#R programming
Explore tagged Tumblr posts
Text
R Programming Resources
These are the resources I took from my bookmark folder I made like months ago when I wanted to learn R but… I didn’t 😅 Got distracted with other languages! Anyhoo here are some resources plus some new ones I found!
Websites
R-Bloggers R Tutorials - LINK
Stat Method R Tutorials - LINK
W3School - LINK
Tutorialspoint - LINK
Guru99 - LINK
R-Exercises - LINK
Code-School - LINK
R for Data Science - LINK
Dataquest - LINK
Books (PDF)
The Art Of R Programming - LINK
A First Course In Statistical Programming With R - LINK
The Book Of R - LINK
R Programming For Data Science - LINK
R For Beginners - LINK
R Programming [Tutorialspoint] - LINK
Hands-on Programming With R - LINK
YouTube Videos
R Programming Tutorial for Beginners - LINK
R Programming Basics - Data Science and Machine Learning Tutorial - LINK
Introduction to R for Data Science - Data Analysis - LINK
R Programming for Data Science - Full Course - LINK
R Programming Tutorial for Beginners (Simplilearn) - LINK
Miscs
R Cheatsheet - LINK
Hope this helps!! 💻👍🏾💗
#submission ask#my asks#resources#r programming#r programmer#programming#coding#studyblr#codeblr#progblr#studying#comp sci#programmer#student#study life#coding resources#coding study
414 notes
·
View notes
Text
Me: *forgets a double quote*
R Studio:
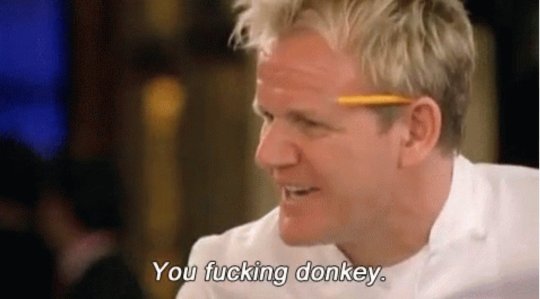
28 notes
·
View notes
Text
Learning R as a regular coder. Wish me luck!
11 notes
·
View notes
Text
Something you should know about me is that I can go rather far to commit to a bit.
I saw this video recently and was inspired for my capstone project (Statistics Major)
By recently I mean last night at 8pm (20:00) with my presentation of this project at 9:30 am today. However I decided it was too great to pass up so hear I am.
My presentation was on bootstrap stats which is used to increase sample size when otherwise unable to. This is supposed to give more accurate sample statistics.
First things first: Acquiring the Spaghettios A-Z
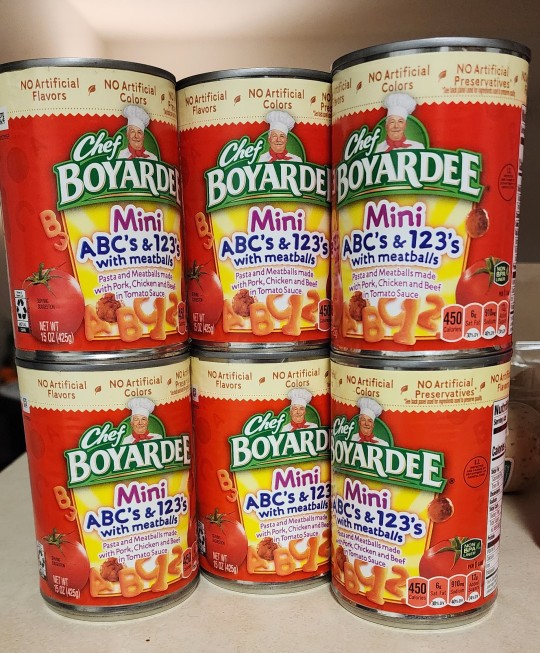
Unfortunately the ones he bought in the video were not in stock so I got these. As you can see these also have numbers. So I had to sort them both alphabetically and remove the meatballs and numbers. This process took about 2 hours with 2 people (me doing 4 cans my partner doing 2)
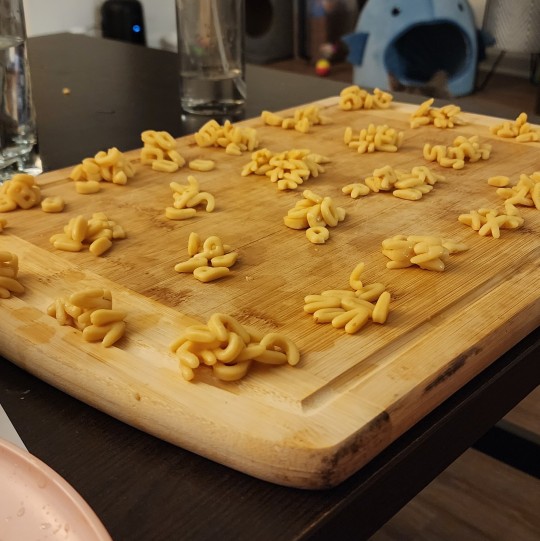
This is what my spreadsheet looked like at the end
I then went online and got the full movie script for Shrek that lacked image descriptions and who said what (aka just the dialog).
Using R studio I found the amount of each letter in the Shrek script.
A 2061, B 431, C 459, D 925, E 3208, F 504, G 691, H 1663, I 1844, J 62, K 535, L 1225, M 717, N 1771, O 2678, P 358, Q 37, R 1511, 2 1600, T 2552, U 1142, V 242, W 779, X 20, Y 1046, Z 9.
I then found the averages for each letter in my 6 cans combined (rounded up). From this I divided the Shrek script by the cans to find how many cans it would take per letter. Then I found the letter with the highest amount of cans needed and thus had my answer (268).
Now I did the bootstrapping. This is basically taking random numbers from my dataset (with replacement) and assigning them to random letters in a new row. I did this and created 3 new datasets. One with 120 entries per letter, one with 1,200, and the last one with 12,000. I then repeated the process that I did with the first can to find the amount of cans needed (256, 268, and 256 respectively).
So from this I can conclude that it would take 256-268 cans to recreate the Shrek script.
#statistics#r#r studio#r programming#spaghettios#bit#silly idea#capstone#bootstrap statistics#Not kidding I finished this 10 minutes before I had to present#I slept a bit last night too#I had nightmares of my data both becoming people and sacks of rice#blame the rice part on my partners mom#then when my alarms went off#I turned off the first the panicking about my sentient data#so yeah#weird#college#student
13 notes
·
View notes
Text
Making barplots and histograms in R but colouring them hotpink because it’s all I have left.
20 notes
·
View notes
Text
I may be horrendous at stats but at least I have the power to make all my graphs bisexual themed so my lecturer is forced to read my garbage while also being involuntarily subscribed to the queer agenda. not sorry :) in fact feeling very devilish about it :)
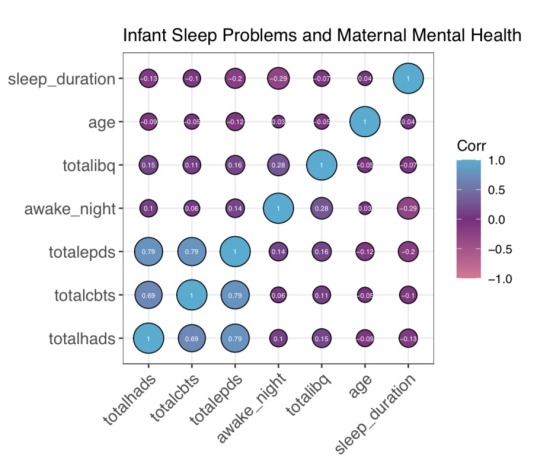
23 notes
·
View notes
Text
Exploring Data Science Tools: My Adventures with Python, R, and More
Welcome to my data science journey! In this blog post, I'm excited to take you on a captivating adventure through the world of data science tools. We'll explore the significance of choosing the right tools and how they've shaped my path in this thrilling field.
Choosing the right tools in data science is akin to a chef selecting the finest ingredients for a culinary masterpiece. Each tool has its unique flavor and purpose, and understanding their nuances is key to becoming a proficient data scientist.
I. The Quest for the Right Tool
My journey began with confusion and curiosity. The world of data science tools was vast and intimidating. I questioned which programming language would be my trusted companion on this expedition. The importance of selecting the right tool soon became evident.
I embarked on a research quest, delving deep into the features and capabilities of various tools. Python and R emerged as the frontrunners, each with its strengths and applications. These two contenders became the focus of my data science adventures.
II. Python: The Swiss Army Knife of Data Science
Python, often hailed as the Swiss Army Knife of data science, stood out for its versatility and widespread popularity. Its extensive library ecosystem, including NumPy for numerical computing, pandas for data manipulation, and Matplotlib for data visualization, made it a compelling choice.
My first experiences with Python were both thrilling and challenging. I dove into coding, faced syntax errors, and wrestled with data structures. But with each obstacle, I discovered new capabilities and expanded my skill set.
III. R: The Statistical Powerhouse
In the world of statistics, R shines as a powerhouse. Its statistical packages like dplyr for data manipulation and ggplot2 for data visualization are renowned for their efficacy. As I ventured into R, I found myself immersed in a world of statistical analysis and data exploration.
My journey with R included memorable encounters with data sets, where I unearthed hidden insights and crafted beautiful visualizations. The statistical prowess of R truly left an indelible mark on my data science adventure.
IV. Beyond Python and R: Exploring Specialized Tools
While Python and R were my primary companions, I couldn't resist exploring specialized tools and programming languages that catered to specific niches in data science. These tools offered unique features and advantages that added depth to my skill set.
For instance, tools like SQL allowed me to delve into database management and querying, while Scala opened doors to big data analytics. Each tool found its place in my toolkit, serving as a valuable asset in different scenarios.
V. The Learning Curve: Challenges and Rewards
The path I took wasn't without its share of difficulties. Learning Python, R, and specialized tools presented a steep learning curve. Debugging code, grasping complex algorithms, and troubleshooting errors were all part of the process.
However, these challenges brought about incredible rewards. With persistence and dedication, I overcame obstacles, gained a profound understanding of data science, and felt a growing sense of achievement and empowerment.
VI. Leveraging Python and R Together
One of the most exciting revelations in my journey was discovering the synergy between Python and R. These two languages, once considered competitors, complemented each other beautifully.
I began integrating Python and R seamlessly into my data science workflow. Python's data manipulation capabilities combined with R's statistical prowess proved to be a winning combination. Together, they enabled me to tackle diverse data science tasks effectively.
VII. Tips for Beginners
For fellow data science enthusiasts beginning their own journeys, I offer some valuable tips:
Embrace curiosity and stay open to learning.
Work on practical projects while engaging in frequent coding practice.
Explore data science courses and resources to enhance your skills.
Seek guidance from mentors and engage with the data science community.
Remember that the journey is continuous—there's always more to learn and discover.
My adventures with Python, R, and various data science tools have been transformative. I've learned that choosing the right tool for the job is crucial, but versatility and adaptability are equally important traits for a data scientist.
As I summarize my expedition, I emphasize the significance of selecting tools that align with your project requirements and objectives. Each tool has a unique role to play, and mastering them unlocks endless possibilities in the world of data science.
I encourage you to embark on your own tool exploration journey in data science. Embrace the challenges, relish the rewards, and remember that the adventure is ongoing. May your path in data science be as exhilarating and fulfilling as mine has been.
Happy data exploring!
21 notes
·
View notes
Text
fuck horoscopes and mbtis, what's your favourite R package
#programmer humor#r programming#biostatistics#bioinformatics#programming#helix talks#ggplot2#my absolute beloved#i just think its neat#trying to plot stuff with base r is suffering to me idk why#also karthik wesanderson and rcolorbrewer because heehee now your graphs can be COLOURFUL
41 notes
·
View notes
Text
Learning About Different Types of Functions in R Programming
Summary: Learn about the different types of functions in R programming, including built-in, user-defined, anonymous, recursive, S3, S4 methods, and higher-order functions. Understand their roles and best practices for efficient coding.
Introduction
Functions in R programming are fundamental building blocks that streamline code and enhance efficiency. They allow you to encapsulate code into reusable chunks, making your scripts more organised and manageable.
Understanding the various types of functions in R programming is crucial for leveraging their full potential, whether you're using built-in, user-defined, or advanced methods like recursive or higher-order functions.
This article aims to provide a comprehensive overview of these different types, their uses, and best practices for implementing them effectively. By the end, you'll have a solid grasp of how to utilise these functions to optimise your R programming projects.
What is a Function in R?
In R programming, a function is a reusable block of code designed to perform a specific task. Functions help organise and modularise code, making it more efficient and easier to manage.
By encapsulating a sequence of operations into a function, you can avoid redundancy, improve readability, and facilitate code maintenance. Functions take inputs, process them, and return outputs, allowing for complex operations to be performed with a simple call.
Basic Structure of a Function in R
The basic structure of a function in R includes several key components:
Function Name: A unique identifier for the function.
Parameters: Variables listed in the function definition that act as placeholders for the values (arguments) the function will receive.
Body: The block of code that executes when the function is called. It contains the operations and logic to process the inputs.
Return Statement: Specifies the output value of the function. If omitted, R returns the result of the last evaluated expression by default.
Here's the general syntax for defining a function in R:
Syntax and Example of a Simple Function
Consider a simple function that calculates the square of a number. This function takes one argument, processes it, and returns the squared value.
In this example:
square_number is the function name.
x is the parameter, representing the input value.
The body of the function calculates x^2 and stores it in the variable result.
The return(result) statement provides the output of the function.
You can call this function with an argument, like so:
This function is a simple yet effective example of how you can leverage functions in R to perform specific tasks efficiently.
Must Read: R Programming vs. Python: A Comparison for Data Science.
Types of Functions in R
In R programming, functions are essential building blocks that allow users to perform operations efficiently and effectively. Understanding the various types of functions available in R helps in leveraging the full power of the language.
This section explores different types of functions in R, including built-in functions, user-defined functions, anonymous functions, recursive functions, S3 and S4 methods, and higher-order functions.
Built-in Functions
R provides a rich set of built-in functions that cater to a wide range of tasks. These functions are pre-defined and come with R, eliminating the need for users to write code for common operations.
Examples include mathematical functions like mean(), median(), and sum(), which perform statistical calculations. For instance, mean(x) calculates the average of numeric values in vector x, while sum(x) returns the total sum of the elements in x.
These functions are highly optimised and offer a quick way to perform standard operations. Users can rely on built-in functions for tasks such as data manipulation, statistical analysis, and basic operations without having to reinvent the wheel. The extensive library of built-in functions streamlines coding and enhances productivity.
User-Defined Functions
User-defined functions are custom functions created by users to address specific needs that built-in functions may not cover. Creating user-defined functions allows for flexibility and reusability in code. To define a function, use the function() keyword. The syntax for creating a user-defined function is as follows:
In this example, my_function takes two arguments, arg1 and arg2, adds them, and returns the result. User-defined functions are particularly useful for encapsulating repetitive tasks or complex operations that require custom logic. They help in making code modular, easier to maintain, and more readable.
Anonymous Functions
Anonymous functions, also known as lambda functions, are functions without a name. They are often used for short, throwaway tasks where defining a full function might be unnecessary. In R, anonymous functions are created using the function() keyword without assigning them to a variable. Here is an example:
In this example, sapply() applies the anonymous function function(x) x^2 to each element in the vector 1:5. The result is a vector containing the squares of the numbers from 1 to 5.
Anonymous functions are useful for concise operations and can be utilised in functions like apply(), lapply(), and sapply() where temporary, one-off computations are needed.
Recursive Functions
Recursive functions are functions that call themselves in order to solve a problem. They are particularly useful for tasks that can be divided into smaller, similar sub-tasks. For example, calculating the factorial of a number can be accomplished using recursion. The following code demonstrates a recursive function for computing factorial:
Here, the factorial() function calls itself with n - 1 until it reaches the base case where n equals 1. Recursive functions can simplify complex problems but may also lead to performance issues if not implemented carefully. They require a clear base case to prevent infinite recursion and potential stack overflow errors.
S3 and S4 Methods
R supports object-oriented programming through the S3 and S4 systems, each offering different approaches to object-oriented design.
S3 Methods: S3 is a more informal and flexible system. Functions in S3 are used to define methods for different classes of objects. For instance:
In this example, print.my_class is a method that prints a custom message for objects of class my_class. S3 methods provide a simple way to extend functionality for different object types.
S4 Methods: S4 is a more formal and rigorous system with strict class definitions and method dispatch. It allows for detailed control over method behaviors. For example:
Here, setClass() defines a class with a numeric slot, and setMethod() defines a method for displaying objects of this class. S4 methods offer enhanced functionality and robustness, making them suitable for complex applications requiring precise object-oriented programming.
Higher-Order Functions
Higher-order functions are functions that take other functions as arguments or return functions as results. These functions enable functional programming techniques and can lead to concise and expressive code. Examples include apply(), lapply(), and sapply().
apply(): Used to apply a function to the rows or columns of a matrix.
lapply(): Applies a function to each element of a list and returns a list.
sapply(): Similar to lapply(), but returns a simplified result.
Higher-order functions enhance code readability and efficiency by abstracting repetitive tasks and leveraging functional programming paradigms.
Best Practices for Writing Functions in R
Writing efficient and readable functions in R is crucial for maintaining clean and effective code. By following best practices, you can ensure that your functions are not only functional but also easy to understand and maintain. Here are some key tips and common pitfalls to avoid.
Tips for Writing Efficient and Readable Functions
Keep Functions Focused: Design functions to perform a single task or operation. This makes your code more modular and easier to test. For example, instead of creating a function that processes data and generates a report, split it into separate functions for processing and reporting.
Use Descriptive Names: Choose function names that clearly indicate their purpose. For instance, use calculate_mean() rather than calc() to convey the function’s role more explicitly.
Avoid Hardcoding Values: Use parameters instead of hardcoded values within functions. This makes your functions more flexible and reusable. For example, instead of using a fixed threshold value within a function, pass it as a parameter.
Common Mistakes to Avoid
Overcomplicating Functions: Avoid writing overly complex functions. If a function becomes too long or convoluted, break it down into smaller, more manageable pieces. Complex functions can be harder to debug and understand.
Neglecting Error Handling: Failing to include error handling can lead to unexpected issues during function execution. Implement checks to handle invalid inputs or edge cases gracefully.
Ignoring Code Consistency: Consistency in coding style helps maintain readability. Follow a consistent format for indentation, naming conventions, and comment style.
Best Practices for Function Documentation
Document Function Purpose: Clearly describe what each function does, its parameters, and its return values. Use comments and documentation strings to provide context and usage examples.
Specify Parameter Types: Indicate the expected data types for each parameter. This helps users understand how to call the function correctly and prevents type-related errors.
Update Documentation Regularly: Keep function documentation up-to-date with any changes made to the function’s logic or parameters. Accurate documentation enhances the usability of your code.
By adhering to these practices, you’ll improve the quality and usability of your R functions, making your codebase more reliable and easier to maintain.
Read Blogs:
Pattern Programming in Python: A Beginner’s Guide.
Understanding the Functional Programming Paradigm.
Frequently Asked Questions
What are the main types of functions in R programming?
In R programming, the main types of functions include built-in functions, user-defined functions, anonymous functions, recursive functions, S3 methods, S4 methods, and higher-order functions. Each serves a specific purpose, from performing basic tasks to handling complex operations.
How do user-defined functions differ from built-in functions in R?
User-defined functions are custom functions created by users to address specific needs, whereas built-in functions come pre-defined with R and handle common tasks. User-defined functions offer flexibility, while built-in functions provide efficiency and convenience for standard operations.
What is a recursive function in R programming?
A recursive function in R calls itself to solve a problem by breaking it down into smaller, similar sub-tasks. It's useful for problems like calculating factorials but requires careful implementation to avoid infinite recursion and performance issues.
Conclusion
Understanding the types of functions in R programming is crucial for optimising your code. From built-in functions that simplify tasks to user-defined functions that offer customisation, each type plays a unique role.
Mastering recursive, anonymous, and higher-order functions further enhances your programming capabilities. Implementing best practices ensures efficient and maintainable code, leveraging R’s full potential for data analysis and complex problem-solving.
#Different Types of Functions in R Programming#Types of Functions in R Programming#r programming#data science
3 notes
·
View notes
Text
I'm gearing up for Advent of Code 2023 (while trying to be realistic about time constraints with self-care, work, and relationships)!
Who wants to do it with me? I'll mostly be coding in R (in base, tidyverse, and other random paradigms/package bundles), Python, Excel, and (maybe) Rust.
Let's do this work-life-programming balance. (I am a very rusty programmer since I mostly do statistical work - I have the aptitude for it but am very inefficient with optimized solutions.) I'll post my solutions here: https://github.com/pritikadasgupta/adventofcode
#advent of code#adventofcode#programming#r language#r#rstudio#python#excel#rust#r programmer#r programming#statistician#dataviz#data scientist#work-life-programming balance
3 notes
·
View notes
Text
We all know they both have different strengths and ideal use cases, but which do you generally prefer to use in your own projects, REST or GraphQL?
11 notes
·
View notes
Note
Hi Izi! I was wondering if you had any advice/resources for learning R quickly? (It’s something my potential grad school advisor really wants me to know, so I’m in a bit of a pinch!) I’m sorry if you’ve been asked this before—thanks so much for your time and help! Your blog is so wonderful! 💗
Hiya!
And thank you!! I don't have any experience with learning R so I don't have any specific advice for R but I do have a list of resources I made for someone in the past since they requested, you can check it out here >> link to the resource 🖤
Best of luck with your studies! 😖🙌🏾✨
#my asks#resources#r programming#r programmer#programming#coding#studyblr#codeblr#progblr#studying#comp sci#programmer#student#study life#coding resources#coding study
9 notes
·
View notes
Text
Passed the fifth quiz for my programming course after procrastinating for a month. That was not as bad as I thought it was going to be.
8 notes
·
View notes
Text
does anybody know how to annotate a boxplot with ggplot on r????
2 notes
·
View notes
Link
13 notes
·
View notes
Text
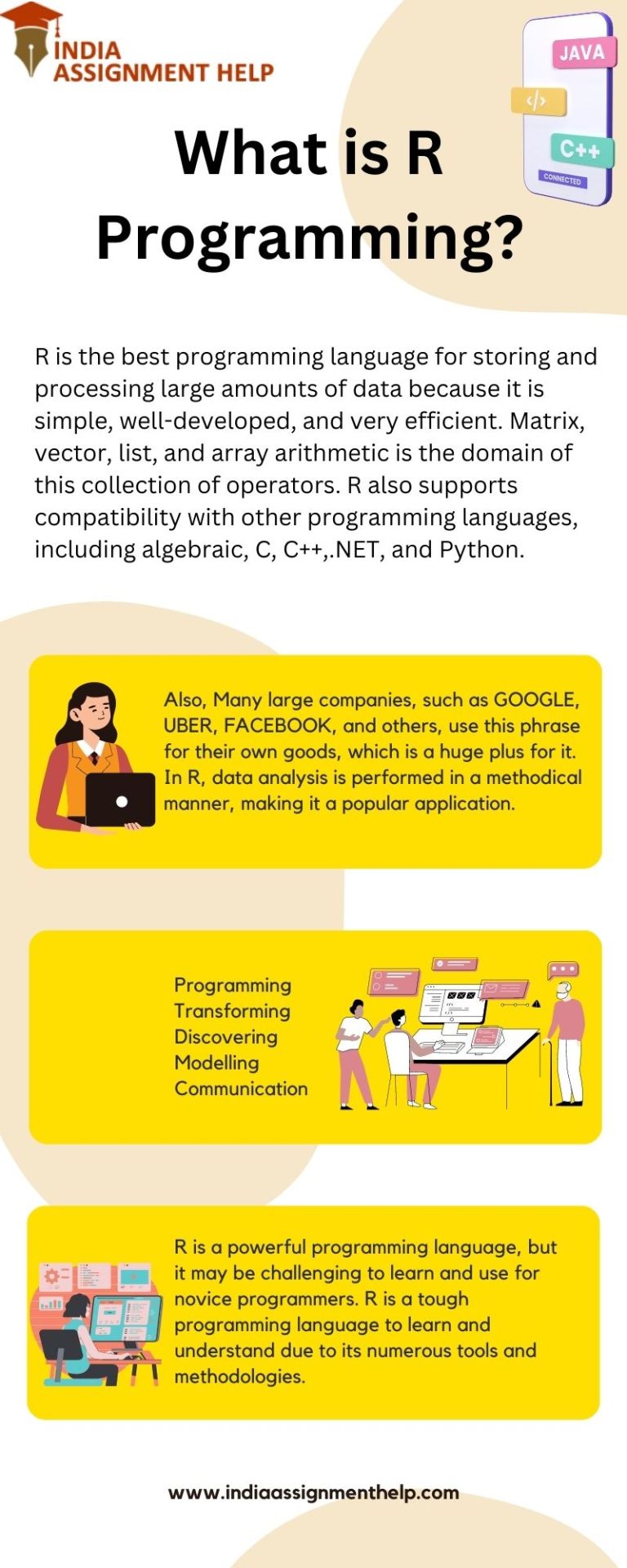
R programming is useful for doing mathematical and statistical calculations and analysis. Statistical analysis is a common application of this technology among students.
3 notes
·
View notes