#Pierre Simon Laplace
Explore tagged Tumblr posts
Text

Lithography of Pierre-Simon Laplace by François-Séraphin Delpech (1797).
Sources: 1, 2.
#i didn't expect him to be so handsome...#laplace#pierre simon laplace#pierre-simon laplace#math history#maths history#history of mathematics#history of science#science history#art#math#maths#françois seraphin delpech#delpech#mathblr
6 notes
·
View notes
Text
The book follows the account of the solar system's origin given by the great French astronomer Pierre-Simon Laplace: a nebula of dust and gas collapsed in on itself because of Newtonian gravitation.
"The Moon: A History for the Future" - Oliver Morton
#book quote#the moon#oliver morton#nonfiction#book#the moon considered a planet a world and a satellite#james nasmyth#james carpenter#solar system#france#astronomy#pierre simon laplace#nebula#dust#gas#collapse#gravity
0 notes
Text

«Así pues, hemos de considerar el estado actual del universo como el efecto de su estado anterior y como la causa del que ha de seguirle. Una inteligencia que en un momento determinado conociera todas las fuerzas que animan a la naturaleza, así como la situación respectiva de los seres que la componen, si además fuera lo suficientemente amplia como para someter a análisis tales datos, podría abarcar en una sola fórmula los movimientos de los cuerpos más grandes del universo y los del átomo más ligero; nada le resultaría incierto y tanto el futuro como el pasado estarían presentes ante sus ojos. El espíritu humano ofrece, en la perfección que ha sabido dar a la astronomía, un débil esbozo de esta inteligencia.»
Pierre-Simon Laplace: Ensayo filosófico sobre las probabilidades. Ediciones Altaya, pág. 25. Barcelona, 1995.
TGO
@bocadosdefilosofia
@dias-de-la-ira-1
#laplace#pierre-simon laplace#pierre simon laplace#ensayo filosófico sobre las probabilidades#universo#astronomía#naturaleza#fuerzas#fuerzas de la naturaleza#totalidad#datos#fórmula#átomos#inteligencia#inteligencia omniabarcante#espíritu humano#pasado#futuro#presente#causalidad#estado anterior#ciencia#determinismo#determinismo científico#teo gómez otero
1 note
·
View note
Text
“We ought to regard the present state of the universe as the effect of its antecedent state and as the cause of the state that is to follow. An intelligence knowing all the forces acting in nature at a given instant, as well as the momentary positions of all things in the universe, would be able to comprehend in one single formula the motions of the largest bodies as well as the lightest atoms in the world, provided that its intellect were sufficiently powerful to subject all data to analysis; to it nothing would be uncertain, the future as well as the past would be present to its eyes. The perfection that the human mind has been able to give to astronomy affords but a feeble outline of such an intelligence.”
― Pierre Simon de Laplace (1749 – 1827), French scholar and polymath whose work was important to the developmentof engineering, mathematics, statistics, physics, astronomy, and philosophy
#pierre simon de laplace#cosmology#universe#evolution#stages of development#creation#intelligent design#the force#all source#nature#planets#stars#motion#cycles#atoms#scaling#fractals#astronomy#observation#science#life
4 notes
·
View notes
Text
Pulp swap au: Rose Stratford and Sia Laplace
Daughter of the astronomer Pierre-Simon Laplace, Sia has followed her father's footstep and has a bright career in the field of astronomy. Besides her research of the skies, Sia continues with her father's theories on casual determinism, a philosophical view that states that every event has been caused by a previous event. She is not happy once she finds about the Cavendish hoax, and will go to confront them to New York.
Sia deserves a situationship with Margaret + the sillies of the main cast adventures. Plus, I think making her the daughter of the guy who invented casual determinism is close enough to the visions, and will be really intersting to shatter her worldviews through magic.
On the other hand, we have Rose Stratford, patron of the Cavendish. She is an author too, and publishes stories under the pseudonim S. Stratford. Her work are mostly short stories and poems of the Romanticism, with exaltated sensations and the environment as a metaphor of one's feelings. However, those who read them notice a loneliness and longing that few authors manage to capture.
When Rose reads the Cavendish stories, she feels a connection with them, and offers them as much help as they may need.
Rose gets to be a little gnc in this au, as a treat. May use she/he pronouns for her, idk yet. But also, the idea of Rose being a Romantic author? And the angst pontential we have with her and Samuel and John? I am so excited to work with her.
Extra doodle because I'm in love with Rose and they are too
#pulp musicals#pulp swap au#sia pulp musicals#rose stratford#the great moon hoax#hyl rambles#hyl draws
18 notes
·
View notes
Text
The Old Gods of CoTL: A Theory/Headcannon
When I first played through Cult of the Lamb, one line from Haro - the enigmatic owl - intrigued me: “Eons agone, these lands were rife with gods and their adherents...Those few who remained spread roots, spun webs, molded this world to meet them and theirs. 'Twere a land of many Gods once. Hundreds.” I was very curious about this as it meant there were once gods aside from the Bishops we know. But something happened that left only Shamura, Kallamar, Narinder, Heket, and Leshy. I wondered for a while who these old gods could’ve been and what were their roles/powers.
Well, I think Relics of the Old Faith may have given us a glimpse of who they might have been.
The main addition of Relics of the Old Faith is, well, the relics! Some of them reference characters we’ve met during the game (Clauneck, Kudaii, the Bishops, etc), but there are some names in there we’ve never seen before. I believe these might be the names of at least some of the old gods from eons ago. Now from what I can tell, relics can be made from either gods (e.g. the Bishops) or simply those blessed with power (Kudaii and Clauneck), so some of these names might not be gods but let’s go through them anyway!
1. GALVAR
So for each relic, we’ll be focusing on two things: what animal the god was, and what their role/power was. Starting off with Galvar, if we’re to believe the relic is reflecting their appearance, Galvar was a ram from the Ovis family due to their curves horns, with my person guess being an Argali due to the fact the horns twisting more than once as well as the overall thickness.
Now one might assume Galvar’s power was size manipulation due to their relic’s power, right? Well not exactly. After all, Heket’s relic doesn’t invoke anything to do with famine, nor does Kallamar’s have anything to do with plague. Instead, look at the description. ‘Famed Friend’. If I had to wager a guess, I would imagine Galvar may have been the classic gentle giant sort of character; looking big and menacing but is actually a big softy and uses their size to protect those weaker. So my guess would be Galvar may have been a god of protection or friendship.
2. LAPLACE
Laplace is a tough one to figure out from their relics. All that’s visible in their original relic is an eye and their blessed/cursed relic doesn’t help. But I can say for certain that they were a god of luck or chance. Not only are their relics dice, but I decided to look up the name Laplace and found a person born in the mid-1700s named Pierre-Simon Laplace who was a mathematician who focused heavily on probabilities. If I had to guess what animal Laplace could be, I would say a rabbit since they are heavily associated with luck.
3. FOWLER
Fowler is one of the easiest ones to guess in terms of species: a bird! But what kind of bird. Well, let’s look at the details. A fowler refers to someone who hunts birds. Also, take note that the only way to get this relic is to fish for it. With this, my guess is that Fowler may have been a great black-backed gull. These gulls not only eat fish, but also other, smaller birds. As for their role, my personal guess is that Fowler was a god of curses. They may have been the one to discover the uses for fervour and would go as far as cannibalising their own cultists to gain more. In other words, ‘drinking deep of their praise’.
4. ICEGORE
Probably one of the easiest ones, Icegore seems to be a yeti whose powers seem to revolve around ice. It’s possible that Icegore was a god of winter or just ice in general.
5. ALA
Going off the relic’s design, we can tell Ala was a predatory creature who seemed to value strength, pain, and survival. They also seem to have no qualms using it against others. But there are so many animals out there with sharp teeth so there’s no way to tell what animal Ala is, right? Well, what if I told you we may not only know what animal Ala is...but we may have even seen them in game. Think about it. A predator...no issue showing off their strength...associated with pain...and sharp teeth...possibly teeth in the darkness...
Ok here me out. I’ve theorised the Fox could’ve been an old Bishop in hiding for a long time. Not only are their eyes similar to the Bishops (black with red pupils) but whenever they show up, it’s through a black and red portal that looks identical to the ones the Bishops enter and exit through. So my current theory is Ala was the god of predators/hunting/murder who went into hiding due to their power deteriorating due to most of their followers being forced to join the Old Faith when they rose to power and was forced to hunt from the shadows to sustain themself, only making themself known to Lambert upon hearing of their crusades against the Bishops.
6. SLYTHOR
Another easy one, Slythor is a snake - specifically a rattlesnake due to their tail. According to their description, I think Slythor was a god of corrosion or decay.
7. SHUNRUE
Just like how small is opposite to big, I imagine Shunrue was the opposite to Galvar in every way. While Galvar is a friendly gentle giant, Shunrue may have been a small, selfish and cowardly creature. While one is a god of friendship and protection, the other is a god of betrayal and selfishness. But as for what animal Shunrue was...well unless he was a blob of goop, we can’t really say for certain. I would guess he would be either a rat (usually associated with selfish behaviours) or a bearded vulture (a predator of the Argali).
8. STIHI
So I did some research to see what animal’s have venomous tongue, and the most likely candidate for Stihi is a Gila Monster.
As for their domain, I decided to look up their name. Turns out a stihi is a fire-breathing storm demon from Albanian mythology. So I would like to guess Stihi is a god of storms or just destruction in general.
9. WARRACKA
...yeah, I have no idea what animal Warracka could be. I even looked up their name and came up with nothing. But due to the closed fist imagery in this and in Stihi’s description, I figured they could be a lizard like them. Specifically a cannibal lizard due to the method of gaining this relic. Therefor, I believe Warracka is an Aegean Wall Lizard - which was recently discovered to eat their own species - and the god of cannibalism.
10. TURUA
Turua seems to be an octopus based on their relic, but their description doesn’t give much of a hint as to what they were the god of. So I looked up their name and it turns out Turua is Maori for ‘twice seen’, as the New Zealand town of the same name was built on a river, and the name came from the reflections seen in the water. So my guesses are Turua is either a god of reflections or illusions, or a god of the depths (maybe they knew Kallamar? Who knows!)
11. AETHRILE
This one has me stumped. There are no clues as to what animal Aetherile could be and looking up their name only shows CotL results. As for their domain, I originally thought they were the original crafter of the relics. But then I had a thought. What if they weren’t exactly a god? What if they were more of a benefactor of gods; someone who would aid them in certain ways? ...and what if ‘Aethrile’ was only one of their many names?
This is a complete theory with little basis, but my guess is ‘Aetherile’ was the first name the Mystic Seller was ever given and they made the powder box as a way to aid gods when they needed it. Just my own little theory though.
I won’t go into the unattainable relics hidden in the game files as I don’t know how cannon those are, so that’s it for my god guesses! But what happened to these gods? How did the relics come to be? Why does Chemach have them? Well join me in the next post where I’ll share my theory on how Chemach’s desire for power indirectly led to Narinder’s imprisonment.
47 notes
·
View notes
Text
Determinisme dari Perspektif Ilmiah: Antara Hukum Alam dan Kebebasan Manusia
Abstrak
Determinisme adalah konsep yang telah lama menjadi bahan perdebatan dalam filsafat dan ilmu pengetahuan. Dari perspektif ilmiah, determinisme didasarkan pada gagasan bahwa alam semesta tunduk pada hukum-hukum alam yang mengatur setiap peristiwa secara kausal. Dalam karya tulis ini, kita akan membahas determinisme dari sudut pandang ilmu pengetahuan modern, dengan fokus pada hukum-hukum fisika, biologi, dan perkembangan teori chaos yang menantang pandangan deterministik. Selain itu, kita juga akan meninjau kompatibilitas antara determinisme dan konsep kebebasan kehendak dalam konteks ilmiah.
Pendahuluan
Determinisme adalah doktrin yang menyatakan bahwa segala sesuatu yang terjadi di alam semesta, termasuk tindakan manusia, ditentukan oleh kondisi-kondisi awal dan hukum alam. Dalam pandangan deterministik yang kuat, tidak ada ruang untuk kebebasan kehendak karena setiap peristiwa, dari skala kosmik hingga individu, adalah hasil dari rangkaian sebab-akibat yang tak terhindarkan. Namun, dalam ilmu pengetahuan modern, muncul beberapa tantangan terhadap determinisme klasik, terutama melalui mekanika kuantum dan teori chaos. Karya tulis ini bertujuan untuk menjelaskan konsep determinisme dari sudut pandang ilmiah dan mengeksplorasi implikasinya terhadap konsep kebebasan manusia.
*Determinisme dalam Fisika Klasik
Fisika klasik, terutama sejak karya Isaac Newton, memberikan fondasi kuat bagi pandangan deterministik. Hukum gerak Newton menyatakan bahwa pergerakan benda di alam semesta ditentukan oleh gaya-gaya yang bekerja padanya, di mana setiap peristiwa dapat diprediksi secara pasti jika kita mengetahui kondisi awalnya dengan akurasi yang sempurna. Ini menimbulkan pandangan alam semesta sebagai “mesin” yang bekerja dengan aturan-aturan deterministik. Pierre-Simon Laplace mengembangkan gagasan ini lebih lanjut melalui hipotesis yang kini dikenal sebagai "Iblis Laplace". Dalam skenario ini, jika ada entitas yang mengetahui posisi dan momentum setiap partikel di alam semesta pada suatu waktu, maka entitas tersebut dapat memprediksi semua peristiwa masa depan.
Tantangan dari Mekanika Kuantum. Meski fisika klasik mendukung determinisme, perkembangan mekanika kuantum pada awal abad ke-20 mengubah pandangan ini. Mekanika kuantum memperkenalkan prinsip ketidakpastian Heisenberg, yang menyatakan bahwa tidak mungkin secara bersamaan mengetahui posisi dan momentum partikel secara akurat. Ini menghasilkan pandangan bahwa alam semesta pada tingkat mikroskopik memiliki aspek indeterministik, di mana peristiwa kuantum bersifat probabilistik, bukan deterministik.
Teori kuantum ini memunculkan perdebatan antara fisikawan yang mendukung interpretasi probabilistik, seperti Niels Bohr dan Werner Heisenberg, dengan mereka yang masih percaya pada determinisme, seperti Albert Einstein yang terkenal dengan ucapannya, "Tuhan tidak bermain dadu." Meskipun peristiwa-peristiwa pada skala kuantum tidak dapat diprediksi secara pasti, beberapa fisikawan tetap berpendapat bahwa mungkin ada variabel tersembunyi yang belum diketahui yang dapat mengembalikan determinisme pada skala subatomik.
*Teori Chaos dan Determinisme
Selain mekanika kuantum, teori chaos juga menantang determinisme klasik. Teori chaos, yang dipelopori oleh Edward Lorenz pada 1960-an, menunjukkan bahwa sistem-sistem dinamis tertentu sangat sensitif terhadap kondisi awal, sehingga perubahan yang sangat kecil dapat menghasilkan hasil yang sangat berbeda. Fenomena ini dikenal sebagai "efek kupu-kupu", di mana kepakan sayap kupu-kupu di Brasil dapat memicu tornado di Texas.
Walaupun teori chaos beroperasi dalam sistem yang sepenuhnya deterministik, ketidakmampuan kita untuk mengetahui kondisi awal dengan presisi yang sempurna membuat peristiwa-peristiwa di masa depan tampak tidak dapat diprediksi. Oleh karena itu, teori chaos memperkenalkan unsur ketidakpastian dalam sistem deterministik, tetapi tidak sepenuhnya menolak determinisme. Sebaliknya, ini menyoroti batasan pengetahuan manusia dalam memprediksi peristiwa-peristiwa yang kompleks.
*Determinisme dalam Biologi dan Neurosains
Determinisme juga muncul dalam biologi, terutama dalam konteks genetika dan neurosains. Dalam biologi, determinisme genetis menyatakan bahwa perilaku individu sebagian besar ditentukan oleh faktor genetis. Misalnya, kecenderungan seseorang terhadap penyakit tertentu, sifat kepribadian, atau bahkan kecerdasan dapat dikaitkan dengan gen yang diwarisi dari orang tua. Namun, konsep ini telah diperkaya dengan pemahaman bahwa lingkungan juga memainkan peran penting dalam perkembangan individu, yang melahirkan pendekatan epigenetika.
Di bidang neurosains, pertanyaan tentang kebebasan kehendak menjadi lebih menonjol. Eksperimen seperti yang dilakukan oleh Benjamin Libet pada 1980-an menunjukkan bahwa aktivitas otak yang terkait dengan pengambilan keputusan terjadi sebelum individu secara sadar menyadari keputusan tersebut. Ini menimbulkan pertanyaan apakah kebebasan kehendak benar-benar ada, atau apakah semua keputusan manusia hanya hasil dari proses neurobiologis yang deterministik.
*Determinisme dan Kebebasan Kehendak
Dalam diskusi ilmiah mengenai determinisme, pertanyaan apakah kebebasan kehendak dapat bertahan dalam dunia yang ditentukan oleh hukum kausal masih menjadi perdebatan. Beberapa ahli filsafat, seperti kompatibilis, berpendapat bahwa kebebasan kehendak dapat ada dalam kerangka deterministik. Mereka berpendapat bahwa kebebasan tidak berarti ketiadaan sebab-akibat, tetapi kemampuan untuk bertindak sesuai dengan motivasi dan keinginan yang selaras dengan kondisi internal seseorang.
Di sisi lain, incompatibilis berpendapat bahwa determinisme sepenuhnya menafikan kebebasan kehendak, karena jika setiap tindakan ditentukan oleh keadaan sebelumnya, maka kebebasan individu hanya ilusi.
Kesimpulan Dari perspektif ilmiah, determinisme menawarkan pandangan alam semesta yang diatur oleh hukum-hukum alam yang ketat. Fisika klasik mendukung determinisme, namun perkembangan dalam mekanika kuantum dan teori chaos menunjukkan bahwa alam semesta lebih kompleks dari yang diperkirakan sebelumnya. Dalam biologi dan neurosains, determinisme memainkan peran dalam menjelaskan perilaku manusia, meskipun faktor lingkungan dan proses neurobiologis juga harus dipertimbangkan.
Dalam kaitannya dengan kebebasan kehendak, ilmu pengetahuan modern menantang gagasan bahwa manusia sepenuhnya bebas dalam memilih tindakannya, tetapi perdebatan ini masih jauh dari kata selesai. Kompatibilitas antara determinisme dan kebebasan kehendak mungkin terletak dalam pemahaman yang lebih mendalam tentang bagaimana hukum alam, otak manusia, dan kesadaran berinteraksi.
Daftar Pustaka 1. Libet, B. (1985). "Unconscious cerebral initiative and the role of conscious will in voluntary action." Behavioral and Brain Sciences. 2. Lorenz, E. N. (1963). "Deterministic Nonperiodic Flow." Journal of the Atmospheric Sciences. 3. Heisenberg, W. (1927). "Über den anschaulichen Inhalt der quantentheoretischen Kinematik und Mechanik." Zeitschrift für Physik. 4. Laplace, P. S. (1814). "A Philosophical Essay on Probabilities."
2 notes
·
View notes
Text
Astute readers may have noticed that exploitation and the labor theory of value were conspicuously absent from my explainer on class relations in my last post. This is because the labor theory of value is incorrect. Mainstream economics has had no issue explaining the price system using only marginal utility (use value), and the concept of a separate "exchange value" has been rendered extraneous. As Pierre-Simon de Laplace said: "Je n'ai pas besoin de cette hypothèse". I don't need that assumption.
I don't even make a super clear distinction between labor and capital in my analysis. I at one point in the text describe the contribution of animals to the production process as labor, but label it as capital in the diagram. I said in Tale of Two Pegasi that humans are just machines with ownership rights over themselves. Similarly, agents that do not have ownership rights over themselves (slaves) are economically indistinct from machines, even if they are ethically distinct. The reason it is useful to describe their contribution as contributing labor rather than contributing capital is that their contribution is inalienable. Its usage is inextricably tied up with their own welfare.
In any case it reveals an issue with the labor theory of value. There's nothing unique about human labor power. If somebody owns an ox, a human slave, and a self-driving automatic plow, does he only create new exchange value if he employs the human to plow his field? Do work horses create value? Labor-power doesn't seem distinct from other productive resources, and adding the assumption that it does into the model does not clarify or explain anything. Occam's razor suggests we should drop it.
4 notes
·
View notes
Text
"A Beginning Of Sharing Thoughts"
ABOUT
Salutations to whomever this may concern,
This is Louie Laplace. Louie is derived from my actual nickname but removed the first syllable of it, inspired by Louie from Ducktales and the Australian tv show Bluey; as I originally wanted it to be spelled as ‘Louey’ but it’s too quirky and childish imo. Laplace derived from a song called ‘Laplace’s Angel’ by Will Wood “The only ones in need of love are those who don’t receive enough so evil ones should get a little more” the song being a twist of a notable published articulation of causal determinism on a scientific basis by Pierre-Simon Laplace in 1814 called ”Laplace’s Demon” An all-seeing, all-knowing being, seeing everything, everywhere all at once. Knowing everything from the speck of dust that lies on your lash to the truthful mass of a supermassive black hole.
A writing piece made by a possibly mentally ill teenager that lives off their delusions being in other universes, Daydreaming in the mornings, Lucid Dreaming in the evenings.
To anyone or the future me reading this, this is the perspective of a persona, an unknowing one.
This is a journal-biography (or whatever that could mean) of everything I feel, think, or do because I hate reality like most people do. This is to somehow spice up my life. (hopefully) I considered journaling just now and I thought I’ll do it now. Wow, great job, me. Let’s see if you’ll forget about this after a few hours.
Talking to myself has been a problem, so why not make it worse. Be the bigger person!!
And as what a specific narrator would say
“This is a story about a person named Louie”
0 notes
Text
How AI is improving simulations with smarter sampling techniques
New Post has been published on https://sunalei.org/news/how-ai-is-improving-simulations-with-smarter-sampling-techniques/
How AI is improving simulations with smarter sampling techniques

Imagine you’re tasked with sending a team of football players onto a field to assess the condition of the grass (a likely task for them, of course). If you pick their positions randomly, they might cluster together in some areas while completely neglecting others. But if you give them a strategy, like spreading out uniformly across the field, you might get a far more accurate picture of the grass condition.
Now, imagine needing to spread out not just in two dimensions, but across tens or even hundreds. That’s the challenge MIT Computer Science and Artificial Intelligence Laboratory (CSAIL) researchers are getting ahead of. They’ve developed an AI-driven approach to “low-discrepancy sampling,” a method that improves simulation accuracy by distributing data points more uniformly across space.
A key novelty lies in using graph neural networks (GNNs), which allow points to “communicate” and self-optimize for better uniformity. Their approach marks a pivotal enhancement for simulations in fields like robotics, finance, and computational science, particularly in handling complex, multidimensional problems critical for accurate simulations and numerical computations.
“In many problems, the more uniformly you can spread out points, the more accurately you can simulate complex systems,” says T. Konstantin Rusch, lead author of the new paper and MIT CSAIL postdoc. “We’ve developed a method called Message-Passing Monte Carlo (MPMC) to generate uniformly spaced points, using geometric deep learning techniques. This further allows us to generate points that emphasize dimensions which are particularly important for a problem at hand, a property that is highly important in many applications. The model’s underlying graph neural networks lets the points ‘talk’ with each other, achieving far better uniformity than previous methods.”
Their work was published in the September issue of the Proceedings of the National Academy of Sciences.
Take me to Monte Carlo
The idea of Monte Carlo methods is to learn about a system by simulating it with random sampling. Sampling is the selection of a subset of a population to estimate characteristics of the whole population. Historically, it was already used in the 18th century, when mathematician Pierre-Simon Laplace employed it to estimate the population of France without having to count each individual.
Low-discrepancy sequences, which are sequences with low discrepancy, i.e., high uniformity, such as Sobol’, Halton, and Niederreiter, have long been the gold standard for quasi-random sampling, which exchanges random sampling with low-discrepancy sampling. They are widely used in fields like computer graphics and computational finance, for everything from pricing options to risk assessment, where uniformly filling spaces with points can lead to more accurate results.
The MPMC framework suggested by the team transforms random samples into points with high uniformity. This is done by processing the random samples with a GNN that minimizes a specific discrepancy measure.
One big challenge of using AI for generating highly uniform points is that the usual way to measure point uniformity is very slow to compute and hard to work with. To solve this, the team switched to a quicker and more flexible uniformity measure called L2-discrepancy. For high-dimensional problems, where this method isn’t enough on its own, they use a novel technique that focuses on important lower-dimensional projections of the points. This way, they can create point sets that are better suited for specific applications.
The implications extend far beyond academia, the team says. In computational finance, for example, simulations rely heavily on the quality of the sampling points. “With these types of methods, random points are often inefficient, but our GNN-generated low-discrepancy points lead to higher precision,” says Rusch. “For instance, we considered a classical problem from computational finance in 32 dimensions, where our MPMC points beat previous state-of-the-art quasi-random sampling methods by a factor of four to 24.”
Robots in Monte Carlo
In robotics, path and motion planning often rely on sampling-based algorithms, which guide robots through real-time decision-making processes. The improved uniformity of MPMC could lead to more efficient robotic navigation and real-time adaptations for things like autonomous driving or drone technology. “In fact, in a recent preprint, we demonstrated that our MPMC points achieve a fourfold improvement over previous low-discrepancy methods when applied to real-world robotics motion planning problems,” says Rusch.
“Traditional low-discrepancy sequences were a major advancement in their time, but the world has become more complex, and the problems we’re solving now often exist in 10, 20, or even 100-dimensional spaces,” says Daniela Rus, CSAIL director and MIT professor of electrical engineering and computer science. “We needed something smarter, something that adapts as the dimensionality grows. GNNs are a paradigm shift in how we generate low-discrepancy point sets. Unlike traditional methods, where points are generated independently, GNNs allow points to ‘chat’ with one another so the network learns to place points in a way that reduces clustering and gaps — common issues with typical approaches.”
Going forward, the team plans to make MPMC points even more accessible to everyone, addressing the current limitation of training a new GNN for every fixed number of points and dimensions.
“Much of applied mathematics uses continuously varying quantities, but computation typically allows us to only use a finite number of points,” says Art B. Owen, Stanford University professor of statistics, who wasn’t involved in the research. “The century-plus-old field of discrepancy uses abstract algebra and number theory to define effective sampling points. This paper uses graph neural networks to find input points with low discrepancy compared to a continuous distribution. That approach already comes very close to the best-known low-discrepancy point sets in small problems and is showing great promise for a 32-dimensional integral from computational finance. We can expect this to be the first of many efforts to use neural methods to find good input points for numerical computation.”
Rusch and Rus wrote the paper with University of Waterloo researcher Nathan Kirk, Oxford University’s DeepMind Professor of AI and former CSAIL affiliate Michael Bronstein, and University of Waterloo Statistics and Actuarial Science Professor Christiane Lemieux. Their research was supported, in part, by the AI2050 program at Schmidt Futures, Boeing, the United States Air Force Research Laboratory and the United States Air Force Artificial Intelligence Accelerator, the Swiss National Science Foundation, Natural Science and Engineering Research Council of Canada, and an EPSRC Turing AI World-Leading Research Fellowship.
1 note
·
View note
Text
How AI is improving simulations with smarter sampling techniques
New Post has been published on https://thedigitalinsider.com/how-ai-is-improving-simulations-with-smarter-sampling-techniques/
How AI is improving simulations with smarter sampling techniques
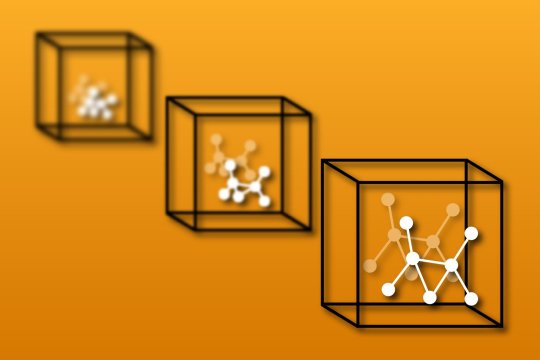
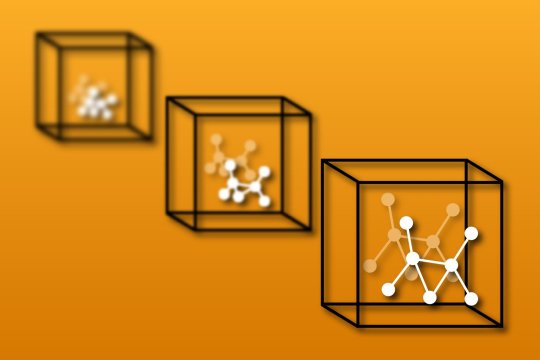
Imagine you’re tasked with sending a team of football players onto a field to assess the condition of the grass (a likely task for them, of course). If you pick their positions randomly, they might cluster together in some areas while completely neglecting others. But if you give them a strategy, like spreading out uniformly across the field, you might get a far more accurate picture of the grass condition.
Now, imagine needing to spread out not just in two dimensions, but across tens or even hundreds. That’s the challenge MIT Computer Science and Artificial Intelligence Laboratory (CSAIL) researchers are getting ahead of. They’ve developed an AI-driven approach to “low-discrepancy sampling,” a method that improves simulation accuracy by distributing data points more uniformly across space.
A key novelty lies in using graph neural networks (GNNs), which allow points to “communicate” and self-optimize for better uniformity. Their approach marks a pivotal enhancement for simulations in fields like robotics, finance, and computational science, particularly in handling complex, multidimensional problems critical for accurate simulations and numerical computations.
“In many problems, the more uniformly you can spread out points, the more accurately you can simulate complex systems,” says T. Konstantin Rusch, lead author of the new paper and MIT CSAIL postdoc. “We’ve developed a method called Message-Passing Monte Carlo (MPMC) to generate uniformly spaced points, using geometric deep learning techniques. This further allows us to generate points that emphasize dimensions which are particularly important for a problem at hand, a property that is highly important in many applications. The model’s underlying graph neural networks lets the points ‘talk’ with each other, achieving far better uniformity than previous methods.”
Their work was published in the September issue of the Proceedings of the National Academy of Sciences.
Take me to Monte Carlo
The idea of Monte Carlo methods is to learn about a system by simulating it with random sampling. Sampling is the selection of a subset of a population to estimate characteristics of the whole population. Historically, it was already used in the 18th century, when mathematician Pierre-Simon Laplace employed it to estimate the population of France without having to count each individual.
Low-discrepancy sequences, which are sequences with low discrepancy, i.e., high uniformity, such as Sobol’, Halton, and Niederreiter, have long been the gold standard for quasi-random sampling, which exchanges random sampling with low-discrepancy sampling. They are widely used in fields like computer graphics and computational finance, for everything from pricing options to risk assessment, where uniformly filling spaces with points can lead to more accurate results.
The MPMC framework suggested by the team transforms random samples into points with high uniformity. This is done by processing the random samples with a GNN that minimizes a specific discrepancy measure.
One big challenge of using AI for generating highly uniform points is that the usual way to measure point uniformity is very slow to compute and hard to work with. To solve this, the team switched to a quicker and more flexible uniformity measure called L2-discrepancy. For high-dimensional problems, where this method isn’t enough on its own, they use a novel technique that focuses on important lower-dimensional projections of the points. This way, they can create point sets that are better suited for specific applications.
The implications extend far beyond academia, the team says. In computational finance, for example, simulations rely heavily on the quality of the sampling points. “With these types of methods, random points are often inefficient, but our GNN-generated low-discrepancy points lead to higher precision,” says Rusch. “For instance, we considered a classical problem from computational finance in 32 dimensions, where our MPMC points beat previous state-of-the-art quasi-random sampling methods by a factor of four to 24.”
Robots in Monte Carlo
In robotics, path and motion planning often rely on sampling-based algorithms, which guide robots through real-time decision-making processes. The improved uniformity of MPMC could lead to more efficient robotic navigation and real-time adaptations for things like autonomous driving or drone technology. “In fact, in a recent preprint, we demonstrated that our MPMC points achieve a fourfold improvement over previous low-discrepancy methods when applied to real-world robotics motion planning problems,” says Rusch.
“Traditional low-discrepancy sequences were a major advancement in their time, but the world has become more complex, and the problems we’re solving now often exist in 10, 20, or even 100-dimensional spaces,” says Daniela Rus, CSAIL director and MIT professor of electrical engineering and computer science. “We needed something smarter, something that adapts as the dimensionality grows. GNNs are a paradigm shift in how we generate low-discrepancy point sets. Unlike traditional methods, where points are generated independently, GNNs allow points to ‘chat’ with one another so the network learns to place points in a way that reduces clustering and gaps — common issues with typical approaches.”
Going forward, the team plans to make MPMC points even more accessible to everyone, addressing the current limitation of training a new GNN for every fixed number of points and dimensions.
“Much of applied mathematics uses continuously varying quantities, but computation typically allows us to only use a finite number of points,” says Art B. Owen, Stanford University professor of statistics, who wasn’t involved in the research. “The century-plus-old field of discrepancy uses abstract algebra and number theory to define effective sampling points. This paper uses graph neural networks to find input points with low discrepancy compared to a continuous distribution. That approach already comes very close to the best-known low-discrepancy point sets in small problems and is showing great promise for a 32-dimensional integral from computational finance. We can expect this to be the first of many efforts to use neural methods to find good input points for numerical computation.”
Rusch and Rus wrote the paper with University of Waterloo researcher Nathan Kirk, Oxford University’s DeepMind Professor of AI and former CSAIL affiliate Michael Bronstein, and University of Waterloo Statistics and Actuarial Science Professor Christiane Lemieux. Their research was supported, in part, by the AI2050 program at Schmidt Futures, Boeing, the United States Air Force Research Laboratory and the United States Air Force Artificial Intelligence Accelerator, the Swiss National Science Foundation, Natural Science and Engineering Research Council of Canada, and an EPSRC Turing AI World-Leading Research Fellowship.
#affiliate#ai#AI-powered#air#air force#Algorithms#applications#approach#Art#artificial#Artificial Intelligence#assessment#author#autonomous#autonomous driving#Boeing#Canada#challenge#classical#cluster#computation#computational science#computer#Computer Science#Computer Science and Artificial Intelligence Laboratory (CSAIL)#Computer science and technology#continuous#course#data#Deep Learning
1 note
·
View note
Text
То, что мы знаем, — ограничено, а то, чего мы не знаем, — бесконечно.
What we know is limited, and what we do not know is infinite.
0 notes
Text
L'Héritage Immortel des Mathématiciens Français
Dans le monde des mathématiques, on ne peut pas exagérer l'impact et l'influence que les mathématiciens français ont eus sur leurs domaines respectifs au cours de l'histoire et encore aujourd'hui. Que ce soit Pierre-Simon Laplace, né en 1749, qui a formulé la première description mathématique complète de la mécanique céleste, ou Augustin-Louis Cauchy, né en 1789, qui a été l'un des premiers à donner au calcul différentiel et intégral une base rigoureuse et solide (connue aujourd'hui sous le nom d'analyse réelle). L'une de mes figures préférées, et peut-être la plus tragique de l'histoire des mathématiques, est Évariste Galois. Durant sa courte vie, Galois a parfaitement résolu une question extrêmement importante sur les solutions d'équations polynomiales qui était restée ouverte pendant plus de 350 ans. En conséquence, Galois a révolutionné tout le domaine de l'algèbre avec son concept de groupes et de corps (connus aujourd'hui sous le nom de théorie de Galois et algèbre abstraite). Non seulement cela, mais il a accompli tout cela avant l'âge de 20 ans, tout en étant emprisonné à plusieurs reprises pour ses activités politiques, et en étant rejeté par l'École Polytechnique (la meilleure école de mathématiques de son époque). Malheureusement, le 30 mai 1832, Galois a été retrouvé dans un champ, ayant reçu une balle dans l'estomac lors d'un duel (les circonstances duquel restent encore inconnues aujourd'hui). Il est mort le lendemain, mais a laissé derrière lui suffisamment de génie pour occuper les mathématiciens et les scientifiques pendant des siècles. Pour en savoir plus sur la vie de Galois, je recommande le livre Whom the Gods Love de Leopold Infeld.

Évariste Galois portrait (aged 15)
1 note
·
View note
Text
Riccioli, Grimaldi, and Dechales all described the effect as part of an argument against the heliocentric system of Copernicus. In other words, they argued that the Earth's rotation should create the effect, and so failure to detect the effect was evidence for an immobile Earth.[3] The Coriolis acceleration equation was derived by Euler in 1749,[4][5] and the effect was described in the tidal equations of Pierre-Simon Laplace in 1778.[6]
0 notes
Text
Sécheresses et pluies extrêmes, les deux faces du changement climatique en France
See on Scoop.it - EntomoNews
Tout semble opposer les épisodes de sécheresse et ceux de pluie intense, et pourtant. Du fait du changement climatique, ces deux extrêmes marchent désormais main dans la main.
Publié: 27 mai 2024, 17:04 CEST
Jean Jouzel
Directeur émérite de Recherche au Laboratoire des Sciences du Climat et de l’Environnement (CEA-CNRS-UVSQ)/Institut Pierre Simon Laplace, Commissariat à l’énergie atomique et aux énergies alternatives (CEA)
Édité par
Sarah Sermondadaz
Cheffe de rubrique environnement et énergie, The Conversation France
Avec la participation de Carine Rocchesani.
Ce texte est publié dans le cadre du colloque international « Les impacts socio-économiques de la sécheresse » qui se tient le 31 mai 2024 et dont The Conversation France est partenaire.
Il est illustré par Cartooning for Peace, un réseau international de dessinateurs et dessinatrices de presse engagés à promouvoir, par le langage universel du dessin de presse, la liberté d’expression et les droits de l’Homme.
0 notes