#Food app scraping
Explore tagged Tumblr posts
Text
Competitor Price Monitoring Services - Food Scraping Services
Competitor Price Monitoring Strategies
Price Optimization
If you want your restaurant to stay competitive, it’s crucial to analyze your competitors’ average menu prices. Foodspark offers a Competitor Price Monitoring service to help you with this task. By examining data from other restaurants and trends in menu prices, we can determine the best price for your menu. That will give you an edge in a constantly evolving industry and help you attract more customers, ultimately increasing profits.
Market Insights
Our restaurant data analytics can help you stay ahead by providing valuable insights into your competitors’ pricing trends. By collecting and analyzing data, we can give you a deep understanding of customer preferences, emerging trends, and regional variations in menu pricing. With this knowledge, you can make informed decisions and cater to evolving consumer tastes to stay ahead.
Competitive Advantage
To stay ahead in the restaurant industry, you must monitor your competitors’ charges and adjust your prices accordingly. Our solution can help you by monitoring your competitors’ pricing strategies and allowing you to adjust your expenses in real-time. That will help you find opportunities to offer special deals or menu items to make you stand out and attract more customers.
Price Gap Tracking
Knowing how your menu prices compare to your competitors is essential to improve your restaurant’s profitability. That is called price gap tracking. Using our tracking system, you can quickly identify the price differences between restaurant and your competitors for the same or similar menu items. This information can help you find opportunities to increase your prices while maintaining quality or offering lower costs. Our system allows you to keep a close eye on price gaps in your industry and identify areas where your expenses are below or above the average menu prices. By adjusting your pricing strategy accordingly, you can capture more market share and increase your profits.
Menu Mapping and SKU
Use our menu and SKU mapping features to guarantee that your products meet customer expectations. Find out which items are popular and which ones may need some changes. Stay adaptable and responsive to shifting preferences to keep your menu attractive and competitive.
Price Positioning
It’s essential to consider your target audience and desired brand image to effectively position your restaurant’s prices within the market. Competitor data can help you strategically set your prices as budget-friendly, mid-range, or premium. Foodspark Competitor Price Monitoring provides data-driven insights to optimize your pricing within your market segment. That helps you stay competitive while maximizing revenue and profit margins.
Competitor Price Index (CPI)
The Competitor Price Index (CPI) measures how your restaurant’s prices compare to competitors. We calculate CPI for you by averaging the prices of similar menu items across multiple competitors. If your CPI is above 100, your prices are higher than your competitors. If it’s below 100, your prices are lower.
Benefits of Competitor Price Monitoring Services
Price Optimization
By continuous monitoring your competitor’s prices, you can adjust your own pricing policies, to remain competitive while maximizing your profit margins.
Dynamic Pricing
Real-time data on competitor’s prices enable to implement dynamic pricing strategies, allowing you to adjust your prices based on market demand and competitive conditions.
Market Positioning
Understanding how your prices compare to those of your competitors helps you position your brand effectively within the market.
Customer Insights
Analyzing customer pricing data can reveal customer behavior and preferences, allowing you to tailor your pricing and marketing strategies accordingly.
Brand Reputation Management
Consistently competitive pricing can enhance your brand’s reputation and make your product more appealing to customers.
Content Source: https://www.foodspark.io/competitor-price-monitoring/
#web scraping services#restaurantdataextraction#Competitor Price Monitoring#Mobile-app Specific Scraping#Real-Time API#Region - wise Restaurant Listings#Services#Food Aggregator#Food Data Scraping#Real-time Data API#Price Monitoring#Food App Scraping#Food Menu Data
0 notes
Text
0 notes
Text
Online food delivery apps scraping
3i Data Scraping provides Food ordering data extractor to scrape online food delivery apps like DoorDash, Postmates, goPuff, Seamless, Zomato, Ubereats, Grubhub, Swiggy, etc.

#food delivery app scraping#Extract Food Ordering Apps Data#web scrape food delivery#food delivery app data extraction#Extract food menu details#competitive price intelligence#food ordering data extractor
3 notes
·
View notes
Text
How to Do Restaurant Data Collection Using Food Intelligence?
At Actowiz Solutions, were your window into the bustling US and UK restaurant industry. Dive into Actowiz Solutions Blog and Harness the Power of Real-Time Data.d practical meal-serving apps.
0 notes
Text
Food Delivery App Scraping Services | Extract Restaurant Menu Data
Elevate your food business with our Food Delivery App Scraping Services. We can extract restaurant menu data from the USA, UK, UAE, Canada, China, India, and Spain.
know more: https://www.mobileappscraping.com/food-delivery-app-scraping-services.php
#Food Delivery App Scraping Services#extracting data from food delivery app#Food Delivery Mobile App Data Scraping#Extract Restaurant Menu Data
0 notes
Text
Exploring the Uber Eats API: A Definitive Guide to Integration and Functionality
In this blog, we delve into the various types of data the Uber Eats API offers and demonstrate how they can be ingeniously harnessed to craft engaging and practical meal-serving apps.
#Uber Eats Data Scraping API#Scrape Uber Eats Data API#Extract Uber Eats Data#Scrape Food Delivery App Data#Food Delivery App Data Scraping
0 notes
Text
How to Scrape Data from Food Delivery App Burger King – Spain
In this blog post, we will guide you through the process of scraping data from Burger Kings food delivery app in Spain.
Know more >>
#Food Delivery App Data Scraper#Scrape Food Delivery App#Food Delivery App Data Extraction#Food Delivery App Data Scraping
0 notes
Text
#Food Delivery Scraping API Services#scrape data from food Delivery apps#Food Scraping API#Web data scraping API
0 notes
Text
How To Scrape Restaurants Reviews From Food Delivery App Like Talabat, Deliveroo, And Zomato

What is a Food Delivery App?
Online food delivery apps are a new way of food distribution. You can get numerous food delivery apps in the marketplace that works like a common platform between food consumers and restaurants. A few restaurant owners make their food ordering apps to help customers order food rapidly and give fresh food. Some leading food delivery applications include Deliveroo, Talabat, and Zomato.
Some Important Food Delivery Growth Statistics
https://www.fooddatascrape.com/assets/img/blog/how-to-scrap-restaurants-reviews-from-food-delivery-apps-like-talabat-deliveroo-and-zomato/Some-Important-Food-Delivery-Growth-Statistics.jpg
Revenue in the food delivery segment touched US$9,207m in 2020. The projected income will show annual growth of 9.5% (CAGR 2020-2024), with market sizing of US$13,233m within 2024! The most significant segment of this market is Restaurant-to-Consumer Delivery, which is getting a market volume of US$4,934m in 2020.
Food Data Scrape offers the finest food delivery app scraping services to extract food delivery apps, including Deliveroo, Talabat, and Zomato with on-time delivery and accuracy. Our food data extraction services assist in getting information like product prices, news, quotations, features, etc. We help you scrape precise data and provide all the required business details.
About Deliveroo
Deliveroo is a well-known British online food delivery company incepted in the year 2013 in London, England. Will Shu and Greg Orlowski founded it. It operates in nearly 200 cities, including Belgium, France, the UK, Italy, Ireland, Singapore, UAE, and Hong Kong. In 2022, the company launched an advertising platform to allow the business to promote products across its app. Deliveroo operates with large chain restaurants across the UK and thousands of independent restaurants.
About Talabat
Talabat is an online food ordering business founded in Kuwait in 2004. This company has been a subordinate of Delivery Hero since 2016 and has become the well-known online food-ordering company in the Middle East. Today, Talabat delivers hundreds of millions of food orders and other products annually across nine regional countries. Their food delivery business works with over 27,000 brands and nearly 50,000 branches.
About Zomato
Zomato is a popular Indian multicultural restaurant assemblage and food delivery company established in 2008 by Deepinder Goyal & Pankaj Chaddah. The company provides menus, information, food delivery options, and user reviews of the restaurants from several partnering restaurants in several Indian cities.
In this blog, we will understand how to scrape restaurant reviews from food delivery apps like Talabat, Deliveroo, and Zomato.
List of Data Fields

At Food Data Scrape, we extract the given data fields to scrape restaurants reviews data from apps like Talabat, Deliveroo, and Zomato:
Restaurant Name
Address
City
Location
Phone Number
Website URL
Image
Number of Reviews
Amenities
Features
Discount Offers
All food delivery apps like Talabat, Deliveroo, and Zomato comprise innumerable information on restaurants, menus, food delivery options, payment options, and more. Using Talabat, Deliveroo, and Zomato restaurant data extraction, you can easily collect menus, locations, reviews, ratings, and more data.
With Food Data Scrape, it’s easy to get a fast turnaround time, as we know you depend on us for Deliveroo restaurant data scraping.
Generally, web scraper break down when targeted websites make changes in the structure or designs, so you need a quick support team that can immediately take action. With us you will get immediate support.
We provide a well-organized Zomato food delivery data scraping service with different customizations. You may need to cope with scraped data and various delivery procedures in other data formats. So, our Talabat restaurant data extraction services can satisfy all the requirements.
Maintenance is a vital portion of any web extraction. This is essential because the web is highly dynamic. All the scraping setups that work today might not work if any targeted apps make any changes. So, Food Data Scrape is the most suitable service provider to scrape restaurants reviews data.
Contact us for all your restaurant review data scraping service requirements. We also provide the best Food Data Scraping and Mobile App Scraping requirements.
#Scrape Restaurants Reviews From Food Delivery App#Deliveroo restaurant data scraping#Zomato food delivery data scraping#Talabat restaurant data extraction services
0 notes
Text
Gig apps trap reverse centaurs in Skinner boxes
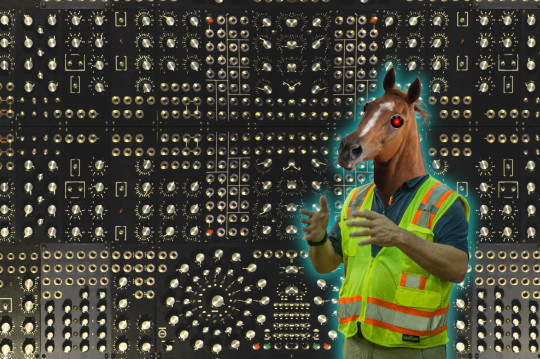
Enshittification is the process by which digital platforms devour themselves: first they dangle goodies in front of end users. Once users are locked in, the goodies are taken away and dangled before business customers who supply goods to the users. Once those business customers are stuck on the platform, the goodies are clawed away and showered on the platform’s shareholders:
https://pluralistic.net/2023/01/21/potemkin-ai/#hey-guys
If you’d like an essay-formatted version of this post to read or share, here’s a link to it on pluralistic.net, my surveillance-free, ad-free, tracker-free blog:
https://pluralistic.net/2023/04/12/algorithmic-wage-discrimination/#fishers-of-men
Enshittification isn’t just another way of saying “fraud” or “price gouging” or “wage theft.” Enshittification is intrinsically digital, because moving all those goodies around requires the flexibility that only comes with a digital businesses. Jeff Bezos, grocer, can’t rapidly change the price of eggs at Whole Foods without an army of kids with pricing guns on roller-skates. Jeff Bezos, grocer, can change the price of eggs on Amazon Fresh just by twiddling a knob on the service’s back-end.
Twiddling is the key to enshittification: rapidly adjusting prices, conditions and offers. As with any shell game, the quickness of the hand deceives the eye. Tech monopolists aren’t smarter than the Gilded Age sociopaths who monopolized rail or coal — they use the same tricks as those monsters of history, but they do them faster and with computers:
https://doctorow.medium.com/twiddler-1b5c9690cce6
If Rockefeller wanted to crush a freight company, he couldn’t just click a mouse and lay down a pipeline that ran on the same route, and then click another mouse to make it go away when he was done. When Bezos wants to bankrupt Diapers.com — a company that refused to sell itself to Amazon — he just moved a slider so that diapers on Amazon were being sold below cost. Amazon lost $100m over three months, diapers.com went bankrupt, and every investor learned that competing with Amazon was a losing bet:
https://slate.com/technology/2013/10/amazon-book-how-jeff-bezos-went-thermonuclear-on-diapers-com.html
That’s the power of twiddling — but twiddling cuts both ways. The same flexibility that digital businesses enjoy is hypothetically available to workers and users. The airlines pioneered twiddling ticket prices, and that naturally gave rise to countertwiddling, in the form of comparison shopping sites that scraped the airlines’ sites to predict when tickets would be cheapest:
https://pluralistic.net/2023/02/27/knob-jockeys/#bros-be-twiddlin
The airlines — like all abusive businesses — refused to tolerate this. They were allowed to touch their knobs as much as they wanted — indeed, they couldn’t stop touching those knobs — but when we tried to twiddle back, that was “felony contempt of business model,” and the airlines sued:
https://www.cnbc.com/2014/12/30/airline-sues-man-for-founding-a-cheap-flights-website.html
And sued:
https://www.nytimes.com/2018/01/06/business/southwest-airlines-lawsuit-prices.html
Platforms don’t just hate it when end-users twiddle back — if anything they are even more aggressive when their business-users dare to twiddle. Take Para, an app that Doordash drivers used to get a peek at the wages offered for jobs before they accepted them — something that Doordash hid from its workers. Doordash ruthlessly attacked Para, saying that by letting drivers know how much they’d earn before they did the work, Para was violating the law:
https://www.eff.org/deeplinks/2021/08/tech-rights-are-workers-rights-doordash-edition
Which law? Well, take your pick. The modern meaning of “IP” is “any law that lets me use the law to control my competitors, competition or customers.” Platforms use a mix of anticircumvention law, patent, copyright, contract, cybersecurity and other legal systems to weave together a thicket of rules that allow them to shut down rivals for their Felony Contempt of Business Model:
https://locusmag.com/2020/09/cory-doctorow-ip/
Enshittification relies on unlimited twiddling (by platforms), and a general prohibition on countertwiddling (by platform users). Enshittification is a form of fishing, in which bait is dangled before different groups of users and then nimbly withdrawn when they lunge for it. Twiddling puts the suppleness into the enshittifier’s fishing-rod, and a ban on countertwiddling weighs down platform users so they’re always a bit too slow to catch the bait.
Nowhere do we see twiddling’s impact more than in the “gig economy,” where workers are misclassified as independent contractors and put to work for an app that scripts their every move to the finest degree. When an app is your boss, you work for an employer who docks your pay for violating rules that you aren’t allowed to know — and where your attempts to learn those rules are constantly frustrated by the endless back-end twiddling that changes the rules faster than you can learn them.
As with every question of technology, the issue isn’t twiddling per se — it’s who does the twiddling and who gets twiddled. A worker armed with digital tools can play gig work employers off each other and force them to bid up the price of their labor; they can form co-ops with other workers that auto-refuse jobs that don’t pay enough, and use digital tools to organize to shift power from bosses to workers:
https://pluralistic.net/2022/12/02/not-what-it-does/#who-it-does-it-to
Take “reverse centaurs.” In AI research, a “centaur” is a human assisted by a machine that does more than either could do on their own. For example, a chess master and a chess program can play a better game together than either could play separately. A reverse centaur is a machine assisted by a human, where the machine is in charge and the human is a meat-puppet.
Think of Amazon warehouse workers wearing haptic location-aware wristbands that buzz at them continuously dictating where their hands must be; or Amazon drivers whose eye-movements are continuously tracked in order to penalize drivers who look in the “wrong” direction:
https://pluralistic.net/2021/02/17/reverse-centaur/#reverse-centaur
The difference between a centaur and a reverse centaur is the difference between a machine that makes your life better and a machine that makes your life worse so that your boss gets richer. Reverse centaurism is the 21st Century’s answer to Taylorism, the pseudoscience that saw white-coated “experts” subject workers to humiliating choreography down to the smallest movement of your fingertip:
https://pluralistic.net/2022/08/21/great-taylors-ghost/#solidarity-or-bust
While reverse centaurism was born in warehouses and other company-owned facilities, gig work let it make the leap into workers’ homes and cars. The 21st century has seen a return to the cottage industry — a form of production that once saw workers labor far from their bosses and thus beyond their control — but shriven of the autonomy and dignity that working from home once afforded:
https://doctorow.medium.com/gig-work-is-the-opposite-of-steampunk-463e2730ef0d
The rise and rise of bossware — which allows for remote surveillance of workers in their homes and cars — has turned “work from home” into “live at work.” Reverse centaurs can now be chickenized — a term from labor economics that describes how poultry farmers, who sell their birds to one of three vast poultry processors who have divided up the country like the Pope dividing up the “New World,” are uniquely exploited:
https://onezero.medium.com/revenge-of-the-chickenized-reverse-centaurs-b2e8d5cda826
A chickenized reverse centaur has it rough: they must pay for the machines they use to make money for their bosses, they must obey the orders of the app that controls their work, and they are denied any of the protections that a traditional worker might enjoy, even as they are prohibited from deploying digital self-help measures that let them twiddle back to bargain for a better wage.
All of this sets the stage for a phenomenon called algorithmic wage discrimination, in which two workers doing the same job under the same conditions will see radically different payouts for that work. These payouts are continuously tweaked in the background by an algorithm that tries to predict the minimum sum a worker will accept to remain available without payment, to ensure sufficient workers to pick up jobs as they arise.
This phenomenon — and proposed policy and labor solutions to it — is expertly analyzed in “On Algorithmic Wage Discrimination,” a superb paper by UC Law San Franciscos Veena Dubal:
https://papers.ssrn.com/sol3/papers.cfm?abstract_id=4331080
Dubal uses empirical data and enthnographic accounts from Uber drivers and other gig workers to explain how endless, self-directed twiddling allows gig companies pay workers less and pay themselves more. As @[email protected] explains in his LA Times article on Dubal’s research, the goal of the payment algorithm is to guess how often a given driver needs to receive fair compensation in order to keep them driving when the payments are unfair:
https://www.latimes.com/business/technology/story/2023-04-11/algorithmic-wage-discrimination
The algorithm combines nonconsensual dossiers compiled on individual drivers with population-scale data to seek an equilibrium between keeping drivers waiting, unpaid, for a job; and how much a driver needs to be paid for an individual job, in order to keep that driver from clocking out and doing something else. @ Here’s how that works. Sergio Avedian, a writer for The Rideshare Guy, ran an experiment with two brothers who both drove for Uber; one drove a Tesla and drove intermittently, the other brother rented a hybrid sedan and drove frequently. Sitting side-by-side with the brothers, Avedian showed how the brother with the Tesla was offered more for every trip:
https://www.youtube.com/watch?v=UADTiL3S67I
Uber wants to lure intermittent drivers into becoming frequent drivers. Uber doesn’t pay for an oversupply of drivers, because it only pays drivers when they have a passenger in the car. Having drivers on call — but idle — is a way for Uber to shift the cost of maintaining a capacity cushion to its workers.
What’s more, what Uber charges customers is not based on how much it pays its workers. As Uber’s head of product explained: Uber uses “machine-learning techniques to estimate how much groups of customers are willing to shell out for a ride. Uber calculates riders’ propensity for paying a higher price for a particular route at a certain time of day. For instance, someone traveling from a wealthy neighborhood to another tony spot might be asked to pay more than another person heading to a poorer part of town, even if demand, traffic and distance are the same.”
https://qz.com/990131/uber-is-practicing-price-discrimination-economists-say-that-might-not-be-a-bad-thing/
Uber has historically described its business a pure supply-and-demand matching system, where a rush of demand for rides triggers surge pricing, which lures out drivers, which takes care of the demand. That’s not how it works today, and it’s unclear if it ever worked that way. Today, a driver who consults the rider version of the Uber app before accepting a job — to compare how much the rider is paying to how much they stand to earn — is booted off the app and denied further journeys.
Surging, instead, has become just another way to twiddle drivers. One of Dubal’s subjects, Derrick, describes how Uber uses fake surges to lure drivers to airports: “You go to the airport, once the lot get kind of full, then the surge go away.” Other drivers describe how they use groupchats to call out fake surges: “I’m in the Marina. It’s dead. Fake surge.”
That’s pure twiddling. Twiddling turns gamification into gamblification, where your labor buys you a spin on a roulette wheel in a rigged casino. As a driver called Melissa, who had doubled down on her availability to earn a $100 bonus awarded for clocking a certain number of rides, told Dubal, “When you get close to the bonus, the rides start trickling in more slowly…. And it makes sense. It’s really the type of shit that they can do when it’s okay to have a surplus labor force that is just sitting there that they don’t have to pay for.”
Wherever you find reverse-centaurs, you get this kind of gamblification, where the rules are twiddled continuously to make sure that the house always wins. As a contract driver Amazon reverse centaur told Lauren Gurley for Motherboard, “Amazon uses these cameras allegedly to make sure they have a safer driving workforce, but they’re actually using them not to pay delivery companies”:
https://www.vice.com/en/article/88npjv/amazons-ai-cameras-are-punishing-drivers-for-mistakes-they-didnt-make
Algorithmic wage discrimination is the robot overlord of our nightmares: its job is to relentlessly quest for vulnerabilities and exploit them. Drivers divide themselves into “ants” (drivers who take every job) and “pickers” (drivers who cherry-pick high-paying jobs). The algorithm’s job is ensuring that pickers get the plum assignments, not the ants, in the hopes of converting those pickers to app-dependent ants.
In my work on enshittification, I call this the “giant teddy bear” gambit. At every county fair, you’ll always spot some poor jerk carrying around a giant teddy-bear they “won” on the midway. But they didn’t win it — not by getting three balls in the peach-basket. Rather, the carny running the rigged game either chose not to operate the “scissor” that kicks balls out of the basket. Or, if the game is “honest” (that is, merely impossible to win, rather than gimmicked), the operator will make a too-good-to-refuse offer: “Get one ball in and I’ll give you this keychain. Win two keychains and I’ll let you trade them for this giant teddy bear.”
Carnies aren’t in the business of giving away giant teddy bears — rather, the gambit is an investment. Giving a mark a giant teddy bear to carry around the midway all day acts as a convincer, luring other marks to try to land three balls in the basket and win their own teddy bear.
In the same way, platforms like Uber distribute giant teddy bears to pickers, as a way of keeping the ants scurrying from job to job, and as a way of convincing the pickers to give up whatever work allows them to discriminate among Uber’s offers and hold out for the plum deals, whereupon then can be transmogrified into ants themselves.
Dubal describes the experience of Adil, a Syrian refugee who drives for Uber in the Bay Area. His colleagues are pickers, and showed him screenshots of how much they earned. Determined to get a share of that money, Adil became a model ant, driving two hours to San Francisco, driving three days straight, napping in his car, spending only one day per week with his family. The algorithm noticed that Adil needed the work, so it paid him less.
Adil responded the way the system predicted he would, by driving even more: “My friends they make it, so I keep going, maybe I can figure it out. It’s unsecure, and I don’t know how people they do it. I don’t know how I am doing it, but I have to. I mean, I don’t find another option. In a minute, if I find something else, oh man, I will be out immediately. I am a very patient person, that’s why I can continue.”
Another driver, Diego, told Dubal about how the winners of the giant teddy bears fell into the trap of thinking that they were “good at the app”: “Any time there’s some big shot getting high pay outs, they always shame everyone else and say you don’t know how to use the app. I think there’s secret PR campaigns going on that gives targeted payouts to select workers, and they just think it’s all them.”
That’s the power of twiddling: by hoarding all the flexibility offered by digital tools, the management at platforms can become centaurs, able to string along thousands of workers, while the workers are reverse-centaurs, puppeteered by the apps.
As the example of Adil shows, the algorithm doesn’t need to be very sophisticated in order to figure out which workers it can underpay. The system automates the kind of racial and gender discrimination that is formally illegal, but which is masked by the smokescreen of digitization. An employer who systematically paid women less than men, or Black people less than white people, would be liable to criminal and civil sanctions. But if an algorithm simply notices that people who have fewer job prospects drive more and will thus accept lower wages, that’s just “optimization,” not racism or sexism.
This is the key to understanding the AI hype bubble: when ghouls from multinational banks predict 13 trillion dollar markets for “AI,” what they mean is that digital tools will speed up the twiddling and other wage-suppression techniques to transfer $13T in value from workers and consumers to shareholders.
The American business lobby is relentlessly focused on the goal of reducing wages. That’s the force behind “free trade,” “right to work,” and other codewords for “paying workers less,” including “gig work.” Tech workers long saw themselves as above this fray, immune to labor exploitation because they worked for a noble profession that took care of its own.
But the epidemic of mass tech-worker layoffs, following on the heels of massive stock buybacks, has demonstrated that tech bosses are just like any other boss: willing to pay as little as they can get away with, and no more. Tech bosses are so comfortable with their market dominance and the lock-in of their customers that they are happy to turn out hundreds of thousands of skilled workers, convinced that the twiddling systems they’ve built are the kinds of self-licking ice-cream cones that are so simple even a manager can use them — no morlocks required.
The tech worker layoffs are best understood as an all-out war on tech worker morale, because that morale is the source of tech workers’ confidence and thus their demands for a larger share of the value generated by their labor. The current tech layoff template is very different from previous tech layoffs: today’s layoffs are taking place over a period of months, long after they are announced, and laid off tech worker is likely to be offered a months of paid post-layoff work, rather than severance. This means that tech workplaces are now haunted by the walking dead, workers who have been laid off but need to come into the office for months, even as the threat of layoffs looms over the heads of the workers who remain. As an old friend, recently laid off from Microsoft after decades of service, wrote to me, this is “a new arrow in the quiver of bringing tech workers to heel and ensuring that we’re properly thankful for the jobs we have (had?).”
Dubal is interested in more than analysis, she’s interested in action. She looks at the tactics already deployed by gig workers, who have not taken all this abuse lying down. Workers in the UK and EU organized through Worker Info Exchange and the App Drivers and Couriers Union have used the GDPR (the EU’s privacy law) to demand “algorithmic transparency,” as well as access to their data. In California, drivers hope to use similar provisions in the CCPA (a state privacy law) to do the same.
These efforts have borne fruit. When Cornell economists, led by Louis Hyman, published research (paid for by Uber) claiming that Uber drivers earned an average of $23/hour, it was data from these efforts that revealed the true average Uber driver’s wage was $9.74. Subsequent research in California found that Uber drivers’ wage fell to $6.22/hour after the passage of Prop 22, a worker misclassification law that gig companies spent $225m to pass, only to have the law struck down because of a careless drafting error:
https://www.latimes.com/california/newsletter/2021-08-23/proposition-22-lyft-uber-decision-essential-california
But Dubal is skeptical that data-coops and transparency will achieve transformative change and build real worker power. Knowing how the algorithm works is useful, but it doesn’t mean you can do anything about it, not least because the platform owners can keep touching their knobs, twiddling the payout schedule on their rigged slot-machines.
Data co-ops start from the proposition that “data extraction is an inevitable form of labor for which workers should be remunerated.” It makes on-the-job surveillance acceptable, provided that workers are compensated for the spying. But co-ops aren’t unions, and they don’t have the power to bargain for a fair price for that data, and coops themselves lack the vast resources — “to store, clean, and understand” — data.
Co-ops are also badly situated to understand the true value of the data that is extracted from their members: “Workers cannot know whether the data collected will, at the population level, violate the civil rights of others or amplifies their own social oppression.”
Instead, Dubal wants an outright, nonwaivable prohibition on algorithmic wage discrimination. Just make it illegal. If firms cannot use gambling mechanisms to control worker behavior through variable pay systems, they will have to find ways to maintain flexible workforces while paying their workforce predictable wages under an employment model. If a firm cannot manage wages through digitally-determined variable pay systems, then the firm is less likely to employ algorithmic management.”
In other words, rather than using market mechanisms too constrain platform twiddling, Dubal just wants to make certain kinds of twiddling illegal. This is a growing trend in legal scholarship. For example, the economist Ramsi Woodcock has proposed a ban on surge pricing as a per se violation of Section 1 of the Sherman Act:
https://ilr.law.uiowa.edu/print/volume-105-issue-4/the-efficient-queue-and-the-case-against-dynamic-pricing
Similarly, Dubal proposes that algorithmic wage discrimination violates another antitrust law: the Robinson-Patman Act, which “bans sellers from charging competing buyers different prices for the same commodity. Robinson-Patman enforcement was effectively halted under Reagan, kicking off a host of pathologies, like the rise of Walmart:
https://pluralistic.net/2023/03/27/walmarts-jackals/#cheater-sizes
I really liked Dubal’s legal reasoning and argument, and to it I would add a call to reinvigorate countertwiddling: reforming laws that get in the way of workers who want to reverse-engineer, spoof, and control the apps that currently control them. Adversarial interoperability (AKA competitive compatibility or comcom) is key tool for building worker power in an era of digital Taylorism:
https://www.eff.org/deeplinks/2019/10/adversarial-interoperability
To see how that works, look to other jursidictions where workers have leapfrogged their European and American cousins, such as Indonesia, where gig workers and toolsmiths collaborate to make a whole suite of “tuyul apps,” which let them override the apps that gig companies expect them to use.
https://pluralistic.net/2021/07/08/tuyul-apps/#gojek
For example, ride-hailing companies won’t assign a train-station pickup to a driver unless they’re circling the station — which is incredibly dangerous during the congested moments after a train arrives. A tuyul app lets a driver park nearby and then spoof their phone’s GPS fix to the ridehailing company so that they appear to be right out front of the station.
In an ideal world, those workers would have a union, and be able to dictate the app’s functionality to their bosses. But workers shouldn’t have to wait for an ideal world: they don’t just need jam tomorrow — they need jam today. Tuyul apps, and apps like Para, which allow workers to extract more money under better working conditions, are a prelude to unionization and employer regulation, not a substitute for it.
Employers will not give workers one iota more power than they have to. Just look at the asymmetry between the regulation of union employees versus union busters. Under US law, employees of a union need to account for every single hour they work, every mile they drive, every location they visit, in public filings. Meanwhile, the union-busting industry — far larger and richer than unions — operate under a cloak of total secrecy, Workers aren’t even told which union busters their employers have hired — let alone get an accounting of how those union busters spend money, or how many of them are working undercover, pretending to be workers in order to sabotage the union.
Twiddling will only get an employer so far. Twiddling — like all “AI” — is based on analyzing the past to predict the future. The heuristics an algorithm creates to lure workers into their cars can’t account for rapid changes in the wider world, which is why companies who relied on “AI” scheduling apps (for example, to prevent their employees from logging enough hours to be entitled to benefits) were caught flatfooted by the Great Resignation.
Workers suddenly found themselves with bargaining power thanks to the departure of millions of workers — a mix of early retirees and workers who were killed or permanently disabled by covid — and they used that shortage to demand a larger share of the fruits of their labor. The outraged howls of the capital class at this development were telling: these companies are operated by the kinds of “capitalists” that MLK once identified, who want “socialism for the rich and rugged individualism for the poor.”
https://twitter.com/KaseyKlimes/status/821836823022354432/
There's only 5 days left in the Kickstarter campaign for the audiobook of my next novel, a post-cyberpunk anti-finance finance thriller about Silicon Valley scams called Red Team Blues. Amazon's Audible refuses to carry my audiobooks because they're DRM free, but crowdfunding makes them possible.
Image: Stephen Drake (modified) https://commons.wikimedia.org/wiki/File:Analog_Test_Array_modular_synth_by_sduck409.jpg
CC BY 2.0 https://creativecommons.org/licenses/by/2.0/deed.en
—
Cryteria (modified) https://commons.wikimedia.org/wiki/File:HAL9000.svg
CC BY 3.0 https://creativecommons.org/licenses/by/3.0/deed.en
—
Louis (modified) https://commons.wikimedia.org/wiki/File:Chestnut_horse_head,_all_excited.jpg
CC BY-SA 2.0 https://creativecommons.org/licenses/by-sa/2.0/deed.en
[Image ID: A complex mandala of knobs from a modular synth. In the foreground, limned in a blue electric halo, is a man in a hi-viz vest with the head of a horse. The horse's eyes have been replaced with the sinister red eyes of HAL9000 from Kubrick's '2001: A Space Odyssey.'"]
#pluralistic#great resignation#twiddler#countertwiddling#wage discrimination#algorithmic#scholarship#doordash#para#Veena Dubal#labor#brian merchant#app boss#reverse centaurs#skinner boxes#enshittification#ants vs pickers#tuyul#steampunk#cottage industry#ccpa#gdpr#App Drivers and Couriers Union#shitty technology adoption curve#moral economy#gamblification#casinoization#taylorization#taylorism#giant teddy bears
3K notes
·
View notes
Text
(Article date: December 26, 2024)
I liked this Rolling Stone article about the upcoming potential TikTok ban. People like to make fun of TikTok users, but it really bugs me that so many people aren't thinking of the folks relying on TikTok for income. Livelihoods are being impacted. The category of "influencer" includes a lot of small-time artists barely scraping by. A significant number of my Patreon backers & regular donors found me via tumblr. If that went away overnight, I'd be screwed, and it's the same with TikTok for a LOT of people.
It reminds me a lot of Etsy changes screwing over indie artists--and as an Etsy seller, holy shit, y'all, so many Etsy sellers rely on TikTok to advertise. I don't, but I know several who do. TikTok pulls major, major numbers.
Like, the platform has issues, absolutely, but those issues exist across social media platforms, and a TikTok-specific ban is such a blatant case of "privacy violations are only okay when AMERICA does it!"
Article highlight:
Much of the talk around finances on the app has remained largely antagonistic against large-scale influencers. Users have joked that a ban will finally force big-name creators to get real jobs or join the real world. But smaller creators say this mindset completely leaves them out of the equation. ‘TikTok being banned is going to hurt me financially and I’m not an influencer. I’m not a big creator,” posted one emotional TikTok user, who said she usually only has around $122 left after bills for food, gas, and her mother’s medical supplies each month. “I entered the creative rewards program in July and I started making TikTok Shop videos once a month in July as well. It’s not been life-changing money, but it has been life-saving money for me. I don’t mean to be dramatic but it has saved us. I’m going to have to go back to figuring out how to survive.”
258 notes
·
View notes
Text
Kroger Grocery Data Scraping | Kroger Grocery Data Extraction
Shopping Kroger grocery online has become very common these days. At Foodspark, we scrape Kroger grocery apps data online with our Kroger grocery data scraping API as well as also convert data to appropriate informational patterns and statistics.
#food data scraping services#restaurantdataextraction#restaurant data scraping#web scraping services#grocerydatascraping#zomato api#fooddatascrapingservices#Scrape Kroger Grocery Data#Kroger Grocery Websites Apps#Kroger Grocery#Kroger Grocery data scraping company#Kroger Grocery Data#Extract Kroger Grocery Menu Data#Kroger grocery order data scraping services#Kroger Grocery Data Platforms#Kroger Grocery Apps#Mobile App Extraction of Kroger Grocery Delivery Platforms#Kroger Grocery delivery#Kroger grocery data delivery
1 note
·
View note
Text
iWeb Scraping provides the Best Restaurant Menu and Locations Data Scraping Services in USA, UAE, Australia and UK to scrape and extract Website for Restaurant Menus, Locations data.
1 note
·
View note
Text
Online food delivery apps scraping
3i Data Scraping provides Food ordering data extractor to scrape online food delivery apps like DoorDash, Postmates, goPuff, Seamless, Zomato, Ubereats, Grubhub, Swiggy, etc.

#food delivery app scraping#Extract Food Ordering Apps Data#web scrape food delivery#food delivery app data extraction#Extract food menu details#competitive price intelligence
1 note
·
View note
Text
Exploring the Uber Eats API: A Definitive Guide to Integration and Functionality
In this blog, we delve into the various types of data the Uber Eats API offers and demonstrate how they can be ingeniously harnessed to craft engaging and practical meal-serving apps.
#Uber Eats Data Scraping API#Scrape Uber Eats Data API#Extract Uber Eats Data#Scrape Food Delivery App Data#Food Delivery App Data Scraping
0 notes
Text
At the Top
Keigo Takami/ Hawks x reader
W.C~ 2k
~ Your date at the Fair turns from a questionable experience to a full-blown disaster when you and your online date get stranded at the top of the Ferris Wheel.

With the fair in town, you thought that spending the afternoon eating ridiculously sounding fair food, playing games, and riding the rides sounded like a picture-perfect first-date idea. But that's the problem with being a romantic.
You tend to get your hopes up…
"So… Are you having a good time so far?" the man sitting across from you asks for the 9th time this evening. The two of you had matched on a dating app a few weeks ago, and after many late nights of texting that filled your stomach with butterflies and heart with hope, you finally took the step and asked if he would like to actually go out.
But the evening has been less than ideal. Your date, although good-looking, clearly has some serious baggage from his last relationship that even your 'I can fix him' mindset wants to turn away from.
He has refused to go on ride after ride after ride with you, claiming that he and his friends rode all of them last year and thought they were just a waste of time. And he turned his nose up at any of the mouthwateringly atrocious fair food you wanted to try out and dragged you to the only place on the fairgrounds that sold smoothie bowls, which may be delicious, but they are something you could eat any other day whilst cheddar cheese flavored ice cream is not.
You try to hide your frown as you spin the deep purple, soupy concoction with your spoon as you stare across at your dark haired date and tell him what you think is a convincing lie, "Yes, I'm having a great time. Thank you for the acai bowl."
"Don't mention it," he chuckles, wiping his berry-dusted chin with his sleeve. "I'm glad I was able to find us something in this place that was organic. All that deep-fried garbage they sell at the other stalls is repulsing."
"Maybe," you say, stirring your bowl even more, really not wanting to engage in any kind of debate with this guy. You take a bite of your bowl, and while it is good, you hate that it costs the same as half a tank of gas.
Luckily, your date paid for that.
Just getting up and leaving is always an option, but there is still hope that things can turn around, or at least you'll have a decent bad date story to tell your friends when the night is over.
"How about we go on the Ferris wheel?" he says at last, boredly scraping the button of his paper bowl with his spoon.
"Really?" you say, thankful that you are finally able to go on one freaking ride on this date. Maybe you were being too critical of your date…
"Why not?" he says, "I didn't go on that one yet."
oh…
~
When you like someone, the idea of being wedged together on the Ferris wheel is something straight out of a romance movie, But when the already little spark of attraction you are feeling for your date has been drowned and smothered by the murky waters of his overflowing ego, the act is tortuous.
Despite the little legroom in your pod, your date has decided to take up most of it with his wide stance; his obvious manspreading gets more and more stifling as you rise slowly into the air. The multicolored light bulbs of the wheel flicker, and you wonder briefly if that is normal.
It's getting a bit cold up here, isn't it?" he asks with a sly smile. It's honestly not cold at all; in fact, the warm air is kinda stuffy. Before you can say anything, his arm slings over your shoulder, and you dig your nails into your palm; it doesn't feel right; you know how you feel when you are attracted to someone, and this is not it.
The flash of a camera phone catches your attention and you blink away the spots in your vision.
"Oh, that's perfect," he chuckles, looking at the selfie he just took of the both of you. "I'll send it to you and we can set it for our lock screens."
"Don't you think it's a little soon for that?" you ask, now wondering what kinda psycho you are trapped on this ride with.
"Hey, when you know you know Baby Cakes," he says with an almost hallmark channel level of confidence as he looks at the photo with a delusional smile. "Oh, we look great. I gotta send this photo to my Mom; she is gonna love you."
"Oh… how nice," you say dryly. You feel sick… in that moment, you decide that when you touch down on solid ground, you are going to get the hell out of dodge and take a cab home and leave this guy in the dust.
The ride reaches its peak, and you sigh; at least you only have a few minutes left of this tortuous experience.
"Hey, have you ever kissed someone at the top of a Ferris wheel before?" he asks, leaning in close.
"Not really my thing," you say, scooting as far away from him as you can. But in the little car, you can't really go far.
"Oh come on, don't be so shy, y/n, I don't ~" he starts to say when the ride suddenly jolts to a stop. Your little pod rocking back and forth."
"What was that?" he asks, getting a bit out of your bubble to look around. His lack of proximity makes you sigh in relief before realizing that you are stuck at the top of the Ferris wheel with this dude.
Hopefully he doesn't try anything…
"Oh my god, oh my god, we're gonna die," he says, completely freaking out. "This is all your fault, y/n." He glares at you with tears streaming down your face as snot pours from his nose as he rocks the cart back and forth.
You are speechless and have no idea what to do at this moment. All you can hope is that your date won't pee himself next to you in this little pod.
You look down at the pavement; at least if you fall, you won't have to deal with this guy anymore…
Suddenly, a red feather whizzes by your face. You follow it with your eyes. Turning your head, you come face-to-face with the most beautiful man you've ever seen.
Bright golden eyes staring into yours warmly from behind a yellow visor. Crimson wings beat softly in the air. "Hey there, you look like you could use a hand."
"H-hawks." Your date says addressing the pro hero. "Thank God you're here. Please get me down."
"No problem, folks, I'll get you down; it looks like that machine down there is a little jammed, so you'll have to bear with me." His feathers harden as they whittle away at the steel safety harness that keeps you trapped in the air.
"Now, don't move, all right? I had to cut the beam to get you guys out of here, so hang tight in those seat belts." He looks at you and holds out a hand. "how about I start with you? Wanna get back on solid ground?"
You smile. "So badly." His hand has a pleasant warmth through it, but right before a hero can gently take you out of your seat. The bench starts to swing rapidly.
"No!" your date screams, unbuckling his seatbelt. "don't take them, take me." he stands and lunges towards the Pro Hero, only to be halted in the air by a cluster of feathers latching to his clothing.
His arm pushes you off balance, and you slip, letting out a yelp as you wonder if this is the end. Frightfully, you imagine yourself becoming one with the pavement below, the worst end to the worst date of your life.
Strong arms are quick to grab you, and you are pulled into the safety of the winged hero's chest. "Don't worry, I gotcha," he says softly; the world beneath you makes your head spin. "Hey… Don't look down, you're safe. What's your name?"
The unwavering care in his voice calms you, and you answer. Out of the corner of your eye, you see the feathers bringing your date down to the ground and setting him on the pavement with an unceremonious plop.
"Serves him right," you mutter, "This has been the worst first date of my life."
"Don't tell me there's gonna be a second." He jokes as his feathers start to free the other passengers, setting them on the ground; he brushes a strand of hair out of your face and winks, "Just between you and me, I think you can do way better than that guy."
Your cheeks heat under the hero's surprisingly flirty banter, and you realize that you are still nestled comfortably in his arms, high above the ground. But instead of feeling worried, you feel strangely comfortable. Your stomach seems to flutter as you are brought down to earth.
"There you go," he says gently, placing you on solid ground. Although you are safe and sound, you can't help but feel a little disappointed that your short time with the Pro hero has to end so soon.
"Thank you for helping me out up there," you say, remembering your manners.
"It was my pleasure," he says; his golden gaze flickers from you to behind you and narrows. "Hey, I hope I'm not crossing a line or anything, but it looks like you may need a bit more saving." He gestures over to your still-sniveling date, who doesn't seem to notice you at all.
"Ugh, where were you three hours ago?" you chuckle dryly.
Your little comment sends him into a fit of laughter so hard he has to remove his visor to wipe tears from his eyes. "Sorry, I guess that saving your life was the best I could do."
"And you call yourself a hero," you shake your head and fight the smile on your lips.
"Can I make it up to you?" he asks earnestly. "My patrol ends in a few, so I could take you home or somewhere else, maybe grab some food."
Your brain shorts out for a moment as you are shocked by this unexpected turn of events.
Is he flirting with you?
This is Hawks, one of the most eligible bachelors in the country, and he is offering to take you to get food.
"It seems like you are going above and beyond the call of duty," you comment, watching as he tucks his wings to his back.
"Well, it just seems right," he says honestly. "You look like you need a better day, and I'm starving. So what do you say? Wanna use me as a good old-fashioned rebound?"
The corners of your lips turn upward. "I would."
"Perfect, then let me work my magic, and we can get out of here." he smiles, the toothy grin looking much more natural than the ones you see plastered on the magazine covers. He turns and walks over to a sidekick in an elastic red suit. "Hey, this one is a bit shaken up, so I'm gonna help them home; you got everything handled over here, right?"
They nod, and he turns on a dime, walking over to you with a poorly concealed pep in his step. "Are you ready to go?"
You nod eagerly. And motions for you to hang on tight to him. Although just a few minutes ago, you were disgusted at the idea of even brushing knuckles with your previous date, being in the arms of the hero is comfortable.
He launches the two of you into the air, and the wind tickles your face. Leaving your sniveling date in the dust.
"So where to first?" he yells above the breeze.
"There's a really good fried chicken food truck near my place if you want to try it out?" you say after a bit of thought.
His gaze fills with affection, and his laughter rises with his wings. "Oh, I think we are gonna get along just fine."

Tagging: @pixelcafe-network @sleepyyshroom @anjodedesgostoeerros @isaacdaknight @qardasngan @dog55teeth @atigerandabear
#my hero academia hawks#bnha fluff#my hero academia#bnha#hawks x reader fluff#keigo takami x reader#hawks x reader#keigo tamaki#bnha x reader#x reader
149 notes
·
View notes