#Fp64 precision nvidia
Explore tagged Tumblr posts
Text
Upgrade Your Computing Power: NVIDIA H100 80GB Stock Available Now at VIPERA!
Are you ready to elevate your computing experience to unparalleled heights? Look no further, as VIPERA proudly announces the availability of the NVIDIA H100 80GB, a game-changer in the world of high-performance GPUs. Donât miss out on this opportunity to supercharge your computational capabilities â order your NVIDIA H100 80GB now exclusively at VIPERA!
Specifications:
Form Factor:
H100 SXM
H100 PCIe
Performance Metrics:
FP64 (Double Precision):
H100 SXM: 34 teraFLOPS
H100 PCIe: 26 teraFLOPS
FP64 Tensor Core:
H100 SXM: 67 teraFLOPS
H100 PCIe: 51 teraFLOPS
FP32 (Single Precision):
H100 SXM: 67 teraFLOPS
H100 PCIe: 51 teraFLOPS
TF32 Tensor Core:
H100 SXM: 989 teraFLOPS
H100 PCIe: 756 teraFLOPS
BFLOAT16 Tensor Core:
H100 SXM: 1,979 teraFLOPS
H100 PCIe: 1,513 teraFLOPS
FP16 Tensor Core:
H100 PCIe: 1,513 teraFLOPS
H100 SXM: 1,979 teraFLOPS
FP8 Tensor Core:
H100 SXM: 3,958 teraFLOPS
H100 PCIe: 3,026 teraFLOPS
INT8 Tensor Core:
H100 SXM: 3,958 TOPS
H100 PCIe: 3,026 TOPS
GPU Memory:
80GB
Unmatched Power, Unparalleled Possibilities:
The NVIDIA H100 80GB is not just a GPU; itâs a revolution in computational excellence. Whether youâre pushing the boundaries of scientific research, diving into complex AI models, or unleashing the full force of graphic-intensive tasks, the H100 stands ready to meet and exceed your expectations.

Why Choose VIPERA?
Exclusive Availability: VIPERA is your gateway to securing the NVIDIA H100 80GB, ensuring you stay ahead in the technological race.
Unrivaled Performance:Â Elevate your projects with the unprecedented power and speed offered by the H100, setting new standards in GPU capabilities.
Cutting-Edge Technology:Â VIPERA brings you the latest in GPU innovation, providing access to state-of-the-art technologies that define the future of computing.
Donât miss out on the chance to revolutionize your computing experience. Order your NVIDIA H100 80GB now from VIPERA and unlock a new era of computational possibilities!
M.Hussnain Visit us on social media: Facebook Twitter LinkedIn Instagram YouTube TikTok
#nvidia#nvidiah100#nvidiah10080gb#availablestock#h100available#nvidiah100stock#viperatech#vipera#stockavailable
0 notes
Text
AMD Ryzen 7995WX: Crushing TFLOPs of Xbox Series X & PS5!

AMD Ryzen Threadripper 7995WX CPU Has More FP32 TFLOPs Than Xbox Series X, PS5, RTX 3060 GPU
The AMD Ryzen Threadripper 7995WX central processing unit (CPU) is a monstrous piece of hardware. Not only has it established new benchmarks for multi-threaded performance, but it also has capabilities for FP32 computation that are astonishingly high. These capabilities are faster than the most recent generation of gaming consoles and are on par with some of the most well-known GPUs now available on the market.
Its FP32 Computing Capabilities Are Very Impressive, Offering More TFLOPs Than the Xbox Series X and PS5 Consoles
The AMD Ryzen Threadripper PRO incorporates 96 cores, 192 threads, 384 MB of L3 cache, and clock rates that may go as high as 5.15 GHz into a chip that has a thermal design power (TDP) of 350 watts. This allows the processor to achieve unprecedented levels of performance.
Additionally, the cache has a size of 384 megabytes. Despite the fact that the device was created for high-end workstations and desktop PCs, it appears as though the chip may also offer some decent software emulation capabilities directly off the CPU. This is the case despite the fact that the hardware appears to have these capabilities.
Taking a look at the performance characteristics of the chip that were published on Github (via InstLatX64), we can see that the chip offers up to 12.16 TFLOPs of FP32 (Single-Precision) Compute performance and 6.0 TFLOPs of FP64 (Double-Precision) Compute performance within the AIDA64 GPGPU test. This information is available to us because the characteristics of the chipâs performance were made public. The benchmark makes use of native x64 machine code; hence, it should not be compared to GPU benchmarks that make use of the OpenCL application programming interface (API).
These benchmarks are designed exclusively for research that evaluates the performance of multiple system configurations. An increase that is about five times greater is provided by the Threadripper 7995WX when compared to the performance that is provided by an Intel Core i9-13900K CPU, which is around 2.5 TFLOPs.
On the other hand, letâs move on to the more amusing part of our discussion, which is a comparison with the most recent generation of game consoles created by Microsoft and Sony. On the PlayStation 5, the greatest FP32 compute power available is 10.29 TFLOPs, whereas on the Xbox Series X, the maximum FP32 compute power available is 12.15 TFLOPs. According to Steamâs Hardware Survey, the most popular graphics processing unit (GPU) is the NVIDIA GeForce RTX 3060 (12.7 TFLOPs).
The Steam Hardware Survey also found that the AMD Ryzen Threadripper PRO 7995WX CPU not only outperforms both of the aforementioned gaming consoles in terms of raw TFLOPs, but it is also practically on par with this GPU.
Read more on govindhtech.com
0 notes
Text
Fp64 precision nvidia
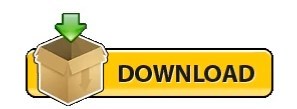
#FP64 PRECISION NVIDIA SOFTWARE#
#FP64 PRECISION NVIDIA PROFESSIONAL#
Networks: ShufeNet-v2 (224x224), MobileNet-v2 (224x224) Pipeline represents end-to-end performance with video capture and decode. But if money is truly no object, we couldnât fault you for chasing that extra 5%. NVIDIA T4 ShufeNet v2 NVIDIA A2 SystemConguration: Supermicro SYS-1029GQ-TRT, 2S Xeon Gold 6240 â2.6GHz, 512GB DDR4, 1x NVIDIA A2 OR 1x NVIDIA T4 Measured performance with Deepstream 5.1. The Gigabyte Aorus GeForce RTX 2080 Ti Xtreme 11G we tested offered more than 95% of Titan Vâs average frame rate. FP64 (double-precision) and FP16 (half-precision) arithmetic units. Affluent gamers are better off with an overclocked GeForce RTX 2080 Ti from one of Nvidiaâs board partners. Last but not least, those interested in double-precision capabilities (FP64) of consumer Ampere need to know there are two dedicated FP64 cores per SM, or exactly 1/64th of FP32. Recent developments in the GPU sector have opened up new avenues for boosting performance.
#FP64 PRECISION NVIDIA PROFESSIONAL#
In the meantime, a complete TU102 processor is an absolute monster for professional applications able to utilize its improved Tensor cores or massive 24GB of GDDR6 memory, including deep learning and professional visualization workloads. Pascal is the codename for a GPU microarchitecture developed by Nvidia, as the successor to the Maxwell architecture. For example, on a GTX 780 Ti, the FP64 performance is 1/24 FP32. So vendors like NVIDIA and AMD do not cram FP64 compute cores in their GPUs. This is because they are targeted towards gamers and game developers, who do not really care about high precision compute. Itâll be interesting to see how Nvidia enables Turingâs highest-profile fixed-function feature. GPUs, at least consumer grade, are not built for high performance FP64.
#FP64 PRECISION NVIDIA SOFTWARE#
Nevertheless, Nvidia says itâs working with rendering software partners to exploit ray tracing acceleration through Microsoft DXR and OptiX, citing pre-release versions of Chaos Groupâs Project Lavina, OTOYâs OctaneRender, and Autodeskâs Arnold GPU renderer. That latter synthetic shows Titan RTX about 6% ahead of GeForce RTX 2080 Ti, with both Turing-based cards way ahead of Titan V. Through enhancements in NVIDIA CUDA-X math. Mostly, there isnât anything to test outside of Battlefield V and 3DMark's Port Royal. The third generation of Tensor Cores in A100 enables matrix operations in full, IEEE-compliant, FP64 precision. Iterative refinement for dense systems, Ax b, can work in a manner simlar to the pseudocode snippet below. Up to 5.2 TFLOPS FP64 double-precision floating-point performance (10. We didnât even touch on Titan RTXâs RT cores, which accelerate BVH traversal and ray casting functions. The approach is very simple: use lower precision to compute the expensive flops and then iteratively refine the solution in order to achieve the FP64 solution.
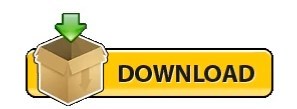
0 notes
Text
NVIDIA TITAN RTX
NVIDIA's TITAN arrangement of designs cards has been a fascinating one since the dispatch of the first in 2013. That Kepler-based GTX TITAN model topped at 4.5 TFLOPS single-exactness (FP32), execution that was supported to 5.1 TFLOPS with the arrival of the TITAN Black the next year.
Quick forward to the current day, where we presently have the TITAN RTX, bragging 16.3 TFLOPS single-accuracy, and 32.6 TFLOPS of half-exactness (FP16). Twofold exactness (FP64) used to be standard admission on the prior TITANs, however today, you'll need the Volta-based TITAN V for opened execution (6.1 TFLOPS), or AMD's Radeon VII for in part opened execution (3.4 TFLOPS).
Of late, half-accuracy has collected a great deal of consideration by the ProViz showcase, since it's optimal for use with profound learning and AI, things that are developing in fame at an incredibly brisk pace. Add explicitly tuned Tensor centers to the blend, and profound learning execution on Turing turns out to be genuinely amazing.
NVIDIA TITAN RTX Graphics Card
Tensors are not by any means the only party stunt the TITAN RTX has. Like the remainder of the RTX line (on both the gaming and genius side), RT centers are available in the TITAN RTX, helpful for quickening continuous beam following outstanding tasks at hand. The centers should be explicitly bolstered by engineers, utilizing APIs, for example, DXR and VKRay. While support for NVIDIA's innovation began lukewarm, industry support has grown a great deal since the first disclosing of RTX at SIGGRAPH a year ago.
At E3 in June, a bunch of games had beam following related declarations, including Watch_Dogs: Legion, Cyberpunk 2077, Call of Duty: Modern Warfare, and obviously, Quake II RTX. On the plan side, a few designers have just discharged their RTX quickened arrangements, while a lot more are underway. NVIDIA has been gabbing of late about the Adobes and Autodesks of the world assisting with developing the rundown of RTX-implanted programming. We wouldn't be astonished if more RTX goodness was uncovered at SIGGRAPH this year once more.
For profound learning, the TITAN RTX's solid FP16 execution is quick all alone, however there are a couple of advantages locally available to help take things to the following level. The Tensor centers help in a significant part of the increasing speed, however the capacity to utilize blended exactness is another enormous part. With it, insignificant information following will be put away in single-accuracy, while the key information will get crunched into equal parts exactness. Everything consolidated, this can support preparing execution by 3x over the base GPU.
NVIDIA's TITAN RTX and GeForce RTX 2080 Ti - Backs
Likewise eminent for Turing is simultaneous number/coasting point tasks, which permits games (or programming) to execute INT and FP activities in equal without stumbling over one another in the pipeline. NVIDIA has noted in the past that with games like Shadow of the Tomb Raider, an example set of 100 guidelines included 62 FP and 38 INT, and that this simultaneous component legitimately improves execution accordingly.
Another significant element of TITAN RTX is its capacity to utilize NVLink, which basically consolidates the memory pools of two cards together, bringing about a solitary framebuffer that can be utilized for the greatest potential tasks. Since GPUs scale commonly very well with the sorts of outstanding tasks at hand the card focuses on, it's the genuine memory pooling that is going to offer the best advantage here. Gaming content that could likewise exploit multi-GPU would see an advantage with two cards and this connector, also.
Since it's an element selective to these RTX GPUs at the present time, it merits referencing that NVIDIA likewise packages a VirtualLink port at the back, permitting you to connect your HMD for VR, or in the most pessimistic scenario, use it as a full-fueled USB-C port, either for information move or telephone charging.
With the entirety of that secured, we should investigate the general current NVIDIA workstation stack:
NVIDIA's Quadro and TITAN Workstation GPU Lineup
Cores Base MHz Peak FP32 Memory Bandwidth TDP Price
GV100 5120 1200 14.9 TFLOPS 32 GB 8 870 GB/s 185W $8,999
RTX 8000 4608 1440 16.3 TFLOPS 48 GB 5 624 GB/s ???W $5,500
RTX 6000 4608 1440 16.3 TFLOPS 24 GB 5 624 GB/s 295W $4,000
RTX 5000 3072 1350 11.2 TFLOPS 16 GB 5 448 GB/s 265W $2,300
RTX 4000 2304 1005 7.1 TFLOPS 8 GB 1 416 GB/s 160W $900
TITAN RTX 4608 1350 16.3 TFLOPS 24 GB 1 672 GB/s 280W $2,499
TITAN V 5120 1200 14.9 TFLOPS 12 GB 4 653 GB/s 250W $2,999
P6000 3840 1417 11.8 TFLOPS 24 GB 6 432 GB/s 250W $4,999
P5000 2560 1607 8.9 TFLOPS 16 GB 6 288 GB/s 180W $1,999
P4000 1792 1227 5.3 TFLOPS 8 GB 3 243 GB/s 105W $799
P2000 1024 1370 3.0 TFLOPS 5 GB 3 140 GB/s 75W $399
P1000 640 1354 1.9 TFLOPS 4 GB 3 80 GB/s 47W $299
P620 512 1354 1.4 TFLOPS 2 GB 3 80 GB/s 40W $199
P600 384 1354 1.2 TFLOPS 2 GB 3 64 GB/s 40W $179
P400 256 1070 0.6 TFLOPS 2 GB 3 32 GB/s 30W $139
Notes 1 GDDR6; 2 GDDR5X; 3 GDDR5; 4 HBM2
5 GDDR6 (ECC); 6 GDDR5X (ECC); 7 GDDR5 (ECC); 8 HBM2 (ECC)
Design: P = Pascal; V = Volta; RTX = Turing
The TITAN RTX matches the Quadro RTX 6000 and 8000 for having the most elevated number of centers in the Turing lineup. NVIDIA says the TITAN RTX is around 3 TFLOPS quicker in FP32 over the RTX 2080 Ti, and luckily, we have results for the two cards covering a wide-scope of tests to perceive how they analyze.
What's not found in the specs table above is the real execution of the beam following and profound learning segments. This next table enables away from of that to up:
NVIDIA's Quadro and TITAN â RTX Performance
RT Cores RTX-OPS Rays Cast 1 FP16 2 INT8 3 Deep-learning 2
TITAN RTX 72 84 T 11 32.6 206.1 130.5
RTX 8000 72 84 T 10 32.6 206.1 130.5
RTX 6000 72 84 T 10 32.6 206.1 130.5
RTX 5000 48 62 T 8 22.3 178.4 89.2
RTX 4000 36 43 T 6 14.2 28.5 57
Notes 1 Giga Rays/s; 2 TFLOPS; 3 TOPS
You'll see that the TITAN RTX has a higher "beams cast" spec than the top Quadros, which may owe its gratitude to higher timekeepers. Different specs are indistinguishable over the best three GPUs, with evident downsizing occurring as we move descending. Right now, the Quadro RTX 4000 (approximately a GeForce RTX 2070 equal) is the most reduced end current-gen Quadro from NVIDIA. Once more, SIGGRAPH is nearly upon us, so it may be the case that NVIDIA will have an equipment shock coming up; maybe a RTX 2060 Quadro identical.
When the RTX 2080 Ti as of now offers so much execution, who precisely is the TITAN RTX for? NVIDIA is focusing on it to a great extent at scientists, yet it optionally goes about as one of the quickest ProViz cards available. It could be selected by the individuals who need the quickest GPU arrangement going, and also a colossal 24GB framebuffer. 24GB may be excessive for a ton of current perception work, yet with profound learning, 24GB gives a great deal of breathing room.
In spite of all it offers, TITAN RTX can't be called an "extreme" answer for ProViz since it comes up short on some Quadro enhancements that the namesake GPUs have. That implies in certain top of the line structure suites like Siemens NX, a genuine Quadro may demonstrate better. Yet, on the off chance that you don't utilize any outstanding tasks at hand that experience explicit upgrades, the TITAN RTX will be very appealing given its list of capabilities (and that framebuffer!) If you're at any point befuddled about advancements in your product of decision, if you don't mind leave a remark!
Two or three years prior, NVIDIA chose to give some affection to the TITAN arrangement with driver upgrades that brings some equality among TITAN and Quadro. We would now be able to state that TITAN RTX appreciates a similar sort of execution helps that the TITAN Xp completed two years prior, something that will be reflected in a portion of the charts ahead.
Test PC and What We Test
On the accompanying pages, the consequences of our workstation GPU test gauntlet will be seen. The tests picked spread a wide scope of situations, from rendering to figure, and incorporates the utilization of both manufactured benchmarks and tests with true applications from any semblance of Adobe and Autodesk.
Nineteen designs cards have been tried for this article, with the rundown commanded by Quadro and Radeon Pro workstation cards. There's a sound sprinkling of gaming cards in there also, in any case, to show you any conceivable streamlining that might be occurring on either.
It would be ideal if you note that the testing for this article was directed a few months prior, before an invasion of movement and item dispatches. Illustrations card drivers discharged since our testing may improve execution in specific cases, however we wouldn't anticipate any eminent changes, having mental soundness checked a lot of our typical tried programming on both AMD and NVIDIA GPUs. In like manner, the past rendition of Windows was utilized for this specific testing, yet that additionally didn't uncover any burdens when we rational soundness checked in 1903.
As of late, we've invested a ton of energy cleaning our test suites, and furthermore our interior testing contents. We're right now during the time spent rebenchmarking various GPUs for a forthcoming glance at ProViz execution with cards from both AMD's Radeon RX 5700 and NVIDIA's GeForce SUPER arrangement. Luckily, results from those cards don't generally eat into a top-end card like the TITAN RTX, so lateness hasn't thwarted us this time.
The specs of our test rig are seen beneath:
Techgage Workstation Test System
Processor Intel Core i9-9980XE (18-center; 3.0GHz)
Motherboard ASUS ROG STRIX X299-E GAMING
Memory HyperX FURY (4x16GB; DDR4-2666 16-18-18)
Graphics AMD Radeon VII (16GB)
AMD Radeon RX Vega 64 (8GB)
AMD Radeon RX 590 (8GB)
AMD Radeon Pro WX 8200 (8GB)
AMD Radeon Pro WX 7100 (8GB)
AMD Radeon Pro WX 5100 (8GB)
AMD Radeon Pro WX 4100 (4GB)
AMD Radeon Pro WX 3100 (4GB)
NVIDIA TITAN RTX (24GB)
NVIDIA TITAN Xp (12GB)
NVIDIA GeForce RTX 2080 Ti (11GB)
NVIDIA GeForce RTX 2060 (6GB)
NVIDIA GeForce GTX 1080 Ti (11GB)
NVIDIA GeForce GTX 1660 Ti (6GB)
NVIDIA Quadro RTX 4000 (8GB)
NVIDIA Quadro P6000 (24GB)
NVIDIA Quadro P5000 (12GB)
NVIDIA Quadro P4000 (8GB)
NVIDIA Quadro P2000 (5GB)
Audio Onboard
Storage Kingston KC1000 960G
click here to know more
1 note
·
View note
Text
Tesla p100 fp64

#TESLA P100 FP64 PRO#
#TESLA P100 FP64 SOFTWARE#
#TESLA P100 FP64 PROFESSIONAL#
The new Volta SM is 50% more energy efficient than the previous generation Pascal design, enabling major boosts in FP32 and FP64 performance in the same power envelope. New Streaming Multiprocessor (SM) Architecture Optimized for Deep Learning Volta features a major new redesign of the SM processor architecture that is at the center of the GPU. This table 1 compares NVIDIA Âź Tesla Âź accelerators over the past 5 years. (Measured on pre-production Tesla V100.) COMPARISONS Right: Given a target latency per image of 7ms, Tesla V100 is able to perform inference using the ResNet-50 deep neural network 3.7x faster than Tesla P100. AMD on the flip side can implement theirs on their own processors, and Intel who knows.Figure 1: Left: Tesla V100 trains the ResNet-50 deep neural network 2.4x faster than Tesla P100. FLOPs are great, but you still have to feed them and not require an entire team of grad students to implement it.ÄźDIT: Read through the devblog and I guess they are getting unified memory added, only concern is performance not over NVLink and the feature (with good performance at least) being limited to PowerPC. With 3D memory(DDR, not just HBM) coming that could be a huge boost for a lot of HPC tasks and they'd pay for it. I've ran into that headache before(granted it was a a 1400 core cluster and not a GPU), but managing memory for large data sets can be a nightmare.
#TESLA P100 FP64 SOFTWARE#
Some of the scientific modeling guys would be the opposite.ÄȘs for the software side, something like AMDs HSA work and letting GPUs address system memory transparently seems like it might be more significant. My understanding of deep learning is they hammer away at FP16, and would seldom use FP64. Using NVLink they could still link different adapters together to achieve performance goals. I'd think splitting it into a Tesla with strong FP64 performance and a Quadro with strong FP16/32 might be more practical. It's an impressive piece of hardware, my only concern is how useful both FP16 and FP64 are together. Regarding costs, just worth pointing out that NVIDIA will also continue selling the Tesla K and M range, for now anyway but here AMD can compete in terms of hardware precision (albeit just not compete in the broad software integration-relationships NVIDIA currently has).
#TESLA P100 FP64 PROFESSIONAL#
Which ever way you look at it putting cost aside (which will work in AMDs favour for some clients below large scale HPC-research), this is a pretty impressive card for the HPC-research world and meets the requirements for a very large demographic of it, in the professional workstation environment I assume they will change this subtly for Quadro cards. P100 has 2x performance to AMD FirePro S9170 at FP32, AMD's position is further compounded that NVIDIA is pushing FP16 as part of mix precision future solutions and this is 2x performance to NVIDIAs Pascal FP32 (theory only and will need real world example).
#TESLA P100 FP64 PRO#
P100 has 2x performance to AMD FirePro S9170 at FP64 (this is Hawaii and will improve on the 14nm but worth noting the 290x was 1.4 tflops to the S9170 2.6 tflops so there will still be a restricting ratio relationship between built for consumer and the pro version, the earlier S9100 was I think 2.1 fltops) P100 as mentioned has over 5x the FP64 performance of AMD Duo Pro (however similar situation to NVIDIA they sell their older architecture for FP64, the now superseded Tesla Kepler against S9100 range Hawaii) Using a mix of both puts 32/16 the P100 in a very strong position, and separately shows how much FP64 is on this die. P100 has fp16 that is 2x its performance of its P100 fp32 - currently I think the GCN architecture is limited in FP16 implementation (for AMD fingers crossed their 14nm pro cards has better support although still needs to be shown by NVIDIA-real world applications how much this can help with Deep Learning) P100 is roughly 30% slower to AMD Duo Pro at FP32 but has over 5x more FP64 performance. Usually the AMD figure is a theoretical peak tflops, as I mentioned before NVIDIAs are not perfect but probably get the edge with real-world scientific applications due to their software experience-CUDA-relationship with said software companies. That is where the power is with this card IMO, and AMD will definitely need to get FP16 working well as this could be a big part in the future of deep learning. This is a mixed precision GPU and I get the feeling NVIDIA with their software expertise will be pushing for clients to mix the use of FP64 and FP32, and mix the use of the FP32 and FP16. I really feel many are missing what has been achieved with this chip. I have been reading recently several additional forum sites beyond those I am a member and responses to the Tesla P100 and where people are being critical in it only has so much double precision only bit better than older cards/it only has so much single precision worst than AMD Duo Pro.

0 notes
Text
Nvidia gtx titan fp64

#Nvidia gtx titan fp64 install#
#Nvidia gtx titan fp64 windows#
Moreover, GTX Titan is smaller, more silent and less power-hungry, thus fitting more types of configurations. GeForce GTX Titan is more multipurpose: it has larger memory size with fast access time, winning at high resolutions. The other top solution, GTX 690, is made for quite different use: to get maximum fps ignoring the drawbacks of multi-chip AFR-rendering. GeForce GTX Titan doesn't replace any of NVIDIA's video cards. From the angle of marketing this looks reasonable: the GPU is used in the world's fastest Titan supercomputer (as well as in NVIDIA Tesla K20X), therefore, the name Titan will surely be associated with the high-end hardware. NVIDIA's flagship video adapter received a word instead of a numerical rating in its name.
Up to 250W power consumption (8-pin and 6-pin power connectors).
2 x Dual Link DVI-I, Mini HDMI, DisplayPort 1.2.
187.3 Gtexel/s theoretical texture fetch.
40.1 Gpixel/s theoretical peak fillrate.
4.5/1.3 TFLOPS calculating performance (FP32/FP64).
6008 (4 x 1502) MHz effective memory clock rate.
GeForce GTX Titan reference specifications
Integrated support for 4 displays at the same time (2 x Dual Link DVI, HDMI 1.4a, DisplayPort 1.2).
Integrated support for RAMDAC, 2 x Dual Link DVI, HDMI, DisplayPort.
6 wide ROPs (48 pixels) supporting antialiasing up to 32x, also with FP16, FP32 frame buffers each unit features an array of configurable ALUs and handles Z generation and comparison, MSAA, blending.
240 texture addressing and filtering units supporting FP16, FP32 precision in textures, as well as support for trilinear and anisotropic filtering for all texture formats.
15 Stream Multiprocessors, including 2880 scalar ALUs for FP32 and 960 scalar ALUs for FP64 computing according to the IEEE 754-2008 standard).
876MHz average boost clock rate (the standard is 836 MHz).
384-bit memory bus, 6 independent 64-bit controllers, support for GDDR5.
Hardware support for the DirectX 11 API, including the Shader Model 5.0, geometry and compute shaders, as well as tessellation.
Unified architecture with an array of processors for stream processing of vertices, pixels, etc.
GK110 came to the gaming hardware market right from the Tesla segment. Computational only.Later, AMD released its fastest single-chip solution, Radeon HD 7970 GHz Edition, so NVIDIA was finally forced to make the move. That being said, FP64 is likely used for the reasons mentioned above. I wonder if you can modify ampere to become a workstation card. Maybe if that video card had ECC VRAM, the performance or accuracy would of improved (for 3D rendering) but I can't confirm. The other point to this debate is the lack of ECC VRAM on the GTX 780 / consumer card. Remember that the GTX Titan was also a similar chip (GK110- 400) but had the "Titan driver" to unlock FP64 performance. However, I mention this because this is still common practice (soft limit performance) to keep workstation cards at a higher price. Ultimately, it was better to leave the card as a 780. I hoped it would improve performance in 3D rendering based applications but I was wrong. I don't recall if we modified the vBIOS but I do recall the system read the card as a Tesla/Quadro.
NVIDIA Quadro K6000 Specs - FP64 (double) performance 1.732 TFLOPS (1:3).
NVIDIA GeForce GTX 780 Specs - FP64 (double) performance 173.2 GFLOPS (1:24).
NVIDIA Tesla K20c Specs - FP64 (double) performance1,175 GFLOPS (1:3).
Which theoretically unlocked FP64 performance.
#Nvidia gtx titan fp64 install#
It altered how the card read itself and we were able to install a driver to make the system believe it was a Tesla K20 but it may have been a Quadro (k6000). It's been a while so I don't recall the details. With that card, my brother used a graphite pencil and I believe connected two resistors. Headless works, but is a significant downgrade in usability.ÄȘlso enabling ECC cost me about 5% game performance so I turned it off.
#Nvidia gtx titan fp64 windows#
Newer windows 20h1, 20h2 should do a decent job of assigning the appropriate gpu for the task and you can override w10s gpu choice if you right click to display settings then scroll down to graphics settings and select your program or app to override. You also lose normal nvida control panel functionality with no attached display.īut I see you have an igpu so you could just plug your video out cable into your mobo and see what it would be like to run a headless 3090 in no time flat. These limitations are very hardware specific and results can vary a bunch even with different monitors. No tearing, no stutters, just that I have to deal with the limitations of all of the links in the chain. I'm running a tesla m40 through an intel igpu and, just for an example of the mysteries you will encounter, I'm running it at 1440p57. Click to expand.Neat to try, but many headaches.

0 notes
Text
An important thing to note however is that many tasks require extremely specialised GPU's that only have a passing resemblance to what would usually be considered a GPU, and if you don't have access to those specialised GPU's then you are much better off with CPU's.
Most general-use GPU's are optimized for fp32 arithmetic, because you don't need massive precision to do the calculations needed to display a raster image. AI and similar tasks usually stick to 32-bit floating point maths (or less, those dedicated AI processors often work in fp16 or even INT8), because you don't need very high precision floats to do AI, and so AI can be trained on a wide array of consumer and professional GPU's.
If you want to do, say, molecular dynamics simulations on a GPU, you usually can't get away with fp32. The precision is simply too low, and so you have to bump it up to fp64. The catch here is that almost every GPU takes a massive performance hit when doing fp64, usually at least 16Ă slower but it was 32Ă slower on RTX2000, and on the new RTX3000's it's actually 64Ă slower. Adding 64 bit arithmetic logic is expensive and unnecessary so these graphics-focused cards just don't have it, and faking 64-bit compute with 32-bit maths is slow. Like how you could do floating point maths on old 8080 PC's but it was slow as hell.
With that kind of handicap on fp64 performance, it's very often more cost-effective to just use CPU's unless you have good funding and your funders are making enough money to care about speed over price.
If you're interested in that kind of compute, which you'd use for weather simulations and molecular dynamics and fluids and so on, you need specialised compute GPU's with dedicated fp64 hardware. The Nvidia P100, V100, and A100 and AMD's Instinct MI50 and MI100 for example are all only 2Ă slower when doing fp64 vs fp32, and the old Kepler K40 GPU was 3Ă slower for fp64.
Feels like a stupid question but there any particular reason that graphics accelerator cards are used in so many seemingly unrelated applications? It seems like at some point if this stuff is so generally useful they would be in the CPU, or on the other hand maybe some sort of more generalized computing accessory should be created. (Was wondering about say a Machine Learning accelerator card)
31 notes
·
View notes
Photo

Now this is an interesting one for me. So I'm just going to say it right now. Here is the world's first '7nm' high-performance GPU, the Vega 20, made by AMD. This chip uses TSMC's 'N7' process, built on 7nm lithography (but not using Ultra Violet yet, that comes with N7+). This makes it probably the densest high-performance microprocessor silicon shipping at the time I wrote this entry. That's cool (probably not literally though, it gets hot). The chip contains approximately 13.2 billion transistors and is about 331mmÂČ in size. That actually makes it a pretty mid-sized chip as far as GPUs go, and significantly smaller than Vega 10 on GloFo 14nm: with a die size near 500mmÂČ, and Vega 20 has 700 million more transistors to boot. Hooray for a full node-shrink.
So what's in this chip? Well quite simply put, not a whole lot new. It's still the same GCN 5th generation architecture ('Vega') but shrunk down to 7nm and some relatively major changes to the Compute Units to allow for better mixed-precision operation, notable is the FP64 Double-Precision rate is now half that of FP32. That's a lot of Double Precision throughput. Around 7.5 Trillion 64-bit operations per second on the full silicon used in the Radeon Instinct Mi60.
More about the Radeon VII: This graphics card is not equipped with the full silicon. AMD has turned off (laser cut) four Compute Units (I covered these in previous entries). A full Vega 20 has the same top-level structure to Vega 10 so you get 64 CU arranged in 4 Shader/Compute engines with 16 each. Each SE also houses a quartet of Render Backends (as AMD call them) which can work with 4 pixels per clock. Vega 20 thus has 64 ROPs / 64 pixel/clock cycle. Radeon VII's processor has 15 enabled CU per SE, so you get 60 CU or 3840 ALU and 240 Texture units. That sits right between the RX Vega 56 and 64, in terms of core config. But this card is a lot faster than both of them.
So aside from the changes to allow higher double-precision, AMD also doubled the memory-interface width by adding two more HBM2 stacks (you obviously noticed looking at it, it has four). That widens the interface to 4096 bits (512b/clock, that's mental). Sharing the same memory width as the Fiji chip in Fury and Fury X. (also GP100 and GV100, from NVIDIA). But the Vega 20 ships with 2Gbps data rate as standard on all cards, so that's over a Terabyte per second of raw memory bandwidth. Your Vega bandwidth woes are a thing of the past. The chip can use 8-hi stacks (1GB per die, 8 dies per stack, 32GB per chip with four stacks) or 4-hi, as used on the Radeon VII. So this bad boy has 16GB of VRAM at 1TB/s all for your high-resolution gaming (and HD texture) needs.
The move to 7nm has allowed AMD to squeeze (at the expense of efficiency, nothing new here) a few hundred Megahertz out of the core frequency, driving the average operating core clock speed to around 1750-1800 MHz on the stock Radeon VII. This is a 200-300 MHz bump over Vega 64 and represents a major chunk of the performance uplift. Of course the more than doubling of the memory bandwidth helped a lot, too.
The rest of the architecture is pretty much the same as you find in the Vega 10 chip. However AMD did do some fairly major changes to the internal data-fabric ('Infinity Fabric') that pumps data around the chip, from the CU's to the memory controllers, etc. That's to support the immense bandwidth the quad-stack HBM2 provides. This also probably increases data-flow performance in the silicon and may account for a few percent higher performance per clock (CU normalised) vs the Vega 10 silicon. Overall the Radeon VII is about 30%~ faster than the Vega 64, this puts it firmly in the GTX 1080 Ti / RTX 2080 range, and is unfortunately priced like that too. But at least you get the 16GB of VRAM.
Efficiency at stock is pretty bad. Especially for a 7nm part. AMD has a long history of driving their GPUs through the metaphorical roof in terms of clock speed, in order to compete with Nvidia in raw performance and that didn't change with Radeon VII. It's actually pretty abysmal for a 7nm part, but at least it's fast enough to compete with the 2080, at the same price, only a couple months after that part launched. Radeon is still in the game. (the performance one). But you can lower the operating clock speed and voltage to put this GPU closer to its 'efficiency sweet spot' and you will be near Turing perf/watt, maybe even more, but at the expense of overall performance, obviously. So there's that.
My card doesn't overclock very well, in fact it barely overclocks at all. But I threw a liquid cooler on it and it runs 1800-1900 Mhz all day and is silent and cool running. Oh, I almost forgot to talk about the thermal issues.
7nm is dense. Very dense. Vega 20 has almost twice the number of transistors per square millimetre to any other GPU. That's a lot of thermal density. AMD has added 64 thermal sensors to this chip and the hottest one is reported as the 'Hot Spot' in the driver. This is problematic for Vega 20, as it is often 30*C+ higher than the GPU diode on the chip's surface. Radeon VII's fans are synced to the Hot Spot and as a result this card gets loud, very quickly. The cooler isn't really good enough to keep the chip cool enough to maintain that performance and be quiet at the same time. Vega 20 is crying for liquid cooling, so that's what I gave it.
0 notes
Text
A couple of days ago, Nvidia revealed its superfast RTX 3000 series which completely dominated all the GPUs right now present in the market. They are the fastest and most powerful graphics cards we could have until now. Even the slowest among them is faster than the higher-end cards in the last generation AKA RTX 2000 series.
Here we are talking about the RTX 3070 which replaces the RTX 2070 from the RTX 20 series of graphics cards and if you want to know what changes did occur from the 20 series GPU to this one, then keep reading.
Architecture
Perhaps the most significant change is the change of the architecture which play the most important role in changing the performance of a particular computer component. The first Ray-Tracing capable graphics cards we have are from 2018 which provided the unknown and infamous technique of projecting lights and shadows dynamically in real-time.
The 20 series RTX graphics cards used the Turing architecture which made it almost 20% faster than the older GTX 10 series graphics cards for the same price along with providing Ray-Tracing effects. However, the 20 series failed in providing good performance with Ray Tracing although they were beast in non-Ray-Traced games which are still the most common but the thing in which they had to excel was out of the game.
How RTX 3070 is faster through Ampere architecture
RTX 3070 uses the Ampere architecture which provides 2nd gen RT Cores and 3rd gen Tensor cores that are beneficial for bumping up the performance or fps numbers without any decrease in quality.
As the RTX 3070 features higher Tensor cores than 2070, itâs easy to accelerate the AI processes like Deep Learning Super Sampling(DLSS), AI Slow-Mo, AI Super-Rez and also matrix operations.
The Turing architecture on the RTX 2070 uses the 12nm process technology and the Ampere uses 7nm. The Ampere architecture comes with two new precisions: The Tensor Float (TF32) and Floating Point(FP64). While the TF32 works like FP32 which is available in the RTX 20 series cards, it speeds up the processes for AI by up to 20 times without any code change as said by Nvidia.
Specs
Nvidia never jumped so high in specs from one generation to other like it did right now. The RTX 3070 completely owns the RTX 2070 with specs that are doubled to make it compete the RTX 2080 Ti.
Take a look at the chart below for a brief comparison between the specs of two:-
[wpsm_comparison_table id=â38âł class=ââ]
While the memory bandwidth and speed are unconfirmed, they will be definitely the same or higher as mentioned in the table as per many sources. Cuda cores are just doubled and Tensor Cores are increased by 1.27 times which means better performance with DLSS AI Acceleration.
Cooler Design
#gallery-0-4 { margin: auto; } #gallery-0-4 .gallery-item { float: left; margin-top: 10px; text-align: center; width: 33%; } #gallery-0-4 img { border: 2px solid #cfcfcf; } #gallery-0-4 .gallery-caption { margin-left: 0; } /* see gallery_shortcode() in wp-includes/media.php */
While there are AIB models available for both the RTX 2070 and 3070, the Founders Edition is what we have to look at in order to compare the cooling efficiency of each cooler. RTX 2070 uses a 2 slot medium-sized heatsink with dual axial 13 blade fans on the same side.
The RTX 3070 features a completely different design for heat dissipation. While it also features dual fans but the heat is eliminated from the aluminium heatsinks on the opposite sides. The fans work both as intake and exhaust fans and unlike the traditional heatsinks like on the RTX 2070, the hot air isnât allowed to exit from all the sides.
Performance
Performance is what makes the RTX 3070 a compelling choice over 2070. While the specs are theoretical, you can actually calculate or determine a theoretical real-world performance. As Nvidia claims, the RTX 3070 is roughly 60% faster than the RTX 2070 and also beats it not competes with the RTX 2080 Ti which is a $1200 card from the Turing series.
There are some benchmarks done by Nvidia officially and the graph on the RTX 3070 page shows how much it is faster than the 2070 and 1070. While these benchmarks cannot be trusted 100%, the chances that these are true are higher and when the reviewers will get their samples, we can clearly determine the accuracy of Nvidiaâs claim.
Source: Nvidia.com
RTX 3070 vs RTX 2070- Whatâs the big change? A couple of days ago, Nvidia revealed its superfast RTX 3000 series which completely dominated all the GPUs right now present in the market.
0 notes
Text
Nvidia Unveils Its First Ampere-Based GPU, Raises Bar for Data Center AI
In lieu of the multi-day extravaganza that is normally Nvidiaâs flagship GTC in San Jose, the company has been rolling out a series of talks and announcements online. Even the keynote has gone virtual, with Jensenâs popular and traditionally rambling talk being shifted to YouTube. To be honest, itâs actually easier to cover keynotes from a livestream in an office anyway, although I do miss all the hands-on demos and socializing that goes with the in-person conference.
In any case, this yearâs event featured an impressive suite of announcements around Nividiaâs new Ampere architecture for both the data center and AI on the edge, beginning with the A100 Ampere-architecture GPU.
Nvidia A100: Worldâs Largest 7nm Chip Features 54 Billion Transistors
Nvidiaâs first Ampere-based GPU, its new A100 is also the worldâs largest and most complex 7nm chip, featuring a staggering 54 billion transistors. Nvidia claims performance gains of up to 20x over previous Volta models. The A100 isnât just for AI, as Nvidia believes it is an ideal GPGPU device for applications including data analytics, scientific computing, and cloud graphics. For lighter-weight tasks like inferencing, a single A100 can be partitioned in up to seven slices to run multiple loads in parallel. Conversely, NVLink allows multiple A100s to be tightly coupled.
All the top cloud vendors have said they plan to support the A100, including Google, Amazon, Microsoft, and Baidu. Microsoft is already planning to push the envelope of its Turing Natural Language Generation by moving to A100s for training.
Innovative TF32 Aims to Optimize AI Performance
Along with the A100, Nvidia is rolling out a new type of single-precision floating-point â TF32 â for the A100âs Tensor cores. It is a hybrid of FP16 and FP32 that aims to keep some of the performance benefits of moving to FP16 without losing as much precision. The A100âs new cores will also directly support FP64, making them increasingly useful for a variety of HPC applications. Along with a new data format, the A100 also supports sparse matrices, so that AI networks that contain many un-important nodes can be more efficiently represented.
Nvidia DGX A100: 5 PetaFLOPS in a Single Node
Along with the A100, Nvidia announced its newest data center computer, the DGX A100, a major upgrade to its current DGX models. The first DGX A100 is already in use at the US Department of Energyâs Argonne National Lab to help with COVID-19 research. Each DGX A100 features 8 A100 GPUs, providing 156 TFLOPS of FP64 performance and 320GB of GPU memory. Itâs priced starting at âonlyâ (their words) $199,000. Mellanox interconnects allow for multiple GPU deployments, but a single DGX A100 can also be partitioned in up to 56 instances to allow for running a number of smaller workloads.
In addition to its own DGX A100, Nvidia expects a number of its traditional partners, including Atos, Supermicro, and Dell, to build the A100 into their own servers. To assist in that effort, Nvidia is also selling the HGX A100 data center accelerator.
Nvidia HGX A100 Hyperscale Data Center Accelerator
The HGX A100 includes the underlying building blocks of the DGX A100 supercomputer in a form factor suitable for cloud deployment. Nvidia makes some very impressive claims for the price-performance and power efficiency gains that its cloud partners can expect from moving to the new architecture. Specifically, with todayâs DGX-1 Systems Nvidia says a typical cloud cluster includes 50 DGX-1 units for training, 600 CPUs for inference, costs $11 million, occupies 25 racks, and draws 630 kW of power. With Ampere and the DGX A100, Nvidia says only one kind of computer is needed, and a lot less of them: 5 DGX A100 units for both training and inference at a cost of $1 million, occupying 1 rack, and consuming only 28 kW of power.
DGX A100 SuperPOD
Of course, if youâre a hyperscale compute center, you can never have enough processor power. So Nvidia has created a SuperPOD from 140 DGX A100 systems, 170 InfiniBand switches, 280 TB/s network fabric (using 15km of optical cable), and 4PB of flash storage. All that hardware delivers over 700 petaflops of AI performance and was built by Nvidia in under three weeks to use for its own internal research. If you have the space and the money, Nvidia has released the reference architecture for its SuperPOD, so you can build your own. Joel and I think it sounds like the makings of a great DIY article. It should be able to run his Deep Space Nine upscaling project in about a minute.
Nvidia Expands Its SaturnV Supercomputer
Of course, Nvidia has also greatly expanded its SaturnV supercomputer to take advantage of Ampere. SaturnV was composed of 1800 DGX-1 Systems, but Nividia has now added 4 DGX A100 SuperPODs, bringing SaturnV to a total capacity of 4.6 exaflops. According to Nvidia, that makes it the fastest AI supercomputer in the world.
For AI on the Edge, Nvidiaâs new EGX A100 offers massive compute along with local and network security
Jetson EGX A100 Takes the A100 to the Edge
Ampere and the A100 arenât confined to the data center. Nvidia also announced a high-powered, purpose-built GPU for edge computing. The Jetson EGX A100 is built around an A100, but also includes Mellanox CX6 DX high-performance connectivity thatâs secured using a line speed crypto engine. The GPU also includes support for encrypted models to help protect an OEMâs intellectual property. Updates to Nvidiaâs Jetson-based toolkits for various industries (including Clara, Jarvis, Aerial, Isaac, and Metropolis) will help OEMs build robots, medical devices, and a variety of other high-end products using the EGX A100.
Now Read:
Hands On With Nvidiaâs New Jetson Xavier NX AI âRobot Brainâ
Nvidia May Be Prepping a Massive GPU With 7,936 CUDA Cores, 32GB HBM2
Leak: Intel is Planning a 400-500W Top-End GPU to Challenge AMD, Nvidia
from ExtremeTechExtremeTech https://www.extremetech.com/extreme/310500-nvidia-raises-the-bar-for-data-center-ai from Blogger http://componentplanet.blogspot.com/2020/05/nvidia-unveils-its-first-ampere-based.html
0 notes
Text
New Radeon Pro VII Wows on Price and Double Precision
Earlier this morning, AMD revealed its new Radeon Pro VII graphics card, its latest workstation-class competitor to Nvidiaâs Quadro line of GPUs. As its name suggests, this is a professional level update on last yearâs Radeon VII GPU, incorporating the same Vega 20 GPU but almost doubling the base Radeon VIIâs double precision performance.
When AMD announced the Radeon VII at its keynote last year, it was the worldâs first 7nm gaming graphics card, using Vega 20 to compete with Nvidiaâs RTX 2080. Now, AMD is applying Vega 20 to its workstation cards, producing a pro-level successor to the Radeon VII that takes almost the same specs and ups the double precision performance to 6.5 TFLOPs, offering support for mixed graphics/compute tasks that almost matches Nvidiaâs much more expensive Quadro GV100.
While this comes at the cost of a slightly lower boost clock and slightly lessened single precision performance, making it not quite as powerful of a gaming machine as its non pro predecessor, this will make it a boon to 3D modelers and financial analysts, who frequently run mixed graphics/compute software.
The AMD Infinity Fabric external link is also a new addition to the Radeon Pro VII, migrating over from the Radeon Instinct MI50/MI60. The purpose here is to make multi-GPU performance more efficient, enabling a total of 168GB/sex bandwidth between two connected GPUs.
All these features also make it Vega 10 Radeon Pro WX 9100, which runs on Vega 10, as well as the Radeon Pro W5700, assuming memory clock speed isnât a priority.
Of course, the key feature for the Radeon Pro VII compared to its Nvidia Quadro counterparts is price. Launching for $1,800, itâs primed to severely undercut Nvidiaâs double precision king, the Quadro GV100, while also remaining competitive with the Quadro RTX 5000 and 6000. While its feature set is unique- the GV100 is only really a fair comparison when it comes to FP64 support- this makes it a strong buy for value, assuming it fits your needs.
0 notes
Text
NVidia Titan V Review 2018 â Buyerâs Guide
Although nvidia officially unveiled its volta-primarily based gv100 gpu over seven months ago, and an unwitting intern might also have leaked snap shots of the cardboard weâre going to expose you right here today rapidly thereafter, the nvidia titan v presenting the gv100 gpu started delivery just this past week. the nvidia titan v targets a totally particular target market and is designed for expert and academic deep gaining knowledge of programs, which in part explains its lofty $3,000 price tag.
in contrast to nvidia's preceding-gen consumer flagship, the titan xp, the titan v is honestly now not designed for game enthusiasts. but because it capabilities nvidiaâs modern-day gpu architecture and doubtlessly foreshadows subsequent-yearâs customer-centered voltas, we idea it'd be exciting to take the titan v for a spin with various applications and video games, to look just what the cardboard may want to do. as youâll witness, the titan v is a beast, specifically in relation to scientific computing applications.at its default clocks, the titan v offers a height texture fillrate of 384 gigatexels/s, which is most effective slightly better than a titan xp. the 12gb of hbm2 reminiscence on-board the gv100 is linked to the gpu through a 3072-bit interface and offers up 652.8 gb/s of peak bandwidth, which is about 100gb/s more than a titan xp. different functions of the gv100 consist of 5,120 single-precision cuda cores, 2,560 double-precision fp64 cores, and 640 tensor cores, that can offer massive overall performance enhancements in deep mastering workloads, to the track of up to 110tflops. the cores are arranged in 6 gpcs, with 80 sms. there also are 320 texture gadgets on board, and ninety six rops, which is proper in-line with the gp102.store for the gold tint at the faceted factors of its fan shroud, the titan v has a comparable aesthetic to nvidia's titan xp and geforce gtx 1080 ti founder's edition playing cards. it has a faceted fan-shroud with black and gold elements and a see-through window at the middle which famous and array of skinny-fins under. the backside of the cardboard is embellished with a inflexible, black back-plate, although it isn't modular just like the ones at the titan xp or 1080 ti, which permit for more area among adjoining playing cards used in sli mode. why not? due to the fact the titan v doesnât have sli connectors, so the way it behaves in a multi-gpu setup continues to be unknown. we've a query out to nvidia inquiring for explanation there.
0 notes
Text
Shock and Awe in Utah: NVIDIA CEO Springs Special Titan V GPUs on Elite AI Researchers
Some come to Utah to ascend mountain peaks, others to ski down them.
At the Computer Vision and Pattern Recognition conference in Salt Lake City Wednesday, NVIDIAâs Jensen Huang told top AI researchers he wanted to help them achieve a very different kind of peak performance â before unveiling a big surprise.
âThe number of problems you guys are able to solve as a result of deep learning is truly amazing,â Huang said, addressing more than 500 guests at NVIDIAâs âbest of Utahâ themed CVPR bash at the Grand American Hotel. âWeâve dedicated our company to create a computing platform to advance your work. Our goal is to enable you to do amazing research.â
Then he sprung his first surprise on the crowd.
Supporting Todayâs Pioneers of AI
The authors of the CVPR accepted paper âWhat Do Deep Networks Like to Seeâ â submitted by Sebastian Palacio of DFKI and team â were among the twelve groups selected to receive an NVIDIA Pioneer Award.
Huang called up 12 teams of researchers and presented each with an NVIDIA Pioneer Awards.
The awards went to those whoâve used NVIDIAâs AI platform to support great work featured in papers accepted by CVPR and other leading academic conferences.
Wednesday nightâs award recipients were an elite group, representing some of the leading academic institutions that participate in the global NVIDIA AI Labs (NVAIL) program.
Honorees included researchers from the Chinese Academy of Science, DFKI, Peking University, Stanford University, Tsinghua University, University of Toronto, University of Tokyo and University of Washington.
University of Toronto researchers Makarand Tapaswi and Sanja Fidler were one of 12 research groups to receive an NVIDIA Pioneer Award from NVIDIA CEO Jensen Huang at the NVIDIA CVPR party Wednesday night.
But the surprises didnât stop there.
Titans for the Titans of AI
Twenty guests selected at random joined Huang in the the hotelâs center courtyard. There he presented them with signed, limited edition NVIDIA Titan V CEO Edition GPUs, featuring our groundbreaking NVIDIA Volta Tensor Cores and loaded with 32 gigabytes of memory.
Fabio Ramos (center) of the University of Sydney was one of twenty lucky recipients of the NVIDIA Titan V CEO Edition handed out Wednesday night at NVIDIAâs CVPR party. Heâs joined by his colleagues Rafael Possas and Sheila Pinto Caceres.
Among the lucky ones was AI researcher Fabio Ramos, from the University of Sydney who is doing groundbreaking work in the field of robotics.
âMy work is focused on helping robots make decisions autonomously. I hope to use this to help advance my work to help robots take care of elderly people,â he said, as other guests noshed on chicken sandwiches prepared by Food Network star Viet Pham.
While the food and drinks â which included green jello, a nod to Utah tradition â had guests buzzing, researchers were even more eager to dig into their new GPUs.
âIâm eager to start using my GPU to support my work in deep driving,â said Firas Lethaus who is a senior engineer at the development division of automated driving and head of the machine learning competence center at AUDI AG. âWith this new tool, Iâll be able to further examine image data so that self-driving learning systems can better separate relevant from non-relevant information.â
Titans of AI: NVIDIA Jensen Huang is joined by winners of the NVIDIA Titan V CEO Edition giveaway at CVPR.
The limited edition GPU is built on top of NVIDIAâs breakthrough Volta platform. It features our revolutionary Tensor Core architecture to enable multi-precision computing. It can crank through deep learning matrix operations at 125 teraflops at FP16 precision. Or it can blast through FP64 and FP32 operations when thereâs a need for greater range, precision or numerical stability.
With 20 of their peers equipped with some of our most powerful GPUs to accelerate their work, these wonât be the last to be so honored.
âThereâs all kinds of research being done here. As someone who benefits from your work, as a person who is going to enjoy the incredible research you guys do â solving some of the worldâs grand challenges â and to be able to witness artificial intelligence happen in my lifetime, I want to thank all of you guys for that,â Huang said. âYou guys bring me so much joy.â
The post Shock and Awe in Utah: NVIDIA CEO Springs Special Titan V GPUs on Elite AI Researchers appeared first on The Official NVIDIA Blog.
Shock and Awe in Utah: NVIDIA CEO Springs Special Titan V GPUs on Elite AI Researchers published first on https://supergalaxyrom.tumblr.com
0 notes
Text
Nvidia builds âfastest single computer humanity has ever createdâ
New Post has been published on https://www.etechwire.com/nvidia-builds-fastest-single-computer-humanity-has-ever-created/
Nvidia builds âfastest single computer humanity has ever createdâ
Nvidia has just announced its HGX-2 cloud server platform, which it claims powers âthe fastest single computer humanity has ever createdâ.
It combines 16 Tesla V100 graphics cards, which work together to create a giant virtual GPU with half a terabyte of GPU memory and two petaflops of compute power. This is achieved using Nvidia NVSwitch interconnect fabric technology, which links the GPUs together to work as a single GPU.
The announcement was made at Nvidiaâs GTC (GPU Technology Conference) event in Taiwan, which is a bit of an appetizer before the main course of Computex 2018 arrives next week.
While the HGX-2 certainly has some mind-blowing specifications, it wonât be used in standard computers. Instead, it will be capable of high-precision calculations using FP64 and FP32 for scientific computing and simulations, while enabling FP16 and Int8 for AI training.
Teacherâs pet
Jensen Huang, founder and chief executive officer of Nvidia, announced at GTC that âThe world of computing has changed [âŠ] CPU scaling has slowed at a time when computing demand is skyrocketing. NVIDIAâs HGX-2 with Tensor Core GPUs gives the industry a powerful, versatile computing platform that fuses HPC and AI to solve the worldâs grand challenges.â
According to Nvidia, the HGX-2 has achieved record AI training speeds of 15,500 images per second on the ResNet-50 training benchmark, and is powerful enough to replace up to 300 CPU-only servers.
At Computex 2017, Nvidia announced the HGX-1 which became pretty popular, being used by companies that rely on massive datacenters such as Facebook and Microsoft.
Nvidia has high hopes for HGX-2 as well, with some major businesses, including Lenovo, QCT, Supermicro, Foxconn and Wiwynn, announcing plans to launch HGX-2 systems this year.
According to Paul Ju, vice president and general manager of Lenovo DCG , âNVIDIAâs HGX-2 ups the ante with a design capable of delivering two petaflops of performance for AI and HPC-intensive workloads. With the HGX-2 server building block, weâll be able to quickly develop new systems that can meet the growing needs of our customers who demand the highest performance at scale.â
GPU stats
The HGX-2 is powered by the Nvidia Tesla V100 GPU, which comes equipped with 32GB of high-bandwidth memory to deliver 125 teraflops of deep learning performance. Combining 16 of those GPUs together is going to produce some excellent results.
âEvery one of the GPUs can talk to every one of the GPUs simultaneously at a bandwidth of 300 GB/s, 10 times PCI Express,â Huang said, âso everyone can talk to each other all at the same time.â
(Image: © Nvidia)
Nvidia also showed off the Nvidia DGX-2, which is the first system built using the HGX-2 server platform, and comes with 2 petaflops of computing power and 512GB of HBM2 memory.
According to Huang, âthis is the fastest single computer humanity has ever createdâ. Pretty exciting stuff.
0 notes
Text
 NVIDIA ha anunciado durante el GDC 2018 la nueva GPU profesional Quadro GV100, la primera tarjeta PCI Express en incorporar 32GB de memoria HBM2 y en utilizar por primera vez la GV100 que si encontramos en soluciones como DGX-1. Esta nueva GPU tendrå un coste de 9.000 dólares, triplicando de esta manera a las anteriormente lanzadas Titan V.
El GV100 estĂĄ compuesto de seis GPC (Graphics Processing Clusters) con 84 Volta Streaming Multiprocessor Units y 42 TPCs que incluyen dos SM cada uno. Cada SM cuenta con 64 nĂșcleos CUDA que suman un total de 5120 nĂșcleos que pueden utilizarse en cĂĄlculos FP32 y INT32, mientras que tambiĂ©n cuenta con 2560 nĂșcleos capaces de ejecutar cĂĄlculos FP64 (doble precisiĂłn). AdemĂĄs la tarjeta cuenta con 640 Tensor Cores y 320 Texture Units.
Sobre el papel la nueva Quadro GV100 consigue 7.4 TeraFlops de potencia en doble precisión, 14.8 TeraFlops en Single Precision y 29.6 TeraFlops en media precisión. En el lado de la IA se consiguen 118.5 DLOPs, que suponen un ligero aumento frente a las Tesla V100 gracias a las frecuencias de funcionamiento mejoradas.
La memoria se ha configurado con ocho controladoras de 512 bit que componen un bus de 4096 bit para los 32GB de memoria apilada HBM2, a los que se les ha sumado 768KB de memoria L2 que suman un total de 6MB de L2 para el total del chip. Con una frecuencia de 850Mhz para la memoria, se ha conseguido un ancho de banda de 870 GB/s, 150GB/s mås que la Quadro Pascal GP100.
La tarjeta llegarĂĄ al mercado con el habitual modelo de referencia con blower y un PCB que incorpora cuatro salidas Display Port 1.4. En cuanto a consumo, el TDP suma 250W con dos conectores PCIe de 6 y 8 pines respectivamente.
 NVIDIA presenta la nueva Quadro GV 100, la primera tarjeta gråfica PCIe con 32GB HBM2 NVIDIA ha anunciado durante el GDC 2018 la nueva GPU profesional Quadro GV100, la primera tarjeta PCI Express en incorporar 32GB de memoria HBM2 y en utilizar por primera vez la GV100 que si encontramos en soluciones como DGX-1.
0 notes