#Scraping Google Reviews
Explore tagged Tumblr posts
Text
Advanced Steps For Scraping Google Reviews For Informed Decision-Making

Google reviews are crucial to business's and buyer’s information-gathering processes. They play the role in providing validation to customers. There may be actual customers who would read other’s opinions in order to decide whether they want to buy from a specific business place or to use a particular product or even a service. This means that positive reviews will, in a way, increase the trust people have for the product, and new buyers will definitely be attracted. Hence, the acts of positively enhancing the image of different business entities through public endorsements are critical determinants for building a reputable market niche on the World Wide Web.
What is Google Review Scraping?
Google Review Scraping is when automated tools collect customer reviews and related information from Google. This helps businesses and researchers learn what customers think about their products or services. By gathering this data using a Google Maps data scraper, organizations can analyze it to understand how people feel. This includes using tools to find the right business to study, using web scraping to get the data, and organizing it neatly for study.
It's important to follow Google's rules and laws when scraping reviews. Doing it wrong or sending too many requests can get you in trouble, such as being banned or facing legal problems.
Introduction to Google Review API
Google Review API, also known as Google Places API, is a service Google offers developers. It enables them to learn more about places in Google Maps, such as restaurants or stores. This API has remarkable characteristics that permit developers to pull out reviews, ratings, photos, and other significant data about these places.
However, before using the Google Review API, the developers are required to obtain a unique code known as the API key from Google. This key is kind of like a password that allows their apps or websites to ask Google for information. Subsequently, developers can request the API for details regarding a particular place, such as a restaurant's reviews and ratings. Finally, the API provides the details in a form that a programmer can readily incorporate into the application or website in question, commonly in the form of JSON.
Companies and developers employ the Google Review API to display customer reviews about service quality and experience on their websites and then work on the feedback. It is helpful for anyone who seeks to leverage Google's large pool of geographic data to increase the utility of his applications or web pages.
Features of Google Reviews API

The Google Reviews API offers several features that help developers access, manage, and use customer reviews for businesses listed on Google. Here are the main features:
Access to Reviews
You can get all reviews for a specific business, including text reviews and star ratings. Each review includes the review text, rating, reviewer's name, review date, and any responses from the business owner.
Ratings Information
When integrated with Google Map data scraper, the API provides a business's overall star ratings, calculated from all customer reviews. You can see each review's star rating to analyze specific feedback.
Review Metadata
Access information about the reviewer, such as their name and profile picture (if available). Each review includes timestamps for when it was created and last updated. Those responses are also available if the business owner has responded to a review.
Pagination
The API supports pagination, allowing you to retrieve reviews in smaller, manageable batches. This is useful for handling large volumes of reviews without overloading your application.
Sorting and Filtering
You can sort reviews by criteria such as most recent, highest, lowest rating, or most relevant ratings. The API allows you to filter reviews based on parameters like minimum rating, language, or date range.
Review Summaries
Access summaries of reviews, which provide insights into customers' common themes and sentiments.
Sentiment Analysis
Some APIs might offer sentiment analysis, giving scores or categories indicating whether the review sentiment is positive, negative, or neutral.
Language Support
The API supports reviews in multiple languages, allowing you to access and filter reviews based on language preferences.
Integration with Google My Business
The Reviews API integrates with Google My Business, enabling businesses to manage their online presence and customer feedback in one place.
Benefits of Google Reviews Scraping

Google Reviews data scraping can help businesses analyze trends, monitor competitors, and make strategic decisions. Google Maps scraper can be beneficial in different ways. Let’s understand the benefits :
Understanding Customers Better
Through reviews, management can always understand areas customers appreciate or dislike in products or services offered. This enables them to advance their prospects in a way that will enhance the delivery of services to the customers.
Learning from Competitors
Businesses can use the reviews to compare themselves to similar companies. It assists them in visually discovering areas in which they are strong and areas with room for improvement. It is like getting a sneak peek at what other competitors are up to as a means of countering them.
Protecting and Boosting Reputation
Reviews enable business organizations to monitor their image on social media. Renters feel that companies can show engagement by addressing them when they post negative comments, demonstrating that the business wants to improve their experiences. Prospective consumers also benefit when positive reviews are given as much attention as negative ones from a seller's standpoint.
Staying Ahead in the Market
The review allows businesses to see which products customers are most attracted to and the current trend. This assists them in remaining competitive and relevant in the market, allowing them to make the necessary alterations when market conditions change.
Making Smarter Decisions
Consumer feedback is highly reliable as a source of information for making conclusions. Hence, no matter what the business is doing, be it improving its products, planning the following marketing strategy, or identifying areas of focus, the data from the reviews should be handy.
Saving Time and Effort
Automated methods are easier to use to collect reviews than manual methods, which is one reason why they are preferred. This implies that they will spend less time gathering the data and, therefore, can devote adequate time using it to transform their business.
Steps to Extract Google Reviews
It is easy to utilize Google Review Scraper Python for the effective extraction of reviews and ratings. Scraping Google reviews with Python requires the following pre-determined steps mentioned below:
Modules Required
Scraping Google reviews with Python requires the installation of various modules.
Beautiful Soup: This tool scrapes data by parsing the DOM (Document Object Model). It extracts information from HTML and XML files.# Installing with pip pip install beautifulsoup4 # Installing with conda conda install -c anaconda beautifulsoup4
Scrapy: An open-source package designed for scraping large datasets. Being open-source, it is widely and effectively used.
Selenium: Selenium can also be utilized for web scraping and automated testing. It allows browser automation to interact with JavaScript, handle clicks, scrolling, and move data between multiple frames.# Installing with pip pip install selenium # Installing with conda conda install -c conda-forge selenium
Driver manager of Chrome
# Below installations are needed as browsers # are getting changed with different versions pip install webdriver pip install webdriver-manager
Web driver initialization
from selenium import webdriver from webdriver_manager.chrome import ChromeDriverManager # As there are possibilities of different chrome # browser and we are not sure under which it get # executed let us use the below syntax driver = webdriver.Chrome(ChromeDriverManager().install())
Output
[WDM] – ====== WebDriver manager ====== [WDM] – Current google-chrome version is 99.0.4844 [WDM] – Get LATEST driver version for 99.0.4844 [WDM] – Driver [C:\Users\ksaty\.wdm\drivers\chromedriver\win32\99.0.4844.51\chromedriver.exe] found in cache
Gather reviews and ratings from Google
In this case, we will attempt to get three entities—books stores, restaurants, and temples—from Google Maps. We will create specific requirements and combine them with the location using a Google Maps data scraper. from selenium import webdriver from webdriver_manager.chrome import ChromeDriverManager from selenium.webdriver.support.ui import WebDriverWait from selenium.webdriver.common.action_chains import ActionChains from selenium.webdriver.support import expected_conditions as EC from selenium.common.exceptions import ElementNotVisibleException from selenium.webdriver.common.by import By from selenium.common.exceptions import TimeoutException from bs4 import BeautifulSoup driver = webdriver.Chrome(ChromeDriverManager().install()) driver.maximize_window() driver.implicitly_wait(30) # Either we can hard code or can get via input. # The given input should be a valid one location = "600028" print("Search By ") print("1.Book shops") print("2.Food") print("3.Temples") print("4.Exit") ch = "Y" while (ch.upper() == 'Y'): choice = input("Enter choice(1/2/3/4):") if (choice == '1'): query = "book shops near " + location if (choice == '2'): query = "food near " + location if (choice == '3'): query = "temples near " + location driver.get("https://www.google.com/search?q=" + query) wait = WebDriverWait(driver, 10) ActionChains(driver).move_to_element(wait.until(EC.element_to_be_clickable( (By.XPATH, "//a[contains(@href, '/search?tbs')]")))).perform() wait.until(EC.element_to_be_clickable( (By.XPATH, "//a[contains(@href, '/search?tbs')]"))).click() names = [] for name in driver.find_elements(By.XPATH, "//div[@aria-level='3']"): names.append(name.text) print(names)
Output
The output of the given command will provide the required data in a specific format.
How to Scrape Google Reviews Without Getting Blocked

Scraping Google Reviews without getting blocked involves several best practices to ensure your scraping activities remain undetected and compliant with Google's policies. If you're making a Google review scraper for a company or project, here are ten tips to avoid getting blocked:
IP Rotation
If you use the same IP address for all requests, Google can block you. Rotate your IP addresses or use new ones for each request. To scrape millions of pages, use a large pool of proxies or a Google Search API with many IPs.
User Agents
User Agents identify your browser and device. Using the same one for all requests can get you blocked. Use a variety of legitimate User Agents to make your bot look like a real user. You can find lists of User Agents online.
HTTP Header Referrer
The Referrer header tells websites where you came from. Setting the Referrer to "https://www.google.com/" can make your bot look like a real user coming from Google.
Make Scraping Slower
Bots scrape faster than humans, which Google can detect. Add random delays (e.g., 2-6 seconds) between requests to mimic human behavior and avoid crashing the website.
Headless Browser
Google's content is often dynamic, relying on JavaScript. Use headless browsers like Puppeteer JS or Selenium to scrape this content. These tools are CPU intensive but can be run on external servers to reduce load.
Scrape Google Cache
Google keeps cached copies of websites. Scraping cached pages can help avoid blocks since requests are made to the cache, not the website. This works best for non-sensitive, frequently changing data.
Change Your Scraping Pattern
Bots following a single pattern can be detected. To make your bot look like a real user, you must use human behavior with random clicks, scrolling, and other activities.
Avoid Scraping Images
Images are large and loaded with JavaScript, consuming extra bandwidth and slowing down scraping. Instead, focus on scraping text and other lighter elements.
Adapt to Changing HTML Tags
Google changes its HTML to improve user experience, which can break your scraper. Regularly test your parser to ensure it's working, and consider using a Google Search API to avoid dealing with HTML changes yourself.
Captcha Solving
Captchas differentiate humans from bots and can block your scraper. Use captcha-solving services sparingly, as they are slow and costly. Spread out your requests to reduce the chances of encountering captchas.
Conclusion
It can also be said that Google reviews affect the local SEO strategy in particular. It was noted that the number and relevance of reviews can affect the business’s ranking in the local searches. Increased ratings and favorable reviews tell search engines that the industry is credible and provides relevant goods and/or services to the particular locality, which in turn boosts its likelihood of ranking higher in SERPs. ReviewGators has extensive expertise in creating customized and best Google Maps scrapers to ease the extraction process. Therefore, Google reviews are purposefully maintained and utilized as business promotion tools in the sphere of online marketing to increase brand awareness, attract local clientele, and, consequently, increase sales and company performance.
Know more https://www.reviewgators.com/advanced-steps-to-scraping-google-reviews-for-decision-making.php
0 notes
Text
Google Search Results Data Scraping
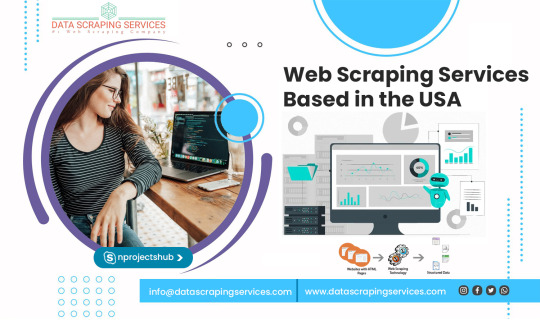
Google Search Results Data Scraping
Harness the Power of Information with Google Search Results Data Scraping Services by DataScrapingServices.com. In the digital age, information is king. For businesses, researchers, and marketing professionals, the ability to access and analyze data from Google search results can be a game-changer. However, manually sifting through search results to gather relevant data is not only time-consuming but also inefficient. DataScrapingServices.com offers cutting-edge Google Search Results Data Scraping services, enabling you to efficiently extract valuable information and transform it into actionable insights.
The vast amount of information available through Google search results can provide invaluable insights into market trends, competitor activities, customer behavior, and more. Whether you need data for SEO analysis, market research, or competitive intelligence, DataScrapingServices.com offers comprehensive data scraping services tailored to meet your specific needs. Our advanced scraping technology ensures you get accurate and up-to-date data, helping you stay ahead in your industry.
List of Data Fields
Our Google Search Results Data Scraping services can extract a wide range of data fields, ensuring you have all the information you need:
-Business Name: The name of the business or entity featured in the search result.
- URL: The web address of the search result.
- Website: The primary website of the business or entity.
- Phone Number: Contact phone number of the business.
- Email Address: Contact email address of the business.
- Physical Address: The street address, city, state, and ZIP code of the business.
- Business Hours: Business operating hours
- Ratings and Reviews: Customer ratings and reviews for the business.
- Google Maps Link: Link to the business’s location on Google Maps.
- Social Media Profiles: LinkedIn, Twitter, Facebook
These data fields provide a comprehensive overview of the information available from Google search results, enabling businesses to gain valuable insights and make informed decisions.
Benefits of Google Search Results Data Scraping
1. Enhanced SEO Strategy
Understanding how your website ranks for specific keywords and phrases is crucial for effective SEO. Our data scraping services provide detailed insights into your current rankings, allowing you to identify opportunities for optimization and stay ahead of your competitors.
2. Competitive Analysis
Track your competitors’ online presence and strategies by analyzing their rankings, backlinks, and domain authority. This information helps you understand their strengths and weaknesses, enabling you to adjust your strategies accordingly.
3. Market Research
Access to comprehensive search result data allows you to identify trends, preferences, and behavior patterns in your target market. This information is invaluable for product development, marketing campaigns, and business strategy planning.
4. Content Development
By analyzing top-performing content in search results, you can gain insights into what types of content resonate with your audience. This helps you create more effective and engaging content that drives traffic and conversions.
5. Efficiency and Accuracy
Our automated scraping services ensure you get accurate and up-to-date data quickly, saving you time and resources.
Best Google Data Scraping Services
Scraping Google Business Reviews
Extract Restaurant Data From Google Maps
Google My Business Data Scraping
Google Shopping Products Scraping
Google News Extraction Services
Scrape Data From Google Maps
Google News Headline Extraction
Google Maps Data Scraping Services
Google Map Businesses Data Scraping
Google Business Reviews Extraction
Best Google Search Results Data Scraping Services in USA
Dallas, Portland, Los Angeles, Virginia Beach, Fort Wichita, Nashville, Long Beach, Raleigh, Boston, Austin, San Antonio, Philadelphia, Indianapolis, Orlando, San Diego, Houston, Worth, Jacksonville, New Orleans, Columbus, Kansas City, Sacramento, San Francisco, Omaha, Honolulu, Washington, Colorado, Chicago, Arlington, Denver, El Paso, Miami, Louisville, Albuquerque, Tulsa, Springs, Bakersfield, Milwaukee, Memphis, Oklahoma City, Atlanta, Seattle, Las Vegas, San Jose, Tucson and New York.
Conclusion
In today’s data-driven world, having access to detailed and accurate information from Google search results can give your business a significant edge. DataScrapingServices.com offers professional Google Search Results Data Scraping services designed to meet your unique needs. Whether you’re looking to enhance your SEO strategy, conduct market research, or gain competitive intelligence, our services provide the comprehensive data you need to succeed. Contact us at [email protected] today to learn how our data scraping solutions can transform your business strategy and drive growth.
Website: Datascrapingservices.com
Email: [email protected]
#Google Search Results Data Scraping#Harness the Power of Information with Google Search Results Data Scraping Services by DataScrapingServices.com. In the digital age#information is king. For businesses#researchers#and marketing professionals#the ability to access and analyze data from Google search results can be a game-changer. However#manually sifting through search results to gather relevant data is not only time-consuming but also inefficient. DataScrapingServices.com o#enabling you to efficiently extract valuable information and transform it into actionable insights.#The vast amount of information available through Google search results can provide invaluable insights into market trends#competitor activities#customer behavior#and more. Whether you need data for SEO analysis#market research#or competitive intelligence#DataScrapingServices.com offers comprehensive data scraping services tailored to meet your specific needs. Our advanced scraping technology#helping you stay ahead in your industry.#List of Data Fields#Our Google Search Results Data Scraping services can extract a wide range of data fields#ensuring you have all the information you need:#-Business Name: The name of the business or entity featured in the search result.#- URL: The web address of the search result.#- Website: The primary website of the business or entity.#- Phone Number: Contact phone number of the business.#- Email Address: Contact email address of the business.#- Physical Address: The street address#city#state#and ZIP code of the business.#- Business Hours: Business operating hours#- Ratings and Reviews: Customer ratings and reviews for the business.
0 notes
Text
youtube
#Scrape Google Ratings#Google Ratings Scraping#Scrape Google Reviews#Google Reviews Scraping#Google Reviews scraper#Google Ratings Scraper#Youtube
0 notes
Text
A new tool lets artists add invisible changes to the pixels in their art before they upload it online so that if it’s scraped into an AI training set, it can cause the resulting model to break in chaotic and unpredictable ways.
The tool, called Nightshade, is intended as a way to fight back against AI companies that use artists’ work to train their models without the creator’s permission. Using it to “poison” this training data could damage future iterations of image-generating AI models, such as DALL-E, Midjourney, and Stable Diffusion, by rendering some of their outputs useless—dogs become cats, cars become cows, and so forth. MIT Technology Review got an exclusive preview of the research, which has been submitted for peer review at computer security conference Usenix.
AI companies such as OpenAI, Meta, Google, and Stability AI are facing a slew of lawsuits from artists who claim that their copyrighted material and personal information was scraped without consent or compensation. Ben Zhao, a professor at the University of Chicago, who led the team that created Nightshade, says the hope is that it will help tip the power balance back from AI companies towards artists, by creating a powerful deterrent against disrespecting artists’ copyright and intellectual property. Meta, Google, Stability AI, and OpenAI did not respond to MIT Technology Review’s request for comment on how they might respond.
Zhao’s team also developed Glaze, a tool that allows artists to “mask” their own personal style to prevent it from being scraped by AI companies. It works in a similar way to Nightshade: by changing the pixels of images in subtle ways that are invisible to the human eye but manipulate machine-learning models to interpret the image as something different from what it actually shows.
Continue reading article here
#Ben Zhao and his team are absolute heroes#artificial intelligence#plagiarism software#more rambles#glaze#nightshade#ai theft#art theft#gleeful dancing
22K notes
·
View notes
Text
How to Scrape Google Reviews Using Google Maps API & Python?
In the digital age, online reviews play a pivotal role in shaping consumer decisions. For businesses, understanding customer sentiment on platforms like Google Reviews is crucial. Harnessing the power of Python and the Google Maps API, we can automate the process of scraping Google Reviews to gain valuable insights. In this blog, we'll walk you through the steps to scrape Google Reviews efficiently.
Step 1: Set Up Your Google Cloud Platform (GCP) Account
Before diving into the code, you need to set up a Google Cloud Platform (GCP) account and create a new project. Enable the Google Maps JavaScript API and obtain an API key. This key acts as your passport to access Google Maps services.
Step 2: Install Required Libraries
Fire up your Python environment and install the necessary libraries. Use the following commands to install googlemaps and pandas:pip install googlemaps pip install pandas
These libraries will help you interact with the Google Maps API and manage data efficiently.
Step 3: Write the Python Script
Create a new Python script and import the required libraries. Initialize the Google Maps API client with your API key.import googlemaps import pandas as pd api_key = 'YOUR_API_KEY' gmaps = googlemaps.Client(key=api_key)
Step 4: Retrieve Place Details
Choose the location for which you want to scrape reviews. You'll need the place ID, which you can obtain using the places API.place_id = 'YOUR_PLACE_ID' place_details = gmaps.place(place_id=place_id, fields=['name', 'rating', 'reviews'])
Step 5: Extract and Store Reviews
Now, you can extract reviews from the obtained place details and store them in a pandas DataFrame for further analysis.reviews = place_details['reviews'] df_reviews = pd.DataFrame(reviews) df_reviews.to_csv('google_reviews.csv', index=False)
This snippet saves the reviews in a CSV file for easy access and sharing.
Step 6: Analyze and Visualize
With your reviews in hand, you can perform sentiment analysis, aggregate ratings, or visualize the data. Utilize Python's data manipulation and visualization tools to gain insights into customer sentiments.# Example: Calculate average rating average_rating = df_reviews['rating'].mean() print(f'Average Rating: {average_rating}')
Step 7: Respect Terms of Service
While scraping Google Reviews is powerful, it's crucial to respect Google's Terms of Service. Ensure that your usage complies with the policies to avoid any legal repercussions.
Conclusion
Scraping Google Reviews using the Google Maps API and Python opens up a world of possibilities for businesses and researchers. From understanding customer sentiments to making data-driven decisions, the insights gained can be invaluable. By following the steps outlined in this guide, you can embark on a journey of automating the extraction and analysis of Google Reviews, putting the power of Python and the Google Maps API to work for you.
Remember, ethical use and compliance with terms of service are paramount in the world of web scraping. Happy coding!
0 notes
Text
Google Play Data Scraper | Scrape Google Play Store Data
Are you looking to Scrape information from Google Play Data Scraper? Our Google Play Data Scraper can extract reviews, product descriptions, prices, merchant names, and merchant affiliation links from any country domain on Google SERP.

0 notes
Text
Extract Voluminous Real-Time Data With The Ready-To-Use Amazon Scraper
Automate the entire data scraping pipeline with a dynamic, comprehensive, and scalable Amazon scraper from ApiScrapy. You can fetch voluminous data including product prices, seller information, customer reviews, and bestseller ranking faster with ApiScrapy.
For more details visit: https://apiscrapy.com/amazon-scraper/
About AIMLEAP - Apiscrapy
Apiscrapy is a division of AIMLEAP, AIMLEAP is an ISO 9001:2015 and ISO/IEC 27001:2013 certified global technology consulting and service provider offering Digital IT, AI-augmented Data Solutions, Automation, and Research & Analytics Services.
AIMLEAP has been recognized as ‘The Great Place to Work®’. With focus on AI and an automation-first approach, our services include end-to-end IT application management, Mobile App Development, Data Management, Data Mining Services, Web Data Scraping, Self-serving BI reporting solutions, Digital Marketing, and Analytics solutions.
We started in 2012 and successfully delivered projects in IT & digital transformation, automation-driven data solutions, and digital marketing for more than 750 fast-growing companies in the USA, Europe, New Zealand, Australia, Canada; and more.
⭐An ISO 9001:2015 and ISO/IEC 27001:2013 certified
⭐Served 750+ customers
⭐ 11+ Years of industry experience
⭐98% Client Retention
⭐Great Place to Work® Certified
⭐ Global Delivery Centers in the USA, Canada, India & Australia
Email: [email protected]
USA: 1-30235 14656
Canada: +1 4378 370 063
India: +91 810 527 1615
Australia: +61 402 576 615
#amazonscarappertool#amazonscraper#amazon product data#amazon product api#amazon scraper#amazon#apiscrapy#scraping tool#google web crawler#free web crawler#scraping service#data as a service#amazon price api#amazon reviews api
0 notes
Text
Google App Store Reviews Scraper | Scraping Tools & Extension

Scrape Google Play Reviews Scraper and downloads them for datasets including name, text information, and date. Input the ID or URL of the apps as well as get information for all the reviews.Our Google App Store Reviews Scraper helps you extract data from Google App Store. Use Data Scraping Tools to scrape name, etc. in countries like USA,
know more : https://www.actowizsolutions.com/google-app-store-reviews-scraper.php
#Google Play Store Scraper#Google Play Store Reviews Scraper#Google Play Store Reviews Scraping Tools#Google Play Store Reviews Scraping Extension
0 notes
Text
Copyright takedowns are a cautionary tale that few are heeding

On July 14, I'm giving the closing keynote for the fifteenth HACKERS ON PLANET EARTH, in QUEENS, NY. Happy Bastille Day! On July 20, I'm appearing in CHICAGO at Exile in Bookville.
We're living through one of those moments when millions of people become suddenly and overwhelmingly interested in fair use, one of the subtlest and worst-understood aspects of copyright law. It's not a subject you can master by skimming a Wikipedia article!
I've been talking about fair use with laypeople for more than 20 years. I've met so many people who possess the unshakable, serene confidence of the truly wrong, like the people who think fair use means you can take x words from a book, or y seconds from a song and it will always be fair, while anything more will never be.
Or the people who think that if you violate any of the four factors, your use can't be fair – or the people who think that if you fail all of the four factors, you must be infringing (people, the Supreme Court is calling and they want to tell you about the Betamax!).
You might think that you can never quote a song lyric in a book without infringing copyright, or that you must clear every musical sample. You might be rock solid certain that scraping the web to train an AI is infringing. If you hold those beliefs, you do not understand the "fact intensive" nature of fair use.
But you can learn! It's actually a really cool and interesting and gnarly subject, and it's a favorite of copyright scholars, who have really fascinating disagreements and discussions about the subject. These discussions often key off of the controversies of the moment, but inevitably they implicate earlier fights about everything from the piano roll to 2 Live Crew to antiracist retellings of Gone With the Wind.
One of the most interesting discussions of fair use you can ask for took place in 2019, when the NYU Engelberg Center on Innovation Law & Policy held a symposium called "Proving IP." One of the panels featured dueling musicologists debating the merits of the Blurred Lines case. That case marked a turning point in music copyright, with the Marvin Gaye estate successfully suing Robin Thicke and Pharrell Williams for copying the "vibe" of Gaye's "Got to Give it Up."
Naturally, this discussion featured clips from both songs as the experts – joined by some of America's top copyright scholars – delved into the legal reasoning and future consequences of the case. It would be literally impossible to discuss this case without those clips.
And that's where the problems start: as soon as the symposium was uploaded to Youtube, it was flagged and removed by Content ID, Google's $100,000,000 copyright enforcement system. This initial takedown was fully automated, which is how Content ID works: rightsholders upload audio to claim it, and then Content ID removes other videos where that audio appears (rightsholders can also specify that videos with matching clips be demonetized, or that the ad revenue from those videos be diverted to the rightsholders).
But Content ID has a safety valve: an uploader whose video has been incorrectly flagged can challenge the takedown. The case is then punted to the rightsholder, who has to manually renew or drop their claim. In the case of this symposium, the rightsholder was Universal Music Group, the largest record company in the world. UMG's personnel reviewed the video and did not drop the claim.
99.99% of the time, that's where the story would end, for many reasons. First of all, most people don't understand fair use well enough to contest the judgment of a cosmically vast, unimaginably rich monopolist who wants to censor their video. Just as importantly, though, is that Content ID is a Byzantine system that is nearly as complex as fair use, but it's an entirely private affair, created and adjudicated by another galactic-scale monopolist (Google).
Google's copyright enforcement system is a cod-legal regime with all the downsides of the law, and a few wrinkles of its own (for example, it's a system without lawyers – just corporate experts doing battle with laypeople). And a single mis-step can result in your video being deleted or your account being permanently deleted, along with every video you've ever posted. For people who make their living on audiovisual content, losing your Youtube account is an extinction-level event:
https://www.eff.org/wp/unfiltered-how-youtubes-content-id-discourages-fair-use-and-dictates-what-we-see-online
So for the average Youtuber, Content ID is a kind of Kafka-as-a-Service system that is always avoided and never investigated. But the Engelbert Center isn't your average Youtuber: they boast some of the country's top copyright experts, specializing in exactly the questions Youtube's Content ID is supposed to be adjudicating.
So naturally, they challenged the takedown – only to have UMG double down. This is par for the course with UMG: they are infamous for refusing to consider fair use in takedown requests. Their stance is so unreasonable that a court actually found them guilty of violating the DMCA's provision against fraudulent takedowns:
https://www.eff.org/cases/lenz-v-universal
But the DMCA's takedown system is part of the real law, while Content ID is a fake law, created and overseen by a tech monopolist, not a court. So the fate of the Blurred Lines discussion turned on the Engelberg Center's ability to navigate both the law and the n-dimensional topology of Content ID's takedown flowchart.
It took more than a year, but eventually, Engelberg prevailed.
Until they didn't.
If Content ID was a person, it would be baby, specifically, a baby under 18 months old – that is, before the development of "object permanence." Until our 18th month (or so), we lack the ability to reason about things we can't see – this the period when small babies find peek-a-boo amazing. Object permanence is the ability to understand things that aren't in your immediate field of vision.
Content ID has no object permanence. Despite the fact that the Engelberg Blurred Lines panel was the most involved fair use question the system was ever called upon to parse, it managed to repeatedly forget that it had decided that the panel could stay up. Over and over since that initial determination, Content ID has taken down the video of the panel, forcing Engelberg to go through the whole process again.
But that's just for starters, because Youtube isn't the only place where a copyright enforcement bot is making billions of unsupervised, unaccountable decisions about what audiovisual material you're allowed to access.
Spotify is yet another monopolist, with a justifiable reputation for being extremely hostile to artists' interests, thanks in large part to the role that UMG and the other major record labels played in designing its business rules:
https://pluralistic.net/2022/09/12/streaming-doesnt-pay/#stunt-publishing
Spotify has spent hundreds of millions of dollars trying to capture the podcasting market, in the hopes of converting one of the last truly open digital publishing systems into a product under its control:
https://pluralistic.net/2023/01/27/enshittification-resistance/#ummauerter-garten-nein
Thankfully, that campaign has failed – but millions of people have (unwisely) ditched their open podcatchers in favor of Spotify's pre-enshittified app, so everyone with a podcast now must target Spotify for distribution if they hope to reach those captive users.
Guess who has a podcast? The Engelberg Center.
Naturally, Engelberg's podcast includes the audio of that Blurred Lines panel, and that audio includes samples from both "Blurred Lines" and "Got To Give It Up."
So – naturally – UMG keeps taking down the podcast.
Spotify has its own answer to Content ID, and incredibly, it's even worse and harder to navigate than Google's pretend legal system. As Engelberg describes in its latest post, UMG and Spotify have colluded to ensure that this now-classic discussion of fair use will never be able to take advantage of fair use itself:
https://www.nyuengelberg.org/news/how-explaining-copyright-broke-the-spotify-copyright-system/
Remember, this is the best case scenario for arguing about fair use with a monopolist like UMG, Google, or Spotify. As Engelberg puts it:
The Engelberg Center had an extraordinarily high level of interest in pursuing this issue, and legal confidence in our position that would have cost an average podcaster tens of thousands of dollars to develop. That cannot be what is required to challenge the removal of a podcast episode.
Automated takedown systems are the tech industry's answer to the "notice-and-takedown" system that was invented to broker a peace between copyright law and the internet, starting with the US's 1998 Digital Millennium Copyright Act. The DMCA implements (and exceeds) a pair of 1996 UN treaties, the WIPO Copyright Treaty and the Performances and Phonograms Treaty, and most countries in the world have some version of notice-and-takedown.
Big corporate rightsholders claim that notice-and-takedown is a gift to the tech sector, one that allows tech companies to get away with copyright infringement. They want a "strict liability" regime, where any platform that allows a user to post something infringing is liable for that infringement, to the tune of $150,000 in statutory damages.
Of course, there's no way for a platform to know a priori whether something a user posts infringes on someone's copyright. There is no registry of everything that is copyrighted, and of course, fair use means that there are lots of ways to legally reproduce someone's work without their permission (or even when they object). Even if every person who ever has trained or ever will train as a copyright lawyer worked 24/7 for just one online platform to evaluate every tweet, video, audio clip and image for copyright infringement, they wouldn't be able to touch even 1% of what gets posted to that platform.
The "compromise" that the entertainment industry wants is automated takedown – a system like Content ID, where rightsholders register their copyrights and platforms block anything that matches the registry. This "filternet" proposal became law in the EU in 2019 with Article 17 of the Digital Single Market Directive:
https://www.eff.org/deeplinks/2018/09/today-europe-lost-internet-now-we-fight-back
This was the most controversial directive in EU history, and – as experts warned at the time – there is no way to implement it without violating the GDPR, Europe's privacy law, so now it's stuck in limbo:
https://www.eff.org/deeplinks/2022/05/eus-copyright-directive-still-about-filters-eus-top-court-limits-its-use
As critics pointed out during the EU debate, there are so many problems with filternets. For one thing, these copyright filters are very expensive: remember that Google has spent $100m on Content ID alone, and that only does a fraction of what filternet advocates demand. Building the filternet would cost so much that only the biggest tech monopolists could afford it, which is to say, filternets are a legal requirement to keep the tech monopolists in business and prevent smaller, better platforms from ever coming into existence.
Filternets are also incapable of telling the difference between similar files. This is especially problematic for classical musicians, who routinely find their work blocked or demonetized by Sony Music, which claims performances of all the most important classical music compositions:
https://pluralistic.net/2021/05/08/copyfraud/#beethoven-just-wrote-music
Content ID can't tell the difference between your performance of "The Goldberg Variations" and Glenn Gould's. For classical musicians, the best case scenario is to have their online wages stolen by Sony, who fraudulently claim copyright to their recordings. The worst case scenario is that their video is blocked, their channel deleted, and their names blacklisted from ever opening another account on one of the monopoly platforms.
But when it comes to free expression, the role that notice-and-takedown and filternets play in the creative industries is really a sideshow. In creating a system of no-evidence-required takedowns, with no real consequences for fraudulent takedowns, these systems are huge gift to the world's worst criminals. For example, "reputation management" companies help convicted rapists, murderers, and even war criminals purge the internet of true accounts of their crimes by claiming copyright over them:
https://pluralistic.net/2021/04/23/reputation-laundry/#dark-ops
Remember how during the covid lockdowns, scumbags marketed junk devices by claiming that they'd protect you from the virus? Their products remained online, while the detailed scientific articles warning people about the fraud were speedily removed through false copyright claims:
https://pluralistic.net/2021/10/18/labor-shortage-discourse-time/#copyfraud
Copyfraud – making false copyright claims – is an extremely safe crime to commit, and it's not just quack covid remedy peddlers and war criminals who avail themselves of it. Tech giants like Adobe do not hesitate to abuse the takedown system, even when that means exposing millions of people to spyware:
https://pluralistic.net/2021/10/13/theres-an-app-for-that/#gnash
Dirty cops play loud, copyrighted music during confrontations with the public, in the hopes that this will trigger copyright filters on services like Youtube and Instagram and block videos of their misbehavior:
https://pluralistic.net/2021/02/10/duke-sucks/#bhpd
But even if you solved all these problems with filternets and takedown, this system would still choke on fair use and other copyright exceptions. These are "fact intensive" questions that the world's top experts struggle with (as anyone who watches the Blurred Lines panel can see). There's no way we can get software to accurately determine when a use is or isn't fair.
That's a question that the entertainment industry itself is increasingly conflicted about. The Blurred Lines judgment opened the floodgates to a new kind of copyright troll – grifters who sued the record labels and their biggest stars for taking the "vibe" of songs that no one ever heard of. Musicians like Ed Sheeran have been sued for millions of dollars over these alleged infringements. These suits caused the record industry to (ahem) change its tune on fair use, insisting that fair use should be broadly interpreted to protect people who made things that were similar to existing works. The labels understood that if "vibe rights" became accepted law, they'd end up in the kind of hell that the rest of us enter when we try to post things online – where anything they produce can trigger takedowns, long legal battles, and millions in liability:
https://pluralistic.net/2022/04/08/oh-why/#two-notes-and-running
But the music industry remains deeply conflicted over fair use. Take the curious case of Katy Perry's song "Dark Horse," which attracted a multimillion-dollar suit from an obscure Christian rapper who claimed that a brief phrase in "Dark Horse" was impermissibly similar to his song "A Joyful Noise."
Perry and her publisher, Warner Chappell, lost the suit and were ordered to pay $2.8m. While they subsequently won an appeal, this definitely put the cold grue up Warner Chappell's back. They could see a long future of similar suits launched by treasure hunters hoping for a quick settlement.
But here's where it gets unbelievably weird and darkly funny. A Youtuber named Adam Neely made a wildly successful viral video about the suit, taking Perry's side and defending her song. As part of that video, Neely included a few seconds' worth of "A Joyful Noise," the song that Perry was accused of copying.
In court, Warner Chappell had argued that "A Joyful Noise" was not similar to Perry's "Dark Horse." But when Warner had Google remove Neely's video, they claimed that the sample from "Joyful Noise" was actually taken from "Dark Horse." Incredibly, they maintained this position through multiple appeals through the Content ID system:
https://pluralistic.net/2020/03/05/warner-chappell-copyfraud/#warnerchappell
In other words, they maintained that the song that they'd told the court was totally dissimilar to their own was so indistinguishable from their own song that they couldn't tell the difference!
Now, this question of vibes, similarity and fair use has only gotten more intense since the takedown of Neely's video. Just this week, the RIAA sued several AI companies, claiming that the songs the AI shits out are infringingly similar to tracks in their catalog:
https://www.rollingstone.com/music/music-news/record-labels-sue-music-generators-suno-and-udio-1235042056/
Even before "Blurred Lines," this was a difficult fair use question to answer, with lots of chewy nuances. Just ask George Harrison:
https://en.wikipedia.org/wiki/My_Sweet_Lord
But as the Engelberg panel's cohort of dueling musicologists and renowned copyright experts proved, this question only gets harder as time goes by. If you listen to that panel (if you can listen to that panel), you'll be hard pressed to come away with any certainty about the questions in this latest lawsuit.
The notice-and-takedown system is what's known as an "intermediary liability" rule. Platforms are "intermediaries" in that they connect end users with each other and with businesses. Ebay and Etsy and Amazon connect buyers and sellers; Facebook and Google and Tiktok connect performers, advertisers and publishers with audiences and so on.
For copyright, notice-and-takedown gives platforms a "safe harbor." A platform doesn't have to remove material after an allegation of infringement, but if they don't, they're jointly liable for any future judgment. In other words, Youtube isn't required to take down the Engelberg Blurred Lines panel, but if UMG sues Engelberg and wins a judgment, Google will also have to pay out.
During the adoption of the 1996 WIPO treaties and the 1998 US DMCA, this safe harbor rule was characterized as a balance between the rights of the public to publish online and the interest of rightsholders whose material might be infringed upon. The idea was that things that were likely to be infringing would be immediately removed once the platform received a notification, but that platforms would ignore spurious or obviously fraudulent takedowns.
That's not how it worked out. Whether it's Sony Music claiming to own your performance of "Fur Elise" or a war criminal claiming authorship over a newspaper story about his crimes, platforms nuke first and ask questions never. Why not? If they ignore a takedown and get it wrong, they suffer dire consequences ($150,000 per claim). But if they take action on a dodgy claim, there are no consequences. Of course they're just going to delete anything they're asked to delete.
This is how platforms always handle liability, and that's a lesson that we really should have internalized by now. After all, the DMCA is the second-most famous intermediary liability system for the internet – the most (in)famous is Section 230 of the Communications Decency Act.
This is a 27-word law that says that platforms are not liable for civil damages arising from their users' speech. Now, this is a US law, and in the US, there aren't many civil damages from speech to begin with. The First Amendment makes it very hard to get a libel judgment, and even when these judgments are secured, damages are typically limited to "actual damages" – generally a low sum. Most of the worst online speech is actually not illegal: hate speech, misinformation and disinformation are all covered by the First Amendment.
Notwithstanding the First Amendment, there are categories of speech that US law criminalizes: actual threats of violence, criminal harassment, and committing certain kinds of legal, medical, election or financial fraud. These are all exempted from Section 230, which only provides immunity for civil suits, not criminal acts.
What Section 230 really protects platforms from is being named to unwinnable nuisance suits by unscrupulous parties who are betting that the platforms would rather remove legal speech that they object to than go to court. A generation of copyfraudsters have proved that this is a very safe bet:
https://www.techdirt.com/2020/06/23/hello-youve-been-referred-here-because-youre-wrong-about-section-230-communications-decency-act/
In other words, if you made a #MeToo accusation, or if you were a gig worker using an online forum to organize a union, or if you were blowing the whistle on your employer's toxic waste leaks, or if you were any other under-resourced person being bullied by a wealthy, powerful person or organization, that organization could shut you up by threatening to sue the platform that hosted your speech. The platform would immediately cave. But those same rich and powerful people would have access to the lawyers and back-channels that would prevent you from doing the same to them – that's why Sony can get your Brahms recital taken down, but you can't turn around and do the same to them.
This is true of every intermediary liability system, and it's been true since the earliest days of the internet, and it keeps getting proven to be true. Six years ago, Trump signed SESTA/FOSTA, a law that allowed platforms to be held civilly liable by survivors of sex trafficking. At the time, advocates claimed that this would only affect "sexual slavery" and would not impact consensual sex-work.
But from the start, and ever since, SESTA/FOSTA has primarily targeted consensual sex-work, to the immediate, lasting, and profound detriment of sex workers:
https://hackinghustling.org/what-is-sesta-fosta/
SESTA/FOSTA killed the "bad date" forums where sex workers circulated the details of violent and unstable clients, killed the online booking sites that allowed sex workers to screen their clients, and killed the payment processors that let sex workers avoid holding unsafe amounts of cash:
https://www.eff.org/deeplinks/2022/09/fight-overturn-fosta-unconstitutional-internet-censorship-law-continues
SESTA/FOSTA made voluntary sex work more dangerous – and also made life harder for law enforcement efforts to target sex trafficking:
https://hackinghustling.org/erased-the-impact-of-fosta-sesta-2020/
Despite half a decade of SESTA/FOSTA, despite 15 years of filternets, despite a quarter century of notice-and-takedown, people continue to insist that getting rid of safe harbors will punish Big Tech and make life better for everyday internet users.
As of now, it seems likely that Section 230 will be dead by then end of 2025, even if there is nothing in place to replace it:
https://energycommerce.house.gov/posts/bipartisan-energy-and-commerce-leaders-announce-legislative-hearing-on-sunsetting-section-230
This isn't the win that some people think it is. By making platforms responsible for screening the content their users post, we create a system that only the largest tech monopolies can survive, and only then by removing or blocking anything that threatens or displeases the wealthy and powerful.
Filternets are not precision-guided takedown machines; they're indiscriminate cluster-bombs that destroy anything in the vicinity of illegal speech – including (and especially) the best-informed, most informative discussions of how these systems go wrong, and how that blocks the complaints of the powerless, the marginalized, and the abused.
Support me this summer on the Clarion Write-A-Thon and help raise money for the Clarion Science Fiction and Fantasy Writers' Workshop!
If you'd like an essay-formatted version of this post to read or share, here's a link to it on pluralistic.net, my surveillance-free, ad-free, tracker-free blog:
https://pluralistic.net/2024/06/27/nuke-first/#ask-questions-never
Image: EFF https://www.eff.org/files/banner_library/yt-fu-1b.png
CC BY 3.0 https://creativecommons.org/licenses/by/3.0/deed.en
#pluralistic#vibe rights#230#section 230#cda 230#communications decency act#communications decency act 230#cda230#filternet#copyfight#fair use#notice and takedown#censorship#reputation management#copyfraud#sesta#fosta#sesta fosta#spotify#youtube#contentid#monopoly#free speech#intermediary liability
673 notes
·
View notes
Text
hrm, I see that Fandom wiki is slowly rolling out the Quick Answers module again, the thing that everyone across all sorts of fandom spaces were dunking on because it uses GenAI to scrape the articles to try to provide short answers to simple questions it supposes people have (and was getting things WILDLY wrong, and not even regular Fandom editors wanted this module)
it does not seem that wikis selected to have Quick Answers can opt out, and the current system is that there is a dashboard where editors with the appropriate user rights can review and correct the answers—and the Answers are auto-published after thirty days. Unclear if they will still auto-publish if nobody gets around to reviewing them.
the Quick Answers is specifically stated to make Fandom wikis "more relevant and accessible to Google and eventually enhance the SEO", so it's all just for SEO (and thus ultimately for ad revenue)
anyway, install Indie Wiki Buddy, check out their list of independent wikis, check out the Nintendo Indie Wiki Alliance and the Independent Wiki Federation, visit and support and contribute to the non-Fandom wikis if one exists for your topic area
#for anyone wondering why Encyclopedia Exandria can't really TRY to appear higher in Google results—this sort of thing is why#Fandom is specifically engineered for SEO and to game Google as much as possible even down to their infrastructure#they have teams and pages and “best practices” encouraging editors to edit in ways to play to SEO rather than to information building
238 notes
·
View notes
Text
Ok like nobody seems to have noticed but Juliette Blevins has recently put out a case that Great Andamanese might actually have been Austroasiatic all along (to complement Jarawan possibly being an Austronesian relative). There's some stuff that's certainly suggestive, but it'll be a bit more work needed before I'm ready to accept these 32 proposed correspondences as anything more than chance, particularly after the Indo-Vasconic debacle. Still, below the cut I'm going to try and give this a fair review.
All of this is from 'Linguistic clues to Andamanese pre-history: Understanding the North-South divide', in The Language of Hunter Gatherers, edited by Tom Güldemann, Patrick McConvell and Richard Rhodes and published in 2020 (a free version of the chapter can be found on Google Scholar).
Looking through the data, it actually seems relatively rigorous as a set of comparisons; she's done a shallow reconstruction of a Proto-Great-Andamanese from wordlists (seemingly a relatively trivial exercise, though with caveats noted below) and is seemingly comparing these to reconstructions from the Mon-Khmer comparative dictionary.
Many of the correspondences are basically identical between the two reconstructions with at most minimal semantic differences, e.g. (in the order PGA~PAA respectively) *buə 'clay' ~ *buəh 'ash, powdery dust'; *muən 'pus, dirt' ~ *muən 'pimple'; *cuər 'current, flow' ~ *cuər 'flow, pour'; *cuəp 'fasten, adjoin' ~ *bcuup/bcuəp 'adjoin, adhere'. However, I wonder if the Proto-GA reconstructions here have been massaged a bit to fit the Austroasiatic correspondence more closely; in Aka-Kede for example, each of these words shows a different vowel; pua, mine, cor(ie), cup. It's not fatal by any means (in fact if the correspondences could be shown to be more complex than simple identity that would actually help the argument), but definitely annoying.
There's a couple of PGA items which are presented as having a straightforward sound correpondence in PAA where the semantics is close but doesn't quite match, but also alongside a semantic match that differs slightly in sound, e.g. by a slightly different initial consonant, e.g. *raic 'bale out' ~ *raac 'sprinkle' /*saac 'bale out'; *pila 'tusk, tooth' ~ *plaaʔ 'blade'/*mlaʔ 'tusk, ivory'; *luk 'channel' ~ *ru(u)ŋ 'channel'/*lu(u)k 'have a hole'. I think there's possibly a plausible development here, with perhaps one form taking on the other's semantics because of taboo, or maybe due to an actual semantic shift (she notes that the Andamanese use boar tusks as scrapers, which could explain a 'blade'~'tusk' correspondence in itself).
There's an item which seems dubious on the PAA side, e.g. she proposes a correspondence *wət ~ *wət for 'bat, flying fox' but I can't find a *wət reconstructed anywhere in the MKCD with that meaning, not even in Bahnaric where she claims it comes from (there is a *wət reconstructed but with a meaning 'turn, bend'). Meanwhile, *kut 'fishing net' ~ *kuut 'tie, knot' seems wrong at first, as search for *kuut by itself only brings up a reconstruction *kuut 'scrape, scratch', however there is also a reconstruction *[c]kuut which does mean 'tie, knot'.
There's an interesting set of correspondences where PGA has a final schwa that's absent from the proposed PAA cognates, e.g. *lakə 'digging stick' ~ *lak 'hoe (v.)'; *ɲipə 'sandfly' ~ *jɔɔp 'horsefly'; *loŋə 'neck' ~ *tlu(u)ŋ 'throat'.
More generally, a substantial proportion of the proposed correspondences are nouns in Great Andamanese but verbs/adjective (stative verbs) in Austroasiatic, some of which are above, but also including e.g. *cuiɲ 'odour' ~ *ɟhuuɲ/ɟʔuuɲ 'smell, sniff'; *raic 'juice' ~ *raac 'sprinkle' (a separate correspondence to 'bale out' above); *mulə 'egg' ~ *muul 'round'; *ciəp 'belt, band' ~ *cuup/cuəp/ciəp 'wear, put on'. This also doesn't seem too much of an issue, given the general word-class flexibility in that part of the world, though there don't seem to be any correspondences going the other way, which could perhaps be a sign of loaning/relexification instead.
I mentioned that a lot of these seem to be exact matches, but of course what you really want to indicate relatedness are non-indentical but regular correspondences, and here is where I can see the issues probably starting to really arise. We've already noted some of the vowel issues, but we also have some messiness with some of the consonants, though at the very least the POA matches pretty much every time (including reasonable caveats like sibilants patterning with palatals and the like). However, that still leaves us with some messes.
The liquids and coronals especially are misaligned a fair bit in ways which could do with more correspondences to flesh out. Here's a list of the correspondences found in initial position in the examples given.
*l ~ *l: *lat ~ *[c]laat 'fear', *lakə 'digging stick' ~ *lak 'hoe'
*l ~ *r: *lap ~ *rap 'count' (*luk 'channel' ~ *ru(u)ŋ 'have a hole'/*lu(u)k 'channel' could be in either of these)
*r ~ *r: *raic 'juice' ~ *raac 'sprinkle'
*r ~ *ɗ: *rok ~ *ɗuk 'canoe'
*t ~ *ɗ: *tapə 'blind' ~ [ɟ]ɗaap 'pass hand along'
*t ~ *t: *ar-təm ~ *triəm 'old' (suggested that metathesis occurred, though to me there probably would need to be some reanalysis as well to make this work)
I invite any of my mutuals more experienced with the comparative method to have a look for yourselves and see what you make of the proposal as it currently stands. It would certainly be an interesting development if more actual correspondences could be set up, though I do have to wonder if more work would also be needed on Austroasiatic to double-check these reconstructions as well.
73 notes
·
View notes
Text
How Easy Is It To Scrape Google Reviews?
Have you ever wished you could put together all the Google reviews? Those insightful glimpses into customer experiences will fuel product development and skyrocket sales. But manually combing through endless reviews can be a tiring job. What if there was a way to automate this review roundup and unlock the data in minutes? This blog delves deep into the world of scraping Google Reviews, showing you how to transform this tedious task into a quick and easy win, empowering you to harness the power of customer feedback.
What is Google Review Scraping?
Google Reviews are very useful for both businesses and customers. They provide important information about customers' thoughts and can greatly affect how people see a brand.
It's the process of automatically collecting data from Google Reviews on businesses. Instead of manual copy paste work, scraping tools extract information like:
Review text
Star rating
Reviewer name (if available)
Date of review
Google Review Scraper
A Google Review scraper is a program that automatically gathers information about different businesses from Google Reviews.
Here's how Google Review Scraper works:
You provide the scraper with the URL of a specific business listing on Google Maps. This tells the scraper exactly where to find the reviews you're interested in.
The scraper acts like a web browser, but it analyses the underlying website structure instead of displaying the information for you.
The scraper finds the parts that have the review details you want, like the words in the review, the number of stars given, the name of the person who wrote it (if it's there), and the date of the review.
After the scraper collects all the important information, it puts it into a simple format, like a CSV file, which is easy to look at and work within spreadsheets or other programs for more detailed study.
Google Review Scraping using Python
Google discourages web scraping practices that overload their servers or violate user privacy. While it's possible to scrape Google Reviews with Python, it's important to prioritize ethical practices and respect their Terms of Service (TOS). Here's an overview:
Choose a Web Scraping Library
Popular options for Python include Beautiful Soup and Selenium. Beautiful Soup excels at parsing HTML content, while Selenium allows more browser-like interaction, which can help navigate dynamic content on Google.
Understand Google's Structure
Using browser developer tools, inspect the HTML structure of a Google business listing with reviews. Identify the elements containing the needed review data (text, rating, etc.). Identify the HTML tags associated with this data.
Write Python Script
Import the necessary libraries (requests, Beautiful Soup).
Define a function to take a business URL as input.
Use requests to fetch the HTML content of the business listing.
Use Beautiful Soup to parse the HTML content and navigate to the sections containing review elements using the identified HTML tags (e.g., CSS selectors).
Extract the desired data points (text, rating, name, date) and store them in a list or dictionary.
Consider using loops to iterate through multiple pages of reviews if pagination exists.
Finally, write the extracted data to a file (CSV, JSON) for further analysis.
Alternate Methods to Scrape Google Reviews
Though using web scraping libraries is the most common approach, it does involve coding knowledge and is complex to maintain. Here are some of the alternative approaches with no or minimal coding knowledge.
Google Maps API (Limited Use):
Google offers a Maps Platform with APIs for authorized businesses. This might be a suitable option if you need to review data for specific locations you manage. However, it requires authentication and might not be suitable for large-scale scraping of public reviews.
Pre-built Scraping Tools
Several online tools offer scraping functionalities for Google Reviews. These tools often have user-friendly interfaces and handle complexities like dynamic content. Consider options with clear pricing structures and responsible scraping practices.
Cloud-Based Scraping Services
Cloud services use their own servers to collect data from the web, which helps prevent problems with too much traffic on Google's servers and reduces the risk of your own IP address getting blocked. They have tools that deal with website navigation, where to keep the data, and changing the internet address so you don't get caught. Usually, you pay for these services based on how much you use them.
Google My Business Reviews (For Your Business):
If you want to analyze reviews for your business, Google My Business offers built-in review management tools. This platform lets you access, respond to, and analyze reviews directly. However, this approach only works for your business and doesn't offer access to reviews of competitors.
Partner with Data Providers
Certain data providers might offer access to Google Review datasets. This can be a good option if you need historical data or a broader range of reviews. Limited access might exist, and data acquisition might involve costs. Research the data source and ensure ethical data collection practices.
Understanding the Challenges
Scraping Google reviews can be a valuable way to gather data for analysis, but it comes with its own set of hurdles. Here's a breakdown of the common challenges you might face:
Technical Challenges
Changing Layouts and Algorithms Google frequently updates its website design and search algorithms. This can break your scraper if it relies on specific HTML elements or patterns that suddenly change.
Bot Detection Mechanisms Google has sophisticated systems in place to identify and block automated bots. These can include CAPTCHAs, IP bans, and browser fingerprinting, making it difficult for your scraper to appear as a legitimate user.
Dynamic Content A lot of Google reviews use JavaScript to load content dynamically. This means simply downloading the static HTML of a page might not be enough to capture all the reviews.
Data Access Challenges
Rate Limiting Google limits the frequency of requests from a single IP address within a certain timeframe, exceeding can lead to temporary blocks, hindering your scraping process.
Legality and Ethics Google's terms of service forbid scraping their content without permission. It's important to be aware of the legal and ethical implications before scraping Google reviews.
Data Quality Challenges
Personalization Google personalizes search results, including reviews, based on factors like location and search history. This means the data you scrape might not be representative of a broader audience.
Data Structuring Google review pages contain a lot of information beyond the reviews themselves, like ads and related searches. Extracting the specific data points you need (reviewer name, rating, etc.) can be a challenge.
Incomplete Data Your scraper might miss reviews that are loaded after the initial page load or hidden behind "See more" buttons.
If you decide to scrape Google reviews, be prepared to adapt your scraper to these challenges and prioritize ethical considerations. Scraping a small number of reviews might be manageable, but scaling up to scrape a large amount of data can be complex and resource-intensive.
Conclusion
While Google Review scraping offers a treasure trove of customer insights, navigating its technical and ethical complexities can be a real adventure. Now, you don't have to go it alone. Third-party data providers like ReviewGators can be your knight in shining armour. They handle the scraping responsibly and efficiently, ensuring you get high-quality, compliant data.
This frees you to focus on what truly matters: understanding your customers and leveraging their feedback to take your business to the next level.
Know more https://www.reviewgators.com/how-to-scrape-google-reviews.php
0 notes
Text
If only the warfare that nearly wiped out humanity had actually finished the job. Then Dev and the other remaining genetically Altered supersoldiers wouldn't be facing what could be their final days scraping by. They went from science experiments to vermin and today is the last straw. Their plan to finally end the fighting backfires, and now they face an even more frightening reality. The new human leader, Alessandra, doesn't want them dead. She needs their help. Dev isn't sure if his decision to help her will save them... or get them all killed.
Bound to Ashes (originally released in 2014) is a fast-paced, character-driven post-apocalyptic sci-fi novel (~90k words) about learning to trust and doing what's right even though no right has ever been done to you.
Status: OPEN for Beta Reading and FREE. (Link goes to the Google Doc folder.) Check out the additional document for feedback guidelines.
Reviews and more under the cut.
Content warning for language and violence.

I love post-apocalyptic settings. The idea of humanity as it is now getting a "reset" is compelling. But I was disappointed by the vast majority of post-apoc media rife with misogyny, alpha male kitsch, and grimdark nihilism. I wanted characters that felt the hopelessness of the world but still chose to be better. I wrote BtA to be the change.
BtA was my first serious writing project when I was 21, back in '12. Since then it has gone through 10 drafts, a few serious beta readers, a self-publishing, an un-self-publishing, and a last polish this year (2024) to finalize series-wide changes.
Here's what readers have said about Bound to Ashes:
"Bound to Ashes is everything I wish Maze Runner was."
"It took me three sentences to fall in love with this book, and it kept me hooked until the very end. Amazing read that I will be passing along to my friends."
"The mental images projected were vibrant and intense, and had me in tears in a bath."
#indie author#indie books#free books#ebook#wattpad#original story#original characters#writing#fiction writing#post apocalyptic#post apocalypse#post-apocalyptic#original fiction#original work#supersoldier#furry#furry book#furry author
33 notes
·
View notes
Text
This project is unfinished and will remain that way. There are bugs. Not all endings are implemented. The ending tracker doesn't work. Images are broken. Nothing will be fixed. There's still quite a bit of content, though, so I am releasing what's here as is.
Tilted Sands is a project I started back when AI Dungeon first came out--the very early version you had to run in a Google colabs notebook. Sometime in late 2018, I think? I was a contributor at Botnik Studios at the time and I was delighted by AI Dungeon, but I knew it would never be a truly satisfying choose your own adventure generator on its own. I would argue that the modern AI Dungeon 2 and NovelAI don't fully function as such even now. That's not how AI works. It has to be guided heavily, the product has to be sculpted by human hands.
Anyway, it inspired me to use Transformer--a GPT2 predictive text writing tool--to craft a more coherent and polished but still silly and definitely AI-flavored CYOA experience. It was an ambitious project, but I was experienced with writing what I like to call "cyborg" pieces--meaning the finished product is, in a way, made by both an AI/algorithm/other bot AND a human writer. Something strange and wonderful that could not have been made by the bot alone, nor by the human writer alone. Algorithms can surprise us and trigger our creative human minds to move in directions we never would've thought to go in otherwise. To me, that's what actual AI art is: a human engaging in a creative activity like writing in a way that also includes utilizing an algorithm of some sort. The results are always fascinating, strangely insightful, and sometimes beautiful.
I worked on Tilted Sands off-and-on for a couple years, and then the entire AI landscape changed practically overnight with DALL-E and ChatGPT. And I soon realized that I cannot continue working on this project. Mainstream, corporate AI is disgustingly unethical and I don't want the predictive text writing I used to enjoy so much to be associated with "AI art". It's not. Before DALL-E and ChatGPT, there were artists and writers who made art by utilizing algorithms, neural networks, etc. Some things were perhaps in an ethical or legal grey area, but people actually did care about that. I remember discussing "would it be ethical to scrape [x]?" with other writers, and sharing databases of things like commercial advertising scripts and public domain content. I liked using mismatched databases to write things, like a corpus of tech product reviews that I used to write a song. The line between transformative art and fair use vs theft was constantly on all of our minds, because we were artists ourselves.
All of the artists and writers I knew in those days who made "cyborg art" have stopped by now. Including me.
But I poured a lot of love and thought and energy into this silly little project, and the thought of leaving it to rot on my hard drive hurt too much. It's not done, but there's a lot there--over 14,000 words, multiple endings and game over scenarios. I had so much fun with it and I wanted to complete it, but I can't. I don't want it to be associated in any way with the current "AI art" scene. It's not.
Please consider this my love letter to what technology-augmented art used to be, and what AI art could have been.
I know I'm not the only one mourning this brief but intense period from about 2014-2019 in which human creativity and developing AI technology combined organically to create an array of beautiful, stupid, silly, terrible, wonderful works of art. If you're also feeling sad and nostalgic about it, I hope you find this silly game enjoyable even in its unfinished state.
In conclusion:
Fuck capitalism, fuck what is currently called AI art, fuck ChatGPT, fuck every company taking advantage of artists and writers and other creative types by using AI.
24 notes
·
View notes
Text
In this blog, we will uncover the meaning of Google reviews and their importance. Then this blog will guide you through the process of extracting Google Reviews using Python and the Google Maps API.
For More Information:-
0 notes
Text
Google Play Data Scraper | Scrape Google Play Store Data
Are you looking to Scrape information from Google Play Data Scraper? Our Google Play Data Scraper can extract reviews, product descriptions, prices, merchant names, and merchant affiliation links from any country domain on Google SERP.
0 notes