#Data Lake Services
Explore tagged Tumblr posts
Text
How Data Lake Solutions Empower Corporate Analysts
Extensive datasets are inevitable in big data and social media analytics. Moreover, a global enterprise must serve a multi-lingual consumer base, highlighting the necessity for scalable data management tools. This page describes the facilities in data lake solutions that help corporate data operators excel in analytics and data governance.
1 note
·
View note
Text
0 notes
Text
Empowering Small Businesses with Big Data Solutions! 🚀 Explore AWS Data Lake & Analytics for SMBs.
0 notes
Text
Businesses can use sophisticated data lake setup services from SG Analytics, a reputable market pioneer. Organizations can harness the power of data lakes by utilizing SG Analytics' experience in data management and analytics. Large amounts of organized and unstructured data may be stored and analyzed more easily with the help of a data lake, which acts as a central repository. Businesses may access priceless insights to fuel growth and innovation using SG Analytics' seamless integration, effective data governance, and advanced analytics capabilities. Utilize the comprehensive solutions from SG Analytics to realize the revolutionary potential of data lakes fully. Explore their data management and analytics services right now by visiting their website!
1 note
·
View note
Text
In the digital age, organizations are inundated with vast amounts of data from various sources. To effectively harness the power of this data, enterprises require robust solutions that enable comprehensive data management. One such solution that has gained significant traction is the Enterprise Data Lake. With its ability to store, process, and analyze large volumes of structured and unstructured data, the Enterprise Data Lake is revolutionizing how organizations handle their data.
0 notes
Text
I really wish I wasn't living in the middle of a natural disaster site right now :D
#'oh we don't get tornadoes in northeast ohio we're on the lake'#welp....it finally happened. We had our first tornado yesterday#and you know where it hit? my childhood home :D#i'm lucky enough to have power but we have no internet and everyone around us is gonna be out until at least the weekend#i go on vacation this weekend but like....y'all....i'm still so freaked out#my ptsd didn't have time to kick in yesterday because i was at work and protecting my students but uhhhh it's kicked in today :D#and only having spotty cell service and data is making it worse#it's hard to stay connected and keep an eye on what's going on when you can't access the fucking internet#but tumblr works lmao
4 notes
·
View notes
Photo
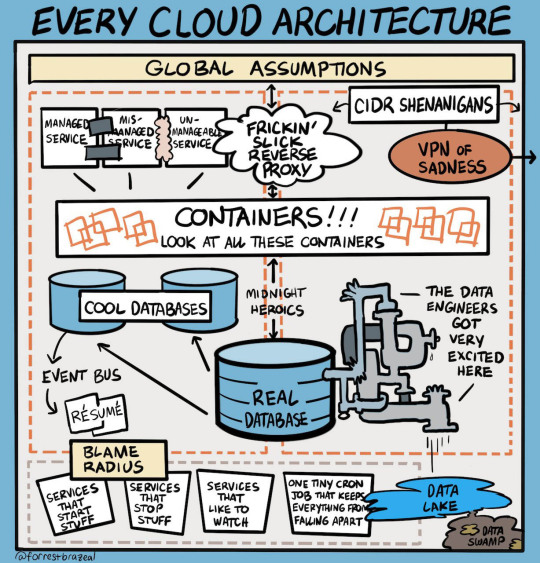
Every Cloud Architecture
#The Cloud#cloud services#CIDR Shenanigans#VPN of Sadness#cool databases#blame radius#one tiny cron#midnight heroics#data lake
1 note
·
View note
Text
Databricks vs. Snowflake: Key Differences Explained
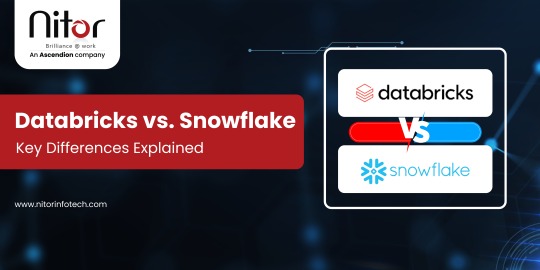
What if businesses could overcome the challenges of data silos, slow query performance, and limited real-time analytics? Well, it's a reality now, as data cloud platforms like Databricks and Snowflake have transformed how organizations manage and analyze their data.
Founded in 2012, Snowflake emerged from the expertise of data warehousing professionals, establishing itself as a SQL-centric solution for modern data needs. In contrast, Databricks, launched shortly after in 2013, originated from the creators of Apache Spark, positioning itself as a managed service for big data processing and machine learning.
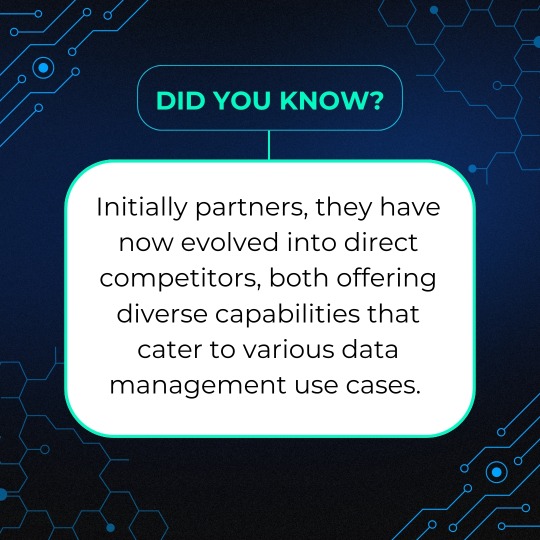
Scroll ahead to discover everything about these platforms and opt for the best option.
Benefits of Databricks and Snowflake
Here are the benefits that you can enjoy with Databricks:
It has been tailored for data science and machine learning workloads.
It supports complex data transformations and real-time analytics.
It adapts to the needs of data engineers and scientists.
It enables teams to work together on projects, enhancing innovation and efficiency.
It allows for immediate insights and data-driven decision-making.
In contrast, here are the benefits you can experience with Snowflake:
It is ideal for organizations focused on business intelligence and analytics.
It helps with storage and the compute resources can be scaled separately, ensuring optimal performance.
It efficiently handles large volumes of data without performance issues.
It is easy to use for both technical and non-technical users, promoting widespread adoption.
It offers a wide range of functionalities to support various industry needs.
Note: Visit their website to learn more about the pricing of Databricks and Snowflake.
Now, let’s compare each of the platforms based on various use cases/features.
Databricks vs. Snowflake: Comparison of Essential Features
When comparing essential features, several use cases highlight the differences between Databricks and Snowflake. Here are the top four factors that will provide clarity on each platform's strengths and capabilities:
1. Data Ingestion: Snowflake utilizes the ‘COPY INTO’ command for data loading, often relying on third-party tools for ingestion. In contrast, Databricks enables direct interaction with data in cloud storage, providing more flexibility in handling various data formats.
2. Data Transformation: Snowflake predominantly uses SQL for data transformations, while Databricks leverages Spark, allowing for more extensive customization and the ability to handle massive datasets effectively.
3. Machine Learning: Databricks boasts of a mature ecosystem for machine learning with features like MLflow and model serving. On the other hand, Snowflake is catching up with the introduction of Snowpark, allowing users to run machine learning models within its environment.
4. Data Governance: Snowflake provides extensive metadata and cost management features, while Databricks offers a robust data catalog through its Unity Catalog (it is still developing its cost management capabilities).
In a nutshell, both Databricks and Snowflake have carved their niches in the data cloud landscape, each with its unique capabilities. As both platforms continue to evolve and expand their feature sets, the above read will help businesses make informed decisions to optimize their data strategies and achieve greater insights.
Feel free to share this microblog with your network and connect with us at Nitor Infotech to elevate your business through cutting-edge technologies.
#data bricks#data warehouse#database warehousing#data lake#snowflake data#software development#snowflake pricing#snowflake#software engineering#blog#software services#artificial intelligence
0 notes
Text
Data Lake Consulting Services | Goognu – Unlock Insights from Your Data
Harness the power of your data with Goognu's Data Lake Consulting Services. We offer expert solutions for efficient data management and insightful analytics.
0 notes
Text
Understanding On-Premise Data Lakehouse Architecture
New Post has been published on https://thedigitalinsider.com/understanding-on-premise-data-lakehouse-architecture/
Understanding On-Premise Data Lakehouse Architecture
In today’s data-driven banking landscape, the ability to efficiently manage and analyze vast amounts of data is crucial for maintaining a competitive edge. The data lakehouse presents a revolutionary concept that’s reshaping how we approach data management in the financial sector. This innovative architecture combines the best features of data warehouses and data lakes. It provides a unified platform for storing, processing, and analyzing both structured and unstructured data, making it an invaluable asset for banks looking to leverage their data for strategic decision-making.
The journey to data lakehouses has been evolutionary in nature. Traditional data warehouses have long been the backbone of banking analytics, offering structured data storage and fast query performance. However, with the recent explosion of unstructured data from sources including social media, customer interactions, and IoT devices, data lakes emerged as a contemporary solution to store vast amounts of raw data.
The data lakehouse represents the next step in this evolution, bridging the gap between data warehouses and data lakes. For banks like Akbank, this means we can now enjoy the benefits of both worlds – the structure and performance of data warehouses, and the flexibility and scalability of data lakes.
Hybrid Architecture
At its core, a data lakehouse integrates the strengths of data lakes and data warehouses. This hybrid approach allows banks to store massive amounts of raw data while still maintaining the ability to perform fast, complex queries typical of data warehouses.
Unified Data Platform
One of the most significant advantages of a data lakehouse is its ability to combine structured and unstructured data in a single platform. For banks, this means we can analyze traditional transactional data alongside unstructured data from customer interactions, providing a more comprehensive view of our business and customers.
Key Features and Benefits
Data lakehouses offer several key benefits that are particularly valuable in the banking sector.
Scalability
As our data volumes grow, the lakehouse architecture can easily scale to accommodate this growth. This is crucial in banking, where we’re constantly accumulating vast amounts of transactional and customer data. The lakehouse allows us to expand our storage and processing capabilities without disrupting our existing operations.
Flexibility
We can store and analyze various data types, from transaction records to customer emails. This flexibility is invaluable in today’s banking environment, where unstructured data from social media, customer service interactions, and other sources can provide rich insights when combined with traditional structured data.
Real-time Analytics
This is crucial for fraud detection, risk assessment, and personalized customer experiences. In banking, the ability to analyze data in real-time can mean the difference between stopping a fraudulent transaction and losing millions. It also allows us to offer personalized services and make split-second decisions on loan approvals or investment recommendations.
Cost-Effectiveness
By consolidating our data infrastructure, we can reduce overall costs. Instead of maintaining separate systems for data warehousing and big data analytics, a data lakehouse allows us to combine these functions. This not only reduces hardware and software costs but also simplifies our IT infrastructure, leading to lower maintenance and operational costs.
Data Governance
Enhanced ability to implement robust data governance practices, crucial in our highly regulated industry. The unified nature of a data lakehouse makes it easier to apply consistent data quality, security, and privacy measures across all our data. This is particularly important in banking, where we must comply with stringent regulations like GDPR, PSD2, and various national banking regulations.
On-Premise Data Lakehouse Architecture
An on-premise data lakehouse is a data lakehouse architecture implemented within an organization’s own data centers, rather than in the cloud. For many banks, including Akbank, choosing an on-premise solution is often driven by regulatory requirements, data sovereignty concerns, and the need for complete control over our data infrastructure.
Core Components
An on-premise data lakehouse typically consists of four core components:
Data storage layer
Data processing layer
Metadata management
Security and governance
Each of these components plays a crucial role in creating a robust, efficient, and secure data management system.
Data Storage Layer
The storage layer is the foundation of an on-premise data lakehouse. We use a combination of Hadoop Distributed File System (HDFS) and object storage solutions to manage our vast data repositories. For structured data, like customer account information and transaction records, we leverage Apache Iceberg. This open table format provides excellent performance for querying and updating large datasets. For our more dynamic data, such as real-time transaction logs, we use Apache Hudi, which allows for upserts and incremental processing.
Data Processing Layer
The data processing layer is where the magic happens. We employ a combination of batch and real-time processing to handle our diverse data needs.
For ETL processes, we use Informatica PowerCenter, which allows us to integrate data from various sources across the bank. We’ve also started incorporating dbt (data build tool) for transforming data in our data warehouse.
Apache Spark plays a crucial role in our big data processing, allowing us to perform complex analytics on large datasets. For real-time processing, particularly for fraud detection and real-time customer insights, we use Apache Flink.
Query and Analytics
To enable our data scientists and analysts to derive insights from our data lakehouse, we’ve implemented Trino for interactive querying. This allows for fast SQL queries across our entire data lake, regardless of where the data is stored.
Metadata Management
Effective metadata management is crucial for maintaining order in our data lakehouse. We use Apache Hive metastore in conjunction with Apache Iceberg to catalog and index our data. We’ve also implemented Amundsen, LinkedIn’s open-source metadata engine, to help our data team discover and understand the data available in our lakehouse.
Security and Governance
In the banking sector, security and governance are paramount. We use Apache Ranger for access control and data privacy, ensuring that sensitive customer data is only accessible to authorized personnel. For data lineage and auditing, we’ve implemented Apache Atlas, which helps us track the flow of data through our systems and comply with regulatory requirements.
Infrastructure Requirements
Implementing an on-premise data lakehouse requires significant infrastructure investment. At Akbank, we’ve had to upgrade our hardware to handle the increased storage and processing demands. This included high-performance servers, robust networking equipment, and scalable storage solutions.
Integration with Existing Systems
One of our key challenges was integrating the data lakehouse with our existing systems. We developed a phased migration strategy, gradually moving data and processes from our legacy systems to the new architecture. This approach allowed us to maintain business continuity while transitioning to the new system.
Performance and Scalability
Ensuring high performance as our data grows has been a key focus. We’ve implemented data partitioning strategies and optimized our query engines to maintain fast query response times even as our data volumes increase.
In our journey to implement an on-premise data lakehouse, we’ve faced several challenges:
Data integration issues, particularly with legacy systems
Maintaining performance as data volumes grow
Ensuring data quality across diverse data sources
Training our team on new technologies and processes
Best Practices
Here are some best practices we’ve adopted:
Implement strong data governance from the start
Invest in data quality tools and processes
Provide comprehensive training for your team
Start with a pilot project before full-scale implementation
Regularly review and optimize your architecture
Looking ahead, we see several exciting trends in the data lakehouse space:
Increased adoption of AI and machine learning for data management and analytics
Greater integration of edge computing with data lakehouses
Enhanced automation in data governance and quality management
Continued evolution of open-source technologies supporting data lakehouse architectures
The on-premise data lakehouse represents a significant leap forward in data management for the banking sector. At Akbank, it has allowed us to unify our data infrastructure, enhance our analytical capabilities, and maintain the highest standards of data security and governance.
As we continue to navigate the ever-changing landscape of banking technology, the data lakehouse will undoubtedly play a crucial role in our ability to leverage data for strategic advantage. For banks looking to stay competitive in the digital age, seriously considering a data lakehouse architecture – whether on-premise or in the cloud – is no longer optional, it’s imperative.
#access control#ai#Analytics#Apache#Apache Spark#approach#architecture#assessment#automation#bank#banking#banks#Big Data#big data analytics#Business#business continuity#Cloud#comprehensive#computing#customer data#customer service#data#data analytics#Data Centers#Data Governance#Data Integration#data lake#data lakehouse#data lakes#Data Management
0 notes
Text
Unlock the Power of Data with Data Lake Solutions
Explore our Data Lake Implementation services at SG Analytics UK to harness the full potential of your data. Our expert team will help you build a robust data lake infrastructure, enabling efficient data storage, management, and advanced analytics. Transform your data into actionable insights with SG Analytics today.
1 note
·
View note
Text
Enhancing Data-Driven Decision-Making with Azure Data Lake Services
Introduction: The Importance of Data-Driven Decision-Making

In today’s fast-paced business environment, data-driven decision-making is essential for success. Organizations that leverage data to guide their strategies and operations are better positioned to adapt to changes, optimize performance, and achieve their goals. However, making data-driven decisions requires access to accurate, timely, and comprehensive data. This is where Azure Data Lake Services play a crucial role, providing the infrastructure needed to collect, store, and analyze vast amounts of data.
In this blog, we’ll explore how Azure Data Lake Services can enhance data-driven decision-making in your organization.
Centralized Data Storage for Comprehensive Insights
One of the key challenges businesses face is managing data from multiple sources. Data silos can lead to incomplete insights, making it difficult to see the full picture. Azure Data Lake Services addresses this challenge by providing a centralized repository for all your data, regardless of its source or format.
Key Benefits:
Unified Data View: By centralizing your data in a single location, Azure Data Lake Services eliminates data silos and provides a unified view of your business. This comprehensive perspective enables more accurate and informed decision-making.
Support for All Data Types: Azure Data Lake Services can store structured, semi-structured, and unstructured data, allowing you to integrate data from various sources, such as databases, IoT devices, and social media platforms.
Scalable Storage: As your data grows, Azure Data Lake Services scales with your needs, ensuring that you always have the capacity to store and analyze your data.
Real-Time Analytics for Timely Decision-Making
In a competitive business environment, the ability to make quick decisions based on real-time data is a significant advantage. Azure Data Lake Services provides the tools needed to perform real-time analytics, allowing you to respond to opportunities and challenges as they arise.
Key Applications:
Real-Time Monitoring: With Azure Data Lake Services, you can monitor key metrics in real-time, enabling you to detect trends, identify issues, and take action immediately. This is particularly valuable in industries such as finance, where timely insights can lead to better investment decisions.
Stream Analytics: Azure Data Lake Services integrates with Azure Stream Analytics, allowing you to process and analyze streaming data from sources such as IoT devices and social media. This enables you to gain real-time insights into customer behavior, market trends, and operational performance.
Predictive Analytics: By analyzing historical data in real-time, Azure Data Lake Services allows you to build predictive models that anticipate future events. This can help you make proactive decisions, such as adjusting inventory levels before a spike in demand or identifying potential equipment failures before they occur.
Seamless Integration with Analytics and BI Tools
For data-driven decision-making to be effective, data must be accessible and actionable. Azure Data Lake Services seamlessly integrates with a wide range of analytics and business intelligence (BI) tools, enabling you to easily analyze data and share insights across your organization.
Key Integrations:
Power BI: Azure Data Lake Services integrates with Power BI, Microsoft’s powerful BI tool, allowing you to create interactive dashboards and reports that visualize data in real-time. This makes it easy for decision-makers to understand complex data and identify trends.
Azure Synapse Analytics: Azure Data Lake Services works seamlessly with Azure Synapse Analytics, providing a comprehensive solution for big data analytics. This integration allows you to analyze large datasets, perform complex queries, and generate insights that drive strategic decisions.
Machine Learning: Azure Data Lake Services integrates with Azure Machine Learning, enabling you to build, train, and deploy machine learning models that predict outcomes and automate decision-making processes.
Best Practices for Leveraging Azure Data Lake Services
To fully leverage the benefits of Azure Data Lake Services for data-driven decision-making, it’s important to follow best practices for data management and analytics.
Key Recommendations:
Data Governance: Establish data governance policies to ensure that your data is accurate, secure, and compliant with regulations. This includes setting access controls, monitoring data usage, and implementing data quality checks.
Automation: Use automation tools to streamline data ingestion, processing, and analysis. Azure Data Lake Services offers several automation features that can help you save time and reduce manual effort.
Continuous Improvement: Regularly review and refine your data analytics processes to ensure that they continue to meet your business needs. This might involve updating data models, exploring new data sources, or adopting new analytics tools.
Conclusion
Azure Data Lake Services is a powerful tool for enhancing data-driven decision-making in your organization. By providing a centralized, scalable, and secure repository for all your data, Azure Data Lake Services enables you to gain comprehensive insights and make timely, informed decisions. Whether you’re looking to optimize operations, improve customer experiences, or drive strategic initiatives, Azure Data Lake Services provides the infrastructure and tools needed to succeed in today’s data-driven world.
Invest in Azure Data Lake Services to empower your organization with the insights needed to stay competitive and achieve your business goals.
0 notes
Text
Maximizing Data Lake Benefits: Atgeir Solutions' Expert Approach
In today's data-driven landscape, businesses rely on efficient data management to gain a competitive edge. Data lakes have emerged as a powerful solution for storing and analyzing vast amounts of structured, unstructured, and semi-structured data. Atgeir Solutions is at the forefront of data lake implementation, offering an expert approach to help organizations leverage their data effectively. Why Data Lakes Matter Data lakes offer a unified storage solution that allows data to be ingested without the need for immediate transformation. This means data can retain its raw form, serving various purposes, including machine learning and data lineage analysis. Atgeir Solutions understands the significance of this feature and helps companies establish a unified landing zone for their data. This approach ensures that data serves its intended purpose, providing organizations with a wealth of valuable insights. Addressing Compliance and Security One of the critical challenges in handling data lakes is ensuring data compliance, especially with regulations like GDPR. Atgeir Solutions takes this concern seriously, implementing robust security measures such as Access Control Lists (ACLs) and responsibility-based controls for team members. This approach ensures that data is safeguarded while maintaining compliance with data protection laws, allowing businesses to store data for longer terms without concerns. Efficient Data Cataloging Organizing data during the ingestion process is vital for future utilization. Atgeir Solutions employs management tools to automate the cataloging of data, making it easy for organizations to find and use their data efficiently. With organized data, businesses can extract meaningful insights and drive actionable outcomes. In-House Expertise Atgeir Solutions' success in data lake implementation is not just due to their robust methodologies but also their team of experienced experts. They understand the intricacies of data management, and their in-house expertise allows them to address challenges that inexperienced teams might struggle with. When it comes to data lake services, Atgeir Solutions is a trusted partner that ensures data operations run smoothly. Data Lake Advantages Implementing a data lake can bring significant advantages to an organization. With the ability to store vast amounts of data in its raw form, businesses can derive valuable insights and drive measurable outcomes. Atgeir Solutions' data lake services are designed to maximize these benefits, ensuring that businesses can turn their data into a valuable asset. Our Proven Process Atgeir Solutions follows a tried and tested methodology to implement data lakes. This methodology has consistently delivered results for their clients. By leveraging their expertise, they help organizations overcome challenges such as slow data analytics, unreasonable costs, poor data pipelines, and inexperienced teams. Their approach is geared towards streamlining data operations and driving success. Conclusion In an era where data is a valuable asset, effective data management is essential. Data lakes have emerged as a crucial solution, enabling businesses to store and analyze data efficiently. Atgeir Solutions stands out as a trusted partner for data lake implementation, offering rapid solutions, compliance, and security measures to help businesses unlock the full potential of their data. By choosing Atgeir Solutions, organizations can transform data into actionable insights and drive measurable outcomes, securing their position in today's data-driven business landscape.
0 notes
Text
In today's data-driven world, effective data storage and management is a major concern for businesses of all sizes. Traditional storage solutions can be expensive, cumbersome, and often fail to meet the growing data needs of modern organizations. This is where cloud storage services like Azure Blob Storage come into play, providing a scalable, secure, and cost-effective solution for data storage needs. Read more..
1 note
·
View note
Text
Data Lake Consulting Implementation | Data Lake Solutions and Services
SG Analytics provides comprehensive data lake implementation services, empowering businesses to centralize and optimize their data management. Their solutions encompass data integration, storage, and analysis, enabling organizations to make informed decisions based on reliable and consolidated information.
1 note
·
View note
Text
Unlocking the Power of Data Lake Implementation Services
Understanding Data Lakes
Before delving into Data Lake Implementation Services, it’s essential to understand what a Data Lake is. A Data Lake is a centralized repository that allows organizations to store vast amounts of structured and unstructured data in its raw format. Unlike traditional data storage systems, Data Lakes can store data of any type and size, making them highly flexible and scalable.
The Importance of Data Lake Implementation Services
Data Lake Implementation Services encompass a range of activities aimed at designing, building, and managing Data Lakes tailored to the specific needs of organizations. These services offer several benefits, including:
1. Scalability: Data Lake Implementation Services enable organizations to build scalable Data Lakes capable of handling large volumes of data. This scalability ensures that organizations can accommodate growing data needs without compromising performance.
2. Flexibility: With Data Lake Implementation Services, organizations can design Data Lakes that support various data types, including structured, semi-structured, and unstructured data. This flexibility allows organizations to store and analyze diverse data sources efficiently.
3. Data Integration: Data Lake Implementation Services facilitate the integration of data from multiple sources into a single, centralized repository. This integration ensures that organizations have a comprehensive view of their data, enabling better decision-making and analysis.
4. Data Governance: Effective Data Lake Implementation Services incorporate robust data governance frameworks to ensure data quality, security, and compliance. This ensures that organizations can trust the integrity of their data and adhere to regulatory requirements.
5. Advanced Analytics: Data Lake Implementation Services empower organizations to leverage advanced analytics and machine learning capabilities to derive valuable insights from their data. By combining Data Lakes with analytics tools, organizations can uncover hidden patterns, trends, and correlations that drive business growth.
1. Data Architecture Design: This involves designing the overall architecture of the Data Lake, including data ingestion, storage, processing, and access layers.
2. Data Ingestion: Data Lake Implementation Services include mechanisms for ingesting data from various sources, such as databases, streaming platforms, IoT devices, and external data feeds.
3. Data Storage: Data Lake Implementation Services define the storage mechanisms for storing raw and processed data within the Data Lake, such as distributed file systems or cloud storage solutions.
4. Data Processing: Data Lake Implementation Services encompass data processing capabilities for transforming, cleansing, and enriching data within the Data Lake using technologies like Apache Spark or Hadoop.
5. Data Governance and Security: Data Lake Implementation Services include features for implementing data governance policies, access controls, encryption, and compliance measures to ensure data security and regulatory compliance.
Conclusion
Data Lake Implementation Data Engineering Services play a crucial role in helping organizations harness the power of their data effectively. By providing scalable, flexible, and integrated Data Lakes, these services enable organizations to derive actionable insights, drive innovation, and gain a competitive edge in today’s data-driven landscape. As organizations continue to prioritize data-driven decision-making, the demand for Data Lake Implementation Services is expected to grow, making them indispensable for organizations looking to unlock the full potential of their data.
0 notes