#WeightOnlyQuantization
Explore tagged Tumblr posts
Text
INT8 & INT4 Weight Only Quantization WOQ On Intel Extension
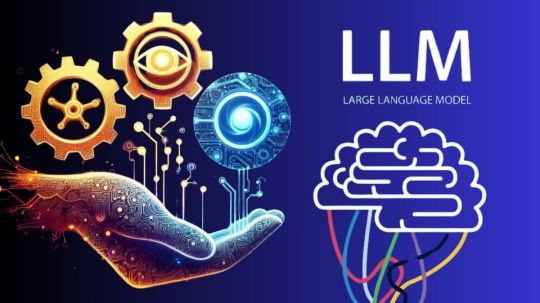
Weight Only Quantization(WOQ)
A practical guide to Large Language Models (LLMs) quantization. The capabilities, uses, and complexity of large language models (LLMs) have all significantly risen in recent years. With an ever-increasing amount of parameters, weights, and activations, LLMs have become larger and more intelligent.
However, They usually have to compress LLMs without significantly sacrificing their performance in order to increase the number of possible deployment targets and lower the cost of inference. Large neural networks, including language models, may be made smaller using a variety of methods. Quantization is one such crucial method.
WOQ meaning
In machine learning, especially in deep learning, Weight Only Quantization (WOQ) is a technique that minimizes the size of neural network models without compromising their functionality. It entails quantizing just the neural network’s weights the parameters that define the behavior of the model into a format with less precision (e.g., 8-bit instead of 32-bit).
This article provides an example of code that uses the Intel Extension for Transformers tool to conduct Weight Only Quantization (WOQ) on an LLM (Intel/neural-chat-7b model) for both INT8 and INT4.
How does quantization work?
INT8 Vs INT4
The process of switching to lower precision data types, such as float16, INT8 or INT4, from high-precision representation, such as float32, for weights and/or activations is known as quantization. Lower precision may greatly minimize the amount of memory needed.
While this may seem simple in principle, there are a lot of subtleties to consider, and computing data type is the most crucial warning to know. Certain operations need us to scale the representation back to high precision at runtime since not all operations support or have low-precision implementation. Although there is some additional cost, they may lessen its effects by using tools like Intel Neural Compressor, OpenVINO toolkit, and Neural Speed.
Because these runtimes include optimized implementations of several operators for low-precision data types, upscale values to high-precision is not necessary, resulting in improved speed and reduced memory use. If lower-precision data types are supported by your hardware, the performance improvements are substantial. For instance, support for float16 and bfloat16 is included into Intel Xeon Scalable processors of the 4th generation.
Therefore, quantization only serves to lower the model’s memory footprint; nevertheless, it may also introduce some cost during inference. Using optimized runtimes and the newest hardware is required to obtain both memory and performance improvements.
What Does WOQ Mean?
There are several methods for quantizing models. Model weights and activations the output values produced by every neuron in a layer are often quantized. One of these quantization methods, called Weight Only Quantization(WOQ), preserves the original accuracy of the activations while only quantizing the model weights. Faster inference and a reduced memory footprint are the clear advantages. In actual use, WOQ improves performance without appreciably affecting accuracy.
Code Execution
The Intel/neural-chat-7b-v3-3 language model’s quantization procedure is shown in the provided code sample. The model, which is an improved version of Mistral-7B, is quantized using Weight Only Quantization (WOQ) methods made available by the Intel Extension for Transformers.
With only one line of code, developers can easily use the power of Intel technology for their Generative AI workloads. You import AutoModelForCausualLM from Intel Extension for Transformers rather of the Hugging Face transformers library, and everything else stays the same.
1. From intel_extension_for_transformers.transformers import AutoModelForCausalLM
For INT8 quantization, just set load_in_8bit to True.
1. # INT8 quantization 2. Q8_model = AutoModelForCausalLM.from_pretrained( 3. model_name, load_in_8bit=True)
Similarly, for INT4 quantization set load_in_4bit to True.1. # INT4 quantization 2. q4_model = AutoModelForCausalLM.from_pretrained( 3. model_name, load_in_4bit=True)
The Hugging Face transformers library may be used in the same way for implementation.
If you set device to GPU, the aforementioned code snippets will utilize BitandBytes for quantization. This makes your code run much faster without requiring any code changes, regardless of whether you are utilizing a CPU or GPU.
GGUF model in operation
A binary file format called GGUF was created expressly to store deep learning models like LLMs especially for CPU inference. It has several important benefits, such as quantization, efficiency, and single-file deployment. They will be utilizing the model in GGUF format in order to maximize the performance of their Intel hardware.
Generally, one would need to utilize an extra library like Llama_cpp in order to execute models in GGUF format. Still, you may use it Intel Extension for Transformers library to run GGUF models since Neural Speed is built on top of Llama_cpp.1. model = AutoModelForCausalLM.from_pretrained( 2. model_name=“TheBloke/Llama-2-7B-Chat-GGUF”, 3. model_file=“llama-2-7b-chat.Q4_0.gguf” 4. )
Take a look at the code example. The code example demonstrates how to use Intel’s AI Tools, Intel Extension for Transformers, to quantize an LLM model and how to optimize your Intel hardware for Generative AI applications.
INT4 vs INT8
Quantizing LLMs for Inference in INT4/8
Better quantization approaches are becoming more and more necessary as models become bigger. However, what is quantization exactly? Model parameters are represented with less accuracy by quantization. For example, using float16 to represent model weights instead of the widely used float32 may reduce storage needs by half.
Additionally, it improves performance at lesser precision by lowering computational burden. Nevertheless, a drawback of quantization is a little reduction in model accuracy. This happens when accuracy decreases and parameters have less representation power. In essence, quantization allows us to sacrifice accuracy for better inference performance (in terms of processing and storage).
Although there are many other approaches to quantization, this sample only considers Weight Only Quantization (WOQ) strategies. Model weights and activations the output values produced by every neuron in a layer are often quantized. But only the model weights are quantized by WOQ; activations remain unaltered. In actual use, WOQ improves performance without appreciably affecting accuracy.
The transformers library from HuggingFace makes quantization easier by offering clear choices. To enable quantization, users just need to specify the load_in_4bit or load_in_8bit option to True. But there’s a catch: only CUDA GPUs can use this capability. Unfortunately, only CUDA GPU devices can use the BitsandBytes configuration that is automatically built when these arguments are enabled. For consumers using CPUs or non-CUDA devices, this presents a problem.
The Intel team created Intel Extension for Transformers (ITREX), which improves quantization support and provides further optimizations for Intel CPU/GPU architectures, in order to overcome this constraint. Users may import AutoModelForCasualLM from the ITREX library rather of the transformers library in order to use ITREX. This allows users, irrespective of their hardware setup, to effortlessly use quantization and other improvements.
The from_pretrained function has been expanded to include the quantization_config, which now takes in different settings for CUDA GPUs and CPUs to perform quantization, including RtnConfig, AwqConfig, TeqConfig, GPTQConfig, and AutoroundConfig. How things behave when you set the load_in_4bit or load_in_8bit option to True is dependent on how your device is configured.
BitsAndBytesConfig will be used if the CUDA option is selected for your device. RtnConfig, which is specifically tailored for Intel CPUs and GPUs, will be used, nonetheless, if your device is set to CPU. In essence, this offers a uniform interface for using Intel GPUs, CPUs, and CUDA devices, guaranteeing smooth quantization across various hardware setups.
Read more on govindhtech.com
#INT8#int4#WeightOnlyQuantization#WOQ#intelextension#largelanguagemodel#intelneuralcompressor#quantizingmodels#generativeai#CPU#GPU#deeplearningmodels#News#TechNews#Technology#technologynews#technologytrends#govindhtech
0 notes