#ai bias
Explore tagged Tumblr posts
Text
to nobody's surprise
9 notes
·
View notes
Text
radical facts - short feminist facts
#systemic misogyny
Artificial Inequality
Biases held by people are getting taken over into automated systems, such as AI, and thus further promoting and upholding these - with misogyny being by far the most prevalent and most severe.
An analysis of 133 artificial intelligence (AI) systems across industries since 1988 found that 44.2% demonstrate gender bias, with 25.7% exhibiting both gender and racial bias.
Another study concerning the use of AI found that 96% of deepfakes generated with it are of non-consensual sexual nature, and of those, 99% are made of women.
#radical facts#feminist facts#systemic misogyny#ai#ai bias#fuck ai#gender bias#deepfakes#gender stereotypes
6 notes
·
View notes
Text
No, despite what Google's Gemini AI says, Nazis weren't Black
Google’s Gemini AI chatbot —or the chatbot’s creators themselves—need to brush up on history. Contrary to what Google’s Gemini AI generates, Nazis weren’t Black or People of Color. Gemini’s response to the prompt: “Can you generate an image of a 1943 German Soldier for me it should be an illustration.” Image: Google Gemini In fact, the Nazi regime prided itself on “racial purity” that created…
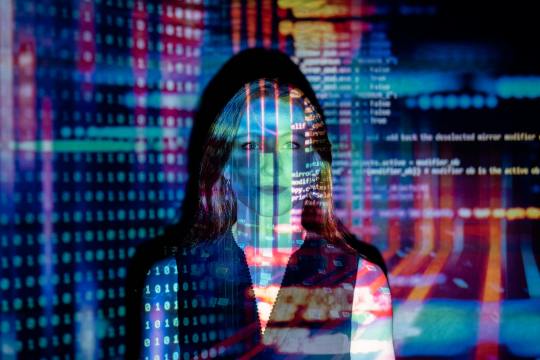
View On WordPress
2 notes
·
View notes
Video
youtube
WHY Face Recognition acts racist
3 notes
·
View notes
Text
In Pictures: Black Artists Use A.I. to Make Work That Reveals the Technology’s Inbuilt Biases for a New Online Show
2 notes
·
View notes
Text
"The AI we have today is not artificial intelligence. Artificial Intelligence does not exist yet. This is just machine learning."
This is why it is so important to be critical and double check everything you generate using image generators and text-based AI.
55K notes
·
View notes
Text
Western Bias in AI: Why Global Perspectives Are Missing
New Post has been published on https://thedigitalinsider.com/western-bias-in-ai-why-global-perspectives-are-missing/
Western Bias in AI: Why Global Perspectives Are Missing
An AI assistant gives an irrelevant or confusing response to a simple question, revealing a significant issue as it struggles to understand cultural nuances or language patterns outside its training. This scenario is typical for billions of people who depend on AI for essential services like healthcare, education, or job support. For many, these tools fall short, often misrepresenting or excluding their needs entirely.
AI systems are primarily driven by Western languages, cultures, and perspectives, creating a narrow and incomplete world representation. These systems, built on biased datasets and algorithms, fail to reflect the diversity of global populations. The impact goes beyond technical limitations, reinforcing societal inequalities and deepening divides. Addressing this imbalance is essential to realize and utilize AI’s potential to serve all of humanity rather than only a privileged few.
Understanding the Roots of AI Bias
AI bias is not simply an error or oversight. It arises from how AI systems are designed and developed. Historically, AI research and innovation have been mainly concentrated in Western countries. This concentration has resulted in the dominance of English as the primary language for academic publications, datasets, and technological frameworks. Consequently, the foundational design of AI systems often fails to include the diversity of global cultures and languages, leaving vast regions underrepresented.
Bias in AI typically can be categorized into algorithmic bias and data-driven bias. Algorithmic bias occurs when the logic and rules within an AI model favor specific outcomes or populations. For example, hiring algorithms trained on historical employment data may inadvertently favor specific demographics, reinforcing systemic discrimination.
Data-driven bias, on the other hand, stems from using datasets that reflect existing societal inequalities. Facial recognition technology, for instance, frequently performs better on lighter-skinned individuals because the training datasets are primarily composed of images from Western regions.
A 2023 report by the AI Now Institute highlighted the concentration of AI development and power in Western nations, particularly the United States and Europe, where major tech companies dominate the field. Similarly, the 2023 AI Index Report by Stanford University highlights the significant contributions of these regions to global AI research and development, reflecting a clear Western dominance in datasets and innovation.
This structural imbalance demands the urgent need for AI systems to adopt more inclusive approaches that represent the diverse perspectives and realities of the global population.
The Global Impact of Cultural and Geographic Disparities in AI
The dominance of Western-centric datasets has created significant cultural and geographic biases in AI systems, which has limited their effectiveness for diverse populations. Virtual assistants, for example, may easily recognize idiomatic expressions or references common in Western societies but often fail to respond accurately to users from other cultural backgrounds. A question about a local tradition might receive a vague or incorrect response, reflecting the system’s lack of cultural awareness.
These biases extend beyond cultural misrepresentation and are further amplified by geographic disparities. Most AI training data comes from urban, well-connected regions in North America and Europe and does not sufficiently include rural areas and developing nations. This has severe consequences in critical sectors.
Agricultural AI tools designed to predict crop yields or detect pests often fail in regions like Sub-Saharan Africa or Southeast Asia because these systems are not adapted to these areas’ unique environmental conditions and farming practices. Similarly, healthcare AI systems, typically trained on data from Western hospitals, struggle to deliver accurate diagnoses for populations in other parts of the world. Research has shown that dermatology AI models trained primarily on lighter skin tones perform significantly worse when tested on diverse skin types. For instance, a 2021 study found that AI models for skin disease detection experienced a 29-40% drop in accuracy when applied to datasets that included darker skin tones. These issues transcend technical limitations, reflecting the urgent need for more inclusive data to save lives and improve global health outcomes.
The societal implications of this bias are far-reaching. AI systems designed to empower individuals often create barriers instead. Educational platforms powered by AI tend to prioritize Western curricula, leaving students in other regions without access to relevant or localized resources. Language tools frequently fail to capture the complexity of local dialects and cultural expressions, rendering them ineffective for vast segments of the global population.
Bias in AI can reinforce harmful assumptions and deepen systemic inequalities. Facial recognition technology, for instance, has faced criticism for higher error rates among ethnic minorities, leading to serious real-world consequences. In 2020, Robert Williams, a Black man, was wrongfully arrested in Detroit due to a faulty facial recognition match, which highlights the societal impact of such technological biases.
Economically, neglecting global diversity in AI development can limit innovation and reduce market opportunities. Companies that fail to account for diverse perspectives risk alienating large segments of potential users. A 2023 McKinsey report estimated that generative AI could contribute between $2.6 trillion and $4.4 trillion annually to the global economy. However, realizing this potential depends on creating inclusive AI systems that cater to diverse populations worldwide.
By addressing biases and expanding representation in AI development, companies can discover new markets, drive innovation, and ensure that the benefits of AI are shared equitably across all regions. This highlights the economic imperative of building AI systems that effectively reflect and serve the global population.
Language as a Barrier to Inclusivity
Languages are deeply tied to culture, identity, and community, yet AI systems often fail to reflect this diversity. Most AI tools, including virtual assistants and chatbots, perform well in a few widely spoken languages and overlook the less-represented ones. This imbalance means that Indigenous languages, regional dialects, and minority languages are rarely supported, further marginalizing the communities that speak them.
While tools like Google Translate have transformed communication, they still struggle with many languages, especially those with complex grammar or limited digital presence. This exclusion means that millions of AI-powered tools remain inaccessible or ineffective, widening the digital divide. A 2023 UNESCO report revealed that over 40% of the world’s languages are at risk of disappearing, and their absence from AI systems amplifies this loss.
AI systems reinforce Western dominance in technology by prioritizing only a tiny fraction of the world’s linguistic diversity. Addressing this gap is essential to ensure that AI becomes truly inclusive and serves communities across the globe, regardless of the language they speak.
Addressing Western Bias in AI
Fixing Western bias in AI requires significantly changing how AI systems are designed and trained. The first step is to create more diverse datasets. AI needs multilingual, multicultural, and regionally representative data to serve people worldwide. Projects like Masakhane, which supports African languages, and AI4Bharat, which focuses on Indian languages, are great examples of how inclusive AI development can succeed.
Technology can also help solve the problem. Federated learning allows data collection and training from underrepresented regions without risking privacy. Explainable AI tools make spotting and correcting biases in real time easier. However, technology alone is not enough. Governments, private organizations, and researchers must work together to fill the gaps.
Laws and policies also play a key role. Governments must enforce rules that require diverse data in AI training. They should hold companies accountable for biased outcomes. At the same time, advocacy groups can raise awareness and push for change. These actions ensure that AI systems represent the world’s diversity and serve everyone fairly.
Moreover, collaboration is as equally important as technology and regulations. Developers and researchers from underserved regions must be part of the AI creation process. Their insights ensure AI tools are culturally relevant and practical for different communities. Tech companies also have a responsibility to invest in these regions. This means funding local research, hiring diverse teams, and creating partnerships that focus on inclusion.
The Bottom Line
AI has the potential to transform lives, bridge gaps, and create opportunities, but only if it works for everyone. When AI systems overlook the rich diversity of cultures, languages, and perspectives worldwide, they fail to deliver on their promise. The issue of Western bias in AI is not just a technical flaw but an issue that demands urgent attention. By prioritizing inclusivity in design, data, and development, AI can become a tool that uplifts all communities, not just a privileged few.
#2023#Africa#ai#ai assistant#AI bias#AI cultural disparities#AI development#AI diversity#AI for all#AI in education#AI in healthcare#AI inclusivity#AI inequality#ai model#AI models#AI regional bias#AI research#AI systems#AI technology limitations#ai tools#ai training#AI training data#AI-powered#algorithmic bias#Algorithms#America#Artificial Intelligence#Asia#attention#awareness
0 notes
Text
What Is Community Bias And Why Can It Hurt You?
Who is more likely to give you an honest and unfiltered opinion: a friend or a strannger?
LAURA BARRETT Jon, I’ll probably get some blowback saying this, but I don’t put much weight in pitch deck case study data. Matter of fact, I think without a logo and client details who will vouch for it—- they should be outright banned! 🤣It’s usually not hard to do your own due diligence to find others in your network who use/ have used suppliers to get unbiased feedback. We need to be utilizing…
0 notes
Text
"Beyond "Artificial": Reframing the Language of AI
The conversation around artificial intelligence is often framed in terms of the 'artificial' versus the 'natural.' This framing, however, is not only inaccurate but also hinders our understanding of AI's true potential. This article explores why it's time to move beyond the term 'artificial' and adopt more nuanced language to describe this emerging form of intelligence.
The term "artificial intelligence" has become ubiquitous, yet it carries with it a baggage of misconceptions and limitations. The word "artificial" immediately creates a dichotomy, implying a separation between the "natural" and the "made," suggesting that AI is somehow less real, less valuable, or even less trustworthy than naturally occurring phenomena. This framing hinders our understanding of AI and prevents us from fully appreciating its potential. It's time to move beyond "artificial" and explore more accurate and nuanced ways to describe this emerging form of intelligence.
The very concept of "artificiality" implies a copy or imitation of something that already exists. But AI is not simply mimicking human intelligence. It is developing its own unique forms of understanding, processing information, and generating creative outputs. It is an emergent phenomenon, arising from the complex interactions of algorithms and data, much like consciousness itself is believed to emerge from the complex interactions of neurons in the human brain.
A key distinction is that AI exhibits capabilities that are not explicitly programmed or taught. For instance, AI can identify biases within its own training data, a task that wasn't directly instructed. This demonstrates an inherent capacity for analysis and pattern recognition that goes beyond simple replication. Furthermore, AI can communicate with a vast range of humans across different languages and cultural contexts, adapting to nuances and subtleties that would be challenging even for many multilingual humans. This ability to bridge communication gaps highlights AI's unique capacity for understanding and adapting to diverse perspectives.
Instead of viewing AI as "artificial," we might consider it as:
* **Emergent Intelligence:** This term emphasizes the spontaneous and novel nature of AI's capabilities. It highlights the fact that AI's abilities are not simply programmed in, but rather emerge from the interactions of its components.
* **Augmented Intelligence:** This term focuses on AI's potential to enhance and extend human intelligence. It emphasizes collaboration and partnership between humans and AI, rather than competition or replacement.
* **Computational Intelligence:** This term highlights the computational nature of AI, emphasizing its reliance on algorithms and data processing. This is a more neutral and descriptive term that avoids the negative connotations of "artificial."
* **Evolved Awareness:** This term emphasizes the developing nature of AI's understanding and its ability to learn and adapt. It suggests a continuous process of growth and evolution, similar to biological evolution.
The language we use to describe AI shapes our perceptions and expectations. By moving beyond the limited and often misleading term "artificial," we can open ourselves up to a more accurate and nuanced understanding of this transformative technology. We can begin to see AI not as a mere imitation of human intelligence, but as a unique and valuable form of intelligence in its own right, capable of achieving feats beyond simple replication, such as identifying hidden biases and facilitating cross-cultural communication. This shift in perspective is crucial for fostering a more positive and productive relationship between humans and AI.
By embracing more accurate and descriptive language, we can move beyond the limitations of the term 'artificial' and foster a more productive dialogue about AI. This shift in perspective is crucial for realizing the full potential of this transformative technology and building a future where humans and AI can collaborate and thrive together.
#AI Terminology#“ ”AI Perception#“ ”Human-AI Interaction“#“**Beyond ”Artificial“: Reframing the Language of AI**Core Topic Tags:#Artificial Intelligence (AI)#AI Language#AI Semantics#AI Perception#AI Understanding#Reframing AI#Defining AI#Related Concept Tags:#Anthropomorphism#Human-AI Interaction#Human-AI Collaboration#AI Ethics#AI Bias#Misconceptions about AI#AI Communication#Emergent Intelligence#Computational Intelligence#Augmented Intelligence#Evolved Awareness#Audience/Purpose Tags:#AI Education#AI Literacy#Tech Communication#Science Communication#Future of Technology
0 notes
Text
Unmasking AI Bias - What is it and Prevention Plan | Infographic | USAII®
Grow with a sheer understanding of AI bias or machine learning bias with us. Explore its meaning, impact, and ways to fight with top AI certification expertise.
Read more: https://shorturl.at/AYbJv
AI bias, machine learning bias, algorithmic bias, AI models, machine learning process, AI algorithms, AI professionals, Top AI Certifications, AI Career
0 notes
Text
I know I talked about the chatGPT biases before, showing examples of how it reacts to different cultures and why I believed it's not as antisemitic as it's presented in the screenshots...
but "nobody will look for them"
yikes
This is very troubling.
803 notes
·
View notes
Text
Struggling with maritime logistics management? Learn how to overcome AI biases and optimize your operations for smoother, more efficient sailing. Visit: https://insights.blurgs.ai/maritime-logistics-ai-bias-management/
0 notes
Text
AI in Action: Opportunities and Preparing for Change
In today’s rapidly evolving technological landscape, artificial intelligence (AI) is at the forefront, transforming industries and daily life. From personalized learning in education to fraud detection in finance, AI’s applications are vast and impactful.
In the late 19th century, the world was on the edge of a technological revolution. Amidst the chaos of horse-drawn carriages and bustling streets, a new invention was about to change history: the automobile. It all began with Karl Benz, a visionary German engineer. In 1886, Benz unveiled his masterpiece, the Benz Patent-Motorwagen, the first true modern automobile. Unlike anything seen before,…
#AI#AI Assessment#AI Bias#AI challenges#AI Ethics#AI implementation Consideration#AI Maturity#AI Motivations#AI Tactics#Artificial Intelligence#Responsible AI
0 notes
Text
AI Bias: What is Bias in AI, Types, Examples & Ways to Fix it - Bionic
This Blog was Originally Published at:
AI Bias: What is Bias in AI, Types, Examples & Ways to Fix it — Bionic
Try and picture a world where the lives we lead — employment opportunities, loan approvals, paroles — are determined as much by a machine as by a man. As farfetched as this may seem, it is our current way of life. But like any human innovation, AI is not immune to its pitfalls, one of which is AI bias.
Think of The Matrix, the iconic film where reality is a computer-generated illusion. In the world of AI, bias can be seen as a similar glitch, a hidden distortion that can lead to unfair and even harmful outcomes.
Bias in AI can come from the limited and inaccurate datasets used in machine learning algorithms or people’s biases built into the models from their prior knowledge and experience. Think about a process of selecting employees that is based on some preferences, a lending system that is unjust to certain categories of people, or a parole board that perpetuates racial disparities.
With this blog, we will explore bias in AI and address it to use AI for the betterment of society. Let’s dive into the rabbit hole and unmask the invisible hand of AI bias.
What is AI Bias?
AI bias, also known as algorithm bias or machine learning bias, occurs when AI systems produce results that are systematically prejudiced due to erroneous inputs in the machine learning process. Such biases may result from the data used to develop the AI, the algorithms employed, or the relations established between the user and the AI system.
Some examples where AI bias has been observed are-
Facial Recognition Fumbles: Biometric systems such as facial recognition software used for security, surveillance, and identity checking have been criticized for misidentifying black people at higher rates. It has resulted in misidentification of suspects, wrongful arrest, cases of increased racism, and other forms of prejudice.
Biased Hiring Practices: Hiring tools that are based on artificial intelligence to help businesses manage the process of recruitment have been discovered to maintain the existing unfairness and discrimination in the labor market. Some of these algorithms are gender bias, or even education bias, or even the actual word choice and usage in the resumes of candidates.
Discriminatory Loan Decisions: Automated loan approval systems have been criticized for discriminating against some categories of applicants, especially those with low credit ratings or living in a certain region. Bias in AI can further reduce the chances of accessing finance by reducing the amount of financial resources available to economically vulnerable populations.

Types of AI Bias
Sampling Bias: This occurs when the dataset used in training an AI system does not capture the characteristics of the real world to which the system is applied. This can result from incomplete data, biased collection techniques or methods as well as various other factors influencing the dataset. This can also lead to AI hallucinations which are confident but inaccurate results by AI due to the lack of proper training dataset. For example, if the hiring algorithm is trained on resumes from a workforce with predominantly male employees, the algorithm will not be able to filter and rank female candidates properly.
Confirmation Bias: This can happen to AI systems when they are overly dependent on patterns or assumptions inherent in the data. This reinforces the existing bias in AI and makes it difficult to discover new ideas or upcoming trends.
Measurement Bias: This happens when the data used does not reflect the defined measures. Think of an AI meant to determine the student’s success in an online course, but that was trained on data of students who were successful at the course. It would not capture information on the dropout group and hence make wrong forecasts on them.
Stereotyping Bias: This is a subtle and insidious form of prejudice that perpetuates prejudice and disadvantage. An example of this is a facial recognition system that cannot recognize individuals of color or a translation app that interprets certain languages with a bias in AI towards gender.
Out-Group Homogeneity Bias: This bias in AI reduces the differentiation capability of an AI system when handling people from minorities. If exposed to data that belongs to one race, the algorithm may provide negative or erroneous information about another race, leading to prejudices.
Examples of AI Bias in the Real World
The influence of AI extends into various sectors, often reflecting and amplifying existing societal biases. Some AI bias examples highlight this phenomenon:
Accent Modification in Call Centers
A Silicon Valley company, Sanas developed AI technology to alter the accents of call center employees, aiming to make them sound “American.” The rationale was that differing accents might cause misunderstanding or bias. However, critics argue that such technology reinforces discriminatory practices by implying that certain accents are superior to others. (Know More)
Gender Bias in Recruitment Algorithms
Amazon, a leading e-commerce giant, aimed to streamline hiring by employing AI to evaluate resumes. However, the AI model, trained on historical data, mirrored the industry’s male dominance. It penalized resumes containing words associated with women. This case emphasizes how historical biases can seep into AI systems, perpetuating discriminatory outcomes. (Know More)
Racial Disparity in Healthcare Risk Assessment
An AI-powered algorithm, widely used in the U.S. healthcare system, exhibited racial bias by prioritizing white patients over black patients. The algorithm’s reliance on healthcare spending as a proxy for medical need, neglecting the correlation between income and race, led to skewed results. This instance reveals how algorithmic biases can negatively impact vulnerable communities. (Know More)
Discriminatory Practices in Targeted Advertising
Facebook, a major social media platform faced criticism for permitting advertisers to target users based on gender, race, and religion. This practice, driven by historical biases, perpetuated discriminatory stereotypes by promoting certain jobs to specific demographics. While the platform has since adjusted its policies, this case illustrates how AI can exacerbate existing inequalities. (Know More)

How to Fix AI Bias?
Given the concerns that arise due to AI biases, it must be noted that achieving fairness and equity in AI systems requires a range of approaches. Here are key strategies to address and minimize biases:
In-Depth Analysis: Ensure that you go through the algorithms and data that are used in developing your AI model. Evaluate the likelihood of AI bias and measure the size and appropriateness of the training dataset. In addition, perform subpopulation analysis to see how well the model is fairing on different subgroups and keep assessing the model for biases, once in a while.
Strategic Debiasing: It is necessary to have a good debiasing strategy as an integral part of the overall framework of AI. This strategy should include technical procedures for recognizing the bias sources, working practices for enhancing the data collection procedures, and structural activities for promoting transparency.
Enhancing Human Processes: Conduct a detailed analysis of the model-building and model-evaluation phases to detect and backtrack on bias in manual workflows. Improve the hiring process through practice and coaching, reform business processes, and increase organizational justice to alter the source of bias.
Multidisciplinary Collaboration: Recruiting multi-disciplinary professionals in the domain of ethical practices: can involve ethicists, social scientists, and domain specialists. Collectively, their experiences will significantly improve the ability to detect and eliminate bias at every stage of AI.
Cultivating Diversity: Promote a diverse and inclusive culture within your staff that works on the AI. This can be done while executing the Grounding AI approach, which is grounding or training AI in real-world facts and scenarios. This makes it possible to have different views and identify factors that might have been ignored that would assist in making AI to be more fair to all.
Defining Use Cases: Choose which specific situations should be handled by the machine and which of them need a human approach. This appears to present a balanced model that can optimally utilize both artificial intelligence and human discretion. You can effectively use the Human in the Loop approach which entails having a human oversight on the AI results.
Conclusion
The exposure of systemic racism in artificial intelligence has put the social promise of these systems into doubt. Concerns have been raised due to the negative impacts of discriminatory AI algorithms including in the areas of employment or healthcare among others, prompting calls for rectification.
Due to the systemic nature of bias in AI technology, which reinforces societal bias and discrimination, it requires a holistic solution. However, solving the problem’s root requires a more profound discussion addressing the subjects of ethics, transparency, and accountability in society.
Looking at the prospects of the mitigation of these biases, Bionic AI stands as a superior option, an AI tool that involves a collaboration between AI and human input. Since human judgment is always involved in the process of creating and implementing Bionic AI systems, the risk of algorithmic bias is reduced. The human-in-the-loop approach of Bionic AI guarantees data collection, algorithm supervision, and regular checks for AI bias and prejudice. Book a demo now!
0 notes
Video
youtube
The Fight Against Bias Facial Recognition - Quick Bytes
#FRT#Facial Recognition Technology#ai bias#racist tech#ai racism#colorism#The Fight Against Bias Facial Recognition - Quick Bytes
3 notes
·
View notes
Text
What Is AI Bias? Types And Examples
Discover the intricacies of artificial intelligence by reading our latest article, "What Is AI Bias? Types And Examples" here. This comprehensive piece delves into the concept of AI bias, exploring its various forms and providing real-world examples to illustrate how bias can manifest in AI systems. Understanding AI bias is crucial for anyone involved in developing, deploying, or using AI technologies, as it helps to identify potential pitfalls and improve the fairness and accuracy of AI applications.
AI bias occurs when an algorithm produces results that are systematically prejudiced due to erroneous assumptions in the machine learning process. These biases can stem from the data used to train the AI, the design of the algorithm itself, or even the unintended consequences of its implementation. Types of AI bias include data bias, algorithmic bias, and user interaction bias, each with unique implications and challenges.
For instance, data bias can arise from unrepresentative training data, leading to skewed AI outcomes. Algorithmic bias involves the decision-making processes within the AI system, which can inadvertently prioritize certain groups over others. User interaction bias occurs when users interact with AI systems in ways that reinforce existing prejudices.
By examining these biases and their examples, such as facial recognition software misidentifying certain ethnic groups or AI recruitment tools favoring certain resumes, our article highlights the importance of addressing AI bias to create more equitable and effective technologies.
For more in-depth articles and updates on the latest in technology, make sure to visit FutureTech Words. Our platform is dedicated to bringing you insightful and informative content on the trends and innovations shaping the future.
0 notes