#ahc yellow
Explore tagged Tumblr posts
Text
The full banner didn't quite fit, so here's the bigger version!
--Adelram
#ahc art#Agent Bishop#Cody Jones#Dark Turtles#tmnt 2003#teenage mutant ninja turtles#ahc red#ahc blue#ahc yellow#ahc purple#ahc cody#ahc bishop#ahc stockman
269 notes
·
View notes
Text
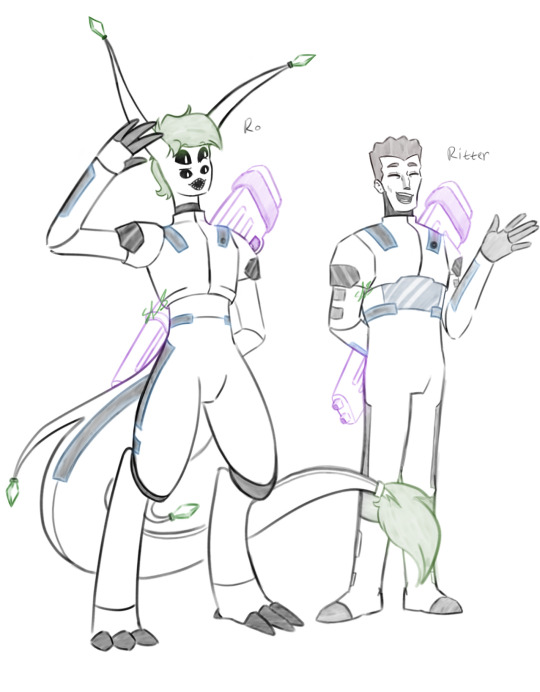

The cast of the AHC AU is growing bigger by the day!
Unsure if I ever posted these properly? So here’s two of our bodyguards (Ro and Ritter) from the first day at the new home, as well as the children and a third bodyguard (Hambone) on a shopping trip just a day later :) I’m particularly proud of the Dark Turtles, safe for Purple this is the first time I’ve drawn full-body drawings of them and they came out great :D also, BOY these turtles are massive, it keeps on surprising me.
Ritter and Hambone belong to my friend Ade aka VioletVulpini who’s co-writing AHC with me, Ro Glowborn is mine :)
#🌹 charlie’s art#🌹 AHC AU#🌹 OC art#🌹 other people’s OCs#tmnt dark leonardo#tmnt dark raphael#tmnt dark michelangelo#tmnt dark donatello#AHC Blue#AHC Red#AHC Yellow#AHC Purple#AHC Cody#this tagging system is gonna get messy.. ah well#🌹 Ro Glowborn
23 notes
·
View notes
Text
I ADORE YOUR CHARACTER DESIFN I ADORE LUIS. AND I LOVE HOW ECLECTIC HE IS I LOVE HOW IN THE YELLOW DRAWINF HES GIVING 1950S IN COUNTER BLENDER COMMERCIAL GOD I ADORE LUIS I ADORE HIM HES EVERYTHIGN HES NOTHINGHE IS THE DIMENSIONS DEMENTIA DOMICILE DOMINANT ALPHA MALE HES BETTER THAN GARFIELD HES BETTER THAN ANYTHITN ELSE IN THIS WORLD AHC MEIN GOTT IN HIMMEL NEVER CHNAGE

new guy just dropped

17 notes
·
View notes
Video
youtube
SATURNITY-SMILE 2 - AHC-DIUB FÔSTER (yellow)
1 note
·
View note
Photo

💛💛💛 . Phoenix Yellow CL9 💦💦 . 🚘 Owner @stxtic.cl9 📸 @nicole_vmsracing 📍 @acurahondaclassic . 👉 Registratiom is open for @dreamfest__ on November 13 at PBIR. Visit www.dream-fest.com for more info 📲 . 🔗 PHOTOS - VIDEOS - EVENTS 🔗 . ➡️ Check out full event videos on our YouTube channel. Link is in our bio 📲 . ➡️ Like us on Facebook at HondasAcurasOnly 👍 . 🔻 Follow @dreamfest__ 🔻 🔻 Follow @hondavseverybody 🔻 🔻 Follow @vmsracing 🔻 🔻 Follow @nyce.tv 🔻 🔻 Follow @wirewhere_mia 🔻 🔻 Follow @raceirra 🔻 🔻 Follow @beardedgaragistas 🔻 🔻 Follow @n1source_javi 🔻 🔻 Follow @hondajunkys 🔻 🔻 Follow @hondalifestyle.tm 🔻 🔻 Follow @activaoooracing_official 🔻 🔻 Follow @elite_garage 🔻 🔻 Follow @whowantsthesaucee🔻 🔻 Follow @house_of_hoopties 🔻 🔻 Follow @honda_religion 🔻 🔻 Follow @the_house_of_honda 🔻 🔻 Follow @vtec_kings 🔻 🔻 Follow @_mass.productions_ 🔻 🔻 Follow @_qualityproductions_ 🔻 . #honda #acura #acuratsx #tsx #tsxgang #cl9 #hondalove #hondanation #hondaporn #hondapower #hondagram #hondalife #hondalifestyle #hondadaily #hondagang #hondaculture #vtec #vtecclub #vtecnation #vtectuning #ahc #haonly__ (at Acura of Pembroke Pines) https://www.instagram.com/p/CV0-9JSvxX7/?utm_medium=tumblr
#honda#acura#acuratsx#tsx#tsxgang#cl9#hondalove#hondanation#hondaporn#hondapower#hondagram#hondalife#hondalifestyle#hondadaily#hondagang#hondaculture#vtec#vtecclub#vtecnation#vtectuning#ahc#haonly__
4 notes
·
View notes
Photo

*Customized By TSP* *Customized Wooden Wall Clock* 🌹 Size: 12 - 14 Inches 🌹 Material : MDF 🌹High Quality 🌹 *Black , Brown & Light Yellow Color Wood Availaible Only* ⚠️Many more designs are available 😻Quality will give you the best and VIP😻 🙀 *Sale Price: 1250/-*🙀 🔥🔥Super Sale Limited Time Offer🔥🔥ahc 💬For more details come inbox 03037911308 or visit us# flashmall.pk https://www.instagram.com/p/CUCe17MMJSk/?utm_medium=tumblr
0 notes
Text
Scientists reveal the nails that hold the most bacteria
Those pretty nails you see women sporting these days are hiding a dirty secret. New research has found gel nails retain the most bacteria after cleaning. Scientists have discovered crevices created as nails grow with gel polish on might make hand sanitising more difficult. What’s more concerning is their study actually looked at the bacteria on healthcare workers nails, and found gel polish harbours the most germs, even after hand sanitiser is used.
“Bacteria is a concern with lash extensions, especially with Staphylococcus aureus (S. aureus), the most common type of bacteria to live on eyelashes,” Chan says. “The waste produced by S. aureus is toxic to the eye and causes inflammation of the lid margins. Lash extensions themselves also harbour extra bacteria, increasing the risk of lid infections like styes and eye infections like bacterial conjunctivitis, commonly known as pink eye.”
Among the symptoms of styes, says Chan, are a bump on the lid with swelling, redness, pain, itching and white or yellow discharge. Bacterial conjunctivitis, meanwhile, presents with redness of the ocular surface, tearing, irritation, yellow-green discharge leading to the lids being matted shut upon waking, and even light sensitivity and blurry vision.
“At their worst, lash extensions can cause vision loss if corneal scarring occurs from bacterial conjunctivitis or an ulcer,” says Chan. “An ulcer can develop if fibres from lash extensions scratch the corneal surface.
“With lash extensions, you should see your optometrist immediately if you experience lid or eye swelling, redness, discharge, irritation, blurry vision or light sensitivity.”
It's time for women to stop wearing acrylics and false lashes. It's time. It's annoying. You are unsettling to look at. There is a lot of bacteria growing on your face and hands. You look like a moth with claws. And for what?
2K notes
·
View notes
Photo

Sometimes i smile like the sun So bright, the light, yellow... That's very much fun. Sometimes i look like the sky Blue, so blue, so high, That's always my try Sometimes i speak like a cuckoo Black, but sweet, so sweet That's my sound flow. Sometimes i roar like a lion Cute but loudy, so crowdy These line have different opinion What all about these sometimes Sometimes i love me more than you. Yes you. #AHC Anniversary -2020 https://www.instagram.com/p/CFIHgYPBHtP/?igshid=1g1th5gwajb4y
0 notes
Text
The Fisherman
My design for the fisherman. when I was stood with the large fish in the AHC this week, I felt really powerful holding the fish in the same way I have drawn here. I felt like the fisherman so I included that here. if there is time to make some costume for the fisherman id love to because a pop of colour in the trousers maybe yellow like many fisherman wear muddied down could be really nice. if I could clip my skirt up or even remove it that could be useful. even some wellies might help that image of the fisherman. this is quite an abstract character which compliments Céleste’s Ciguapa nicely, but id like to have some more fisherman imagery to aid the audiences understanding of the scene. this would all be in the world of the artists work of course in order to keep it relevant and aesthetically coherent.
I thought about the base of the moon from the other group doubling as the Fishermans staff or fishing rod. having said this I’m unsure of if a fishing rod is the best option. if we used this, I thought about using one of the bread groups puppets as bate for the fish to link all our pieces together.
I think this mask ive drawn here is too big and not sitting in the right place as initially I wanted to be able to see through the mouth and wear the top part of the face as a hat but discussions with jess and Ruby brought to my attention that this might not be the most effective placement. having the eyes in the mouth could be distracting to the audience potentially.
I think that the mound that the fisherman emerges from also needs to be removes to allow me to freely move around the space or it needs to be attached in a way that means I don't have to worry about the excess fabric. dust sheets are quite loud when you are inside them so this is something to be considered and they are quite heavy if used for a long time.
I really imagine the fisherman catching the fish in their bare hands and the fish writhing, trying to escape until it sadly gives up. even a moment where a brick from the scenery is used to ‘take a picture to commemorate the catch of the day’.
braces might be a nice touch to the costume to emphasise the Fishermans workwear.

0 notes
Link
Sell ON MC74VHC1G14DFT1G New Stock #MC74VHC1G14DFT1G ON MC74VHC1G14DFT1G New Single Inverter, Schmitt Trigger Input, SC-88A (SC-70-5 / SOT-353), 3000-REEL, MC74VHC1G14DFT1G pictures, MC74VHC1G14DFT1G price, #MC74VHC1G14DFT1G supplier ------------------------------------------------------------------- Email: [email protected] https://www.slw-ele.com/mc74vhc1g14dft1g.html ------------------------------------------------------------------- Manufacturer Part Number: MC74VHC1G14DFT1GBrand Name: ON SemiconductorPbfree Code: ActiveIhs Manufacturer: ON SEMICONDUCTORPart Package Code: SOT-353Package Description: TSSOP,Pin Count: 5Manufacturer Package Code: 419A-02ECCN Code: EAR99HTS Code: 8542.39.00.01Manufacturer: ON SemiconductorRisk Rank: 0.73Family: AHC/VHCJESD-30 Code: R-PDSO-G5JESD-609 Code: e3Length: 2 mmLogic IC Type: INVERTERMoisture Sensitivity Level: 1Number of Functions: 1Number of Inputs: 1Number of Terminals: 5Operating Temperature-Max: 125 °COperating Temperature-Min: -55 °CPackage Body Material: PLASTIC/EPOXYPackage Code: TSSOPPackage Shape: RECTANGULARPackage Style: SMALL OUTLINE, THIN PROFILE, SHRINK PITCHPeak Reflow Temperature (Cel): 260Propagation Delay (tpd): 20.5 nsQualification Status: Not QualifiedSeated Height-Max: 1.1 mmSupply Voltage-Max (Vsup): 5.5 VSupply Voltage-Min (Vsup): 2 VSupply Voltage-Nom (Vsup): 3.3 VSurface Mount: YESTechnology: CMOSTemperature Grade: MILITARYTerminal Finish: Tin (Sn)Terminal Form: GULL WINGTerminal Pitch: 0.65 mmTerminal Position: DUALTime Single Inverter, Schmitt Trigger Input, SC-88A (SC-70-5 / SOT-353), 3000-REEL Shunlongwei Inspected Every MC74VHC1G14DFT1G Before Ship, All MC74VHC1G14DFT1G with 6 months warranty. Part Number Manufacturer Packaging Descript Qty TC74LCX14FTTOSHIBASSOP14IC LVC/LCX/Z SERIES, HEX 1-INPUT INVERT GATE, PDSO14, 4.40 MM, 0.65 MM PITCH, PLASTIC, TSSOP-14, Gate 1290 PCSMPC507AUTISOP-288-Channel Differential-Input Analog Multiplexer 28-SOIC -40 to 85 1246 PCSPY1112HSTANLEYSMD0805Single Color LED, Yellow Green, Milky White, 1.3mm, 2 X 1.25 MM, 0.80 MM HEIGHT, ROHS COMPLIANT PACKAGE-2 8090 PCSLA1888NM-MPB-ESANYOQFP 5607 PCSOCM242FOKISOP6FET Output Optocoupler 4681 PCSMAX5023SASA-TMAXIMSOP8Fixed Positive LDO Regulator, 3.3V, 1.5V Dropout, BICMOS, PDSO8, 0.150 INCH, MS-012, SOIC-8 2570 PCSHA17431VLPHITACHITwo Terminal Voltage Reference, 1 Output, 2.5V, BIPolar, PDSO5, MPAK-5 87090 PCSAT25080AN-SQ-2.7ATMELSOP8 1877 PCSVBO160-08NO7IXYSBridge Rectifier Diode, 1 Phase, 139A, 800V V(RRM), Silicon, ROHS COMPLIANT, MODULE-4 1609 PCSLTM08C351LTOSHIBA350Mcd, B/R 300 1LCD DOT MATRIX GRAPHIC DISPLAY MODULE,WHITE 250 PCS
0 notes
Text
DOE monitoring yellow alert status for Luzon grid
#PHnews: DOE monitoring yellow alert status for Luzon grid
MANILA -- A yellow alert has been raised over the Luzon grid from 10 a.m. until 4 p.m. Monday, with unplanned outages alone reaching 2,955 megawatts (MW).
Data released by the Department of Energy (DOE) showed that the bulk of the outages are accounted for by outside management control (OMC) at 1,825 MW.
Of this,1,620 MW was caused by the planned outage of the Malampaya gas-to-power facility at offshore northeast Palawan, which the Department of Energy (DOE) earlier said was scheduled between October 12-15, 2019.
This maintenance shutdown affected the natural gas sources of Ilijan Block A (600 MW) and Ilijan Block B (600 MW) of KEPCO Ilijan Corporation in Ilijan, Batangas; and the San Gabriel Power Plant (420 MW), which is operated by the First NatGas Power Corporation (FNPC) in First Gen Clean Energy Complex in Batangas City.
Also part of the OMC outages are the 150 MW deficiency of the Malaya Thermal Power Unit 1 in Pililla, Rizal; and the 55 MW deficiency caused by Unit 5 of the MakBan Geothermal Power in Calauan, Laguna.
The largest forced outage during the day was caused by the condenser tube leak and ongoing washing of boiler and turbine to remove contaminated water from sodium and chloride of Team Energy Corporation’s (TEC) Sual Coal-fired Power Plant Unit 1.
DOE data show that TEC’s Sual coal-fired power plant, which has an installed capacity of 647 MW, was out of operations since last Oct. 11 and is estimated to resume operations on Oct. 21.
Others that are on forced outages to date are the Units 1 and 2 of Prime Meridian Power Corporation’s (PMPC) Avion Natural gas-fired power plant, both of which have a dependable capacity of 48 MW; Unit 1 of GNPower Mariveles Coal Plant Ltd. Co. (GMPC), which has a dependable capacity of 316 MW; Unit 1 of Aboitiz Power Renewables, Inc. (APRI) Tiwi Geothermal Power Plant, which has a 60MW dependable capacity; and the 12MW San Jose 1 Biomass Power Plant, which has an 11MW dependable capacity.
The resumption of operations of these five power plant’s units that are on forced outages is still being determined.
Aside from the forced outages, there are also power plants that are on de-rated operations to date and these include the SEM-Calaca Power Corporation (SCPC) Calaca Unit 2, from 300MW to just 200MW; Angat Hydropower Corporation (AHC) Angat Hydroelectric Power Plant Main Units 1-4, from 200MW to 160MW; and the First Gen Hydro Power Corporation’s (FGHPC) Pantabangan Hydroelectric Power Plant Units 1 and 2, from 120MW to zero.
Energy Undersecretary and spokesperson Felix William Fuentebella assured the public that DOE officials are closely watching the current state of Luzon’s capacity.
“We are closely monitoring the situation. The power bureau is on this,” he told journalists in a Viber message.
It has been a few months since the Luzon grid experienced capacity deficiency.
Yellow and red alert power situations are normally experienced in the grid during the summer months because the hydro-electric power plants are on maintenance shutdown due to water supply issues. (PNA)
***
References:
* Philippine News Agency. "DOE monitoring yellow alert status for Luzon grid." Philippine News Agency. https://www.pna.gov.ph/articles/1083099 (accessed October 15, 2019 at 12:56AM UTC+14).
* Philippine News Agency. "DOE monitoring yellow alert status for Luzon grid." Archive Today. https://archive.ph/?run=1&url=https://www.pna.gov.ph/articles/1083099 (archived).
0 notes
Text
Unfortunately the obscure AU competition will not be happening but that simply means you guys get this picture in high quality now :) selfie time! They can and will beat you up!
- Trauma
Textless version:
#ahc art#ahc cody#ahc yellow#ahc blue#ahc purple#ahc red#ahc bishop#ahc au#actions have consequences au#tmnt 03#tmnt dark turtles#tmnt Cody jones
142 notes
·
View notes
Text
Now with a friend! :D
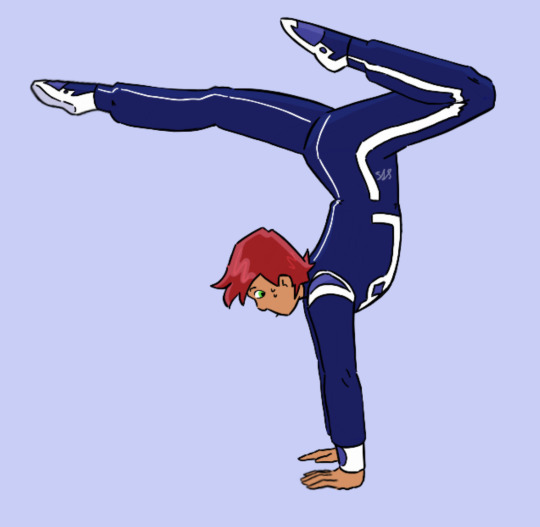
Ninja in training!
#🌹 charlie’s art#AHC AU#ahc Cody#ahc Yellow#teenage mutant ninja turtles#tmnt 2003#2k3 cody#tmnt fast forward#tmnt dark turtles#cody jones
108 notes
·
View notes
Text
Taxi Demand Prediction in NYC
1. Introduction
In big cities like New York, the taxi demand is imbalanced. While in some areas passengers wait for a long time to get a taxi, there are taxis that roam around the city empty. This imbalance has led to a decrease in profit for TLC and per the New York City Mobility report published in October 2016 there is a 11% year-on-year decrease in taxi demand. This decrease in demand is attributed to the increase in popularity of the for-hire vehicles like Uber and Lyft. Uber and Lyft are performing well because of their efficiency that is made possible by the underlying machine learning algorithms and pickup optimizations. With these tools Uber and Lyft have solved the imbalance in the supply and demand. In our project, we have primarily focused on solving the supply-demand problem that the TLC company face. The rationale of our project is to find the factors that influence demand in an area in NYC and to forecast the demand for the future using past data. This will help a) TLC to increase their profits and b) the taxi company efficiently compete with the for-hire-vehicle companies. The report is organized as follows. The existing literature in taxi demand prediction is reviewed in Section 2. Data acquisition and Data Cleaning processes have been presented in Section 3 and 4. Analysis has been presented in Section 5. Other results are summarized in Section 5 followed by our conclusions in Section 6.
2. Background
This section provides the summary of the previous research on taxi demand Prediction. The majority of the existing literature is summarized below.
In the paper Taxi Demand Forecasting Based on Taxi Probe Data by Neural Networks, the author has used applied Neural Networks and back-propagation learning to reveal the relationship of Tokyo. They have also created forecasts using day of the week and amount of precipitation. The author concluded that week is an important factor for predicting the demand because the demand occurs periodically.
From their results the author concludes that 4-hours and 50 neurons in their neural network outperforms other networks.
Zhao, Khryashchev, Freire, Silva and Vo have used the data sets of uber and yellow cabs to compare the performance of the two companies in the 9940 blocks of Manhattan, NYC. They have used Markov predictor, Lempel-Ziv_Welch predictors and Neural Networks to predict the demand and have compared the models. The authors have employed symmetric Mean Absolute Percentage Error to evaluate the algorithms. They have concluded that the performance of Neural network is better with blocks with low predictability and Markov predictor can predict the taxi demand with a high accuracy in the blocks with high predictability.
Matias, Gama and Ferreira have used live data to predict the spatial distribution of passengers to help the taxi companies fulfill their demands in Portugal. The have used Time-Varying Poisson Model, Weighted Time-Varying Poisson Model, ARIMA and Sliding-Window Ensemble Framework and symmetric Mean Percentage error is used to calculate the performance of the model. The maximum value for error found out by the authors was 28.7% and the sliding window Ensemble model was the one that always performed well.
Phithakkitnukoon, Velasco, Bento, Biederman and Rati have just used predicted models to find the number of vacant taxis based on time of the day, day of the week and weather conditions in Lisbon, Portugal. The have implemented an inference engine which is based on the Naïve Bayes Classifier with error based learning algorithm. The historical data for first 30 days data is used as the training set and the next 77 days is used as the testing set. A prediction for the vacant taxis is made for the next 24 hours. The authors have concluded that they had an overall error of 0.8 taxis per square kilometers.
Chang, Tai, Chen and Hsu have used spatial statistical analysis, data mining and clustering algorithms on historical data of the taxi requests to discover the demand distribution. The selection of similarity measure and clustering algorithm decides the result of clustering. DB-Scan, k means, AHC have been used as the different clustering algorithms.
Singhvi, Frazier, Henderson, Mohony, Shmoys and Woodward have dealt with a different demand prediction. The have predicted the demand for bike sharing systems. The have the NYC CitiBike dataset along with weather database to help planners identify the demand for bikes in the city. The have used Linear regression with Log transormations to predict the demand. They have used the data of May to predict the demand that occurs in the next month and so on.
Li, Zhang and Sun, in their paper have investigated the efficient and in efficient passenger finding strategies based on the data from 5350 Taxis in China. They have used the L1 Norm SVM to select the salient patterns for discriminating top- and ordinary-performance taxis. With that algorithm they have built a taxi performance predictor on the selected patterns and have achieved a prediction accuracy of 85.3%.
One major scope for improvement in demand forecasting of Taxi usage is that interaction between different predictors. Although, majority of past models include most important or all the related predictors to predict taxi demand. We have not come across a research paper that has considered and model correlation between various predictor and their interaction. Precisely modeling those correlations might improve the accuracy of the taxi demand. Best accuracy that has been found is 89% till now. Apart from that, one of the papers has also compared location vise performance of different models and this idea can be used for further exploration to generate a larger combinatory model based on small area wise best predictive models.
3. Data:
The yellow and green taxi trip records include fields capturing pick-up and drop-off dates/times, pick-up and drop-off locations, trip distances, itemized fares, rate types, payment types, and driver-reported passenger counts. The data used were collected and provided to the NYC Taxi and Limousine Commission (TLC) by technology providers authorized under the Taxicab & Livery Passenger Enhancement Programs (TPEP/LPEP). The trip data was not created by the TLC. The total size of a single dataset was 2-2.2 GB and there were 12 datasets for 12 months.
The NOAA weather data are land-based observations are collected from instruments sited at locations on every continent. They include temperature, dew point, relative humidity, precipitation, wind speed and direction, visibility, atmospheric pressure, and types of weather occurrences such as hail, fog, and thunder. NCEI provides a broad level of service associated with land-based observations. There were a total of 12 weather datasets.
3.1. Reverse Geocoding:
To predict the demand of the taxis in different areas we needed the zip codes for different areas in New York City. The data set had the values of latitudes and longitudes. We had to get the zipcodes from Google Maps API(http://maps.google.com/maps/api/geocode/json?sensor=false&latlng= ) using a Reversing Geocoding Algorithm. Google API had a limit of 2,500 zip codes extractions for an ip per day.
3.2. Feature Engineering – Pickup and Drop-off Frequencies:
The frequency of the pickups at a location was computed by aggregation the number of pickups that were in the dataset corresponding to a location, data and time. Similar aggregation of the drop-offs were also done to get the frequency of drop-offs. These frequencies were the demand of taxis at a location for a particular time. Outside of New York and New Jersey city values for location (latitude and longitude) has been found by filtering the dataset by range of latitudes and longitudes, and have been removed. Data with missing values of longitude and latitude have been deleted as imputation of those values is practically impossible.
3.3. Feature Engineering - Weather Dataset:
The weather datasets have been taken from NOA. It has total 40 variables and has temperature and relative humidity, snow, rain, amount of snowfall and visibility as the important parameters.
Many variables in this dataset has character codes of weather property. We have referred data dictionary and NOAA literature to convert those variables into categorical variables. Additionally, we have used algebraic dimensionality reduction techniques and correlation properties to merge or remove some variables. Some variables which have no relation with demand of the taxi and location of data (New York city) has been deleted directly. Final dataset has total of 9 weather variables.
The weather dataset didn’t have hourly weather data for 16 hours out of 8760 hours of the year. To impute this data, we have used package ‘missForest’ in R. The package imputes values based on the random forest algorithm.
3.4. Feature Engineering – Final dataset
The final dataset to apply models has been created by merging the transformed taxi demand dataset with final weather dataset by matching the values of each date and hour in both the dataset. The total taxi demand data size is 25 GB and weather data size is 8 Gb, after converting the dataset the final dataset has the size of 56 MB.
4. Exploratory Data Analysis:
Exploratory data analysis is essential part before applying any statistical models for predictive analysis. Exploratory data analysis and data visualization can express large part of different relationships between variable and response. It can also help us in identifying the behavior of response variable for time series data.
Due to large size of each month’s dataset (13-15 million entries) R is not able to plot the unique data points in a plot. Hence, to display some of the complex plots we have learned data visualization software tableau and generated visualizations.
The correlation plot shown above shows the correlation between the various variables. Given that it’s a time series data the variables are not highly correlation. We can still see some positive correlation
The above image shows the demand for taxi cabs for the month of January. The most concentrated part shows the high demand. As we can see the blue part shows the concentrated demand for the area of manhanttan. The bottom part shows the JFK and Lagaurdia which has high demand. This plot shows the busy areas of manhattan. Such plots can be made for other months also.
The image above shows the pick up count for the month of January for the taxi cabs for a particular week. As we can see there is very less demand for the weekends as compared to weekdays
This image shows the demand for the taxi for the January month which has been further broken down in days for whole month. As we can see that there is a dip in the demand for every 7 days which shows that on weekends there is a less demand.
The figure shows the mosaic plot for march month for a week . Th edays are the aggregate sum of all the respective days in that month. Saturday has the highest demand followed by Sunday whch is shown by the darkest colour.
This image represents the avg. fare amount for month of March. The thickness of the band of each color shows the time of the day, so we can say that for 5am the fare amount is low as demand is low. The highest one being 10am to 10pm which are usual office hours.
The figure shows average demand for the month of march with sum of all weeks. It has been divided between hours of day. As we can see that mid night time stamps have the highest peaks as compared to others.
The plot shows the demand based on zipcodes. Here the numbers shows the demand of that location.
The image shows the demand for all the months. The sum of demand is low for February as the number of days is less. This pattern can be seen for other months also.
We can see the variation in demand with amount of snowfall. General trend can be seen towards reduction of demand with increase in amount of snowfall.
5. Models
After studying the data, it was evident that the dataset is a time series and that to with large no of different categories for variables like location (zipcode) and weather variables. After referring to literature on time series, previous work on our dataset and basic statistics of time series. We have selected the best models that can be applied for a time series algorithm and they are described below.
5.1 Support Vector machines:
A Support Vector Machine (SVM) is a discriminative classifier formally defined by a separating hyperplane. In other words, given labeled training data (supervised learning), the algorithm outputs an optimal hyperplane which categorizes new examples. Then, the operation of the SVM algorithm is based on finding the hyperplane that gives the largest minimum distance to the training examples. Twice, this distance receives the important name of margin within SVM’s theory. Therefore, the optimal separating hyperplane maximizes the margin of the training data.
where represents each of the labels of the training examples.
SVM is used for a variety of purposes, particularly classification and regression problems. SVM can be especially useful in time series forecasting, from the stock market to chaotic systems. The method by which SVM works in time series is like classification: Data is mapped to a higher-dimensional space and separated using a maximum-margin hyperplane. However, the new goal differs in that our goal is to find a function that can accurately predict future values
5.2 Random Forest:
Random Forests are an ensemble learning method (also thought of as a form of nearest neighbor predictor) for classification and regression that construct a number of decision trees at training time and outputting the class that is the mode of the classes output by individual trees. Random Forests are a combination of tree predictors where each tree depends on the values of a random vector sampled independently with the same distribution for all trees in the forest. Random Forests are a wonderful tool for making predictions considering they do not overfit because of the law of large numbers. Introducing the right kind of randomness makes them accurate classifiers and regressors. For some number of trees T:
Sample N cases at random with replacement to create a subset of the data (see top layer of figure above). The subset should be about 66% of the total set.
1. At each node:
1. For some number m (see below), m predictor variables are selected at random from all the predictor variables.
2. The predictor variable that provides the best split, according to some objective function, is used to do a binary split on that node.
3. At the next node, choose another m variables at random from all predictor variables and do the same.
Depending upon the value of m, there are three slightly different systems:
• Random splitter selection: m =1
• Breiman’s bagger: m = total number of predictor variables
• Random forest: m << number of predictor variables. Brieman suggests three possible values for m: ½√m, √m, and 2√m
5.3 Bart:
BART is a Bayesian approach to nonparametric function estimation using regression trees. Regression trees rely on recursive binary partitioning of predictor space into a set of hyperrectangles in order to approximate some unknown function f. Predictor space has dimension of the number of variables, which we denote p. Tree-based regression models have an ability to flexibly fit interactions and nonlinearities. Models composed of sums of regression trees have an even greater ability than single trees to capture interactions and non-linearities as well as additive effects in f. BART can be considered a sum-of-trees ensemble, with a novel estimation approach relying on a fully Bayesian probability model. Specifically, the BART model can be expressed as:
Y = f(X) + E ≈ T M 1 (X) + T M 2 (X) + . . . + T Mm (X) + E, E Nn (0, σ2 In ) where Y is the n × 1 vector of responses, X is the n × p design matrix (the predictors columnjoined), E is the n × 1 vector of noise. Here we have m distinct regression trees, each composed of a tree structure, denoted by T, and the parameters at the terminal nodes (also called leaves), denoted by M.
5.4 ARIMA:
ARIMA stands for auto-regressive integrated moving average and is specified by these three order parameters: (p, d, q). The process of fitting an ARIMA model is sometimes referred to as the Box-Jenkins method. ARIMA is most common and efficient method used in time series prediction.
An auto regressive (AR(p)) component is referring to the use of past values in the regression equation for the series Y. The auto-regressive parameter p specifies the number of lags used in the model. For example, AR(2) or, equivalently, ARIMA(2,0,0), is represented as
where φ1, φ2 are parameters for the model.The d represents the degree of differencing in the integrated (I(d)) component. Differencing a series involves simply subtracting its current and previous values d times. Often, differencing is used to stabilize the series when the stationarity assumption is not met, which we will discuss below.
A moving average (MA(q)) component represents the error of the model as a combination of previous error terms et. The order q determines the number of terms to include in the model
Differencing, autoregressive, and moving average components make up a non-seasonal ARIMA model which can be written as a linear equation:
where yd is Y differenced d times and c is a constant.
After applying the ARIMA to our dataset this the output of result that gets generated.
Plot on the top displays the error residual for each prediction values in time series. Graphs below displays autocorrelation function and partial autocorrelation function for prediction. It determines stationarity and seasonality in time series data, which accounts changes in data with variation in time.
Above graph displays predicted values by ARIMA for each hour for a specific day.
5.5 K-nearest neighbors:
In pattern recognition, the k-nearest neighbors algorithm (k-NN) is a non-
parametric method used for classification and regression.[1] In both cases, the input consists of the k closest training examples in the feature space. The output depends on whether k-NN is used for classification or regression:
• In k-NN classification, the output is a class membership. An object is classified by a majority vote of its neighbors, with the object being assigned to the class most common among its k nearest neighbors (k is a positive integer, typically small). If k = 1, then the object is simply assigned to the class of that single nearest neighbor.
• In k-NN regression, the output is the property value for the object. This value is the average of the values of its k nearest neighbors.
k-NN is a type of instance-based learning, or lazy learning, where the function is only approximated locally and all computation is deferred until classification. The k-NN algorithm is among the simplest of all machine learning algorithms. We have used 5 as a K in our analysis.
Both for classification and regression, it can be useful to assign weight to the contributions of the neighbors, so that the nearer neighbors contribute more to the average than the more distant ones.
6. Results:
The comparison of performance of different models applied has been done. The performance measures used for the performance are R^2 for training dataset, RMSE (Root mean square error) for training dataset and RMSE (Root Mean square error) for test dataset.

We don’t have values of R^2 and RMSE for training dataset in case of ARIMA model. But, we can see that ARIMA performance better than other models on the test dataset. In case of Machine learning algorithms Random Forest is clearly performing better than other models.
7. Future Work:
Artificial neural network can be applied to time series analysis. But, transformation of data is required for applying ANN to the time series dataset.
Additionally, we can improve the accuracy of prediction by applying each model for different areas of New York city and creating an ensemble model from it. This can be done by finding the best model for each area and using the best results for each area to predict combined demand for each city in New York city.
0 notes
Text
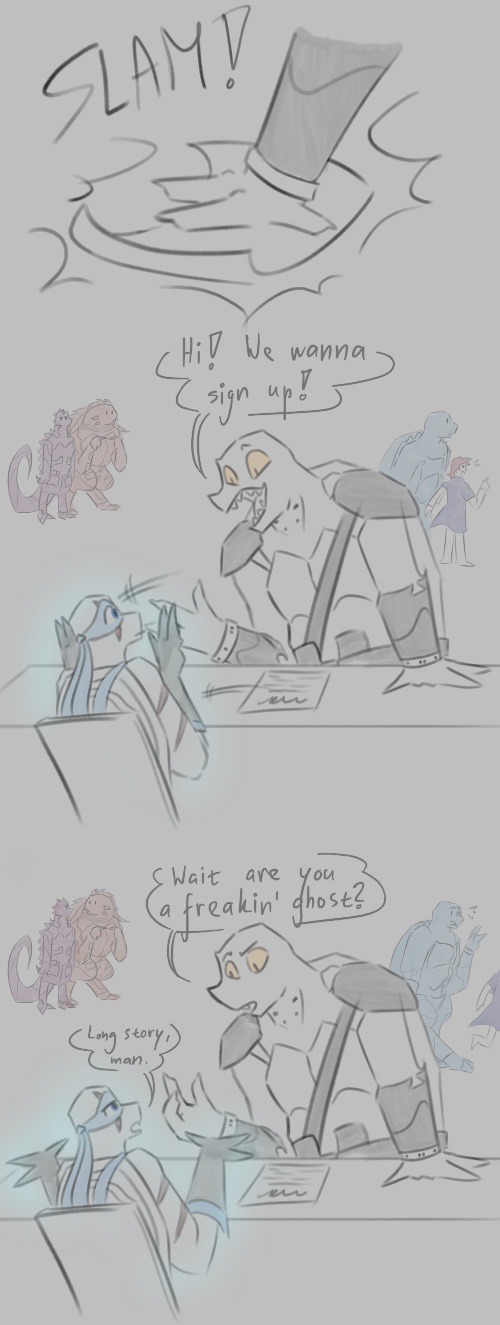
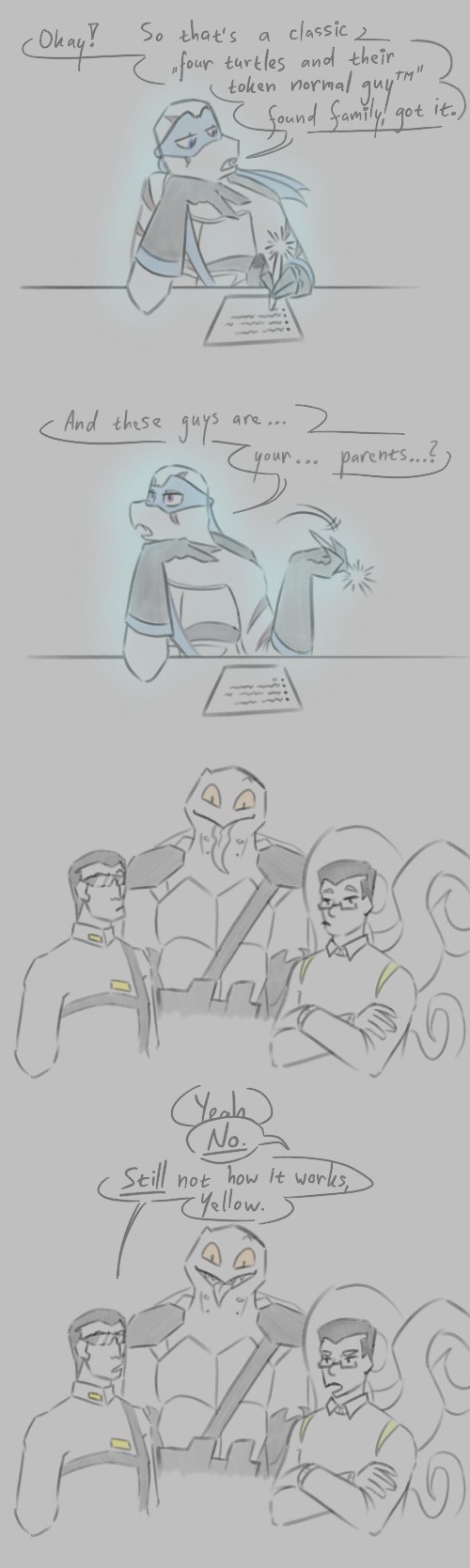
Me and Ade figured it might be easier to have one blog to refer to the AU, and the @obscure-au-comp was a perfect opportunity! Found out the organizer, Vei, has a horrifying and awesome rottmnt au called Spirit Box AU so shoutout to Onleo (Onryo Leo) for becoming the competition receptionist in my mind <3
-- Trauma
#ahc au#ahc art#obscure au competition#ahc blue#ahc yellow#ahc red#ahc purple#ahc bishop#ahc cody#ahc stockman#the ffam's all here#onryo leo#onryo leo au#hope you don't mind me nicknaming him onleo it rhymed and i couldn't help myself
89 notes
·
View notes
Note
Little Yellow is grasping the family concept
So cute :) *Gives him headpats

Watch out, he’s a biter! — Trauma
28 notes
·
View notes