#Insilico Drug Discovery Market
Explore tagged Tumblr posts
Text
"Pharma Tech: Unlocking New Horizons in Medicine and Healthcare"
In recent years, pharmaceutical technology has emerged as a cornerstone of modern healthcare, bridging the gap between scientific discovery and real-world medical solutions. With advances in artificial intelligence (AI), automation, and digital health platforms, the pharmaceutical industry is undergoing a transformation that promises to revolutionize drug development, manufacturing, and patient care. This article explores how pharma tech is reshaping the future of medicine and healthcare.
The Rise of AI and Machine Learning in Drug Discovery
Drug discovery, traditionally a time-consuming and expensive process, has been significantly accelerated by AI and machine learning (ML). Algorithms can now analyze vast datasets to identify potential drug candidates, predict their efficacy, and optimize chemical structures. For instance, AI-driven platforms have enabled researchers to shorten drug discovery timelines from years to mere months, as seen during the development of COVID-19 vaccines and treatments.
Companies like DeepMind and Insilico Medicine are using AI to model protein structures and predict drug interactions, opening doors to novel treatments for complex diseases. These advancements not only save time but also reduce costs, making life-saving medications more accessible.
Digital Therapeutics and Personalized Medicine
Pharma tech is also advancing personalized medicine, tailoring treatments to individual patients based on genetic, environmental, and lifestyle factors. Digital health platforms and wearable devices are collecting real-time data, enabling healthcare providers to deliver targeted interventions.
For example, digital therapeutics—software-based treatments—are being used to manage chronic conditions such as diabetes and mental health disorders. These tools offer personalized recommendations and monitor patient progress, enhancing treatment outcomes.
Transforming Manufacturing with Automation
Automation and advanced analytics are revolutionizing pharmaceutical manufacturing. Smart factories equipped with Internet of Things (IoT) devices and robotics ensure precision and consistency in drug production. These technologies minimize human error, enhance quality control, and streamline supply chains.
Continuous manufacturing—a process where drugs are produced in a non-stop flow—is becoming a reality thanks to pharma tech innovations. This approach reduces production time, lowers costs, and improves scalability, particularly for complex biologics and personalized treatments.
Enhancing Clinical Trials with Virtual Platforms
The clinical trial phase has historically been a bottleneck in bringing drugs to market. Virtual and decentralized clinical trials are addressing this challenge by leveraging digital platforms to recruit participants, collect data, and monitor outcomes remotely.
Telemedicine, wearable devices, and cloud-based systems are making trials more efficient and inclusive. This shift not only speeds up the research process but also ensures that diverse populations are represented, leading to more equitable healthcare solutions.
Tackling Supply Chain Challenges with Blockchain
Blockchain technology is enhancing transparency and security in the pharmaceutical supply chain. By providing a tamper-proof record of transactions, blockchain ensures the authenticity of drugs and combats counterfeit products. This is particularly crucial in global markets where counterfeit medications pose significant health risks.
Pharma tech solutions like blockchain also enable real-time tracking of shipments, ensuring timely delivery and reducing waste due to temperature excursions in cold-chain logistics.
The Future of Pharma Tech
As pharma tech continues to evolve, the integration of emerging technologies such as quantum computing, synthetic biology, and advanced analytics promises to unlock even greater possibilities. From developing precision oncology therapies to combating antibiotic resistance, the potential applications are vast.
However, challenges remain. Issues such as data privacy, regulatory compliance, and the digital divide must be addressed to ensure that these advancements benefit all patients equally. Collaboration between industry stakeholders, governments, and technology providers will be essential to overcome these hurdles.
Conclusion
Pharma tech is not just a tool for innovation; it is a catalyst for transforming healthcare at every level. By leveraging cutting-edge technologies, the pharmaceutical industry is unlocking new horizons in medicine, improving patient outcomes, and creating a more efficient and equitable healthcare system. As we stand on the cusp of this technological revolution, the future of healthcare has never looked brighter.
1 note
·
View note
Text
How Do Generative AI Solutions Empower Modern Industries?
Generative AI solutions are revolutionizing industries by enabling automation, enhancing creativity, and providing innovative tools to solve complex problems. From content creation and healthcare diagnostics to architectural design and customer service, these solutions are reshaping how businesses operate and deliver value. In this blog, we’ll explore how generative AI empowers various industries, its applications, and the transformative potential it offers.
What Are Generative AI Solutions?
Generative AI refers to artificial intelligence systems designed to create new data, images, text, audio, or other content based on training data. These solutions leverage machine learning models such as Generative Adversarial Networks (GANs) and Transformer-based models like GPT (Generative Pre-trained Transformer). They are designed to learn patterns from vast datasets and generate outputs that resemble human creativity.
Key features of generative AI solutions include:
Automation of repetitive tasks
Personalization at scale
Enhanced creativity and innovation
Efficient problem-solving capabilities
How Generative AI Empowers Modern Industries
1. Healthcare: Precision and Personalization
Generative AI is making strides in healthcare by improving diagnostics, enhancing patient care, and accelerating drug discovery.
Drug Discovery: AI models can simulate molecular compositions to generate new drug candidates, significantly reducing development time and cost. For instance, companies like Insilico Medicine use generative AI to predict the efficacy of new compounds.
Medical Imaging: Generative models assist in reconstructing high-quality medical images from incomplete or noisy scans, aiding faster diagnosis.
Personalized Medicine: By analyzing genetic data, generative AI can recommend tailored treatment plans, improving patient outcomes.
2. Entertainment: Enhanced Creativity
The entertainment industry is leveraging generative AI to streamline production and deliver innovative experiences.
Content Creation: Generative AI is used to create music, art, and even entire scripts. Tools like DALL·E generate artwork, while OpenAI's Jukebox creates original music.
Gaming: AI enhances game design by generating dynamic characters, landscapes, and storylines, offering immersive experiences.
Virtual Influencers: Brands are now creating AI-generated virtual personalities for advertising and engagement.
3. Manufacturing: Smarter Production
Generative AI is transforming manufacturing by optimizing designs and improving efficiency.
Product Design: Generative design tools like Autodesk's Fusion 360 generate multiple design iterations based on specific constraints, enabling innovation.
Predictive Maintenance: AI predicts equipment failures by analyzing sensor data, reducing downtime and maintenance costs.
Supply Chain Optimization: Generative models help forecast demand, optimize inventory, and streamline logistics.
4. Marketing and Advertising: Personalized Campaigns
Generative AI enables marketers to craft highly personalized and impactful campaigns.
Content Generation: Tools like Jasper AI and Copy.ai create marketing copy, blogs, and ads tailored to target audiences.
Customer Insights: AI generates predictive analytics to understand customer preferences and behavior.
Dynamic Ads: Generative AI creates customized ad variations to maximize audience engagement and ROI.
5. Finance: Risk Mitigation and Innovation
The finance sector benefits from generative AI through improved fraud detection, customer service, and financial modeling.
Fraud Detection: AI identifies suspicious activities by analyzing transactional data patterns.
Portfolio Optimization: Generative models simulate financial scenarios to suggest optimal investment strategies.
Chatbots: AI-powered assistants provide personalized financial advice and enhance customer service.
6. Architecture and Construction: Designing the Future
Generative AI is transforming how architects and engineers design and build structures.
Generative Design: AI-based design tools propose optimized layouts and structures, balancing cost, aesthetics, and sustainability.
Construction Automation: AI generates detailed construction plans, reducing errors and improving efficiency.
Virtual Reality (VR) Integration: Generative AI enhances VR environments, enabling stakeholders to visualize projects before construction.
7. Retail: Enhanced Shopping Experiences
Retailers are using generative AI to provide personalized shopping experiences and optimize operations.
Virtual Try-Ons: AI creates realistic simulations of customers trying products like clothing or makeup.
Inventory Management: Predictive modeling optimizes inventory levels, minimizing waste and meeting demand.
Customer Interaction: Chatbots powered by generative AI provide tailored recommendations and 24/7 support.
8. Education: Personalized Learning
Generative AI is reshaping education by creating customized learning experiences.
Curriculum Design: AI generates personalized study plans based on individual learning needs and goals.
Content Creation: Tools like Quizlet and Duolingo leverage AI to create engaging quizzes and lessons.
Tutoring: Virtual tutors powered by AI provide real-time feedback and support to learners.
9. Legal and Professional Services: Streamlined Workflows
Generative AI is streamlining operations in the legal and professional services sectors.
Document Drafting: AI generates contracts, legal briefs, and other documents with minimal human intervention.
Case Analysis: AI tools analyze past case data to predict outcomes or suggest strategies.
Compliance Automation: Generative AI automates compliance processes, reducing risks and errors.
10. Energy: Sustainable Solutions
In the energy sector, generative AI is driving sustainability and operational efficiency.
Energy Modeling: AI predicts energy demand and generates optimized distribution plans.
Renewable Energy: AI simulates designs for solar panels or wind turbines to maximize energy output.
Smart Grids: AI-enabled grids optimize power distribution and reduce energy wastage.
Challenges and Ethical Considerations
While generative AI offers immense potential, it also presents challenges:
Bias in Models: AI systems can replicate and amplify biases present in training data.
Intellectual Property Concerns: Ownership of AI-generated content remains a grey area.
Misuse Risks: Generative AI can be exploited to create deepfakes or misinformation.
Energy Consumption: Training large models requires substantial computational resources, impacting sustainability.
Addressing these challenges requires robust regulations, ethical guidelines, and continued innovation to make AI more inclusive and efficient.
The Future of Generative AI in Industries
The adoption of generative AI is expected to grow as industries continue to explore its potential. Future advancements may include:
Cross-Industry Collaboration: Generative AI solutions tailored to solve interdisciplinary challenges.
Human-AI Collaboration: Enhanced tools to support human creativity and decision-making.
Improved Accessibility: Democratizing access to generative AI technologies for small and medium-sized enterprises.
Sustainability Focus: Developing energy-efficient AI models to minimize environmental impact.
Conclusion
Generative AI solutions are reshaping the landscape of modern industries by enhancing creativity, streamlining operations, and driving innovation. From healthcare and entertainment to finance and energy, the potential of AI is vast and transformative. By addressing challenges and adopting these technologies responsibly, businesses can unlock new possibilities and stay ahead in an increasingly competitive world.
Generative AI is not just a technological advancement; it’s a cornerstone for the future of industry innovation. Are you ready to embrace it?
0 notes
Text
AI in Drug Discovery Market to Hit $7.1 Billion by 2032
The global AI in Drug Discovery Market was valued at USD 1.3 Billion in 2024 and it is estimated to garner USD 7.1 Billion by 2032 with a registered CAGR of 23.72% during the forecast period 2024 to 2032.
The report throws light on the competitive scenario of the global AI in Drug Discovery Market to know the competition at global levels. Market experts also provided the outline of each leading player of the global AI in Drug Discovery Market for the market, considering the key aspects such as the areas of operation, production, and product portfolio. In addition, the companies in the report are studied based on vital factors such as company size, market share, market growth, revenue, production volume, and profit.
The global AI in Drug Discovery Market is fragmented with various key players. Some of the key players identified across the value chain of the global AI in Drug Discovery Market include IBM Corporation (US), Microsoft (US), and Google (US), NVIDIA Corporation (US), Atomwise, Inc. (US), Deep Genomics (Canada), Cloud Pharmaceuticals (US), Insilico Medicine (US), BenevolentAI (UK), Exscientia (UK), Cyclica (Canada), BIOAGE (US), Numerate (US), NuMedii (US), Envisagenics (US), twoXAR (US), OWKIN, Inc. (US), XtalPi (US), Verge Genomics (US), and BERG LLC (US). etc. Considering the increasing demand from global markets various new entries are expected in the AI in Drug Discovery Market at regional as well as global levels.
Download AI in Drug Discovery Market Sample Report PDF: https://www.vantagemarketresearch.com/artificial-intelligence-in-drug-discovery-market-1239/request-sample
Top Competitors:
IBM Corporation (US), Microsoft (US), and Google (US), NVIDIA Corporation (US), Atomwise, Inc. (US), Deep Genomics (Canada), Cloud Pharmaceuticals (US), Insilico Medicine (US), BenevolentAI (UK), Exscientia (UK), Cyclica (Canada), BIOAGE (US), Numerate (US), NuMedii (US), Envisagenics (US), twoXAR (US), OWKIN, Inc. (US), XtalPi (US), Verge Genomics (US), and BERG LLC (US).
Understanding the Industry's Growth, has released an Updated report on the AI in Drug Discovery Market. The report is mixed with crucial market insights that will support the clients to make the right business decisions. This research will help new players in the global AI in Drug Discovery Market to sort out and study market needs, market size, and competition. The report provides information on the supply and market situation, the competitive situation and the challenges to the market growth, the market opportunities, and the threats faced by the major players.
Regional Analysis
-North America [United States, Canada, Mexico]
-South America [Brazil, Argentina, Columbia, Chile, Peru]
-Europe [Germany, UK, France, Italy, Russia, Spain, Netherlands, Turkey, Switzerland]
-Middle East & Africa [GCC, North Africa, South Africa]
-Asia-Pacific [China, Southeast Asia, India, Japan, Korea, Western Asia]
You Can Buy This Report From Here: https://www.vantagemarketresearch.com/buy-now/artificial-intelligence-in-drug-discovery-market-1239/0
Full Analysis Of The AI in Drug Discovery Market:
Key findings and recommendations point to vital progressive industry trends in the global AI in Drug Discovery Market, empowering players to improve effective long-term policies.
The report makes a full analysis of the factors driving the development of the market.
Analyzing the market opportunities for stakeholders by categorizing the high-growth divisions of the market.
Questions answered in the report
-Who are the top five players in the global AI in Drug Discovery Market?
-How will the global AI in Drug Discovery Market change in the next five years?
-Which product and application will take the lion's share of the global AI in Drug Discovery Market?
-What are the drivers and restraints of the global AI in Drug Discovery Market?
-Which regional market will show the highest growth?
-What will be the CAGR and size of the global AI in Drug Discovery Market during the forecast period?
Read Full Research Report with [TOC] @ https://www.vantagemarketresearch.com/industry-report/artificial-intelligence-ai-in-drug-discovery-market-2220
Reasons to Purchase this AI in Drug Discovery Market Report:
-Analysis of the market outlook on current trends and SWOT analysis.
-The geographic and country level is designed to integrate the supply and demand organizations that drive industry growth.
-AI in Drug Discovery Industry dynamics along with market growth opportunities in the coming years.
-AI in Drug Discovery Market value (million USD) and volume (million units) data for each segment and sub-segment.
1 year consulting for analysts along with development data support in Excel. Competitive landscape including market share of major players along with various projects and strategies adopted by players in the last five years.
Market segmentation analysis including qualitative and quantitative analysis including the impact on financial and non-economic aspects.
Complete company profiles that include performance presentations, key financial overviews, current developments, SWOT analyzes and strategies used by major AI in Drug Discovery Market players.
Check Out More Reports
Global Skincare Market: Report Forecast by 2032
Global Behavioral Mental Health Software Market: Report Forecast by 2032
Global Dual Interface Payment Card Market: Report Forecast by 2032
Global Cold Chain Market: Report Forecast by 2032
Global Food Can Market: Report Forecast by 2032
#AI in Drug Discovery Market#AI in Drug Discovery Market 2024#Global AI in Drug Discovery Market#AI in Drug Discovery Market outlook#AI in Drug Discovery Market Trend#AI in Drug Discovery Market Size & Share#AI in Drug Discovery Market Forecast#AI in Drug Discovery Market Demand#AI in Drug Discovery Market sales & price
0 notes
Text
Biosimulation Market worth $9.18 billion by 2029
The global biosimulation market is projected to reach USD 9.18 billion by 2029 from USD 4.24 billion in 2024, at a CAGR of 16.7% from 2024 to 2029. The major factors driving the growth of the biosimulation market include the high rate of clinical trial failures, the growing necessity of being able to predict drug pharmacokinetics and pharmacodynamics, as well as toxicity management. According to a research article published by the National Library of Medicine in February 2022, the drug discovery and development process takes about 10-15 years for a new drug to be approved for clinical use. And 90% of the drug candidates fail during the phases I, II, and III of clinical trials and drug approvals. The possible reasons stated for the failure include lack of clinical efficacy, unmanageable toxicity, poor drug-like properties, lack of commercial needs, and poor strategic planning. The use of biosimulation helps address these challenges to increase the chances of drug approval and facilitate swift trial processes.
Download PDF Brochure:
Browse in-depth TOC on "Biosimulation Market"
153 - Tables
54 - Figures
350 - Pages
By application, the drug discovery segment is expected to capture the largest share of the biosimulation market. This large share is attributed to the rising burden of chronic and infectious diseases, coupled with technological advancements that enhance understanding of disease mechanisms. Some other drivers for the drug discovery segment include the emphasis on personalized medicine, increased investment in drug discovery, and supportive regulatory frameworks.
Pharmaceutical and biotechnology companies are expected to hold a major share of the biosimulation market by end users. Companies have been putting in much effort in new drug discovery and development, having strong candidate drugs in their pipelines. With stringent regulatory requirements, pharma and biotech companies heavily rely on biosimulation tools to support clinical trial designs and optimize therapeutic dosing. In 2023, the US FDA approved 55 novel drug therapies, while as of 2024, 36 novel drug therapies have been approved so far, reflecting the scope of the segment and its substantial large market share.
Request Sample Pages:
The major players in the biosimulation market with a significant global presence are Certara USA. (US), Simulations Plus. (US), Dassault Systèmes (France), Schrödinger, Inc. (US), Advanced Chemistry Development, Inc. (Canada), Chemical Computing Group ULC. (Canada), Rosa & Co. LLC. (US), Genedata AG (US), Physiomics Plc (United Kingdom), In Silico Biosciences. (US), Allucent. (US), OpenEye, Cadence Molecular Sciences. (US), Cellworks Group, Inc. (US), VeriSIM Life. (US), Netabolics SRL (Italy), Charnwood Discovery (United Kingdom), The MathWorks, Inc. (US), ANSYS, Inc (US), Instem Group of Companies (United Kingdom), Insilico Medicine (US), SCM – Software Chemistry & Materials (Netherlands), BioSymetrics, Inc. (Canada), Atomwise Inc. (US), insitro. US), and Clinithink. (US). The market players have adopted strategies such as acquisitions, collaborations, partnerships, mergers, product/service launches & enhancements, and approvals to strengthen their position in the biosimulation market. The product and technology innovations have helped the market players expand globally by providing biosimulation and modeling solutions.
Certara USA.:
As a global leader in manufacturing software and services for drug discovery and development, Certara provides Model-informed Drug Development (MIDD) software solutions to support all stages of drug development, from preclinical through clinical and commercial. Its proprietary, end-to-end platform integrates generative AI technology with biosimulation, regulatory science, and market access solutions. The company boasts a strong presence in North America, Europe, and Asia Pacific. The customer base includes more than 2,400 biopharmaceutical companies, academic institutes, and regulatory agencies from 66 countries.
Dassault Systèmes:
Dassault Systèmes is a multinational software company that develops and sells 3D design software and intelligence products for modeling and simulation. The company offers a number of products and services - 3DEXCITE, 3DEXPERIENCE, 3DVIA, BIOVIA, DraftSight, CATIA, DELMIA, ENOVIA, EXALEAD, GEOVIA, NETVIBES, SIMULIA, for multiple industries, including aerospace & defence, architecture, engineering & construction, consumer goods & retail, consumer packaged goods & retail, energy, process & utilities, financial and business services, high-tech, industrial equipment, life sciences, marine & offshore, natural resources, and transportation & mobility. Dassault Systèmes operates in 140 countries with more than 194 offices across North America, Europe, Asia-Pacific, Latin America, the Middle East, and Africa, serving more than 270,000 customers.
Schrödinger:
Schrödinger is a scientific leader in developing state-of-the-art chemical simulation software for pharmaceutical and biotechnology research. It operates through two business segments: software and drug discovery. The company provides products ranging from general molecular modeling programs to a full-featured drug design software suite using ligand—and structure-based methods. Schrödinger has a geographical presence in the US, Europe, the Middle East, Africa, and Asia-Pacific.
For more information, Inquire Now!
About MarketsandMarkets™
MarketsandMarkets™ has been recognized as one of America's best management consulting firms by Forbes, as per their recent report.
MarketsandMarkets™ is a blue ocean alternative in growth consulting and program management, leveraging a man-machine offering to drive supernormal growth for progressive organizations in the B2B space. We have the widest lens on emerging technologies, making us proficient in co-creating supernormal growth for clients.
Earlier this year, we made a formal transformation into one of America's best management consulting firms as per a survey conducted by Forbes.
The B2B economy is witnessing the emergence of $25 trillion of new revenue streams that are substituting existing revenue streams in this decade alone. We work with clients on growth programs, helping them monetize this $25 trillion opportunity through our service lines - TAM Expansion, Go-to-Market (GTM) Strategy to Execution, Market Share Gain, Account Enablement, and Thought Leadership Marketing.
Built on the 'GIVE Growth' principle, we work with several Forbes Global 2000 B2B companies - helping them stay relevant in a disruptive ecosystem. Our insights and strategies are molded by our industry experts, cutting-edge AI-powered Market Intelligence Cloud, and years of research. The KnowledgeStore™ (our Market Intelligence Cloud) integrates our research, facilitates an analysis of interconnections through a set of applications, helping clients look at the entire ecosystem and understand the revenue shifts happening in their industry.
Contact:
Mr. Rohan Salgarkar
MarketsandMarkets Inc.
1615 South Congress Ave.
Suite 103, Delray Beach, FL 33445
USA : 1-888-600-6441
UK +44-800-368-9399
Email: [email protected]
Visit Our Website: https://www.marketsandmarkets.com/
0 notes
Text
Beyond the Hype: Unveiling the Real Impact of Generative AI in Drug Discovery
New Post has been published on https://thedigitalinsider.com/beyond-the-hype-unveiling-the-real-impact-of-generative-ai-in-drug-discovery/
Beyond the Hype: Unveiling the Real Impact of Generative AI in Drug Discovery
Since Insilico Medicine developed a drug for idiopathic pulmonary fibrosis (IPF) using generative AI, there’s been a growing excitement about how this technology could change drug discovery. Traditional methods are slow and expensive, so the idea that AI could speed things up has caught the attention of the pharmaceutical industry. Startups are emerging, looking to make processes like predicting molecular structures and simulating biological systems more efficient. McKinsey Global Institute estimates that generative AI could add $60 billion to $110 billion annually to the sector. But while there’s a lot of enthusiasm, significant challenges remain. From technical limitations to data quality and ethical concerns, it’s clear that the journey ahead is still full of obstacles. This article takes a closer look at the balance between the excitement and the reality of generative AI in drug discovery.
The Hype Surrounding Generative AI in Drug Discovery
Generative AI has captivated the imagination of the pharmaceutical industry with its potential to drastically accelerate the traditionally slow and expensive drug discovery process. These AI platforms can simulate thousands of molecular combinations, predict their efficacy, and even anticipate adverse effects long before clinical trials begin. Some industry experts predict that drugs that once took a decade to develop will be created in a matter of years, or even months with the help of generative AI.
Startups and established companies are capitalizing on the potential of generative AI for drug discovery. Partnerships between pharmaceutical giants and AI startups have fueled dealmaking, with companies like Exscientia, Insilico Medicine, and BenevolentAI securing multi-million-dollar collaborations. The allure of AI-driven drug discovery lies in its promise of creating novel therapies faster and cheaper, providing a solution to one of the industry’s biggest challenges: the high cost and long timelines of bringing new drugs to market.
Early Successes
Generative AI is not just a hypothetical tool; it has already demonstrated its ability to deliver results. In 2020, Exscientia developed a drug candidate for obsessive-compulsive disorder, which entered clinical trials less than 12 months after the program started — a timeline far shorter than the industry standard. Insilico Medicine has made headlines for discovering novel compounds for fibrosis using AI-generated models, further showcasing the practical potential of AI in drug discovery.
Beyond developing individual drugs, AI is being employed to address other bottlenecks in the pharmaceutical pipeline. For instance, companies are using generative AI to optimize drug formulations and design, predict patient responses to specific treatments, and discover biomarkers for diseases that were previously difficult to target. These early applications indicate that AI can certainly help solve long-standing challenges in drug discovery.
Is Generative AI Overhyped?
Amid the excitement, there is growing skepticism regarding how much of generative AI’s hype is grounded versus inflated expectations. While success stories grab headlines, many AI-based drug discovery projects have failed to translate their early promise into real-world clinical results. The pharmaceutical industry is notoriously slow-moving, and translating computational predictions into effective, market-ready drugs remains a daunting task.
Critics point out that the complexity of biological systems far exceeds what current AI models can fully comprehend. Drug discovery involves understanding an array of intricate molecular interactions, biological pathways, and patient-specific factors. While generative AI is excellent at data-driven prediction, it struggles to navigate the uncertainties and nuances that arise in human biology. In some cases, the drugs AI helps discover may not pass regulatory scrutiny, or they may fail in the later stages of clinical trials — something we’ve seen before with traditional drug development methods.
Another challenge is the data itself. AI algorithms depend on massive datasets for training, and while the pharmaceutical industry has plenty of data, it’s often noisy, incomplete, or biased. Generative AI systems require high-quality, diverse data to make accurate predictions, and this need has exposed a gap in the industry’s data infrastructure. Moreover, when AI systems rely too heavily on historical data, they run the risk of reinforcing existing biases rather than innovating with truly novel solutions.
Why the Breakthrough Isn’t Easy
While generative AI shows promise, the process of transforming an AI-generated idea into a viable therapeutic solution is a challenging task. AI can predict potential drug candidates but validating those candidates through preclinical and clinical trials is where the real challenge begins.
One major hurdle is the ‘black box’ nature of AI algorithms. In traditional drug discovery, researchers can trace each step of the development process and understand why a particular drug is likely to be effective. In contrast, generative AI models often produce outcomes without offering insights into how they arrived at those predictions. This opacity creates trust issues, as regulators, healthcare professionals, and even scientists find it difficult to fully rely on AI-generated solutions without understanding the underlying mechanisms.
Moreover, the infrastructure required to integrate AI into drug discovery is still developing. AI companies are working with pharmaceutical giants, but their collaboration often reveals mismatched expectations. Pharma companies, known for their cautious, heavily regulated approach, are often reluctant to adopt AI tools at a pace that startup AI companies expect. For generative AI to reach its full potential, both parties need to align on data-sharing agreements, regulatory frameworks, and operational workflows.
The Real Impact of Generative AI
Generative AI has undeniably introduced a paradigm shift in the pharmaceutical industry, but its real impact lies in complementing, not replacing, traditional methods. AI can generate insights, predict potential outcomes, and optimize processes, but human expertise and clinical testing are still crucial for developing new drugs.
For now, generative AI’s most immediate value comes from optimizing the research process. It excels in narrowing down the vast pool of molecular candidates, allowing researchers to focus their attention on the most promising compounds. By saving time and resources during the early stages of discovery, AI enables pharmaceutical companies to pursue novel avenues that may have otherwise been deemed too costly or risky.
In the long term, the true potential of AI in drug discovery will likely depend on advancements in explainable AI, data infrastructure, and industry-wide collaboration. If AI models can become more transparent, making their decision-making processes clearer to regulators and researchers, it could lead to a broader adoption of AI across the pharmaceutical industry. Additionally, as data quality improves and companies develop more robust data-sharing practices, AI systems will become better equipped to make groundbreaking discoveries.
The Bottom Line
Generative AI has captured the imagination of scientists, investors, and pharmaceutical executives, and for good reason. It has the potential to transform how drugs are discovered, reducing both time and cost while delivering innovative therapies to patients. While the technology has demonstrated its value in the early phases of drug discovery, it is not yet prepared to transform the entire process.
The true impact of generative AI in drug discovery will unfold over the coming years as the technology evolves. However, this progress depends on overcoming challenges related to data quality, model transparency, and collaboration within the pharmaceutical ecosystem. Generative AI is undoubtedly a powerful tool, but its true value depends on how it’s applied. Although the current hype may be exaggerated, its potential is genuine — and we are only at the beginning of discovering what it can accomplish.
#ADD#adoption#ai#AI for molecular prediction#AI in biotechnology#AI in drug development#AI models#AI platforms#AI systems#ai tools#Algorithms#applications#approach#Article#Artificial Intelligence#attention#billion#Biology#biomarkers#biotechnology#black box#box#challenge#change#Collaboration#Companies#complexity#data#data quality#data-driven
0 notes
Text
Generative AI in Healthcare: Revolutionizing the Future of Medicine

The healthcare industry is undergoing a transformative revolution with the advent of generative AI, a cutting-edge technology that is reshaping the way medical professionals diagnose, treat, and manage patient care. As healthcare systems across the globe grapple with challenges such as rising costs, increasing patient demands, and the need for personalized treatments, generative AI in healthcare is emerging as a powerful tool to address these issues effectively.
What is Generative AI?
Generative AI refers to a subset of artificial intelligence that uses machine learning models to generate new content, data, or solutions based on existing information. Unlike traditional AI systems that follow predefined rules, generative AI models learn patterns from large datasets and generate new outputs that mimic human-like creativity and reasoning. This technology has gained significant traction in various industries, including healthcare, due to its ability to process complex data and generate insights that were previously unattainable.
The Role of Generative AI in Healthcare
1. Enhancing Diagnostics and Early Detection
Generative AI in healthcare is revolutionizing diagnostics by analyzing vast amounts of medical data to identify patterns and anomalies that might go unnoticed by human eyes. Advanced algorithms can process medical images, such as X-rays, MRIs, and CT scans, to detect diseases like cancer, cardiovascular conditions, and neurological disorders at an early stage. For example, Google's DeepMind has developed an AI model capable of diagnosing over 50 eye diseases with an accuracy comparable to that of experienced ophthalmologists. By enabling early detection, generative AI is enhancing patient outcomes and reducing the burden on healthcare systems.
2. Personalized Treatment Plans
The era of one-size-fits-all treatments is gradually fading away, thanks to the capabilities of AI in healthcare. This technology enables the creation of personalized treatment plans by analyzing a patient's genetic makeup, medical history, lifestyle, and other relevant factors. By tailoring treatments to individual patients, healthcare providers can optimize therapy effectiveness and minimize adverse effects. For instance, IBM's Watson for Oncology uses generative AI to recommend personalized cancer treatment plans by analyzing vast volumes of medical literature and patient data, helping oncologists make informed decisions.
3. Drug Discovery and Development
Generative AI is significantly accelerating the drug discovery and development process, which traditionally takes years and billions of dollars to complete. By simulating molecular interactions and predicting the effectiveness of potential drug compounds, generative AI models can identify promising candidates for further testing. This technology has the potential to revolutionize the pharmaceutical industry by reducing the time and cost associated with bringing new drugs to market. Notably, Insilico Medicine, a biotech company, successfully used generative AI to design a new drug compound for fibrosis, showcasing the immense potential of this technology in drug discovery.
4. Optimizing Patient Care and Management
The integration of generative AI in healthcare is optimizing patient care and management by streamlining administrative tasks and enhancing patient engagement. AI-powered chatbots and virtual assistants can handle appointment scheduling, answer patient queries, and provide medication reminders, freeing up healthcare professionals to focus on more complex tasks. Furthermore, generative AI can analyze patient data to predict disease progression, allowing healthcare providers to intervene proactively and provide timely interventions.
5. Improving Surgical Precision
Generative AI is making significant strides in surgical precision by assisting surgeons with real-time insights and guidance during complex procedures. AI-powered robotic systems can analyze preoperative data and assist surgeons in making precise incisions, minimizing the risk of human error and improving surgical outcomes. These advanced systems enhance surgical accuracy and reduce recovery times, benefiting both patients and healthcare providers.
Challenges and Ethical Considerations
While generative AI holds immense promise in transforming healthcare, it also presents challenges and ethical considerations that must be addressed:
1. Data Privacy and Security
The use of generative AI in healthcare relies on vast amounts of patient data, raising concerns about data privacy and security. Safeguarding sensitive patient information is crucial to prevent data breaches and maintain patient trust. Healthcare organizations must implement robust cybersecurity measures and adhere to strict data protection regulations to mitigate these risks.
2. Bias and Fairness
Generative AI models can inadvertently inherit biases from the data they are trained on, leading to disparities in healthcare outcomes. Ensuring fairness and equity in AI-driven healthcare systems requires continuous monitoring and validation to eliminate biases and promote unbiased decision-making.
3. Regulatory Compliance
The rapid advancement of generative AI in healthcare has outpaced regulatory frameworks, creating a need for updated regulations that address AI-specific challenges. Regulatory bodies must collaborate with healthcare providers and AI developers to establish guidelines that ensure the safe and ethical use of generative AI technologies.
4. Human-AI Collaboration
The integration of generative AI in healthcare should complement, not replace, human expertise. Healthcare professionals must be trained to work alongside AI systems and interpret AI-generated insights effectively. A collaborative approach between humans and AI is essential to achieve optimal patient outcomes.
The Future of Generative AI in Healthcare
The future of generative AI in healthcare is promising, with continued advancements poised to reshape the industry. As AI technologies evolve, we can expect even more sophisticated models that enhance diagnostic accuracy, personalize treatments, and drive innovations in medical research. Here are some potential future developments:
1. AI-Driven Predictive Analytics
Generative AI's predictive capabilities will advance further, enabling healthcare providers to anticipate disease outbreaks, predict patient needs, and allocate resources efficiently. Predictive analytics can revolutionize public health strategies and improve healthcare delivery on a global scale.
2. Telemedicine and Remote Monitoring
Generative AI will play a pivotal role in expanding telemedicine services and remote patient monitoring. AI-powered platforms can analyze patient data in real time, providing healthcare providers with actionable insights and enabling remote interventions when necessary. This development is particularly significant in underserved and rural areas with limited access to healthcare facilities.
3. AI-Enhanced Medical Research
Generative AI will accelerate medical research by analyzing vast datasets and generating hypotheses for further investigation. This technology can uncover new insights into disease mechanisms, identify potential drug targets, and facilitate collaborations between researchers worldwide.
4. AI-Driven Mental Health Support
Generative AI has the potential to revolutionize mental health support by offering personalized interventions and real-time assistance. AI-powered chatbots and virtual therapists can provide immediate support to individuals in need, bridging the gap between traditional therapy sessions.
Conclusion
Generative AI in healthcare is ushering in a new era of medical innovation, transforming the way we diagnose, treat, and manage patient care. This technology holds the potential to enhance diagnostic accuracy, personalize treatments, and accelerate drug discovery, ultimately improving patient outcomes and reducing healthcare costs. However, it is essential to address the challenges and ethical considerations associated with generative AI to ensure its responsible and equitable implementation. As the healthcare industry continues to embrace generative AI, collaboration between humans and AI will be key to unlocking the full potential of this transformative technology.
0 notes
Text
Artificial Intelligence in Drug Discovery Market Forecast 2024: Trends and Analysis

Artificial Intelligence in Drug Discovery Market Outlook, Scope & Overview:
Industry reports indicate that the global artificial intelligence (AI) in drug discovery market was valued at USD 1.42 billion in 2023 and is projected to reach USD 11.37 billion by 2031, growing at a CAGR of 29.7% over the forecast period 2024-2031.
Technological Advancements to Drive Growth of Global AI in Drug Discovery Market
The adoption of AI technologies in drug discovery will continue to influence global market revenues. Pharmaceutical companies and research institutions are increasingly utilizing AI to enhance the efficiency of drug discovery processes, reduce the time and cost associated with drug development, and improve the success rate of new drug candidates.
As a product segment, machine learning algorithms currently hold a significant share of the global AI in drug discovery market. This segment is anticipated to grow at a year-over-year rate of 29.7% in 2024 over 2023 and reach USD 11.37 billion in revenues by 2031. The increasing application of AI in target identification, lead optimization, and clinical trials is expected to drive market growth.
AI in Drug Discovery Solutions – Market Dynamics
Drivers:
AI in drug discovery solutions are witnessing significant growth in the global market due to their ability to analyze large datasets, predict drug-target interactions, and identify potential drug candidates more efficiently than traditional methods. The growing adoption of AI by pharmaceutical companies to accelerate drug development pipelines, coupled with advancements in AI algorithms and computational power, are key factors driving the adoption of AI in drug discovery worldwide. Additionally, the rising prevalence of chronic diseases and the need for personalized medicine are further propelling market growth.
Restraints:
Despite the growth potential, challenges such as data privacy concerns, high implementation costs, and the need for high-quality, annotated datasets are hindering the widespread adoption of AI in drug discovery solutions. Moreover, regulatory uncertainties and the complexity of integrating AI with existing drug discovery workflows pose additional challenges to market expansion.
AI in Drug Discovery Solutions – Market Outlook
The proven benefits of AI in improving the efficiency and success rates of drug discovery processes have contributed to the market's growth. AI in drug discovery solutions are expected to witness increased adoption across major markets, including North America, Europe, and Asia Pacific, driven by advancements in AI technology and the growing focus on precision medicine and personalized healthcare.
Global AI in Drug Discovery Market
The rise in demand for AI in drug discovery solutions in developed and emerging markets is expected to drive market growth over the forecast period. North America currently holds a significant market share in the global AI in drug discovery market, with the US being a key contributor to market revenues. Europe and Asia Pacific regions are also experiencing rapid adoption of AI solutions in drug discovery, supported by increasing investments in AI research and development and favorable regulatory frameworks.
Key Players in the AI in Drug Discovery Solutions Market
Leading companies in the AI in drug discovery solutions market include IBM Watson Health, BenevolentAI, Insilico Medicine, and Atomwise, Inc. These companies are at the forefront of developing and commercializing advanced AI platforms for various drug discovery applications, including target identification, lead optimization, and clinical trial design.
In conclusion, the global AI in drug discovery market is poised for substantial growth over the forecast period, driven by technological advancements, increasing healthcare digitization, and the expanding adoption of AI solutions in drug development and personalized medicine.
Other Trending Reports
Cell Banking Outsourcing Market Share by Company
Artificial Intelligence In Genomics Market Share by Company
Softgel Capsule Market Share by Company
Anxiety Disorder Treatment Market Share by Company
0 notes
Text
Stem Cells Market will grow at highest pace owing to growing R&D activities in regenerative medicine

Stem cells are undifferentiated biological cells that can differentiate into specialized cells and can divide through mitosis to produce more stem cells. They are found in all multicellular organisms. Stem cells are invaluable for drug development, personalized medicine and gene therapy. The major applications of stem cells are in regenerative medicine, drug screening and toxicity testing. On the basis of source, stem cells can be broadly classified into embryonic stem cells and adult stem cells. Embryonic stem cells are derived from the embryo inner cell mass. Adult stems cells are isolated from adult tissues and cells including bone marrow, adipose tissue, heart, gut, skin and retina. The Global Stem Cells Market is estimated to be valued at US$ 14.87 Mn in 2024 and is expected to exhibit a CAGR of 7.9% over the forecast period 2024 To 2031. Key Takeaways Key players operating in the Stem Cells are Abzena Ltd., Clarivate, Immunetrics Inc., GNS Healthcare, Dassault Systemes, Evotec, Novadiscovery, Insilico Medicine Inc., and InSilicoTrials Technologies, among others. The key players are engaged in expanding their product portfolios in stem cell research by developing innovative techniques for isolation and differentiation of stem cells. The demand for Stem Cells Market Demand is growing mainly due to increasing prevalence of chronic and lifestyle diseases and growing geriatric population globally. Stem cell therapy is considered as a potential treatment for various fatal diseases like cancer, myocardial infarction and diabetes. The increasing success of clinical trials is further driving the growth of the market. Technological advancements in stem cell manufacturing and 3D organoids are further enhancing the applications of stem cells in drug discovery and toxicity testing. Crispr/Cas9 gene editing, spheroid cell culturing and single cell sequencing are the latest technologies being used for manipulating stem cells. Market Trends Growing Focus on Induced Pluripotent Stem Cells: Induced pluripotent Stem Cell Market Size And Trends (iPSCs) have emerged as a major trend in stem cell research as they can be generated from adult tissues such as skin and blood cells. iPSCs have potential applications in disease modeling, drug development and personalized regenerative medicine. Increasing Adoption of 3D Organoid Technologies: 3D organoids are miniature 3D structures grown from stem cells which mimic in vivo tissue structures. Organoids technology is gaining significant popularity due to its potential to revolutionize drug development, toxicity testing and disease modeling. Organoids can replicate the complexity of human tissues better than 2D cell cultures. Market Opportunities Regenerative Medicine Applications: Stem cell therapy holds huge potential in the field of regenerative medicine in treatment of degenerative diseases. Areas such as cardiac disorders, bone disorders, diabetes, neurological disorders and skin injuries offer major opportunities. Drug Discovery and Toxicology Testing: Stem cells provide a predictive human disease model for drug discovery and toxicity assessment. Their ability to replicate human tissues makes them ideal for preclinical drug development and toxicology studies. This opens up major revenue opportunities. Impact of COVID-19 on the Stem Cells Market
The COVID-19 pandemic has significantly impacted the growth of the stem cells market. During the initial outbreak, many research activities and clinical trials involving stem cells were halted to divert resources towards COVID-19 treatment and management. This led to delays in new product development and launch plans of various market players. The demand for stem cell therapy also declined as non-essential procedures were postponed during lockdowns to prevent virus spread in healthcare facilities. However, post-COVID, focus on stem cell research has increased as scientists are exploring its potential in developing therapies against complications arising due to COVID-19 infection such as pulmonary fibrosis. Market players are investing more in R&D activities involving mesenchymal stem cells for treatment of acute respiratory distress syndrome caused by coronavirus. Overall, though COVID-19 stalled market growth in the short-term, focus on stem cell based solutions for COVID-19 related issues is expected to boost the stem cells industry over the coming years. q The North American region currently holds the largest share of the global stem cells market in terms of value. This can be attributed to presence of major market players and higher healthcare spending on emerging cell-based therapies. The United States is the most prominent country dominating the North American as well as global stem cell market. The Asia Pacific region is identified as the fastest growing market for stem cells globally. This growth can be accredited to improving healthcare infrastructure, rising medical tourism, and increasing investments by global market players to tap the opportunities in emerging Asian countries like China, India, and South Korea.
Get more insights on, Stem Cells Market
About Author: Money Singh is a seasoned content writer with over four years of experience in the market research sector. Her expertise spans various industries, including food and beverages, biotechnology, chemical and materials, defense and aerospace, consumer goods, etc. (https://www.linkedin.com/in/money-singh-590844163)
*Note:1. Source: Coherent Market Insights, Public Source, Desk Research 2. We have leveraged AI tools to mine information and compile it
#Stem Cell Market Size#Stem Cell Market trends#Stem Cell Market demand#Stem Cell Market growth#Stem Cell#Stem Cell Market#coherent market insights
1 note
·
View note
Text
Generative AI in Healthcare: Innovations and Challenges
Generative AI transforms the healthcare industry by offering innovative solutions to complex problems, from drug discovery to personalized treatment plans. This blog explores some of the most promising applications of Generative AI in healthcare, as well as the challenges and ethical considerations that come with these advancements.
Innovations in Healthcare with Generative AI
Drug Discovery: Drug discovery is one of the most groundbreaking applications of Generative AI. Traditional drug development is a lengthy and costly process, often taking over a decade and billions of dollars to bring a new drug to market. Generative AI models can analyze vast datasets of chemical compounds and predict new drug candidates more efficiently. For instance, companies like Insilico Medicine and Atomwise use AI to identify potential drug molecules, significantly reducing the time and cost involved in drug discovery.
Medical Imaging: Generative AI is also revolutionizing medical imaging. AI-powered tools can enhance the quality of medical images, making it easier for doctors to diagnose conditions accurately. Generative models like GANs (Generative Adversarial Networks) can reconstruct high-quality images from low-resolution scans, particularly useful in settings with limited access to advanced imaging technology. Additionally, AI can help identify patterns and anomalies in medical images that might be missed by the human eye, improving early detection rates for diseases such as cancer.
Personalized Treatment Plans: Personalized medicine is another area where Generative AI shows great promise. By analyzing a patient’s genetic makeup, lifestyle, and medical history, AI can generate customized treatment plans that are tailored to the individual’s unique needs. This approach improves the effectiveness of treatments and minimizes the risk of adverse reactions. For example, AI-driven platforms can recommend specific drug combinations for cancer patients based on their tumour’s genetic profile, enhancing the chances of successful treatment.
Virtual Health Assistants: Generative AI is powering virtual health assistants to provide patients with personalized health advice, schedule appointments, and monitor chronic conditions. These AI-driven assistants use natural language processing to understand and respond to patient queries, making healthcare more accessible and convenient. For instance, platforms like Ada Health and Babylon Health offer AI-based health assessment tools that guide patients through a series of questions to provide a preliminary diagnosis and recommendations for further action.
Challenges and Ethical Considerations
While the potential of Generative AI in healthcare is immense, it comes with its challenges and ethical concerns.
Data Privacy and Security: Healthcare data is susceptible, and using AI requires access to large datasets containing personal health information. Ensuring the privacy and security of this data is paramount. There is a risk of data breaches and misuse, which can have severe consequences for patients. Healthcare providers and AI developers must implement robust data protection measures and comply with HIPAA (Health Insurance Portability and Accountability Act) regulations to safeguard patient information.
Bias and Fairness: AI models are only as good as the data they are trained on. If the training data is biased, the AI’s predictions and recommendations will also be biased, potentially leading to disparities in healthcare outcomes. For example, if an AI system is trained predominantly on data from a particular demographic group, it may not perform as well for other groups. It is crucial to use diverse and representative datasets to train AI models and to monitor and address any biases that may arise continually.
Regulatory and Ethical Standards: Integrating AI into healthcare raises important regulatory and ethical questions. Clear guidelines and standards are needed to ensure the safe and ethical use of AI in healthcare. This includes validating the accuracy and reliability of AI tools, obtaining proper approvals from regulatory bodies, and maintaining transparency with patients about the use of AI in their care.
Integration with Existing Systems: Implementing AI solutions in healthcare often requires integrating them with existing electronic health record (EHR) systems and workflows. This can be challenging due to interoperability issues and the complexity of healthcare IT infrastructure. Successful integration requires collaboration between AI developers, healthcare providers, and IT professionals to ensure seamless and effective adoption.
Conclusion
Generative AI holds tremendous potential to revolutionize healthcare by improving drug discovery, enhancing medical imaging, personalizing treatment plans, and providing virtual health assistance. However, addressing the challenges and ethical considerations associated with its use is essential to ensure that these advancements benefit all patients equitably and safely. As the field continues to evolve, ongoing collaboration and innovation will be vital to unlocking the full potential of Generative AI in healthcare.
For more details about our services, please visit our website – Flentas Technologies
0 notes
Text
GenAI Disruptive Industries
Revolutionizing Content Creation: The Role of Generative AI in Media
Generative AI has revolutionized content creation by automating and enhancing various aspects of the creative process. This technology, powered by advanced algorithms like GPT-3, enables natural language generation that can produce human-like text for articles, stories, and marketing copy. Content creators benefit from AI's ability to generate diverse and engaging content at scale, freeing up time for ideation and strategy. Additionally, Generative AI has extended beyond text to multimedia, with tools like DALL-E capable of generating unique images based on textual prompts. This innovation is reshaping digital media, enabling personalized content experiences and improving efficiency in content production workflows. By leveraging Generative AI, businesses and creators can deliver more relevant and compelling content to their audiences, driving engagement and innovation in the content creation landscape.
Innovative Applications of Generative AI in Healthcare
Generative AI isn't just for art and media—it's also making significant strides in healthcare. GenAI is driving transformative advancements in healthcare by offering innovative applications across diagnostics, treatment, and personalized medicine. One notable area is medical imaging, where AI algorithms like Generative Adversarial Networks (GANs) generate synthetic images to aid in diagnosis and research. These tools enhance the accuracy and efficiency of medical imaging interpretation, leading to improved patient outcomes. Moreover, Generative AI facilitates drug discovery by simulating molecular structures and predicting potential drug candidates, accelerating the development of novel therapies. Insilico Medicine's platform (ReLEHF) utilizes Generative Adversarial Networks (GANs) and reinforcement learning to generate novel molecules with specific properties that could be potential drug candidates
Chatbots powered by AI provide virtual medical consultations, offering accessible and timely healthcare services to patients. Overall, Generative AI is revolutionizing healthcare by leveraging data-driven insights and automation to enhance medical decision-making and patient care.
Sustainable Design and Manufacturing with Generative AI
Generative AI is playing a pivotal role in advancing sustainable design and manufacturing practices by optimizing product development processes and reducing environmental impact. By harnessing algorithms like topology optimization, Generative AI can generate intricate and efficient designs that minimize material usage while maintaining structural integrity. This approach not only reduces waste in manufacturing but also promotes the adoption of eco-friendly materials and production methods. Additionally, AI-driven simulations can analyze and optimize supply chain logistics, further reducing carbon footprint and resource consumption. Companies utilizing Generative AI are able to create innovative, sustainable products that meet performance requirements while aligning with environmental objectives. This integration of technology with sustainability is reshaping the manufacturing landscape, fostering a more responsible approach to product design and production.
One notable example is Autodesk, which offers software solutions like Autodesk Generative AI Design software. This tool employs AI algorithms to explore numerous design possibilities based on specified constraints, helping engineers and designers create optimized and lightweight components for various industries, including automotive and aerospace. Another innovative company is Siemens, which uses AI-driven simulation software to optimize manufacturing processes, enhancing production efficiency and resource utilization.
Artistic Expression through Generative AI
Generative AI has revolutionized artistic expression by providing artists with powerful tools to explore new creative possibilities and expand the boundaries of traditional art forms. Algorithms like StyleGAN and DeepDream can generate visually stunning and surreal artworks, offering artists a way to collaborate with AI in the creative process. Artists can input specific parameters or styles into these models, allowing them to experiment with different artistic techniques and generate unique visual outputs. Generative AI also enables the creation of interactive and generative art installations, blurring the lines between art and technology. This technology has democratized art creation by making sophisticated tools accessible to artists worldwide, fostering innovation and pushing the evolution of contemporary art.
0 notes
Text
AI in Drug Discovery Market to See Huge Growth by 2029
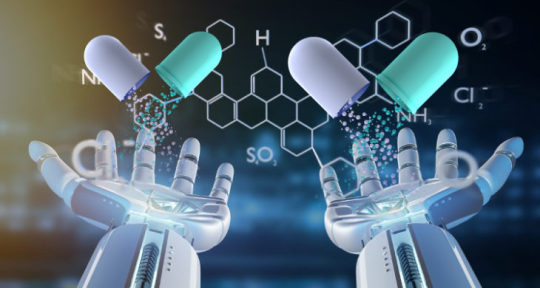
Global AI in Drug Discovery Market Report from AMA Research highlights deep analysis on market characteristics, sizing, estimates and growth by segmentation, regional breakdowns & country along with competitive landscape, player’s market shares, and strategies that are key in the market. The exploration provides a 360° view and insights, highlighting major outcomes of the industry. These insights help the business decision-makers to formulate better business plans and make informed decisions to improved profitability. In addition, the study helps venture or private players in understanding the companies in more detail to make better informed decisions. Major Players in This Report Include, IBM Corporation (United States), Microsoft (United States), Google (United States), NVIDIA Corporation (United States), Atomwise, Inc. (United States), Deep Genomics (Canada), Cloud Pharmaceuticals (United States), Insilico Medicine (United States), Benevolent AI (United Kingdom), Exscientia (United Kingdom). Free Sample Report + All Related Graphs & Charts @: https://www.advancemarketanalytics.com/sample-report/127272-global-ai-in-drug-discovery-market Artificial intelligence for drug discovery is a technology that uses and different algorithms that value add in decision-making processes for drug discovery. The increasing cases of rare diseases and demand for personalized drugs are the major factor fueling the growth of the global Al for Drug Discovery marker. Market Drivers
Increasing Pressure on the Drug Manufacturer to Reduce Drug Price
Growing Number of Cross-Industry Collaborations and Partnerships
Market Trend
Research Applying AI to Drug Discovery is Accelerating
Data Sharing
Opportunities
Implementation of AI reduces researches and development gap in the drug manufacturing process and help in the targeted manufacturing of the drugs.
Huge Investments in Research & Developments
Challenges
Protecting Intellectual Property and Data
Enquire for customization in Report @: https://www.advancemarketanalytics.com/enquiry-before-buy/127272-global-ai-in-drug-discovery-market In this research study, the prime factors that are impelling the growth of the Global AI in Drug Discovery market report have been studied thoroughly in a bid to estimate the overall value and the size of this market by the end of the forecast period. The impact of the driving forces, limitations, challenges, and opportunities has been examined extensively. The key trends that manage the interest of the customers have also been interpreted accurately for the benefit of the readers. The AI in Drug Discovery market study is being classified by Application (Immuno-oncology, Neurodegenerative Diseases, Cardiovascular Diseases, Metabolic Diseases, Others), Technology (Machine Learning, Deep Learning, Others), End-User (Pharmaceutical & Biotechnology, Research Organizations, Academic & Government Institutes), Component (Software, Services) The report concludes with in-depth details on the business operations and financial structure of leading vendors in the Global AI in Drug Discovery market report, Overview of Key trends in the past and present are in reports that are reported to be beneficial for companies looking for venture businesses in this market. Information about the various marketing channels and well-known distributors in this market was also provided here. This study serves as a rich guide for established players and new players in this market. Get Reasonable Discount on This Premium Report @ https://www.advancemarketanalytics.com/request-discount/127272-global-ai-in-drug-discovery-market Extracts from Table of Contents AI in Drug Discovery Market Research Report Chapter 1 AI in Drug Discovery Market Overview Chapter 2 Global Economic Impact on Industry Chapter 3 Global Market Competition by Manufacturers Chapter 4 Global Revenue (Value, Volume*) by Region Chapter 5 Global Supplies (Production), Consumption, Export, Import by Regions Chapter 6 Global Revenue (Value, Volume*), Price* Trend by Type Chapter 7 Global Market Analysis by Application ………………….continued This report also analyzes the regulatory framework of the Global Markets AI in Drug Discovery Market Report to inform stakeholders about the various norms, regulations, this can have an impact. It also collects in-depth information from the detailed primary and secondary research techniques analyzed using the most efficient analysis tools. Based on the statistics gained from this systematic study, market research provides estimates for market participants and readers. Contact US : Craig Francis (PR & Marketing Manager) AMA Research & Media LLP Unit No. 429, Parsonage Road Edison, NJ New Jersey USA – 08837 Phone: +1 201 565 3262, +44 161 818 8166 [email protected]
#Global AI in Drug Discovery Market#AI in Drug Discovery Market Demand#AI in Drug Discovery Market Trends#AI in Drug Discovery Market Analysis#AI in Drug Discovery Market Growth#AI in Drug Discovery Market Share#AI in Drug Discovery Market Forecast#AI in Drug Discovery Market Challenges
0 notes
Text
AI is Revolutionizing Drug Discovery: A Glimpse into the Future of Medicine
In the realm of medicine and healthcare, the quest for new drugs and therapies has always been a challenging and time-consuming endeavor. However, the emergence of artificial intelligence (AI) has brought about a revolutionary transformation in the field of drug discovery. In this blog, we will explore how AI is reshaping the drug discovery process, accelerating the development of life-saving medications, and offering new hope for patients around the world.
Read more: https://www.grgonline.com/post/from-data-to-miracle-cures-how-ai-is-revolutionizing-drug-discovery
The Traditional Drug Discovery Process
Traditionally, drug discovery has been a lengthy and costly process that involves multiple stages, from target identification and validation to lead compound identification, preclinical testing, and clinical trials. It often takes more than a decade and billions of dollars to bring a single drug to market, and many potential treatments never make it past the initial stages due to inefficiencies in the process.
AI-Powered Drug Discovery
Target Identification and Validation: AI algorithms can analyze vast amounts of biological data, including genomics, proteomics, and medical records, to identify potential drug targets. This enables researchers to pinpoint the root causes of diseases more quickly and accurately.
Drug Design and Optimization: AI-driven computational modeling and virtual screening can predict the interactions between drugs and their target proteins with remarkable precision. This allows for the rapid design and optimization of drug candidates, reducing the need for extensive laboratory testing.
High-Throughput Screening: AI-powered robots and automated systems can conduct high-throughput screening of thousands of compounds, identifying potential drug candidates much faster than traditional methods.
Clinical Trial Optimization: AI can analyze patient data to identify the most suitable candidates for clinical trials, making the recruitment process more efficient. Additionally, machine learning algorithms can predict patient responses to treatments, leading to more personalized and effective therapies.
Drug Repurposing: AI can scan existing databases of approved drugs and identify potential candidates for repurposing in the treatment of different diseases. This not only saves time but also reduces the risks associated with developing entirely new compounds.
Case Studies and Success Stories
Insilico Medicine: This AI-driven company used deep learning algorithms to discover a novel drug candidate for fibrosis in just 46 days, a process that typically takes several years.
BenevolentAI: Using AI and natural language processing, BenevolentAI identified a potential drug for amyotrophic lateral sclerosis (ALS), a neurodegenerative disease. This discovery is currently in clinical trials.
IBM Watson for Drug Discovery: IBM's AI platform, Watson, has been used to analyze vast volumes of biomedical literature and help researchers identify new drug targets.
Challenges and Ethical Considerations
While AI holds great promise in drug discovery, it also raises important challenges and ethical questions. Data privacy, bias in algorithms, and the need for regulatory oversight are just a few of the issues that need to be addressed as AI becomes more integrated into the pharmaceutical industry.
Conclusion
AI is ushering in a new era of drug discovery, offering unprecedented opportunities to accelerate the development of life-saving medications and treatments. As AI algorithms continue to improve and more data becomes available, we can expect even greater breakthroughs in the field of medicine. While challenges exist, the potential benefits of AI-driven drug discovery are immense, and they offer hope to millions of patients worldwide who are awaiting innovative therapies and cures for a wide range of diseases. The future of medicine is undoubtedly being shaped by the transformative power of artificial intelligence.
0 notes
Text
Key Industries & Applications in the Global Deep Learning Market
Over the years, deep learning has emerged as a transformative technology, revolutionizing the way various industries operate. Accordingly, the domain has become instrumental in the field of data analysis, predictive modeling, and process optimization. As per Inkwood Research, the global deep learning market is anticipated to grow with a CAGR of 39.67% during the forecast period 2023-2032.

In this blog, we will explore how major industries are leveraging the potential of deep learning in order to drive innovation and efficiency in their operations. We will also highlight real-world examples of successful deep learning applications in each of these industries.
1. Healthcare
Healthcare organizations of all specialties and types are becoming increasingly inclined toward how artificial intelligence (AI) can facilitate better patient care, while improving efficiencies and reducing costs. In this regard, the healthcare industry has been at the forefront of adopting deep learning for a wide range of applications, such as –
Medical Imaging: Deep learning algorithms have significantly improved the accuracy of medical image analysis. For instance, Aidoc (Israel), a leading provider of AI-powered radiology solutions, aims to improve the efficacy as well as accuracy of radiology diagnoses. The company’s platform utilizes deep learning algorithms in order to analyze medical images and aid radiologists in prioritizing and detecting critical findings.
Drug Discovery: Pharmaceutical companies are using deep learning to expedite drug discovery processes. Insilico Medicine (United States), for example, employs deep learning models to predict potential drug candidates, reducing the time and cost associated with developing new medications. The company’s early bet on deep learning is yielding significant results – a drug candidate discovered via its AI platform is now entering Phase II clinical trials for the treatment of idiopathic pulmonary fibrosis.
Disease Diagnosis: Deep learning is aiding in disease diagnosis through predictive modeling. PathAI (United States), for instance, utilizes deep learning-based AI solutions to assist pathologists in the detection, diagnosis, and prognosis of several cancer subtypes, thus improving the accuracy of diagnoses and patient outcomes.
2. Finance & Banking Services
The success of deep learning as a data processing technique has piqued the interest of the financial research community. Moreover, with the proliferation of Fintech over recent years, the use of deep learning in the finance & banking services industry has become highly prevalent across the following applications –
Fraud Detection: Banks and financial institutions deploy deep learning models to detect fraudulent activities in real time. Companies like Feedzai (Portugal) use deep learning algorithms to analyze transaction data and identify unusual patterns indicative of fraud. In July 2023, the company announced the launch of Railgun, a next-generation fraud detection engine, featuring advanced AI to secure millions from the surge in financial crime.
Algorithmic Trading: Hedge funds and trading firms leverage deep learning for algorithmic trading. Investors are utilizing deep learning models to evaluate and anticipate stock and foreign exchange markets, given the advantage of artificial intelligence.
Credit Scoring: Deep learning is transforming the credit scoring landscape. LenddoEFL (Singapore), for instance, creates, collects, and analyzes information from consent-based alternative data sources for an accurate understanding of creditworthiness. The company’s unique credit decisioning tools draw from large, diverse, and unstructured data sources through deep learning, artificial intelligence, and advanced modeling techniques.
3. Manufacturing
Here’s how the manufacturing industry benefits from deep learning in optimizing production processes and quality control –
Predictive Maintenance: Manufacturers use deep learning to predict equipment failures and schedule maintenance proactively. General Electric’s (United States) Predix platform employs deep learning to support innovative IoT solutions to help reduce downtime and maintenance costs.
Quality Control: Deep learning-based image recognition systems inspect products for defects on production lines. Real-time deep learning approaches are essential for automated industrial processes in product manufacturing, where vision-based systems effectively control the fabrication quality on the basis of specific structural designs.
Supply Chain Optimization: According to industry sources, deep learning models have the potential to generate between $1-2 trillion annually in supply chain management. In this regard, deep learning is used in the manufacturing sector to optimize supply chain operations by predicting demand patterns, enhancing inventory levels, and improving logistics planning.
Deep learning’s ability to process vast amounts of data and recognize complex patterns is transforming the way industries operate, making processes more efficient and enhancing customer experiences. As businesses strive to remain competitive in an increasingly data-driven world, leveraging deep learning and staying updated on its latest developments will be crucial for the overall growth of the global deep learning market.
FAQs:
What are the key components of a deep learning system?
A deep learning system typically comprises input data, a deep neural network architecture, loss functions, optimization algorithms, and labeled training data.
How does deep learning differ from traditional machine learning?
Deep learning, unlike traditional machine learning, eliminates the need for manual feature engineering by allowing models to learn and extract features automatically from data.
0 notes
Text
Global In Silico Clinical Trials Market Is Estimated To Witness High Growth Owing To Growing Demand For Virtual Clinical Trials

The global In Silico Clinical Trials Market is estimated to be valued at US$ 3,173.1 Mn in 2022 and is expected to exhibit a CAGR of 7.95% over the forecast period 2023-2030, as highlighted in a new report published by Coherent Market Insights. A) Market Overview: Silico Clinical Trials are virtual trials conducted using computer models and simulations to evaluate drug efficacy and safety. These trials provide several advantages over traditional clinical trials, such as reduced time and cost, ethical considerations, and the ability to collect large amounts of data. Virtual trials eliminate the need for physical participation, making it more convenient for patients and reducing the burden on healthcare systems. They also allow for the exploration of various drug combinations and dosages, accelerating the development of personalized medicine. B) Market Key Trends: One key trend in the In Silico Clinical Trials Market is the growing demand for virtual clinical trials. With advancements in technology and the increasing need for efficient drug development processes, pharmaceutical companies are increasingly adopting virtual trials. These trials provide real-time data analysis, reduce patient recruitment time, and offer cost-effective solutions for drug development. For example, Insilico Medicine Inc., a key player in the market, utilizes artificial intelligence (AI) algorithms to accelerate the drug discovery process. Their AI-based platform enables researchers to identify potential drug candidates and predict their efficacy using virtual models. C) PEST Analysis: Political: Regulatory frameworks play a crucial role in the adoption of virtual clinical trials. Governments need to establish guidelines and standards to ensure patient safety and data privacy. Economic: Silico Clinical Trials offer cost-effective solutions compared to traditional clinical trials. They reduce the need for physical locations, extensive patient recruitment efforts, and travel expenses. Social: Virtual trials provide opportunities for patients who may otherwise be unable to participate in traditional trials due to geographical constraints, physical limitations, or personal commitments. Technological: Advancements in technology, such as AI, machine learning, and big data analytics, have facilitated the growth of virtual trials. These technologies enable researchers to analyze complex data sets and make predictions about drug efficacy and safety. D) Key Takeaways: Paragraph 1: The global In Silico Clinical Trials Market is expected to witness high growth, exhibiting a CAGR of 7.95% over the forecast period. This growth can be attributed to increasing demand for virtual trials due to their ability to reduce time and cost in drug development. For example, the use of virtual models and simulations enables researchers to predict drug efficacy and safety before conducting physical trials. Paragraph 2: Regionally, North America is expected to dominate the In Silico Clinical Trials Market . The region has a well-established healthcare infrastructure, favorable regulatory environment, and a high adoption rate of advanced technologies. Additionally, collaborations between pharmaceutical companies, academic institutions, and research organizations in North America contribute to the region's growth in the market. In conclusion, the In Silico Clinical Trials Market is witnessing significant growth due to the increasing demand for virtual trials. Advancements in technology, cost-effectiveness, and the ability to collect real-time data are driving the adoption of virtual trials in drug development. The market is dominated by key players who are continuously investing in research and development to stay ahead in the competitive landscape. As virtual trials become more widely accepted, they have the potential to revolutionize the drug development process and improve patient outcomes.
#In Silico Clinical Trials Market#Medical Devices#In Silico Clinical Trials Market Growth#In Silico Clinical Trials Market Analysis#In Silico Clinical Trials Market Forecast#In Silico Clinical Trials Market Future#In Silico Clinical Trials Market Overview
0 notes
Text
AI in Healthcare Market to be 10X in the Next 7 Years: Access All Free Data
Global AI in Healthcare Market sales are expected to be $15.5 billion in 2023. This revenue will further grow at a CAGR of 38.4% from 2023 to reach $150.7 billion in 2030.
Healthcare and biopharmaceutical companies are currently initiating clinical trials that employ generative artificial intelligence (AI) to augment the process of drug discovery and development. In June 2023, Insilico Medicine, a biotechnology startup headquartered in Hong Kong and backed by over $400 million in funding, successfully developed a pharmaceutical product aimed at addressing idiopathic pulmonary fibrosis, a persistent respiratory condition. Exscientia, BenevolentAI, BPGbio, Deep Genomics, Recursion, and Moderna are among the prominent companies at the forefront of AI drug discovery.
The healthcare market has witnessed significant advancements in Generative AI, which are outlined below:
· In April 2023, Microsoft Corp. and Epic made an announcement regarding the expansion of their established strategic collaboration. The objective of this expansion is to incorporate generative AI into the healthcare sector by leveraging the capabilities of Azure OpenAI Service1 and integrating it with Epic's renowned electronic health record (EHR) software.
· Google is currently engaged in the testing phase of an artificial intelligence program that has been specifically trained to provide highly proficient responses to medical inquiries. This endeavor is in direct competition with other industry players, such as Microsoft, as they strive to transform recent advancements in AI technology into practical solutions that can be readily utilized by healthcare professionals.
Get Access to the Free Smart Book @ https://nforming.com/blog/healthcare/ai-in-healthcare-market-analysis-2023/
0 notes