for those who wish to complete the course in a shorter time frame.
Each batch is kept small to ensure that students receive personalized attention and an interactive learning experience. The course schedule balances theoretical lessons with practical application, ensuring a holistic understanding of data analytics.
Career Opportunities After the Course
Upon completing the Data Analytics Course in Rohini, you will be equipped with the skills needed to pursue various roles in the field of data analytics. The demand for data professionals is growing across industries, and this course can open doors to numerous career opportunities.
Potential Career Roles:
Data Analyst: Responsible for analyzing data to identify trends and insights that inform business decisions.
Business Analyst: Focuses on using data to drive business strategy and improve operational efficiency.
Data Scientist: Applies advanced analytics techniques, including machine learning, to solve complex business problems.
Data Engineer: Designs and maintains the data infrastructure, ensuring that data is accessible and usable for analysis.
BI Analyst/Developer: Creates dashboards and reports that help organizations track performance and make data-driven decisions.
The skills gained from this course are highly transferable, allowing you to work in a variety of industries, including finance, healthcare, technology, retail, and more. Additionally, the course provides a solid foundation for further specialization in advanced analytics fields.
Why Choose the Data Analytics Course at H-34/1, Rohini?
Choosing the right course is crucial for your career development. The Data Analytics Course at H-34/1, next to Ayodhya Chowk, Sector 3, Rohini, Delhi, 110085 offers several advantages that make it an excellent choice for aspiring data professionals.
Comprehensive Curriculum: The course covers all essential topics, from the basics of data analytics to advanced techniques. This ensures that you gain a well-rounded education that prepares you for real-world challenges.
Experienced Instructors: The course is taught by professionals with extensive experience in the field of data analytics. Their industry knowledge and practical insights provide students with valuable learning experiences that go beyond textbooks.
Practical Learning: The course emphasizes hands-on learning, with numerous workshops and projects that allow students to apply what they’ve learned. This practical approach ensures that you are well-prepared to handle real-world data analytics tasks.
Flexible Scheduling: With various batch options available, the course can accommodate the schedules of both students and working professionals. Whether you prefer weekend classes or an accelerated course, you can find an option that suits your needs.
Placement Assistance: The training center offers placement assistance to help students secure job opportunities after completing the course. This includes resume building, interview preparation, and access to job listings in the field of data analytics.
How to Enroll
Enrolling in the Data Analytics Course in Rohini is simple. To get started, you can visit the training center at H-34/1, next to Ayodhya Chowk, Sector 3, Rohini, Delhi, 110085 or contact them at 98111-28610. The center’s staff will provide you with all the information you need, including course schedules, fees, and any prerequisites.
If you’re ready to take the next step in your career, don’t miss out on this opportunity to join one of the best Data Analytics courses in Rohini. With the knowledge and skills you gain from this course, you’ll be well on your way to becoming a successful data analytics professional.
Conclusion
In today’s data-driven world, the ability to analyze and interpret data is more important than ever. The Data Analytics Course at H-34/1, next to Ayodhya Chowk, Sector 3, Rohini, Delhi, 110085 offers a comprehensive, practical education that prepares you for a successful career in this growing field. Whether you’re a beginner or a professional looking to upgrade your skills, this course provides everything you need to excel in data analytics. Don’t wait—contact 98111-28610 today to learn more and start your journey towards a rewarding career in data analytics.
0 notes
Text
Firebird to Cassandra Migration – Ask On Data
Migrating from Firebird, a relational database, to Cassandra, a NoSQL database, is a significant shift that enables businesses to harness scalability and distributed computing. The process of Firebird to Cassandra Migration requires careful planning, schema redesign, and data transformation to ensure the transition is smooth and effective.
Why Migrate from Firebird to Cassandra?
Scalability: Firebird is designed for small to medium workloads, whereas Cassandra excels in handling large-scale distributed systems with high availability.
Flexibility: Cassandra’s schema-less structure allows for easier adjustments to evolving data requirements compared to Firebird’s fixed schema.
High Availability: Cassandra’s architecture provides fault tolerance and ensures continuous operation, making it ideal for applications requiring zero downtime.
Steps for Firebird to Cassandra Migration
1. Assessment and Planning
Start by analysing your Firebird database, including schema structure, relationships, and data types. Determine the equivalent Cassandra table structure, considering its denormalized data model. Identify key queries to design tables with optimal partitioning and clustering keys.
2. Schema Redesign
Since Cassandra does not support relational concepts like joins and foreign keys, redesign your schema for denormalized tables. For example, a normalized Orders and Customers relationship in Firebird may need to be combined into a single table in Cassandra to optimize read performance.
3. Data Transformation
Export data from Firebird using tools like fbexport or custom SQL queries. Convert the data into a format compatible with Cassandra, such as CSV. Map Firebird data types to Cassandra types; for instance, Firebird’s VARCHAR maps to Cassandra’s TEXT.
4. Data Loading
Use Cassandra’s COPY command or tools like cqlsh and ETL (Extract, Transform, Load) pipelines to load data. For large datasets, tools like Apache Spark can facilitate distributed processing for faster migration.
5. Testing and Validation
After loading the data, validate its accuracy by running sample queries on Cassandra and comparing results with Firebird. Test the application functionality to ensure that queries perform as expected in the new database.
6. Cutover and Monitoring
Once testing is complete, switch your application’s backend to Cassandra. Monitor the system for performance and consistency issues during the initial phase to address any anomalies promptly.
Challenges in Firebird to Cassandra Migration
Schema Mapping: Transitioning from a relational to a NoSQL schema requires a paradigm shift in data modeling.
Data Volume: Migrating large datasets can be time-intensive and requires robust tools to avoid errors.
Application Refactoring: Applications may need updates to adapt to Cassandra’s query language (CQL) and denormalized data model.
Why Choose Ask On Data for Migration?
Ask On Data simplifies the complex process of Firebird to Cassandra Migration with its advanced automation and robust features. Designed to handle intricate database transitions, Ask On Data ensures:
Data Integrity: Accurate data mapping and transformation to avoid inconsistencies.
Efficiency: Optimized migration workflows to minimize downtime.
Scalability: Support for large-scale migrations with distributed systems compatibility.
With Ask On Data, businesses can achieve seamless transitions from Firebird to Cassandra, unlocking the full potential of a scalable, high-performance database solution.
Conclusion
Migrating from Firebird to Cassandra is a transformative step for organizations aiming to scale their applications and achieve high availability. By following a structured approach and leveraging tools like Ask On Data, businesses can ensure a successful migration that empowers them to meet growing demands efficiently.
0 notes
Text
data analytics training in noida
Comprehensive Data Analytics Training in Noida at Softcrayons Pvt Ltd
In the era of big data and advanced analytics, the ability to analyze and interpret data effectively is crucial for driving business success and innovation. Softcrayons Pvt Ltd in Noida offers an extensive Data Analytics training program designed to equip individuals with the skills and knowledge required to excel in this rapidly evolving field. This blog post provides a detailed overview of the training program, highlighting its features, benefits, and the impact it can have on your career.
Introduction**
From improving customer experiences to optimizing supply chains, data-driven decisions can significantly enhance organizational efficiency and profitability. As companies increasingly rely on data to guide their strategies, the demand for skilled data analysts is growing. Softcrayons Pvt Ltd in Noida recognizes this need and offers a comprehensive training program designed to prepare individuals for a successful career in data analytics.
Program Overview
Curriculum and Course Structure**
The Data Analytics training program at Softcrayons Pvt Ltd is meticulously designed to provide a well-rounded education in data analytics. The curriculum is structured to cover a broad spectrum of topics, ensuring that participants gain both theoretical knowledge and practical
a. Introduction to Data Analytics:**
Overview of Data Analytics
Importance and Applications of Data Analytics
Key Concepts and Terminology
b. Data Preprocessing:**
Data Cleaning Techniques
Handling Missing Data
Data Transformation and Normalization c. Statistical Analysis:**
Descriptive Statistics
Inferential Statistics
Hypothesis Testing d. Data Visualization:**
Principles of Data Visualization
Tools and Techniques for Effective Visualization
Creating Dashboards and Reports e. Advanced Analytics:**
Predictive Analytics
Machine Learning Algorithms
Time Series Analysis f. Big Data Technologies:**
Introduction to Big Data
Hadoop and Spark
Data Warehousing and ETL Processes g. Hands-On Projects:**
Real-World Case Studies
Live Data Analysis Projects
Capstone Project
Learning Methodology**
Softcrayons Pvt Ltd employs a blended learning approach that combines theoretical instruction with practical application. The training program includes:
a. Interactive Lectures:**
Delivered by industry experts
Covering fundamental and advanced concepts
b. Hands-On Labs:**
Practical exercises using industry-standard tools
Real-world scenarios to apply learning
c. Group Discussions:**
Collaborative learning through group activities
Sharing insights and solving problems together
d. One-on-One Mentorship:**
Personalized guidance from experienced mentors
Tailored support for individual learning needs
e. Real-World Projects:**
Engaging in live projects to gain practical experience
Applying concepts to real business challenges
Tools and Technologies**
The training program emphasizes proficiency in essential tools and technologies used in data analytics. Participants will gain hands-on experience with:
a. Python:**
Libraries such as Pandas, NumPy, and Scikit-Learn
Data manipulation and machine learning
b. R:**
Statistical analysis and visualization
Packages like ggplot2 and dplyr
c. Tableau:**
Data visualization and dashboard creation
Interactive and dynamic reports
d. Hadoop and Spark:**
Big data processing and analysis
Distributed computing frameworks
e. SQL:**
Database querying and management
Data extraction and manipulation
Benefits of the Training Program**
Expert Instruction**
One of the key advantages of Softcrayons' Data Analytics training is the opportunity to learn from seasoned industry professionals. The instructors bring a wealth of experience and practical knowledge, providing valuable insights into current industry trends and best practices.
Practical Experience**
The training program emphasizes hands-on learning through live projects and case studies. This practical experience allows participants to apply theoretical concepts to real-world scenarios, enhancing their problem-solving skills and building confidence.
Industry-Relevant Skills**
Participants gain proficiency in industry-standard tools and technologies, ensuring they are well-prepared for the demands of the job market. The skills acquired through the program are directly applicable to a wide range of roles in data analytics.
Personalized Support**
The program includes one-on-one mentorship, offering personalized guidance and support. This ensures that participants receive tailored assistance to address their specific learning needs and career goals.
Career Advancement**
Completing the Data Analytics training program opens up numerous career opportunities. Graduates are well-equipped to pursue roles such as data analyst, business intelligence analyst, data scientist, and more. The program also includes career support services to help participants secure job placements.
Case Studies and Success Stories**
Case Study: Retail Industry**
A participant in the training program applied their knowledge to analyze customer data for a retail company. By identifying purchasing patterns and trends, they helped the company optimize its inventory management and improve customer targeting strategies.
Success Story: Career Transition**
Another participant used the skills gained from the training program to transition from a marketing role to a data analyst position. With the ability to analyze and interpret data, they successfully secured a new role at a leading tech company and have since advanced to a senior data scientist position.
Conclusion**
Softcrayons Pvt Ltd in Noida offers a highly detailed and practical Data Analytics training program that prepares individuals for a successful career in data science. With a well-rounded curriculum, expert instruction, and hands-on experience, participants gain the skills and confidence needed to excel in the field of data analytics.
Whether you're looking to start a new career or enhance your existing skills, Softcrayons provides the tools and support necessary to achieve your goals. Enroll in the Data Analytics training program today and take the next step toward a rewarding career in the world of data.
Feel free to adjust or add specific details as needed.
#data analytics course in noida#data analytics course#best data analytics institute in noida#best data analytics institute
0 notes