#Hadoop Market size
Explore tagged Tumblr posts
Text
How to Start a Career in Big Data: A Comprehensive Guide

Big Data is reshaping industries worldwide, creating countless opportunities for individuals to build dynamic, data-driven careers. If you’re considering a career in Big Data, you’re not alone. The demand for data professionals is skyrocketing, and the need for individuals with analytical, technical, and managerial skills is higher than ever. This guide will walk you through the steps to kickstart your career in this exciting and rapidly evolving field.
What Is Big Data?
Big Data refers to the vast amounts of structured and unstructured data that organizations collect daily. This data is so massive that traditional data processing methods fall short, necessitating advanced tools and technologies. Big Data includes everything from customer interactions and social media activities to sensor data from IoT devices.https://internshipgate.com
Why Big Data Matters
In today's world, data is considered the new oil. Companies use Big Data analytics to gain insights, improve decision-making, and optimize performance. Whether it’s personalized marketing, predicting trends, or enhancing customer experiences, Big Data is at the heart of innovation.
Key Skills Required for a Career in Big Data
Starting a career in Big Data requires a unique set of skills that span both technical and analytical domains. Below are the essential competencies you’ll need:
1. Programming Skills
Knowing programming languages such as Python, R, or Java is crucial in Big Data roles. These languages help in data manipulation, automation, and building algorithms for data analysis.
2. Data Analysis and Statistics
A solid understanding of statistics and data analysis is fundamental for working with Big Data. You'll need to interpret large datasets and derive meaningful insights from them.
3. Knowledge of Big Data Tools
Familiarity with tools like Hadoop, Spark, and NoSQL databases (e.g., MongoDB, Cassandra) is essential for handling large-scale data efficiently. These tools allow you to store, process, and analyze massive datasets in real-time.
4. Data Visualization
Data visualization tools like Tableau or Power BI enable Big Data professionals to present complex data in a comprehensible and visually appealing manner.
5. Cloud Computing
With the shift towards cloud-based data storage, knowing how to work with cloud platforms such as AWS, Google Cloud, or Microsoft Azure is becoming increasingly important.
6. Soft Skills
Problem-solving, critical thinking, and communication skills are as vital as technical abilities. Big Data professionals need to collaborate with different departments and explain data findings to non-technical stakeholders.
Educational Pathways to Big Data Careers
There is no one-size-fits-all approach to breaking into Big Data. However, certain academic qualifications can give you an edge.
1. Bachelor's Degree in Computer Science, Data Science, or Related Fields
A solid foundation in computer science, statistics, or data science is essential. Universities offer a wide range of courses tailored to Big Data and data analysis.
2. Master's Degree or Specialized Certification
Pursuing a master’s degree in data science, business analytics, or a related field can significantly boost your job prospects. Additionally, online certifications such as Google's Professional Data Engineer or AWS Certified Big Data – Specialty are great options for career advancement.
Top Big Data Job Roles
Once equipped with the necessary skills and education, you can explore various job roles in the Big Data field:
1. Data Scientist
Data scientists analyze complex datasets to discover patterns and trends that can help businesses make informed decisions. This role requires a mix of technical and analytical skills.
2. Data Analyst
Data analysts focus on interpreting data, providing actionable insights, and making recommendations to drive business decisions.
3. Big Data Engineer
Big Data engineers design, develop, and manage scalable systems for storing, processing, and analyzing data.
4. Machine Learning Engineer
In this role, you’ll develop algorithms that allow computers to learn from and make predictions based on data, enabling businesses to automate processes and improve accuracy.
5. Business Intelligence Analyst
Business Intelligence (BI) analysts focus on converting data into actionable business insights. They use data visualization tools and techniques to present their findings.
Industries That Rely on Big Data
Big Data is transforming multiple industries. Below are some of the sectors where Big Data plays a significant role:
1. Healthcare
In healthcare, Big Data is used for predictive analytics, patient management, and optimizing treatment plans based on data from electronic health records.
2. Finance
Financial institutions leverage Big Data for fraud detection, risk management, and improving customer experiences through personalized services.
3. Retail
Retailers use Big Data for trend forecasting, inventory management, and personalizing marketing strategies to enhance customer satisfaction.
4. Telecommunications
Telecom companies rely on Big Data to optimize networks, predict customer churn, and improve service delivery.
How to Gain Practical Experience in Big Data
While education is essential, hands-on experience is equally crucial for success in Big Data.
1. Internships
Many companies offer internships for aspiring Big Data professionals. Internships provide real-world experience and help you apply what you’ve learned in a practical setting.
2. Projects
Working on data projects, either through academic programs or self-initiated efforts, can help you gain a deeper understanding of Big Data tools and methodologies.
3. Hackathons and Competitions
Participating in data science competitions like Kaggle can enhance your skills and expose you to real-world Big Data challenges.
4. Open Source Contributions
Contributing to open-source Big Data projects is another way to gain practical experience and network with professionals in the field.
Building a Portfolio
A strong portfolio is crucial when applying for Big Data roles. Here's how to build one:
1. Showcase Projects
Highlight your most significant projects, including any data analytics or visualization work you’ve done.
2. Document Tools and Technologies Used
Clearly specify the Big Data tools and technologies you’ve used in each project. This demonstrates your technical proficiency.
3. Include Certifications
List any certifications or courses you’ve completed. Employers value candidates who continuously improve their skills.
Networking in the Big Data Field
Networking is key to advancing in any career, including Big Data. Here are some ways to build professional connections:
1. Attend Conferences and Meetups
Big Data conferences and local meetups are great places to meet industry professionals and learn about the latest trends.
2. Join Online Communities
Platforms like LinkedIn, Reddit, and specialized Big Data forums offer a space to connect with others in the field.
3. Participate in Webinars
Many organizations offer webinars on Big Data topics. These events provide valuable knowledge and networking opportunities.
Common Challenges in Starting a Big Data Career
Starting a career in Big Data can be daunting. Here are some common challenges and how to overcome them:
1. Rapidly Changing Technologies
The field of Big Data is constantly evolving, making it essential to stay up-to-date with new tools and technologies.
2. Data Privacy and Security
As a Big Data professional, you’ll need to navigate complex issues around data privacy and security.
3. Finding the Right Job Role
Given the wide range of Big Data roles, finding the one that fits your skills and interests can be challenging. Take time to explore different areas of specialization.
FAQs: How to Start a Career in Big Data
1. Do I need a degree to start a career in Big Data?
While a degree is helpful, many professionals enter the field through certifications and practical experience.
2. What programming languages should I learn for Big Data?
Python, R, and Java are widely used in Big Data for data analysis, automation, and building algorithms.
3. Can I transition into Big Data from a non-technical background?
Yes, many people transition from business, finance, and other non-technical fields by acquiring relevant skills and certifications.
4. What is the salary range for Big Data professionals?
Salaries vary by role and location, but Big Data professionals can expect competitive pay, often exceeding six figures.
5. How do I gain practical experience in Big Data?
Internships, data projects, hackathons, and open-source contributions are excellent ways to gain hands-on experience.
6. What industries hire Big Data professionals?
Big Data professionals are in demand across industries such as healthcare, finance, retail, telecommunications, and more.
Conclusion
Starting a career in Big Data can be highly rewarding, offering a dynamic work environment and countless opportunities for growth. By building a solid foundation in programming, data analysis, and Big Data tools, you’ll be well on your way to success in this exciting field. Don’t forget to continuously learn, stay up-to-date with industry trends, and expand your network.https://internshipgate.com
#career#internship#internshipgate#virtualinternship#internship in india#job opportunities#big data#analytics#education
1 note
·
View note
Text
Navigating the Path to Becoming an AI Engineer: Essential Skills, Career Outlook, and Opportunities

What Does an AI Engineer Do?
An AI engineer is responsible for creating systems and models that enable machines to perform tasks that typically require human intelligence, such as decision-making, natural language processing, and visual perception. This role involves working with vast amounts of data, designing complex algorithms, and applying machine learning techniques to optimize model performance.
A few key responsibilities include:
Developing machine learning models: Creating algorithms that can learn from and adapt to new data without explicit programming.
Data preprocessing: Cleaning and transforming data to ensure it’s ready for analysis.
Model deployment and monitoring: Ensuring models perform well in real-world settings and refining them as needed.
Essential Skills for AI Engineers
To excel as an AI engineer, one needs a diverse set of skills. Here are some of the most critical ones:
Programming Proficiency AI engineers must have strong programming skills, particularly in languages like Python, R, and Java. Knowledge of libraries such as Keras, TensorFlow, and PyTorch is also essential for implementing machine learning algorithms.
Mathematics and Statistics A deep understanding of linear algebra, calculus, and probability theory helps AI engineers create more effective algorithms. These skills are fundamental for understanding model behavior and performance.
Data Management Working with large data sets requires expertise in data handling, storage, and processing. Familiarity with big data technologies like Hadoop and Spark is beneficial, as is proficiency in SQL for data extraction and manipulation.
Machine Learning and Deep Learning AI engineers should be proficient in machine learning techniques, such as supervised and unsupervised learning, and deep learning frameworks like TensorFlow. This includes understanding how neural networks function and training them for specific applications.
Problem-Solving and Creativity AI solutions often require out-of-the-box thinking. Strong problem-solving abilities allow AI engineers to devise unique approaches to technical challenges and improve model efficiency.
Career Outlook and Opportunities
The demand for AI engineers continues to grow as businesses across sectors look to integrate AI into their processes. The global AI market size is projected to reach new heights in the coming years, meaning job opportunities for AI engineers will likely remain plentiful. Salaries for AI engineers are competitive, often surpassing those of other IT roles due to the specialized knowledge required.
Some popular industries hiring AI engineers include:
Healthcare: AI is used in diagnostics, personalized medicine, and patient monitoring.
Finance: From fraud detection to algorithmic trading, AI transforms financial services.
Manufacturing: Predictive maintenance and quality control are just a few ways AI is enhancing efficiency.
Retail: Personalization and recommendation engines help retailers improve customer engagement.
Breaking Into the AI Engineering Field
Starting a career in AI engineering often requires a solid educational background in computer science, mathematics, or a related field. However, many aspiring AI engineers also transition from other tech roles by upskilling through online courses and certifications. Platforms like Coursera, Udacity, and edX offer AI and machine learning courses that provide hands-on experience.
Final Thoughts
Becoming an AI engineer is a rewarding journey for those passionate about data, algorithms, and innovation. As AI continues to shape the future, the role of AI engineers will only become more integral to technological advancement. Whether you’re considering entering this field or seeking to hire an AI specialist, understanding these core skills and industry insights can help you make informed decisions in the dynamic AI landscape.
0 notes
Text
Hadoop Market Outlook: Global Trends and Forecast Analysis (2023-2032)
The global demand for hadoop was valued at USD 36518.5 Million in 2022 and is expected to reach USD 485934 Million in 2030, growing at a CAGR of 38.2% between 2023 and 2030.
The Hadoop market has witnessed significant growth, driven by the increasing need for big data analytics across various industries. As organizations accumulate vast amounts of unstructured data, Hadoop has emerged as a powerful, cost-effective solution for managing and analyzing large data sets. Key industries, including retail, banking, healthcare, and telecommunications, are leveraging Hadoop to gain insights, improve decision-making, and enhance customer experiences. The open-source nature of Hadoop, combined with its scalability and flexibility, has made it an attractive option for businesses of all sizes. However, challenges such as complex implementation, a shortage of skilled professionals, and security concerns persist. The market is expected to grow as companies continue to adopt data-driven strategies and invest in advanced analytics. With ongoing developments in cloud computing and integration with other data-processing platforms, the Hadoop market is poised for continued expansion, driven by the increasing demand for real-time analytics and business intelligence solutions.
The key drivers fueling growth in the Hadoop market include:
Explosion of Big Data: The rapid increase in the volume of structured and unstructured data from various sources, such as social media, IoT devices, and enterprise applications, has created a demand for powerful data processing and storage solutions like Hadoop.
Cost-Effective Data Storage: Hadoop provides a highly cost-efficient way to store and manage large datasets, making it an attractive option for organizations looking to reduce data storage costs.
Scalability and Flexibility: Hadoop's distributed computing model allows businesses to scale their data storage and processing capabilities easily, adapting to growing data needs over time.
Increasing Demand for Data Analytics: Companies are increasingly leveraging data analytics to gain insights, improve decision-making, and enhance customer experiences, driving adoption of platforms like Hadoop for big data analysis.
Cloud Integration: With the rise of cloud computing, Hadoop has become more accessible and easier to deploy on cloud platforms, enabling businesses to process big data without significant infrastructure investment.
Real-Time Analytics: The need for real-time insights and business intelligence is growing, and Hadoop, in combination with other tools, enables organizations to perform near real-time data analysis.
IoT and Machine Learning Applications: The proliferation of IoT and the growing adoption of machine learning have increased demand for data processing platforms like Hadoop, which can handle and analyze vast amounts of sensor and machine-generated data.
Open-Source Nature: As an open-source platform, Hadoop provides flexibility and a lower total cost of ownership, allowing organizations to modify and optimize the software based on specific business requirements.
Access Complete Report - https://www.credenceresearch.com/report/hadoop-market
Key Players
Amazon Web Services
Cisco Systems Inc
Cloudera Inc
Datameer Inc
Hitachi Data Systems
Fair Isaac Corporation
MapR Technologies
MarkLogic
Microsoft Corporation
Teradata Corporation
The Hadoop market demonstrates varying growth patterns and adoption levels across different regions:
North America: This region holds the largest share of the Hadoop market, driven by early adoption of big data technologies and strong demand from sectors such as retail, finance, and healthcare. The presence of major technology companies and advanced IT infrastructure facilitates the deployment of Hadoop. Additionally, growing demand for advanced analytics and business intelligence tools is pushing organizations to invest in big data solutions like Hadoop.
Europe: Europe is a significant market for Hadoop, particularly in industries such as banking, manufacturing, and telecommunications. Increasing regulatory requirements, such as GDPR, are compelling organizations to implement robust data management and analytics systems. Countries like the UK, Germany, and France are at the forefront, with businesses focusing on data-driven strategies to enhance competitiveness and customer experiences.
Asia-Pacific: The Asia-Pacific region is witnessing rapid growth in Hadoop adoption, fueled by expanding IT infrastructure, increasing digital transformation efforts, and a growing e-commerce sector. Countries like China, India, and Japan are driving the demand due to rising awareness of big data analytics and increased investments in cloud-based Hadoop solutions. Additionally, the proliferation of IoT and smart city projects in the region is generating massive data volumes, further driving the need for Hadoop solutions.
Latin America: In Latin America, the Hadoop market is growing steadily, with adoption primarily concentrated in Brazil, Mexico, and Argentina. The rise of digital initiatives, along with increased investment in big data and cloud technologies, is supporting market expansion. However, limited technical expertise and infrastructural challenges still pose obstacles to widespread adoption in this region.
Middle East and Africa: The Hadoop market in the Middle East and Africa is emerging, as governments and enterprises invest in digital transformation and big data technologies. Key industries driving adoption include telecommunications, banking, and public sector organizations. While there is significant potential for growth, challenges such as limited infrastructure and the need for skilled professionals remain barriers. Nonetheless, increasing investments in smart city projects and IoT are expected to drive Hadoop adoption in this region over the coming years.
Overall, North America and Asia-Pacific lead the Hadoop market, while Europe follows closely behind. Emerging regions such as Latin America and the Middle East & Africa hold promising growth potential as digital transformation and data-centric strategies become more widespread.
Segmentation
By Hadoop Components
Hadoop Distributed File System (HDFS)
MapReduce
Hadoop Common
Hadoop YARN (Yet Another Resource Negotiator)
Hadoop Ecosystem Projects
By Deployment Types
On-Premises Hadoop
Cloud-Based Hadoop
Hybrid Deployments
By Applications and Use Cases
Data Warehousing
Log and Event Data Analysis
Data Lakes
Machine Learning and AI
IoT Data Analysis
Genomic Data Analysis
Financial Data Analysis
By Industry Verticals
Financial Services
Healthcare
Retail and E-commerce
Telecommunications
Government and Public Sector
Energy and Utilities
By Hadoop Service Providers
Hadoop Distribution Providers
Cloud Service Providers
Consulting and Support Services
By Company Size
Small and Medium-Sized Enterprises (SMEs)
Large Enterprises
By Security and Compliance
Hadoop Security Solutions
Regulatory Compliance
Browse the full report – https://www.credenceresearch.com/report/hadoop-market
Contact Us:
Phone: +91 6232 49 3207
Email: [email protected]
Website: https://www.credenceresearch.com
0 notes
Text
Data Wrangling For Success: Streamlining Processes In Small And Large Enterprises
In the age of big data, organizations are continuously seeking ways to streamline their processes and seek knowledgeable insights from the vast amounts of data they collect. The need for efficiency and precision has never been higher, making data-wrangling tools an essential resource for businesses of all sizes. Data wrangling, or the process of cleaning and transforming raw data into a structured format, is the key to unlocking the potential of your data for business intelligence and analytics.
The Role of Data Wrangling in Today's Enterprises
Data wrangling plays a crucial role in helping businesses prepare their data for analysis. It involves gathering, filtering, and reformatting data from various sources so it can be effectively used by business intelligence (BI) tools. With the right data-wrangling software, enterprises can accelerate decision-making processes by providing clean and actionable data. Whether you are a small startup or a large multinational corporation, having organized data is the utmost step to gaining a competitive edge in your market.
Streamlining Small Enterprise Operations through Data Wrangling
Small businesses, with their limited resources, can benefit immensely from data-wrangling techniques. By using data wrangling tools, they can filter and aggregate data in a way that simplifies their operations. Instead of relying on manual processes, small enterprises can automate data preparation, ensuring that only the most relevant data is used for decision-making. This streamlined approach not only saves time but also helps small businesses stay agile in a competitive market, allowing them to respond to changes and opportunities more quickly.
How Large Enterprises Benefit from Advanced-Data Wrangling Techniques
For large enterprises, the volume of data collected can be overwhelming. Advanced data wrangling techniques allow large companies to manage big data more effectively. Using a platform like IRI Voracity, large enterprises can filter, scrub, and aggregate their data in a single job, reducing the time and effort required to prepare data for BI tools. This approach helps companies build data subsets that are easier to analyze, leading to faster insights and more informed business decisions.
Choosing the Right Provider for Data Wrangling
When selecting a provider for data wrangling, it's essential to choose one that offers robust and scalable solutions. Innovative Routines International (IRI), Inc. is a top provider in this space, known for its powerful data preparation tools like Voracity and CoSort. IRI's Voracity platform allows businesses to rapidly prepare big data for BI and analytics by filtering, transforming, and aggregating data in a single process. Built on the Eclipse framework and powered by CoSort or Hadoop engines, Voracity ensures that businesses can prepare data efficiently for multiple targets, whether it's for dashboards, scorecards, or scatter plots.
With the right provider, businesses can simplify the complexities of data wrangling and unlock the full potential of their data.
Conclusion
In today's data-driven world, businesses must embrace data wrangling to stay competitive. Whether you are a small enterprise looking to streamline operations or a large corporation managing vast amounts of data, the right data-wrangling tool can transform how you handle data. By choosing trusted providers like IRI, businesses can ensure their data is ready for insightful analysis, leading to better decision-making and overall success.
0 notes
Text
What is difference between Data Science and Big Data?
While these terms are loosely used interchangeably, they refer to very distinct concepts in the sphere of data analysis.
Big Data
Focus: The core aspect of big data is related to handling, storing, and processing huge data volumes.
Characteristics: Characterized by the 3Vs, namely, Volume, Velocity, and Variety.
Tools: Hadoop, Spark, NoSQL databases.
Goal: The goal is to deal with technical issues that have been created by large volumes of data processing and storage.
Data Science
Focus: A superset of tasks involving extracting insights and knowledge from data, ignoring the size.
Techniques: It has various statistical, mathematical, and machine learning techniques at its core.
Tools: Python, R, SQL, TensorFlow, PyTorch Goal: Extract meaningful information, patterns, and predictions from data to inform decision-making.
In essence, Big Data deals with how to deal with large datasets. Data Science deals with what you can do with that data.
Relationship: Though Big Data provides the base for data science, it is the latter which applies techniques to uncover useful insights from these vast datasets.
Example: A company might use Big Data to hold and process terabytes of transaction data for its customers. The data scientist analyzes this information for trends, prediction of future behavior, and personalization of their marketing efforts.
Summary
Big Data is basically a subset of the former, that is, Data Science. Big Data goes towards the technical aspects dealing with large data sets, while Data Science is a wider discipline of knowledge discovery or extraction from data.
0 notes
Text
Sharing Economy Market Scope & Growth Projection till 2032
Sharing Economy Market provides in-depth analysis of the market state of Sharing Economy manufacturers, including best facts and figures, overview, definition, SWOT analysis, expert opinions, and the most current global developments. The research also calculates market size, price, revenue, cost structure, gross margin, sales, and market share, as well as forecasts and growth rates. The report assists in determining the revenue earned by the selling of this report and technology across different application areas.
Geographically, this report is segmented into several key regions, with sales, revenue, market share and growth Rate of Sharing Economy in these regions till the forecast period
North America
Middle East and Africa
Asia-Pacific
South America
Europe
Key Attentions of Sharing Economy Market Report:
The report offers a comprehensive and broad perspective on the global Sharing Economy Market.
The market statistics represented in different Sharing Economy segments offers complete industry picture.
Market growth drivers, challenges affecting the development of Sharing Economy are analyzed in detail.
The report will help in the analysis of major competitive market scenario, market dynamics of Sharing Economy.
Major stakeholders, key companies Sharing Economy, investment feasibility and new market entrants study is offered.
Development scope of Sharing Economy in each market segment is covered in this report. The macro and micro-economic factors affecting the Sharing Economy Market
Advancement is elaborated in this report. The upstream and downstream components of Sharing Economy and a comprehensive value chain are explained.
Browse More Details On This Report at @https://www.globalgrowthinsights.com/market-reports/sharing-economy-market-100581
Global Growth Insights
Web: https://www.globalgrowthinsights.com
Our Other Reports:
Bonded Abrasive MarketMarket Forecast
Global Gas Dynamic Cold Spray Equipment MarketMarket Size
Healthcare Workforce Management System MarketMarket Growth
Seamless Underwear MarketMarket Analysis
Macadamia MarketMarket Size
Global Heat Pump Water Heater MarketMarket Share
Global Pharmacy Automation Equipment MarketMarket Growth
Biological Chip MarketMarket
BDP Flame Retardants MarketMarket Share
Computer Mice MarketMarket Growth Rate
Webtoons MarketMarket Forecast
Global Industrial Robot MarketMarket Size
Healthcare Nanotechnology MarketMarket Growth
Master Data Management MarketMarket Analysis
Energy Management V2H (Vehicle-To-Home) Power Supply Systems MarketMarket Size
Global Contract Lifecycle Management System MarketMarket Share
Global Hadoop MarketMarket Growth
Cannabis and Hemp MarketMarket
Hard Seltzer MarketMarket Share
Internet of Robotic Things MarketMarket Growth Rate
Residential Energy Storage Systems MarketMarket Forecast
Global GPS Watch Tracker MarketMarket Size
Drone Identification Systems MarketMarket Growth
Fire Retardant OSB (Oriented Strand Board) MarketMarket Analysis
Exothermic Welding MarketMarket Size
Global Cables MarketMarket Share
Global Safety Prefilled Syringes MarketMarket Growth
Baby Cosmetics MarketMarket
Medical Audiometers MarketMarket Share
Online Proctoring Services for Higher Education MarketMarket Growth Rate
Cyber Security Software MarketMarket Forecast
Global Consulting Services MarketMarket Size
Enterprise Information Management Solution MarketMarket Growth
Optical Fiber Patch Cord MarketMarket Analysis
Employers Liability Insurance Market Market Size
Global Liquid Metal Detectors MarketMarket Share
Global Cyclic Corrosion Test Cabinets MarketMarket Growth
Integrated Circuit Optical Couplers Market Market
Self-Climbing System MarketMarket Share
Nano Silver Mouthwash Market Market Growth Rate
0 notes
Text
The global demand forhadoop market was valued at USD 36518.5 Million in 2022 and is expected to reach USD 485934 Million in 2030, growing at a CAGR of 38.2% between 2023 and 2030. The explosion of big data has revolutionized industries across the globe, driving the need for robust data management solutions. One technology that has significantly shaped this landscape is Hadoop. Since its inception, Hadoop has become synonymous with big data analytics, offering scalable, cost-effective, and flexible solutions for managing vast amounts of data. This article explores the growth, key drivers, and future prospects of the Hadoop market.Hadoop, an open-source framework developed by the Apache Software Foundation, emerged from the need to handle large datasets that traditional databases struggled to manage. Inspired by Google's MapReduce and Google File System papers, Hadoop was designed to process and store massive data efficiently across distributed systems. It comprises two main components: the Hadoop Distributed File System (HDFS) for data storage and MapReduce for data processing.
Browse the full report at https://www.credenceresearch.com/report/hadoop-market
Market Growth
The Hadoop market has witnessed exponential growth over the past decade. According to various industry reports, the global Hadoop market was valued at approximately USD 35 billion in 2020 and is projected to reach USD 87 billion by 2025, growing at a compound annual growth rate (CAGR) of around 20%. This remarkable growth is driven by several factors, including the increasing volume of structured and unstructured data, advancements in technology, and the rising adoption of cloud-based solutions.
Key Drivers
1. Data Explosion: The rapid proliferation of data from various sources such as social media, IoT devices, and enterprise applications has created an urgent need for effective data management solutions. Hadoop's ability to handle petabytes of data cost-effectively makes it a preferred choice for organizations.
2. Scalability and Flexibility: Hadoop’s architecture allows for horizontal scaling, meaning that organizations can easily add more nodes to handle increasing data volumes without significant changes to the existing infrastructure. Its flexibility to process both structured and unstructured data is also a significant advantage.
3. Cost Efficiency: Traditional data warehouses and databases can be expensive to scale. Hadoop, being open-source, offers a cost-effective alternative, reducing the total cost of ownership. Its ability to run on commodity hardware further lowers expenses.
4. Cloud Integration: The integration of Hadoop with cloud platforms has been a game-changer. Cloud providers like AWS, Microsoft Azure, and Google Cloud offer Hadoop as a service, making it more accessible to businesses of all sizes. This has simplified the deployment and management of Hadoop clusters, driving its adoption.
Challenges
Despite its advantages, Hadoop faces several challenges that could impact its market growth. These include:
1. Complexity: Implementing and managing Hadoop clusters can be complex, requiring specialized skills and expertise. This complexity can be a barrier for smaller organizations.
2. Security Concerns: As with any data management system, security is a critical concern. Ensuring data privacy and protection in Hadoop environments requires robust security measures, which can be challenging to implement.
3. Competition: The big data analytics market is highly competitive, with numerous alternatives to Hadoop emerging. Technologies like Apache Spark, which offers faster processing for certain workloads, and various commercial big data platforms present significant competition.
Future Prospects
The future of the Hadoop market looks promising, driven by continuous advancements and evolving business needs. Several trends are likely to shape its trajectory:
1. AI and Machine Learning: The integration of Hadoop with AI and machine learning frameworks is expected to open new avenues for advanced analytics. This will enhance its capabilities in predictive analytics, real-time processing, and data-driven decision-making.
2. Edge Computing: As edge computing gains traction, Hadoop is likely to play a pivotal role in managing and processing data at the edge. This will be particularly relevant for industries like IoT, where real-time data processing is crucial.
3. Enhanced Security: Ongoing developments in cybersecurity are expected to address the security concerns associated with Hadoop, making it a more secure choice for enterprises.
4. Hybrid Deployments: The trend towards hybrid cloud deployments is expected to benefit Hadoop, as organizations seek to leverage the best of both on-premises and cloud environments.
Key Players
Amazon Web Services
Cisco Systems Inc
Cloudera Inc
Datameer Inc
Hitachi Data Systems
Fair Isaac Corporation
MapR Technologies
MarkLogic
Microsoft Corporation
Teradata Corporation
Others
Segmentation
By Hadoop Components
Hadoop Distributed File System (HDFS)
MapReduce
Hadoop Common
Hadoop YARN (Yet Another Resource Negotiator)
Hadoop Ecosystem Projects
By Deployment Types
On-Premises Hadoop
Cloud-Based Hadoop
Hybrid Deployments
By Applications and Use Cases
Data Warehousing
Log and Event Data Analysis
Data Lakes
Machine Learning and AI
IoT Data Analysis
Genomic Data Analysis
Financial Data Analysis
By Industry Verticals
Financial Services
Healthcare
Retail and E-commerce
Telecommunications
Government and Public Sector
Energy and Utilities
By Hadoop Service Providers
Hadoop Distribution Providers
Cloud Service Providers
Consulting and Support Services
By Company Size
Small and Medium-Sized Enterprises (SMEs)
Large Enterprises
By Security and Compliance
Hadoop Security Solutions
Regulatory Compliance
By Region
North America
The U.S.
Canada
Mexico
Europe
Germany
France
The U.K.
Italy
Spain
Rest of Europe
Asia Pacific
China
Japan
India
South Korea
South-east Asia
Rest of Asia Pacific
Latin America
Brazil
Argentina
Rest of Latin America
Middle East & Africa
GCC Countries
South Africa
Rest of the Middle East and Africa
Browse the full report at https://www.credenceresearch.com/report/hadoop-market
About Us:
Credence Research is committed to employee well-being and productivity. Following the COVID-19 pandemic, we have implemented a permanent work-from-home policy for all employees.
Contact:
Credence Research
Please contact us at +91 6232 49 3207
Email: [email protected]
Website: www.credenceresearch.com
0 notes
Text
0 notes
Text
The Role of Open Source Data Analytics Software in Empowering Organizations
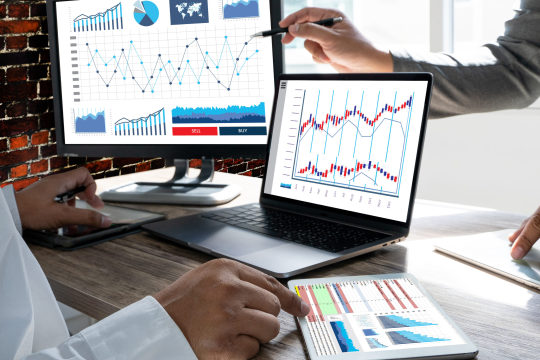
In the data-driven landscape of the modern business world, the ability to analyze vast quantities of information has become indispensable. Data analytics software stands at the forefront of this revolution, offering tools that transform raw data into actionable insights. Among these, open source data analytics software has emerged as a powerful force, democratizing access to advanced analytics capabilities and empowering organizations of all sizes. The adoption of open source solutions such as R, Python, and Apache Hadoop is reshaping the way businesses approach data analysis, offering a blend of accessibility, flexibility, and community-supported innovation.
Democratizing Data Analytics
One of the most significant contributions of open source data analytics software is its role in democratizing data analytics. Traditional proprietary analytics tools often come with high licensing fees, putting them out of reach for small to medium-sized enterprises (SMEs) and startups. Open source software, on the other hand, is freely available, enabling organizations with limited budgets to leverage sophisticated analytics capabilities. This accessibility fosters a more level playing field where SMEs can compete with larger corporations, driving innovation and efficiency across industries.
Flexibility and Customization
Open source data analytics software offers unparalleled flexibility, allowing organizations to tailor tools to their specific needs. Unlike closed-source platforms, which may limit customization or require expensive add-ons for additional functionality, open source solutions enable users to modify and extend the software. This adaptability is crucial for businesses operating in niche markets or with unique data processing requirements. The ability to customize analytics tools not only enhances operational efficiency but also allows for the development of bespoke solutions that can provide a competitive edge.
Fostering Innovation through Community Collaboration
The open source model thrives on community collaboration, drawing on the collective expertise of developers, data scientists, and industry experts worldwide. This collaborative environment accelerates the pace of innovation, with continuous improvements, new features, and bug fixes being contributed regularly. Users benefit from a rapidly evolving ecosystem of tools that are often at the cutting edge of data analytics technology. Moreover, the open exchange of ideas and solutions within the community fosters learning and skill development, enabling users to stay abreast of the latest trends and techniques in data science.
Challenges and Considerations
Despite its many advantages, the adoption of open source data analytics software is not without challenges. Organizations may face issues related to software support, as community-based assistance varies in responsiveness and expertise. Ensuring data security and compliance with industry regulations when using open source tools can also be a concern, necessitating additional measures to protect sensitive information. Furthermore, successfully integrating open source analytics into existing IT infrastructures requires a certain level of technical proficiency, highlighting the importance of investing in training and development for staff.
The Future of Open Source in Data Analytics
Looking forward, the role of open source software in data analytics is poised to grow even more significant. As businesses increasingly recognize the value of data-driven decision-making, the demand for accessible, flexible analytics tools will continue to rise. The open source community's commitment to innovation and collaboration suggests that these platforms will remain at the forefront of technological advancements, offering powerful solutions for predictive analytics, machine learning, and beyond.
Moreover, the trend towards hybrid models that combine open source flexibility with the support and security features of proprietary software indicates a promising direction for the future of data analytics. Such models can provide the best of both worlds, enabling organizations to leverage the strengths of open source while mitigating its limitations.
Conclusion
Open source data analytics software has fundamentally transformed the landscape of business intelligence, offering tools that empower organizations to harness the power of data. By providing free access to sophisticated analytics capabilities, fostering a culture of collaboration and innovation, and offering unparalleled flexibility, open source solutions are enabling businesses to drive efficiency, innovation, and competitive advantage. As the data analytics field continues to evolve, open source software will undoubtedly play a crucial role in shaping its future, democratizing access to information and insights that fuel organizational success.
0 notes
Text
0 notes
Text
Data Lake Market Manufacturers, Research Methodology, Competitive Landscape and Business Opportunities by 2032
The global data lake market size is expected to reach USD 89.53 Billion in 2032 and register a steady revenue CAGR of 20.6% during the forecast period, according to latest analysis by Emergen Research. Increasing need to extract in-depth insights from rising volumes of data to gain a competitive advantage is a key factor driving market revenue growth. Data lake gathers data from several sources, which is kept in the original format and can be instantly imported. Size of data can be increased using this technique while saving time on the creation of data structures, schema, and transformations. Data lakes allow many roles within an organization, such as data scientists, data developers, and business analysts, to access data using their chosen analytic tools and frameworks. A few examples include commercial solutions from data warehousing and business intelligence providers as well as open source frameworks such as Apache Hadoop, Presto, and Apache Spark. These providers can run analytics using data lakes instead of migrating their data to a different analytics solution.
0 notes
Text
0 notes
Text
Discovering R7i Instances for Big Data, AI, and Memory
Big Data and R7i instances
The Amazon Elastic Compute Cloud (Amazon EC2) R7i instance is now generally available, thanks to an announcement from Intel and Amazon Web Services (AWS). With Xeon you can trust and AWS’s vast global footprint, this compute-optimized EC2 custom instance is powered by 4th Gen Intel Xeon processors and Intel Accelerator Engines.
Over the course of the projection period, the in-memory database market alone is anticipated to grow at a CAGR of 19%. At this critical juncture, machine learning and artificial intelligence (AI) are growing rapidly, requiring more processing power to analyze vast volumes of data faster. As enterprises continue to migrate more and more to the cloud, security is becoming increasingly important. Furthermore, data lakes are a sort of architecture that is becoming more and more popular and are completely changing how businesses store and use data.
Global enterprises’ adoption of memory-intensive workloads and big data trends call for the strength of specialized cloud computing instances like R7i, which may provide superior, automated decision-making that aids in the successful achievement of business decision-making goals.
An Excellent Option for Tasks Requiring a Lot of Memory and SAP Certified
All memory-intensive workloads, including SAP, SQL, and NoSQL databases, distributed web scale in-memory caches, in-memory databases like SAP HANA, and real-time big data analytics like Hadoop and Spark, are well suited for these SAP-certified instances. An early analysis for SAP indicates very good ~30% Better SAPS/$ over R6i that businesses can use right now.
Utilize Built-in Accelerators to Boost AI and Big Data
Four inbuilt accelerators are present in the R7i instances, and they each offer the following acceleration features:
The Intel AMX extensions, also known as the Intel Advanced Matrix Extensions, are intended to speed up workloads involving matrix operations and machine learning. By offering specific hardware instructions and registers designed for matrix computations, it increases the efficiency of these operations. Multiplication and convolution are two basic matrix operations that are used in many different computer tasks, particularly in machine learning methods.
The Intel Data Streaming Accelerator (IntelDSA) allows developers to fully use their data-driven workloads by improving data processing and analytics capabilities for a variety of applications. DSA gives you access to hardware acceleration that is optimized and provides outstanding performance for operations involving a lot of data.
The Intel In-Memory Analytics Accelerator (Intel IAA) is a prospective higher power efficiency accelerator that runs analytic and database applications. In-memory databases, open-source databases, and data stores like RocksDB and ClickHouse are supported by in-memory compression, decompression, encryption at very high throughput, and a set of analytics primitives.
By offloading encryption, decryption, and compression, Intel QuickAssist Technology (Intel QAT) accelerators free up CPU cores and lower power consumption. Additionally, it allows encryption and compression to be combined into a single data flow.
All R7i instance sizes have access to Advanced Matrix Extensions. The instances r7i.metal-24xl and r7i.metal-48xl will support the Intel QAT, Intel IAA, and Intel DSA accelerators.
Reduced Total Cost/Adaptability/Optimal Solution Selection
Compared to R6i instances, R7i instances offer price performance that is up to 15% better. With up to 192 vCPUs and 1,536 GiB of memory, R7i instances can provide up to 1.5x more virtual CPUs and memory than R6i instances, allowing you to consolidate workloads on fewer instances.
R7i instances come with the newest DDR5 RAM and larger instance sizes up to 48xlarge. Additionally, clients using R7i instances can now attach up to 128 EBS volumes (compared to 28 EBS volume attachments on R6i).
The variety and depth of EC2 instances available on AWS are enhanced by R7i instances. R7i offers 11 sizes with different capacities for vCPU, memory, networking, and storage, including two bare-metal sizes (r7i.metal-24xl and r7i.metal-48xl) that are coming shortly.
Accessibility
The following AWS Regions are home to R7i instances:
Europe (Stockholm, Spain), US West (Oregon), and US East (North Virginia, Ohio).
Businesses and partners in the big data and in-memory database analytics computing community may now meet their future demands for high performance, efficiency, TCO, and transformation thanks to the announcement of Intel and AWS’s new R7i instance.
Read more on Govindhtech.com
#govindhtech#technology#technews#news#ai#bigdata#r7iInstances#R6iInstances#EC2Instances#DDR5RAM#machinelearning
0 notes
Text
Seven Big Data Challenges and How to Solve Them
Organizations can get stuck at the early stages of Big Data projects because they might not be aware of Big Data challenges and how to solve them. We will take a look at some of the most common scary Big Data challenges and how to solve the challenges if we ever encounter them.
1st Big Data Challenge: Lack of In-Depth Understanding of Big Data
One of the major Big Data challenges is the lack of proper understanding of Big Data. Some employees in an organization might struggle to understand the proper meaning of Big Data, which can lead to such an organization lacking the needed Big Data initiatives that will help with decision-making. If an organization does not have a sufficient understanding of Big Data basics and the benefits it offers, there’s a high tendency of their Big Data adoption projects failing.
The Solution:
The top management at every organization should adopt a Big Data culture. This involves arranging Big Data workshops and training for staff at all levels. Employees can also enroll in Big Data Online Course or register for Big Data Hadoop Certification. This will encourage the acceptance and proper understanding of Big Data at all levels, which in turn promotes change.
2nd Big Data Challenge: Difficulty in Managing Big Data Growth
Just as the name implies, Big Data can experience huge growth over a period of time. When this happens, it can be quite difficult to handle the massive data growth. Also, adjusting to this Big Data growth turns out to be a serious challenge that needs to be addressed. From Big Data online training research, it is estimated that the amount of data stored in IT data centers will continue to accumulate for years to come. So, figuring out how to manage Big Data growth storage will become a pressing challenge that needs to be addressed and solved.
The Solution:
The best way to solve a Big Data growth challenge is to learn how to manage large data sets. Some techniques can be deployed such as tiering, deduplication, and compression. When data is compressed, the number of bits in the data is reduced which will then reduce the data size. Deduplication of data involves removing unwanted data duplicates, while the tiering technique involves storing data in different cloud storage tiers. A Big Data tool like Hadoop can be used to solve this challenge. The Big Data Hadoop Certification will be useful for this issue.
3rd Big Data Challenge: Lack of Skilled Data Professionals
Big Data is a modern-day technology that requires the control of skilled data professionals. They include Data Analysts, Data Scientists, and Data Engineers. These are certified data professionals with Big Data online training and certification. They have the experience of handling sophisticated Big Data tools and they are in high demand across the industry.
The Solution:
A good way to clear this roadblock is to encourage employers to focus on hiring skilled data professionals. Companies can also support the professional development of employees that work with data by enrolling them in Big Data online training. They can also pay for a Big Data Hadoop Certification to help their employees remain loyal to the course of Big Data culture.

4th Big Data Challenge: Confusion with Big Data Technology Selection
There is a massive variety of Big Data technologies available in the market. This means it can be difficult to determine the right technology to use for a particular Big Data project. Confusion might set in when differentiating the technology to use in storing data or the one that will offer the fastest speed. Is it Spark, HBase, Cassandra, or Hadoop? This can be a serious challenge.
The Solution:
The best way to solve this Big Data challenge is to seek professional help. You can hire the services of a Big Data consultant or expert who will guide you on how to use the various Big Data technologies available in the market. Another good strategy involves getting in-depth knowledge of Big Data technologies from a Big Data online course to understand them better.
5th Big Data Challenge: Big Data Security Breaches
One of the most daunting challenges of Big Data lies in how to secure a huge data set. Many organizations focus more on gathering data, analyzing data, and storing data that they leave data security for later. If data sets are not properly secured, hackers can breach through and steal vital information. Companies and organizations can lose a lot of money if this happens.
The Solution:
A good way to solve Big Data security challenges is to put data security first. Data security is very important across every stage of Big Data projects, especially at the design stages. Another effective way to solve data security challenges is for companies to hire security professionals. Other steps that can help include Data Encryption and Real-Time Security Monitoring.
6th Big Data Challenge: Difficulty in Data Integration from Variety of Sources
Data can be integrated from a variety of sources but it is a task that can be quite challenging. For instance, data can be integrated from sources like emails, customer logs, data reports, social media platforms, and even financial reports. The integration of data is vital for data analysis, so it is important to learn data integration for compiling reports and data analysis.
The Solution:
The best way to solve data integration issues is to purchase the right tools. Several tools can be used for data integration from a variety of sources; they include Microsoft SQL, IBM InfoSphere, CloverDX, Centerprise Data Integrator, Xplenty, QlikView, and Oracle Data Service Integrator. You can check out the Big Data online training course to learn how to use these tools.
7th Big Data Challenge: Big Data Handling Expenses
Handling Big Data involves spending a lot of money. These expenses include power supply, new hardware, and recruitment of data professionals. If the software is newly developed, a lot of money will go into configuration and maintenance. Whether a company is using an on-premises data solution or a cloud-based water solution, it is important to learn how to handle the costs.
The Solution:
A good way to reduce the cost of Big Data projects is to analyze company needs and deploy the best Big Data solutions for the project. Applying Big Data on-premises solutions, cloud-based solutions and hybrid solutions will go a long way to reduce the
Cost of handling Big Data in organizations. These are cost-effective solutions that will help you handle data properly.
Tags: BigData Classes with Certification, Big Data Hadoop Online Training, Big Data Hadoop at H2k infosys, Big Data Hadoop, big data analysis courses, online big data courses, Big Data Hadoop Online Training and 100% job guarantee courses, H2K Infosys, Big Data Fundamentals, Hadoop Architecture, HDFS Setup and Configuration, Programming,Management,HBase Database, Hive Data Warehousing, Pig Scripting, Apache Spark, Kafka Streaming, Data Ingestion and Processing, Data Transformation
#BigDataClasseswithCertification #BigDataHadoop #BigDataHadoopCourseOnline #BigDataHadoopTraining #BigDataHadoopCourse, #H2KInfosys, #ClusterComputing, #RealTimeProcessing, #MachineLearning, #AI, #DataScience, #CloudComputing#BigDataAnalytics, #DataEngineering
Contact: +1-770-777-1269
Mail: [email protected]
Location: Atlanta, GA - USA, 5450 McGinnis Village Place, # 103 Alpharetta, GA 30005, USA.
Facebook: https://www.facebook.com/H2KInfosysLLC
Instagram: https://www.instagram.com/h2kinfosysllc/
Youtube: https://www.youtube.com/watch?v=BxIG2VoC70c
Visit: https://www.h2kinfosys.com/courses/hadoop-bigdata-online-training-course-details BigData Hadoop Course: bit.ly/3KJClRy
#education#h2kinfosys#online training#online course#online courses#big data#hadoop#hadoop big data#bigdata#hadooptraining
0 notes
Text
TRENDING TECHNOLOGIES
What is Big Data?
Big data is a term used to describe a collection of unstructured, organized, and semi-structured large amounts of data that have been gathered by many organizations and contain a wide range of information. The Fresh York Stock Exchange (NYSE), for instance, produces around one terabyte of new trade data each year as an example of big data.
Big Data can also be described by the following categories:
Volume: - As it relates to the volume of data a firm or organization has, it is the most significant big data attribute. Terabytes, gigabytes, zettabytes, and yottabytes are units of measurement for data volume. The volume of the data is a very important factor in assessing its value.
Variety: - Another feature of big data informs us of the various data types obtained by various sources. As it affects performance, it is the biggest problem the data industry is currently facing.
Velocity: - It speaks about how quickly data is generated and processed. Any big data process must have high velocity. It establishes the true potential of the data.
Importance of Big Data
Organizations may harness their data and use big data analytics to find new opportunities. This results in wiser company decisions, more effective operations, greater profitability, and happier clients. Businesses that employ big data and advanced analytics benefit in a variety of ways, including cost reduction, quicker and better decision-making, the development and marketing of new goods and services, etc.
Latest technologies used in big data industry
Artificial Intelligence: - It is one of the trending technologies. Big Data is playing a key role in the advancement of AI through its two subgroups: Machine Learning and Deep Learning.
Machine Learning: - It refers to the ability of computers to learn without being monotonously programmed. Applying this to Big Data analytics enables systems to analyse historical data, recognize patterns, build models, and predict future outcomes. Deep learning is a type of machine learning that mimics the working of human brain by creating artificial neural networks that use multiple layers of the algorithm to analyse data.
Predictive analysis is a subpart of big data analytics, and primarily works towards predicting future behaviour by using prior data. It works by leveraging Data mining, Machine Learning technologies, and statistical modelling along with some mathematical models to forecast future events. With the help of predictive analytics models, organizations can organize historical as well as the latest data to strain out trends and behaviours that could occur at a particular time.
HADOOP: - It is currently one of the evolving big data tools. It is an open-source software framework developed for storing and processing Big Data by Apache Software Foundation. Hadoop processes and stores data in a distributed computing environment across the cluster of commodity hardware Hadoop is a profitable, fault-tolerant, and highly available framework that can process data of any size and format and is a very unfailing storage tool, also enables you to cluster several computers to analyze large datasets in parallel and more quickly.
MongoDB: - Released in February2009, Mongo is an open-source software to store large scale data and allow to work with the data efficiently is a document-oriented, NoSQL database written in C, C++, and JavaScript and easy to set up. MongoDB is a profitable and highly reliable Big Data technology. It has a powerful query language that supports geo-based search, aggregation, text search, graph search, and more.
R: - It denotes to an open-source project and programming language. A free software that is mainly used for statistical computing, visualization, and integrated developing environments like Eclipse and Visual Studio assistance communication. It has been increasing popularly over the past few years in universities and colleges. According to specialists, the R programming language has the most prominent language across the world. Data miners and statisticians widely use it to design statistical software, primarily in data analytics.
Blockchain: - It is a distributed database system that stores and manages the transaction. This technology plays a crucial role in working towards reducing fraudulent transactions and helps increase financial security.
Conclusion
In applying the analytical power of Big Data Technologies to their supreme potential, businesses can guarantee that their success in every aspect of operations will reach new altitudes, and they can continue to become more competitive in the marketplace. About Rang Technologies: Headquartered in New Jersey, Rang Technologies has dedicated over a decade delivering innovative solutions and best talent to help businesses get the most out of the latest technologies in their digital transformation journey. Read More...
0 notes