#Janice age regression
Explore tagged Tumblr posts
Text
Caregiver Electric Mayhem Headcanons (The Muppet Movie)
Dr. Teeth
His wardrobe is a symphony of chaos, and you’re always welcome to partake. He’s happy to loan you any of his clothes, or take you shopping to create your own crazy outfits. A kiddo as amazing as you was born to stand out!
He often goes on complimentary tangents with flowery (and possibly made-up) words. He loves talking, and he loves you; so talking about you can last for ages.
He loves to sit and color with you, or help you repaint your room, or use face paints on you… Art is something he really enjoys, and he’s always excited to share it with you.
Slow, quiet music isn’t really his scene, but he’ll play some softer tunes if you need help falling asleep. (And if you want something loud and proud to help you wake up, he’s got ya covered!)
He and the others put together a “Cool Kid’s Corner” on the tour bus so you ride along with them. (Dr. Teeth totally got you a beanbag chair so you can travel in maximum comfort.)
Floyd
Spoils the HECK out of you. (Nothing’s too good for his kiddo!) Often, you don’t even need to ask for what you want; he just knows somehow.
Also, ALL the compliments. Everything you do, you do well; anything you wear, you look great in; every time you mess up, you mess up with style. Seriously, your self-esteem may never be higher than it is while you’re with Floyd.
He occasionally loans you his jacket ‘cause he thinks you look adorable wearing it. (And you do.)
As the main songwriter of the band, you can bet he writes songs about you on occasion. Anyone who knows you can tell when a song is about you because he’ll incorporate things you frequently say or do, things you enjoy, and general descriptions of you.
Maybe it’s because of his songsmith skills, but he is great at telling you bedtime stories. He usually tells you the classics, but he describes them so uniquely you often feel like your hearing something brand new.
Janice
She prefers to stick to organic foods for herself, so she is super on top of your food preferences and restrictions. (She also tries to encourages you to eat healthy, but she’ll never force you; it’s a free country after all.)
She likes to wear a bit of makeup, and she loves to help you put on makeup if you choose to wear it. You look fantastic with or without it, of course, but getting extra fancy is always fun.
Whatever age you regress to, Janice treats you a little younger. She can’t help herself - you’re just so cute, she just HAS to baby you!
One of her favorite activities to do with you is tie-dye clothes - or stuffed animals! She has a number of methods for making amazing patterns, and she loves to see you wearing the things you create.
She is super encouraging of everything you do. She’s always trying to boost your confidence.
Animal
In a lot of ways, he’s like a big dog. He’s wild, excitable, and incredibly protective of you. No person will ever bother you ever again, and anything inanimate that upsets you (thunder, darkness, bugs) is gonna be growled at.
He has the highest energy levels of anyone in the band. Any time you want to play, he’s ready! He especially loves games where he gets to chase after you.
Animal is very rough-and-tumble and likes to wrestle, but he’s always very careful not to hurt you.
Sometimes kids just want to be destructive. Maybe you’re upset about something; maybe you’re overly excited. Whatever the reason, Animal is ALWAYS ready to help you break things.
… And sometimes kids just want to sit quietly. Animal isn’t as good at just hanging out, but for you, he’s willing to try.
Zoot
He’s the chillest member of the band. He never pushes you to talk or do things; if you just want to sit on the couch in silence for hours, he’s right there with you.
(Similarly, if you want to hang out and watch movies, he’s always up for that. He really enjoys watching things you enjoy, even if he sometimes spaces out.)
Zoot can be forgetful at times, but he always remembers the really important things. He never forgets what kinds of things upset you, what allergies you have, or your favorite pet names.
If you need to vent, he’s always ready to listen. He’s one of the best people to talk to; he never interrupts and doesn’t offer any advice unless you really want it.
He’s tremendously patient. If you’re struggling to learn something new, he’ll remind you a hundred times without getting frustrated. If you’re throwing a tantrum, he’ll wait you out without ever losing his cool. He’s never gets annoyed no matter what you do or don’t do.
#Eflen writes#Eflen headcanons#The Muppet Movie agere#The Muppet Movie age regression#Electric mayhem agere#Electric mayhem age regression#Dr. Teeth agere#Dr. Teeth age regression#Floyd agere#Floyd age regression#Janice agere#Janice age regression#Animal agere#Animal age regression#Zoot agere#Zoot age regression#sfw age regression#sfw agere#age regression headcanons#caregiver headcanons#fandom agere
34 notes
·
View notes
Text
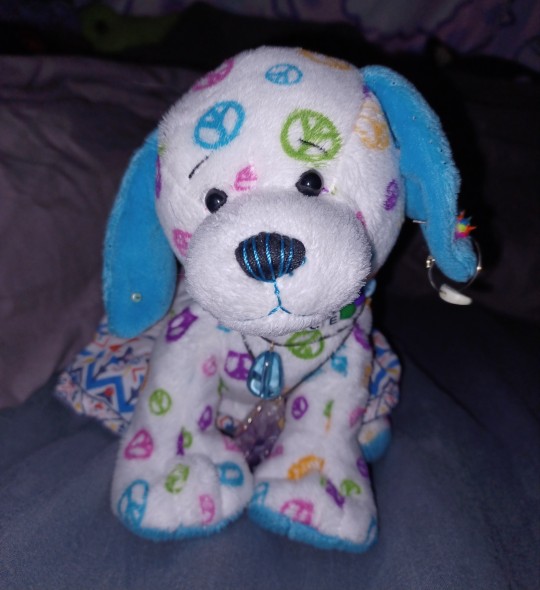
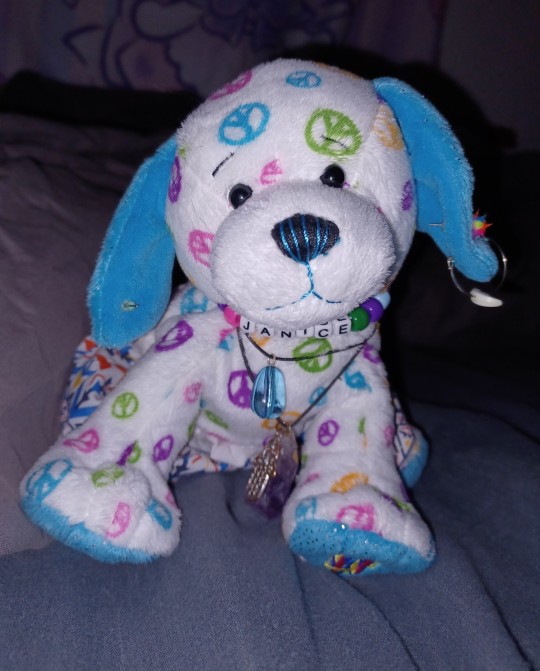

I'm a bit late to posting this but for my birthday I got a peace puppy! I had been looking for one without a code to go with my virtual puppy and we'll here we are! This is Janice (she/her) shes a crystal girly and an artist can't wait to see what adventures her and the other webkinz pups get up to!
#plushies#my plushies: janice#plushie#plushblr#plushie aesthetic#stuffed animals#plush animal#kidcore#my plushies#safe plush#sfw age regression#agere sfw#webkinz puppy#my webkinz#webkinz#webkinz plush#webkinz peace puppy#kinzblr#comfort plush#plushiecore#plushie blog#plushie collection#plushwave#sfw age regressor#stuffed animal#plushie clothes#critter corner plushie sanctuary
170 notes
·
View notes
Text
Last night, I had a dream where a new batch episodes of The New Norm aired, and in one of those episodes Chloe stands up to Norm when he's being a piece crap and out of impulsive rage she locks Norm out the house to give her space.
But in that same episode, it was revealed that Chloe age regresses, Chaz and Janice were her caregivers, so I got minor Chloe x Chaz as a treat. And no, Norm doesn't know about her regression, and I like to think it stayed that way.
Fanfic writers do what you want with that, I guess.
#the new norm chaz#the new norm chloe#the new norm#the new norm janice#age regression#last night i dreamt
14 notes
·
View notes
Text
Okay imma just make a post of a bunch of Electronic Mayhem headcanons
Zoot has chronic pain, particularly in his joints. He 'self medicates' and is partly why he's much more spacey than the rest of the band.
Janice really likes caring for Zoot on bad pain days. She'll be all up in his business even if he insists she doesn't need to, but it's not often he complains anyways, Janice is a great nurse :D
Zoot loves bugs. He doesn't keep any collections/pets because the band spends so much time living out of the van. He does always read up on bugs common to the cities they visit, and loves showing off the ones he finds. Except to Floyd, who can't handle bugs. Zoot also checks if a bug could be poisonous, because Animal tends to eat the bugs Zoot shows off.
Animal uses he/it. People have a tendency to use it/its for Animal which doesn't bother it.
If any of them knew or cared about labels: Pansexual for Animal & Teeth; Bi for Lips, Zoot, Janice and Floyd. But they are all literally just vibing.
Teeth talks like that actually because of a speech impediment (from dyspraxia). He didn't talk much when he was younger cause he was self-conscious. Part of what drew him to music is it doesn't tend to affect him as much when he sings (or so he thinks). These days it doesn't bother him cause his band loved out his stresses about it.
Teeth, Floyd, Animal, and Janice are all runaways.
Floyd left his home at about 15 cause of his drunken abusive father. His mum wouldn't leave with him and he regrets it everyday.
Teeth had his overbearing mum. He left home at 17 after graduating high school and met Floyd. When Teeth bumped into his parents he regressed and fled with them, and attended uni to become a qualified dentist. (This is when Floyd joins the army to not think about being abandoned)
Janice had the same sort of thing, but with a lot of emphasis on being A Proper Young Lady and getting married and having at least four kids. She ditched home at 19 to avoid what was basically an arranged marriage. She still has plenty of religious trauma and was fairly socially stunted from a lack of friends her own age growing up.
Animal was in & out of care homes and medical institutions cos AuDHD making it high needs. It got sick of that shit sometime in its late teens then met Floyd and Teeth, rest is history.
Despite Floyd being a very gruff, sarcastic and rude, he's actually the best at handling Animal. Love makes him patient. He'll also tell you to 'go fuck yourself' if you point out how much he cares, because god forbid people acknowledge that he's a very caring boyfriend.
The band formed before working for The Muppet, minus Lips. He is the youngest of the band and joined after they were already performing for The Muppets. Zoot met Lips while he was busking, and the rest is history <3
Okay this has been sitting in my drafts for long enough. Time to post.
#the muppets#muppets#the electric mayhem#dr teeth and the electric mayhem#dr teeth muppets#janice muppets#floyd muppets#animal muppet#zoot muppets#lips muppet#polymayhem#i wish i had more ideas for lips but man in struggling to find screentime for him#i lack ideas#bonus n5f7 headcanon for people who read the tags:#Teeth is the master of receiving road head & Animal is the master of giving road head#also. mup//pets may//hem is so bad it isnt canon to me. this is an OT6 post. i will not debate my ship. just block me if u hate it.#well i did pinch a bit of what they had goung for teeth's back story. but thats literally the only decent ep in that whole show.#also if i catch any of you infantilising Animal in my notes its on sight (the block button)#THAT IS A SEMIVERBAL FULLY GROWN MAN WHO FUCKS !!! its not a hard concept#talking lollie
24 notes
·
View notes
Text
Friday Night Fightin - Pico Reference Sheet
Got a bit lazy with this one but it was still fun pfft, here's the last of the fightin RGB trio, Derrick the Dino bodyguard.
Friday Night Fightin BF Ref Sheet Friday Night Fightin GF Ref Sheet
Name: Derrick Dino
Nickname: Pico, Dee, DD
Birthday: 30th December
Age: 26
Orientation: Biromantic Aceflux
Species: Dino creature (yes)
Height: 5'6ft
Weight: 185 lbs
Voice: Riikami but a bit more guttural kekw
Relationship Status: Dating
Family: John Dino (fightin!Tankman)
Partners: Stacie Daemon (fightin!GF), Mikey Angel (fightin!BF)
Friends: Neena Arachnes (fightin!Nene), Darnell Phoenix (fightin!Darnell)
Acquaintances: Skid Skelly and Pump Pumpkin (fightin!SkidandPump), Marco Daemon (fightin!DaddyDearest), Janice Daemon (fightin!MommyMearest)
Enemies/Rivals: Darnell Phoenix (fightin!Darnell), Ayumu Obake (fightin!Senpai)
Personality: A taciturn and seemingly distant young man with a permanently annoyed face, but acts more of a tsundere when shown affection by other people, especially from his partners. He doesn't want to admit, but he can be quite the loverboy and would occasionally flirt with either Stacie or Mikey (mostly Stacie, Mic is as oblivious as a rock but that's part of the charm) from time to time. When fighting however, he would become a lot more trigger happy to shoot a bullet through your skull and acts a lot more aggressive and expressive. He takes his role as a bodyguard half seriously at first, but he gets more invested in his job overtime as he fell in love with Stacie and later Mic.
Personality flaw: Whenever something irks him, he becomes more antsy, impulsive and hot headed about it. He has a very hard time trusting others around him, especially strangers and people he doesn't like and would be more protective of his partners. Loud screams and epileptic lights reverts his brain back to the time of the school shooting during middle school, causing himself to hyperventilate and huddle in a dark area or a corner and will instinctively shoot anyone that would suddenly touch him. He's slowly getting better from it, though there are times he would regressed back progress if the trigger was severe.
Likes: Vocaloid, Crunk hip hop, working out at the gym, spending time with his partners, card games, initiating fights, compete in tournament battles with Mic, showing his muscles and physical flexibility, but likes to wear baggy clothing when spending alone time with Mic, hamburgers, Pepsi, gun shooting practice, firearms in general, showing and giving affection his partners (despite how hesitant he may be).
Dislikes: Stacie's parents (doesn't show it), loud screams and flashing lights, green tea, coke (joking), his impulsivity, being vulnerable, waiting.
Powers: Claw slashes, fists and dropkicks (basic combo attacks), Bazooka missiles! (Heavy combo attacks), Air dash and wall climb (launches himself in the air with a bazooka as a double jump), Bullet spray (fires his Uzi's at a certain direction), Claws strike (Pivots and slashes with all three claw hands at the target, Strength boost (Temporarily makes his attacks stronger and faster), Jelly Bones (Is able to contort and bend his body with relative ease).
Backstory (summary): John Dino was definitely a strict father, being a retired military officer and all. Now he works under the Daemon family as a bodyguard and brought him along to get life and work experience at a young age, which was when he met Stacie. At first he didn't really like her, but that soon change down the line as he would spend days together with her, finding her nice to be around now. Since Stacie was still homeschooled he would the mornings and afternoons at his own middle school with his other friends Neena and Darnell who would also visit Stacie from time to time when walking home together. One day without warning, all hell broke loose at the school. Fellow students were screaming and dying as light bulbs would break one by one from the stray bullets, Derrick think of anything else other than to survive (I will not go further than that, I'm not good at writing devastating scenes). Years passed, the memory was still fresh in his mind but kept suppressing it even during college years, and even after meeting Mic. However, he's finally trying to make a change to it as of late, maybe it won't be so bad. So long as he's with his loving partners, he'll definitely be fine.
Additional notes:
He's farsighted so he would wear reading glasses when needed, but rarely wear them.
Man is a love bug in denial, cuddle him and he will wrap around in his appendages in seconds.
Wears silver ear piercings, usually the thicker kinds
Likes contorting his body in odd places to spook his partners and friends.
Strangely likes to hang upside down when bored.
17 notes
·
View notes
Text
New Post has been published on Strange Hoot - How To’s, Reviews, Comparisons, Top 10s, & Tech Guide
New Post has been published on https://strangehoot.com/how-to-do-regression-analysis-in-excel/
How to Do Regression Analysis in Excel

Regression Analysis is a technique to forecast the relationship between data variables of entities such as customers, orders, transactions etc. These are the parameters based on which business decisions can be taken for sales, finance and marketing operations. To understand this in a simpler way, let us take some examples.
Example 1 – Risk Analytics in Retail Banking
Credit risk scores of customers are built by the banking industry to predict the customer’s delinquency behavior and to evaluate credit worthiness of each customer while processing loan applications.
Example 2 – Marketing Analytics in eCommerce
To predict customer’s buying patterns based on the customer’s past transactions from an online portal. The e-commerce industry is highly competitive so they use analytics to predict customer’s purchasing patterns.
Example 3 – Pricing Analytics in Stock Market
Stock brokers and investors use analytics to forecast stock pricing based on the past 52-week pricing trend and many other parameters. The analytics helps them to make the decisions on buying or selling the stocks based on the future pricing predictions.
Example 4 – Behavioral Analytics in Human Resource Industry
Human resource departments / companies use analytics to predict employees’ behavioural patterns that will enable them to decide the increment or promotion to be given on a yearly basis.
Regression Analysis Basics
Before we go for how to do regression in Excel taking a case study, let us understand the dataset and its components.
Population vs. Sample
Population is referred to as all members of a defined group that are considered for studying information on data driven decisions. For example – Current inflation rates of EU countries.
Sample is a part of “population”. It can be biased or unbiased. For example – Current inflation rates of EU countries having per capita income < 50000 EURO per annum.
Types of Data Variables
The below diagram shows types of data variables under each category.
Data Snapshot
The table below gives an example of data snapshot with different data variables.
#Cust.NameCust. IDNo.Of credi cardsGenderMaritalStatusAgeAnnualSalaryMonthly Credit Card Usage1Josh1116695FNeverMarried4388,001Low2John2231126MMarried24592,330Low3Alen1891234MDivorced50272,304Low4Chaya1716903FMarried37140,400Low5Dandre166256MNeverMarried23105, 234Low6Justin1491717MDivorced34358,534Low7Neil1692545MMarried36510,321Low8Emily1497712FNever Married26164,732Low9Janice1756462FMarried35103,345Low10Farhan1706394MNever Married63724,788Low11Tony1131363MMarried70105,450Low
Let us understand the data variables available in the above table.
#Cust.NameCust. IDNo.of credit cardsGenderMaritalStatusAgeAnnualSalaryMonthly Credit Card Usage–––Numerical (Discrete)Categorical (Binary)Categorical (Nominal)Numerical (Discrete)Numerical (Continuous)Categorical (Ordinal)
Summarizing Data
The next step is to summarize data in a presentable format that will give the report on which analysis can be done. The ways to summarize the data are as follows:
Frequency Distribution – this is a simple way of counting distinct discrete values. For example – Number of credit cards owned by 3000 customers as sample data. Can be shown as tabular format or as a graph using Excel.
Steps to do this in Excel:
Go to the Data tab in your Excel Worksheet.
Click Advanced. The dialog is shown
Go to the Number of Cards column. Sort them into ascending order of values.
Insert the formula in # of customers to set the frequency.
You will get the values as below.
Go to the Insert tab. Click the Column icon. Select the 2-D Chart.
The chart will be generated and shown as below.
Grouped Frequency Distribution – this is a method to summarize discrete variables having a large number of observations and range of values. For example – Number of customers falling under different salary ranges or groups.
Follow the steps in Excel as per instructions given in the screen below.
Cumulative Frequency Distribution – this is obtained by accumulating the frequencies to give the total number of observations up to and including the value or group in question. Illustration as per screen below.
Steps to calculate the cumulative frequency distribution are give in the screen below.
Stem Leaf Diagram – this technique is used in a limited set of data. For a large set of data, this method is not used. Example : Given age of 20 individuals in years.
Line Plots – this technique is also not suitable for a large set of data. It is not extensively used in the industry. Example: Given test scores of 20 students.
Measure of Central Tendency – Median
The central tendency measure is robust to the effects of extreme observations.
The median is a value, which splits the data set into two equal halves. Example – Calculate the median for a sample of 3000 individuals having credit cards along with demonstration of extreme observations.
Measure of Central Tendency – Mode
It is the value which occurs with the greatest frequency or the most typical value. For Example, finding the mode for a sample of 3000 individuals having credit cards. Excel has an in-built function “Mode” for granular data. For summarized data, it can be found easily by visual inspection. Illustration as below.
Measure of Spread
The different measures of spread are –
Variance and Standard Deviation – below screen shows how to calculate the values.
The Range – It is a very simple measure of spread defined, as its name suggests, by the difference between the largest and smallest observations in the data set.
The Inter quartile range – the quartiles divide a set of data into four quarters. They are denoted by Q1, Q2 and Q3. Q1 is called the lower quartile and Q3 the upper quartile. Following image shows how to calculate Inter quartile Range for a sample of 3000 individuals having credit cards.
Symmetry and Skewness of data
It represents the shape of the distribution of a dataset, that is, whether it is symmetric or skewed to one side or the other.The approximate shape of a distribution can be determined by looking at a histogram. The below diagram shows mean, median, mode and variance for symmetric and skewed data.
Tabular representation of data as shown below.
After evaluating the dataset by the above measures and techniques, a regression analysis can be conducted. Now, the question is which type of regression model is required for predictions.
The next section describes the measures that will state the relationship between the data variables and determine how strong the relationship is between identified variables.
Regression Analysis Models
Following are different techniques or formulas that are used to do regression analysis. These techniques are nothing but different formulas or equations that are used to determine the relationship between X and Y variables.
Covariance and correlation coefficient are the measures used in regression analysis.
Covariance is a measure that helps to find out the direction of relationship between x variable and y variables. In simple terms, what happens to y when x increases or decreases.
Following equation is used to determine the covariance measure.
Correlation coefficient is the measure that tells how strong the linear relation is between X and Y variables. It is termed as r value that determines the quality of a model. The parameter is represented by p or r values.
Population correlation is denoted by ρ (rho)
Sample correlation is denoted by r.
Following equation is used to determine the correlation coefficient measure for population data.
In real life data analytics, the correlation measure is calculated on sampling distribution. There are a couple of reasons why the sampling is used for analytics.
The physical impossibility of checking all items in the population.
The cost of studying all the items in a population.
The sample results are usually adequate.
Contacting the whole population would often be time consuming.
Features of ρ and r
Unit free and ranges between -1 and 1
The closer to -1, the stronger the negative linear relationship
The closer to 1, the stronger the positive linear relationship
The closer to 0, the weaker the linear relationship
Negative Linear Relationship
Positive Linear Relationship
Relationship Not Linear
No Relationship
Tools used to do Regression Analysis
Following software tools are the most used in performing Regression Analysis. The oldest tool is Microsoft Excel.
SAS (www.sas.com/)
SPSS (http://www-01.ibm.com/software/analytics/spss/ )
R (http://www.r-project.org/)
Microsoft Excel (http://office.microsoft.com/en-in/)
As the spectrum of analytics is widened, dataset volume has increased, the data scientists have started using Python.
We will see a case study to run regression analysis in Excel.
Case Study
An Auto Insurance company having the data of “Loss” amount and policy related information. The company wants to know the factors responsible for losses in multivariate fashion using a regression model.
Prior to running the regression model, following things needs to be in place.
Variable identification –
Identifying the dependent (response) variable.
Identifying the independent (explanatory) variables.
Variable categorization (e.g. Numeric, Categorical, Discrete, Continuous etc.)
Creation of Data Dictionary
Response variable exploration
Distribution analysis
Percentiles
Variance
Frequency distribution
Outlier treatment
Identify the outliers/threshold limit
Cap/floor the values at the thresholds
Independent variable analyses
Identify the prospective independent variables (that can explain response variable)
Bivariate analysis of response variable against independent variables
Variable treatment /transformation
Grouping of distinct values/levels
Mathematical transformation e.g. log, splines etc.
After performing the above data related activities, the last step is iterative in terms of running the regression.
Fitting the regression (first time)
Check for correlation between independent variables
This is to take care of Multicollinearity
Variable selection
Check for the most suitable transformed variable
Select the transformation giving the best fit
Reject the statistically insignificant variables
Fitting the regression (iteration)
Analysis of results
Model comparison
Model performance check
Actual vs Predicted comparison
Steps to do regression analysis in Excel
We will perform the following steps in Excel with reference to the case study explained in the section above.
Data Analysis
Step 1. Snapshot of Data and Data Description
The above data contains policy holders’ and loss amount information (variables)
Policy Number
Age
Years of Driving Experience
Number of Vehicles
Gender
Married
Vehicle Age
Fuel Type
Losses (Dependent/Response Variable)
A Data Dictionary is formed as below.
Sl #Variable NameVariable DescriptionValues StoredVariable Type1Policy NumberUnique Policy NumberUnique value identifying the policyIdentifier2AgeAge of Policy holder16, 17,…,70Numerical (Discrete)3Years of Driving ExperienceYears of Driving Experience of the Policy holder0,1,….,53Numerical (Discrete)4Number of VehiclesNumber of Vehicles insured under the policy1,2,3,4Numerical (Discrete)5GenderGender of the Policy holderF, MCategorical (Binary)6MarriedMarital status of the Policy holderMarried, SingleCategorical (binary)7Vehicle AgeAge of vehicle insured under the policy0,1,…,15Numerical (Discrete)8Fuel TypeFuel type of the vehicle insuredD, PCategorical (Binary)9LossesLoss amount claimed under the policyRange: 13- 3500Numerical (Continuous)
Step 2. Frequency Distribution – Loss amount
Step 3. Calculate Capped Losses using grouped frequency distribution
Step 3. Perform variable analysis in Excel. The first bivariate profiling we will do for Age vs. Capped Loss for policies.
Step 4. The second bivariate profiling we will do for Years of Driving Experience vs. Capped Loss for policies.
Step 5. The next bivariate profiling we will do for Gender vs. Capped Loss for policies.
Step 6. The next bivariate profiling we will do for Marital Status vs. Capped Loss for policies.
Step 7: The next bivariate profiling we will do for Vehicle Age vs. Capped Loss for policies.
Step 8: The next bivariate profiling we will do for Vehicle Age Band vs. Capped Loss for policies.
Step 9: The next bivariate profiling we will do for Vehicles vs. Capped Loss for policies.
Step 10: The next bivariate profiling we will do for Fuel Type vs. Capped Loss for policies.
Preparing Excel for Regression Analysis
Step 1: Open Office icon from the toolbar.
Step 2: Go to Excel Options.
Step 3: Click Add-Ins.
Step 4: From the Manage drop-down, select Excel Add-ins.
Step 5: Select the Analysis Toolpak check box and the Solver Add-in check box.
Step 6: Select the Data tab. Click Data Analysis.
Step 7: In the Data Analysis dialog box, click Regression.
Step 8: Select the data cell reference range. Click OK.
Step 9: See the summary results as below.
Step 10: Picking up the variables based on the following activities.
We will perform the following tests to select by running :
Multicollinearity
Banding of variables
Statistical significance of variables tested
List of independent variables:
Age
Age Band
Years of Driving Experience
Number of Vehicles
Gender
Married
Vehicle Age
Vehicle Age Band
Fuel Type
“Age” and “Years of Driving Experience” are highly correlated (Correlation Coefficient = 0.9972). We can use either of the variables in regression.
Fit two separate models using either of the variables one at a time. Check for goodness of fit (R2 in this case). The variable producing higher R2 gets accepted.
Age
Regression Statistics (Age)Multiple R0.475766R Square0.226354Adjusted R Square0.226303Standard Error201.2306Observations15290
Years of Driving Experience
Regression Statistics(Yrs Driving Experience)Multiple R0.475273R Square0.225885Adjusted R Square0.225834Standard Error201.2916Observations15290
R2 for Age > R2 for Years of Driving Experience
Reject Years of Driving Experience
Banding of Variables
Investigate whether to use “Age” or “Age band”
Fit regression independently using “Age” and “Age Band”
Before fitting regression, “Age Band” needs to be converted to numerical form from categorical. Replace “Age Band” values with “Average Age” for the particular band.
Age BandSum of Age# PoliciesAverage Age16-25 93,770.0 4,563.0 20.6 26-59 270,793.0 6,384.0 42.4 60+ 282,636.0 4,343.0 65.1
Regressions results using “Age” and “Average Age”
Regression Statistics (Age)Multiple R0.475766R Square0.226354Adjusted R Square0.226303Standard Error201.2306Observations15290
Regression Statistics (Average Age)Multiple R0.509969R Square0.260068Adjusted R Square0.26002Standard Error196.7971Observations15290
R2 for Average Age > R2 for Age
Select “Average Age”
Investigate whether to use “Vehicle Age” or “Vehicle Age band”
Fit regression independently using “Vehicle Age” and “Vehicle Age Band”
Before fitting regression, “Vehicle Age Band” needs to be converted to numerical form from categorical. Replace “Vehicle Age Band” values with “Vehicle Average Age” for the particular band.
Vehicle Age BandSum of Vehicle Age# PoliciesAverage Vehicle Age0-5 9,229 3,688 2.506-10 44,298 5,523 8.0211+ 78,819 6,079 12.97
Regressions results using “Vehicle Age” and “Average Vehicle Age”
Regression Statistics (Vehicle Age)Multiple R0.289431325R Square0.083770492Adjusted R Square0.083710561Standard Error218.9903277Observations15290
Regression Statistics (Average Vehicle Age)Multiple R0.303099405R Square0.09186925Adjusted R Square0.091809848Standard Error218.0203272Observations15290
R2 for Average Vehicle Age > R2 for Vehicle Age
Select “Average Vehicle Age”
Now, we have identified the variables that can be used in fitting the regression model.
Age Band in the form of “Average Age” of the band (selected out of “Age” and “Age Band”). Also got selected over “Years of Driving Experience”.
Number of Vehicles
Gender
Married
Vehicle Age Band in the form of “Average Vehicle Age” of the band (selected out of “Vehicle Age” and “Vehicle Age Band”).
Fuel Type
Step 11: Categorical variables to be converted to their numerical equivalent (0,1)
Gender (F = 0 and M = 1)
Married (Married = 0 and Single = 1)
Fuel Type (P = 0, D = 1)
Snapshot of the final data on which we will run the multivariate regression.
Step 11: Run Regression.
Summary of Output
SUMMARY OUTPUT Regression StatisticsMultiple R0.865972274R Square0.749907979Adjusted R Square0.749809794Standard Error114.4310136Observations15290ANOVA dfSSMSFSignificance FRegression6600073213.5100012202.37637.7510880Residual15283200122584.413094.45688Total15289800195798 CoefficientsStandard Errort StatP-valueLower 95%Upper 95%Lower 95.0%Upper 95.0%Intercept624.565295.29192118.022330.00000614.19249634.93809614.19249634.93809Avg Age-5.559740.06546-84.938890.00000-5.68804-5.43144-5.68804-5.43144Number of Vehicles0.178750.970390.184200.85386-1.723332.08082-1.723332.08082Gender Dummy50.883261.8908126.910840.0000047.1770554.5894747.1770554.58947Married Dummy78.398371.9214840.801060.0000074.6320482.1646974.6320482.16469Avg Vehicle Age-15.142200.26734-56.639870.00000-15.66623-14.61818-15.66623-14.61818Fuel Type Dummy267.935592.7484597.486140.00000262.54830273.32287262.54830273.32287
Results Interpretation
Significant Test
Significance test of coefficients based on Normal distribution
H0: b is no different that 0 (i.e. 0 is the coefficient when the variable is not included in regression)
H1: b is different than 0
Test statistic, Z = (b-0)/σ (at 95% two tailed confidence interval, Z = 1.96)
Confidence interval = (b – 1.96 * σ, b + 1.96 * σ)
For the variable to be significant, the interval must not contain “0”.
Example1: Avg Age.
Confidence interval = (-5.560-1.96*0.065, -5.560+1.96*0.065) = (-5.688, -5.431)
No zero in the interval. Hence, significant.
Example2: Number of Vehicles
Confidence interval = (0.179-1.96*0.970, 0.179+1.96*0.970) = (-1.723, 2.080)
Zero is present in the interval. Hence, insignificant.
Summary output after removing insignificant variables.
Predicted Losses = 625.004932715948 – 5.5596551344537 * Avg Age + 50.8828923910091 * Gender Dummy + 78.4016899779131 * Married Dummy -15.1420259903571 * Avg Vehicle Age + 267.935139741526 * Fuel Type Dummy
Interpretation
Takeaway
Running regression in Excel gives you more confidence on your model as you are running each step manually. It is a time consuming and lengthy process, but you get to learn more on datasets and output summary. Other tools such as R, SAS and Python give you quick results but you will not be able to know the algorithm behind the code that is executed and have given you the results.
0 notes
Text
CHL1 hypermethylation as a potential biomarker of poor prognosis in breast cancer.
CHL1 hypermethylation as a potential biomarker of poor prognosis in breast cancer. Oncotarget. 2017 Feb 02;: Authors: Martín-Sánchez E, Mendaza S, Ulazia-Garmendia A, Monreal-Santesteban I, Blanco-Luquin I, Córdoba A, Vicente-García F, Pérez-Janices N, Escors D, Megías D, López-Serra P, Esteller M, Illarramendi JJ, Guerrero-Setas D Abstract The CHL1 gene encodes a cell-adhesion molecule proposed as being a putative tumour-suppressor gene in breast cancer (BC). However, neither the underlying molecular mechanisms nor the clinical value of CHL1 downregulation in BC has been explored. The methylation status of three CpG sites in the CHL1 promoter was analysed by pyrosequencing in neoplastic biopsies from 142 patients with invasive BC and compared with that of non-neoplastic tissues. We found higher CHL1 methylation levels in breast tumours than in non-neoplastic tissues, either from mammoplasties or adjacent-to-tumour, which correlated with lower levels of protein expression in tumours measured by immunohistochemistry. A panel of five BC cell lines was treated with two epigenetic drugs, and restoration of CHL1 expression was observed, indicating in vitro dynamic epigenetic regulation. CHL1 was silenced by shRNA in immortalized but non-neoplastic mammary cells, and enhanced cell proliferation and migration, but not invasion, were found by real-time cell analysis. The prognostic value of CHL1 hypermethylation was assessed by the log-rank test and fitted in a Cox regression model. Importantly, CHL1 hypermethylation was very significantly associated with shorter progression-free survival in our BC patient series, independent of age and stage (p = 0.001). In conclusion, our results indicate that CHL1 is downregulated by hypermethylation and that this epigenetic alteration is an independent prognostic factor in BC. PMID: 28178655 [PubMed - as supplied by publisher] http://dlvr.it/NKlvy7
0 notes