#BigQuery best practices
Explore tagged Tumblr posts
Text
Google BigQuery: A Solução de Análise de Big Data na Nuvem
O Google BigQuery é uma poderosa plataforma de análise de dados em grande escala que faz parte do Google Cloud Platform (GCP). Com o aumento exponencial da quantidade de dados gerados pelas empresas, a necessidade de ferramentas de análise eficientes, rápidas e escaláveis se tornou essencial. O Google BigQuery foi criado para atender a essa demanda, oferecendo uma solução robusta para consultas…
#BigQuery#BigQuery analytics#BigQuery API#BigQuery best practices#BigQuery data types#BigQuery datasets#BigQuery ETL#BigQuery export#BigQuery functions#BigQuery integration#BigQuery joins#BigQuery limits#BigQuery machine learning#BigQuery optimization#BigQuery partitioning#BigQuery performance#BigQuery pricing#BigQuery python#BigQuery queries#BigQuery schema#BigQuery security#BigQuery SQL#BigQuery storage#BigQuery streaming#BigQuery tables#BigQuery tutorial#BigQuery use cases#BigQuery visualization#BigQuery vs Redshift#Google BigQuery
0 notes
Text
Gemini Code Assist Enterprise: AI App Development Tool
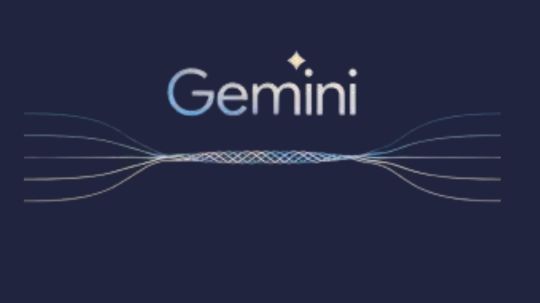
Introducing Gemini Code Assist Enterprise’s AI-powered app development tool that allows for code customisation.
The modern economy is driven by software development. Unfortunately, due to a lack of skilled developers, a growing number of integrations, vendors, and abstraction levels, developing effective apps across the tech stack is difficult.
To expedite application delivery and stay competitive, IT leaders must provide their teams with AI-powered solutions that assist developers in navigating complexity.
Google Cloud thinks that offering an AI-powered application development solution that works across the tech stack, along with enterprise-grade security guarantees, better contextual suggestions, and cloud integrations that let developers work more quickly and versatile with a wider range of services, is the best way to address development challenges.
Google Cloud is presenting Gemini Code Assist Enterprise, the next generation of application development capabilities.
Beyond AI-powered coding aid in the IDE, Gemini Code Assist Enterprise goes. This is application development support at the corporate level. Gemini’s huge token context window supports deep local codebase awareness. You can use a wide context window to consider the details of your local codebase and ongoing development session, allowing you to generate or transform code that is better appropriate for your application.
With code customization, Code Assist Enterprise not only comprehends your local codebase but also provides code recommendations based on internal libraries and best practices within your company. As a result, Code Assist can produce personalized code recommendations that are more precise and pertinent to your company. In addition to finishing difficult activities like updating the Java version across a whole repository, developers can remain in the flow state for longer and provide more insights directly to their IDEs. Because of this, developers can concentrate on coming up with original solutions to problems, which increases job satisfaction and gives them a competitive advantage. You can also come to market more quickly.
GitLab.com and GitHub.com repos can be indexed by Gemini Code Assist Enterprise code customisation; support for self-hosted, on-premise repos and other source control systems will be added in early 2025.
Yet IDEs are not the only tool used to construct apps. It integrates coding support into all of Google Cloud’s services to help specialist coders become more adaptable builders. The time required to transition to new technologies is significantly decreased by a code assistant, which also integrates the subtleties of an organization’s coding standards into its recommendations. Therefore, the faster your builders can create and deliver applications, the more services it impacts. To meet developers where they are, Code Assist Enterprise provides coding assistance in Firebase, Databases, BigQuery, Colab Enterprise, Apigee, and Application Integration. Furthermore, each Gemini Code Assist Enterprise user can access these products’ features; they are not separate purchases.
Gemini Code Support BigQuery enterprise users can benefit from SQL and Python code support. With the creation of pre-validated, ready-to-run queries (data insights) and a natural language-based interface for data exploration, curation, wrangling, analysis, and visualization (data canvas), they can enhance their data journeys beyond editor-based code assistance and speed up their analytics workflows.
Furthermore, Code Assist Enterprise does not use the proprietary data from your firm to train the Gemini model, since security and privacy are of utmost importance to any business. Source code that is kept separate from each customer’s organization and kept for usage in code customization is kept in a Google Cloud-managed project. Clients are in complete control of which source repositories to utilize for customization, and they can delete all data at any moment.
Your company and data are safeguarded by Google Cloud’s dedication to enterprise preparedness, data governance, and security. This is demonstrated by projects like software supply chain security, Mandiant research, and purpose-built infrastructure, as well as by generative AI indemnification.
Google Cloud provides you with the greatest tools for AI coding support so that your engineers may work happily and effectively. The market is also paying attention. Because of its ability to execute and completeness of vision, Google Cloud has been ranked as a Leader in the Gartner Magic Quadrant for AI Code Assistants for 2024.
Gemini Code Assist Enterprise Costs
In general, Gemini Code Assist Enterprise costs $45 per month per user; however, a one-year membership that ends on March 31, 2025, will only cost $19 per month per user.
Read more on Govindhtech.com
#Gemini#GeminiCodeAssist#AIApp#AI#AICodeAssistants#CodeAssistEnterprise#BigQuery#Geminimodel#News#Technews#TechnologyNews#Technologytrends#Govindhtech#technology
2 notes
·
View notes
Text
What is Google Cloud (GCP) MasterClass?
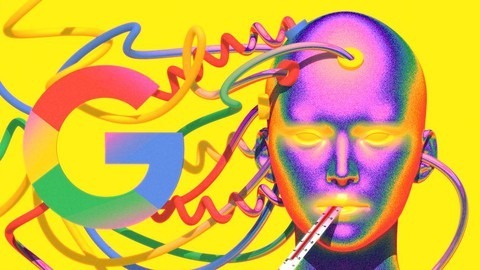
The Google Cloud (GCP) MasterClass is a comprehensive training program designed to provide learners with a deep understanding of Google Cloud’s core services and advanced functionalities. If you’re someone who is serious about building a career in cloud computing, this course could be your key to success. You’ll learn how to manage, deploy, and scale applications using Google Cloud Platform—skills that are in high demand across the tech world.
Why You Should Learn Google Cloud (GCP)
When it comes to cloud computing, Google Cloud (GCP) stands tall alongside AWS and Microsoft Azure. But what makes GCP unique is its integration with Google’s global infrastructure, giving you access to a secure and scalable platform used by some of the biggest names in the industry like Spotify, Snapchat, and Airbnb.
With companies increasingly migrating their IT infrastructure to the cloud, GCP-certified professionals are more sought-after than ever. According to multiple reports, job roles in cloud computing are among the top-paying tech positions, and the demand for Google Cloud skills has been growing exponentially. So, if you're looking for a career that is both lucrative and future-proof, mastering Google Cloud is a great step forward.
What Does the Google Cloud (GCP) MasterClass Offer?
Foundations of Google Cloud Platform (GCP)
The course begins with an overview of GCP—understanding its core components like Compute Engine, Cloud Storage, and BigQuery. You’ll get acquainted with the basics, such as creating a virtual machine, setting up a cloud environment, and managing cloud projects.
Hands-on Experience with Real-World Projects
One of the standout features of this MasterClass is the hands-on labs. You’ll work on actual cloud projects that simulate real-world challenges, giving you practical experience that you can apply in your job or business. These projects are specifically designed to mirror the challenges faced by enterprises using GCP, making this learning experience invaluable.
Mastering Cloud Security and Networking
In today’s digital world, security is a top priority. This course will teach you how to secure your cloud environment, manage access controls, and configure networking solutions using GCP's Identity and Access Management (IAM) and VPC networks.
Advanced Data Analytics and Machine Learning
The MasterClass goes beyond just cloud infrastructure. You’ll dive into data analytics and machine learning with tools like BigQuery and TensorFlow. The Google Cloud (GCP) MasterClass prepares you to handle large-scale data, build predictive models, and use AI-driven solutions to solve complex problems.
Who Is This Course For?
IT professionals looking to transition to cloud computing
Developers who want to deploy and scale apps on Google Cloud
Data engineers and analysts keen on using GCP’s data tools
Business leaders aiming to drive their organization’s digital transformation through the cloud
Students and fresh graduates who want to add an in-demand skill to their resume
No matter where you are in your career, the Google Cloud (GCP) MasterClass can help you upskill and stand out in the competitive job market.
What Will You Achieve After Completing the Google Cloud (GCP) MasterClass?
Google Cloud Certification: Upon completion, you'll be equipped to pursue the Google Cloud Certified Professional exams. Certification acts as an industry-recognized badge of expertise that can significantly boost your career.
Practical Expertise: The hands-on labs and real-world projects ensure you have the practical skills to handle cloud infrastructure, deploy scalable solutions, and implement security best practices.
Career Advancement: With companies globally shifting to cloud infrastructure, GCP-certified professionals are landing high-paying roles like Cloud Architect, Data Engineer, and DevOps Engineer. Whether you're looking to get promoted or switch careers, this MasterClass will give you the tools you need.
Benefits of Enrolling in Google Cloud (GCP) MasterClass
High Job Demand: The demand for cloud professionals with expertise in Google Cloud is at an all-time high. By completing this course, you put yourself in a strong position for roles such as Cloud Engineer, Cloud Solutions Architect, and Data Analyst.
Real-World Skills: You won’t just be learning theory. The MasterClass offers real-world projects, which means you'll be ready to jump into a job and start applying what you've learned.
Lucrative Career Paths: Cloud computing is one of the highest-paying fields in tech, and Google Cloud professionals often command top salaries. Completing this course could be your stepping stone to a rewarding, high-paying career.
Career Flexibility: Google Cloud skills are versatile. Whether you want to work as a freelancer, join a startup, or land a role at a tech giant, the knowledge you gain from the Google Cloud (GCP) MasterClass will serve you well.
Key Features of Google Cloud (GCP) MasterClass:
Comprehensive Course Content: From the fundamentals to advanced GCP tools like BigQuery, Kubernetes, and Cloud Machine Learning Engine, this course covers it all.
Updated Curriculum: The tech industry evolves quickly, but you can be assured that this course keeps pace. You’ll learn the latest GCP features, tools, and best practices to keep you relevant in today’s market.
Industry-Leading Instructors: The course is taught by experts with hands-on experience in Google Cloud and cloud computing. You’ll learn from the best, ensuring that you get top-quality instruction.
Why Should Businesses Invest in GCP?
Businesses are rapidly shifting to cloud-first strategies to save on infrastructure costs and improve scalability. With Google Cloud (GCP), companies can streamline their operations, store vast amounts of data, and deploy machine learning models at scale.
If you're an entrepreneur or part of a business team, having GCP-certified professionals within your organization can help you leverage Google’s powerful cloud ecosystem. Not only can it improve your business’s agility, but it also gives you a competitive edge in today’s fast-paced, tech-driven world.
Conclusion: Take the Leap with Google Cloud (GCP) MasterClass
Whether you’re new to cloud computing or looking to upgrade your cloud skills, the Google Cloud (GCP) MasterClass is the perfect course to take. You’ll learn everything from cloud basics to advanced data analytics and machine learning, all while gaining practical experience with real-world projects.
By the end of the course, you'll be fully prepared to pursue a Google Cloud certification and dive into a high-paying career in cloud computing. If you're ready to transform your future, Google Cloud (GCP) is waiting for you!
Start your journey today and join the ranks of GCP-certified professionals who are leading the charge in today’s digital transformation. Don’t miss out on this opportunity to elevate your career with the Google Cloud (GCP) MasterClass!
0 notes
Text
Google Data Studio: The Game-Changer in search engine optimization Tracking
Introduction
In the ever-evolving international of search engine optimisation (website positioning), staying forward of the game Weraddicted.com is valuable. As search engine marketing mavens, we are continuously in search of approaches to enhance our tactics, monitor our development, and analyze statistics to make recommended judgements. This is the place Google Data Studio comes into play. With its strong beneficial properties and consumer-pleasant interface, Google Data Studio has revolutionized the means we monitor and look at search engine optimisation overall performance. In this text, we'll explore how Google Data Studio is replacing the sport in search engine marketing monitoring and focus on its merits, sophisticated tactics, and absolute best practices.
Google Data Studio: The Basics
Before diving into the complex functions and concepts of Google Data Studio, enable's start with the basics. Google Data Studio is a free records visualization tool that allows for you to create customizable studies and dashboards the usage of archives from numerous assets reminiscent of Google Analytics, Google Ads, and extra. With its drag-and-drop interface, you'll be able to easily create interactive charts, graphs, and tables to visualise your info.
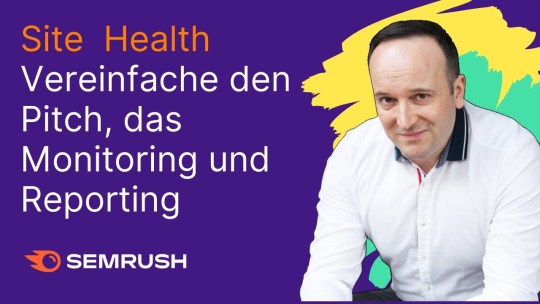
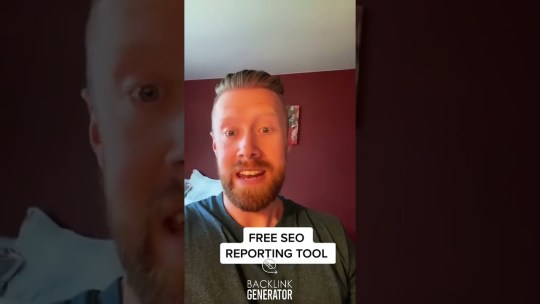
How to Get Started with Google Data Studio?
Getting began with Google Data Studio is understated. All you need is a Google account to get entry to this successful instrument. Once you've gotten logged in, you'll be able to attach your tips assets by means of clicking at the "Create" button and making a choice on "Data Source." From there, you might pick out from a large quantity of connectors plausible or create a custom connector simply by the Google Sheets API.
Why Should You Use Google Data Studio for web optimization Tracking?
Google Data Studio presents countless advantages over basic tactics of website positioning tracking. Here are a few key purposes why you should always take into consideration through it:
youtube
Data Visualization for search engine marketing: Visualizing your search engine marketing info is fundamental for figuring out trends, deciding upon styles, and spotting alternatives for advantage. With its huge wide variety of visualization concepts, Google Data Studio makes it clean to understand not easy info units at a look.
youtube
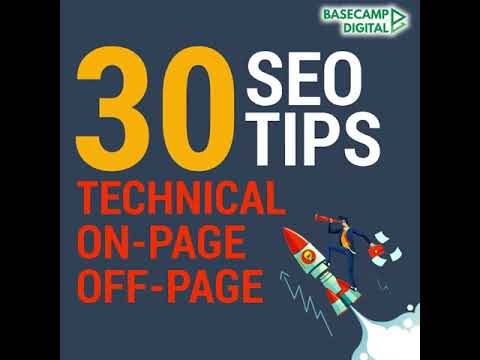
Advanced search engine optimisation Reporting Tools: Google Data Studio delivers complicated reporting resources that mean you can create dynamic and interactive reviews. You can personalize your studies with filters, date levels, and segments to point of interest on one-of-a-kind metrics or dimensions.
Improved search engine marketing Reporting Accuracy: By connecting straight away to your data assets, Google Data Studio guarantees true-time and properly reporting. Say so long to guide info exports and updates!
Integration with Other Google Tools: As a part of the Google Marketing Platform, Google Data Studio seamlessly integrates with different equipment akin to Google Analytics, Google Ads, and BigQuery. This integration allows you to mix facts from varied assets right into a single report for complete evaluation.
Using Google Data Studio for search engine marketing Tracking
Now that we have in mind the fundamentals and merits of riding Google Data Studio for se
0 notes
Text
Which data analysis tool is best for handling large datasets?
When handling large datasets, several data analysis tools stand out, each offering unique features tailored to different needs. Apache Hadoop is a popular choice for distributed data processing, allowing for the storage and computation of large volumes of data across clusters. It’s ideal for batch processing and handling massive amounts of unstructured data. Apache Spark, known for its speed and versatility, improves on Hadoop’s limitations by offering in-memory processing, making it suitable for real-time data analysis. Spark also supports various data sources and formats, making it a flexible option.
For those who prefer a more interactive environment, Python with libraries like Pandas and Dask can handle large datasets efficiently. While Pandas is excellent for smaller data, Dask extends its capabilities to large, distributed data with minimal code changes.
Another robust tool is SQL-based solutions like Google BigQuery or Amazon Redshift, which are cloud-based and optimized for large-scale data querying and analysis. These tools offer scalability and speed, making them perfect for businesses managing growing data needs.
Professionals looking to enhance their knowledge and skills in using these tools can benefit from data analytics certification courses, which provide hands-on experience and theoretical insights into modern data analysis practices.
0 notes
Text
Google Cloud Platform: Empowering Innovation in the Digital Age
In today's rapidly evolving digital landscape, organizations are seeking robust and scalable solutions to meet their growing needs. Google Cloud Platform (GCP) has emerged as a leading cloud computing service, empowering businesses of all sizes to innovate and stay competitive. From startups to multinational corporations, GCP offers a suite of tools and services designed to accelerate growth, enhance security, and optimize operations.
What is Google Cloud Platform?
Google Cloud Platform is a comprehensive cloud computing service that provides a range of solutions including computing power, storage, data analytics, machine learning, and networking. Built on Google’s infrastructure, GCP is designed to be flexible, reliable, and scalable, making it an ideal choice for a wide variety of applications. Whether you're developing a web application, managing large data sets, or deploying machine learning models, GCP offers the resources needed to bring your ideas to life.
Key Features of Google Cloud Platform
Scalability and Flexibility GCP provides scalable solutions that grow with your business. From virtual machines to Kubernetes clusters, GCP’s infrastructure can handle workloads of any size. With the ability to scale up or down based on demand, you can ensure optimal performance and cost-efficiency.
Advanced Data Analytics With tools like BigQuery, Google Cloud offers powerful data analytics capabilities. Businesses can analyze massive datasets in real time, uncovering insights that drive informed decision-making. GCP's data analytics tools integrate seamlessly with other services, enabling comprehensive data management and analysis.
Machine Learning and AI GCP’s AI and machine learning services, such as TensorFlow and AutoML, allow businesses to build and deploy intelligent applications. Whether you’re developing a custom AI model or using pre-trained models, GCP provides the resources to innovate and stay ahead in the AI revolution.
Security and Compliance Security is a top priority for Google Cloud. GCP offers a range of security features, including encryption, identity management, and threat detection. With compliance certifications across various industries, GCP ensures that your data is protected and meets regulatory requirements.
Global Network Google’s global network infrastructure ensures that GCP services are fast and reliable. With data centers in multiple regions, GCP provides low-latency access to your applications and data, no matter where your users are located.
Why Choose Google Cloud Platform?
Cost Efficiency GCP offers a pay-as-you-go pricing model, ensuring that you only pay for the resources you use. With sustained use discounts and custom machine types, you can optimize costs without sacrificing performance.
Innovation at Scale Google Cloud is at the forefront of cloud innovation, continuously developing new tools and services. By choosing GCP, you gain access to the latest technologies, enabling your business to innovate and grow.
Seamless Integration GCP integrates easily with other Google services, such as Google Workspace and Android. This seamless integration allows businesses to build comprehensive solutions that leverage Google’s ecosystem.
Support and Community Google Cloud offers robust support options, including 24/7 customer support and a vibrant community of developers and experts. Whether you need help with a specific issue or want to learn more about best practices, GCP’s support network is there to assist you.
Conclusion
Google Cloud Platform is more than just a cloud service; it's a powerful tool for digital transformation. With its wide array of services, robust security, and innovative technologies, GCP empowers businesses to achieve their goals and stay competitive in the digital age. Whether you're looking to scale your infrastructure, harness the power of AI, or secure your data, Google Cloud Platform offers the solutions you need to succeed.
0 notes
Text
How to Pass the Google Cloud Architect Certification Exam
Achieving the Google Cloud Architect Certification is a significant milestone for any IT professional looking to advance their career in cloud computing. This certification validates your expertise in designing, planning, and managing secure, robust, and scalable cloud solutions using Google Cloud Platform (GCP). Here’s a comprehensive guide to help you pass the Google Cloud Architect Certification exam.

Understanding the Exam Structure
Before diving into preparation, it’s crucial to understand the exam structure. The Google Cloud Architect Certification test evaluates your proficiency in the following areas:
Designing and planning a cloud solution architecture
Managing and provisioning cloud infrastructure
Designing for security and compliance
Analyzing and optimizing technical and business processes
Managing implementations of cloud architecture
Ensuring solution and operations reliability
The exam consists of multiple-choice and multiple-select questions, with a time limit of 2 hours. It will be easier for you to concentrate on your studies if you are familiar with these areas.
Enroll in a Professional Course
One of the best ways to prepare is by enrolling in a professional course. Several online platforms offer comprehensive courses designed specifically for the Google Cloud Architect Certification. These courses cover all exam topics and provide hands-on labs, practice tests, and study materials. Some popular options include Coursera, Udacity, and Google Cloud’s own training platform.
Utilize Official Study Resources
Google Cloud provides official study guides, documentation, and learning paths that are invaluable for your preparation. Moreover, the official Google Cloud Architect Exam Guide is a great starting point, as it outlines the key areas you need to focus on. To learn more, have a look at the case studies, whitepapers, and interactive labs offered by Google Cloud.
Gain Practical Experience
Hands-on experience with the Google Cloud Platform is crucial. Set up your own projects and experiment with various GCP services like Compute Engine, Cloud Storage, BigQuery, and Cloud IAM. Practical knowledge not only helps you grasp theoretical concepts but also boosts your confidence in tackling real-world scenarios presented in the exam.
Join Study Groups and Forums
Participating in online forums and study groups might offer more resources and help. Platforms like Reddit, LinkedIn, and Google Cloud’s community forums are excellent places to connect with fellow aspirants, share study materials, and discuss challenging topics. Moreover, engaging in these communities can also provide insights into exam experiences and tips from those who have already passed.
Practice with Mock Exams
Taking mock exams is one of the most effective ways to prepare for the real test. Mock exams simulate the exam environment and help you identify your strengths and weaknesses. Moreover, google Cloud’s official practice exams and other third-party resources offer numerous practice questions that closely resemble the actual exam.

Review and Revise
Allocate the last few weeks of your preparation to review and revise key concepts. Moreover, focus on areas where you feel less confident and revisit the official study materials and practice tests. Creating a study schedule that covers all domains will ensure a thorough revision.
Conclusion:
Passing the Google Cloud Architect Certification exam and Google Certified Professional Cloud Architect course requires a strategic approach, combining professional courses, practical experience, and consistent practice. By understanding the exam structure, utilizing official resources, gaining hands-on experience, and engaging with study communities, you can confidently prepare for and pass the exam. Therefore this certification not only enhances your knowledge and skills but also opens up new career opportunities in the rapidly growing field of cloud computing.
#certzip#education#usa#best google cloud architect certification#google certified professional cloud architect#architect certification training
0 notes
Text
How Can Beginners Start Their Data Engineering Interview Prep Effectively?
Embarking on the journey to become a data engineer can be both exciting and daunting, especially when it comes to preparing for interviews. As a beginner, knowing where to start can make a significant difference in your success. Here’s a comprehensive guide on how to kickstart your data engineering interview prep effectively.
1. Understand the Role and Responsibilities
Before diving into preparation, it’s crucial to understand what the role of a data engineer entails. Research the typical responsibilities, required skills, and common tools used in the industry. This foundational knowledge will guide your preparation and help you focus on relevant areas.
2. Build a Strong Foundation in Key Concepts
To excel in data engineering interviews, you need a solid grasp of key concepts. Focus on the following areas:
Programming: Proficiency in languages such as Python, Java, or Scala is essential.
SQL: Strong SQL skills are crucial for data manipulation and querying.
Data Structures and Algorithms: Understanding these fundamentals will help in solving complex problems.
Databases: Learn about relational databases (e.g., MySQL, PostgreSQL) and NoSQL databases (e.g., MongoDB, Cassandra).
ETL Processes: Understand Extract, Transform, Load processes and tools like Apache NiFi, Talend, or Informatica.
3. Utilize Quality Study Resources
Leverage high-quality study materials to streamline your preparation. Books, online courses, and tutorials are excellent resources. Additionally, consider enrolling in specialized programs like the Data Engineering Interview Prep Course offered by Interview Kickstart. These courses provide structured learning paths and cover essential topics comprehensively.
4. Practice with Real-World Problems
Hands-on practice is vital for mastering data engineering concepts. Work on real-world projects and problems to gain practical experience. Websites like LeetCode, HackerRank, and GitHub offer numerous challenges and projects to work on. This practice will also help you build a portfolio that can impress potential employers.
5. Master Data Engineering Tools
Familiarize yourself with the tools commonly used in data engineering roles:
Big Data Technologies: Learn about Hadoop, Spark, and Kafka.
Cloud Platforms: Gain experience with cloud services like AWS, Google Cloud, or Azure.
Data Warehousing: Understand how to use tools like Amazon Redshift, Google BigQuery, or Snowflake.
6. Join a Study Group or Community
Joining a study group or community can provide motivation, support, and valuable insights. Participate in forums, attend meetups, and engage with others preparing for data engineering interviews. This network can offer guidance, share resources, and help you stay accountable.
7. Prepare for Behavioral and Technical Interviews
In addition to technical skills, you’ll need to prepare for behavioral interviews. Practice answering common behavioral questions and learn how to articulate your experiences and problem-solving approach effectively. Mock interviews can be particularly beneficial in building confidence and improving your interview performance.
8. Stay Updated with Industry Trends
The field of data engineering is constantly evolving. Stay updated with the latest industry trends, tools, and best practices by following relevant blogs, subscribing to newsletters, and attending webinars. This knowledge will not only help you during interviews but also in your overall career growth.
9. Seek Feedback and Iterate
Regularly seek feedback on your preparation progress. Use mock interviews, peer reviews, and mentor guidance to identify areas for improvement. Continuously iterate on your preparation strategy based on the feedback received.
Conclusion
Starting your data engineering interview prep as a beginner may seem overwhelming, but with a structured approach, it’s entirely achievable. Focus on building a strong foundation, utilizing quality resources, practicing hands-on, and staying engaged with the community. By following these steps, you’ll be well on your way to acing your data engineering interviews and securing your dream job.
#jobs#coding#python#programming#artificial intelligence#education#success#career#data scientist#data science
0 notes
Text
BigQuery Studio From Google Cloud Accelerates AI operations

Google Cloud is well positioned to provide enterprises with a unified, intelligent, open, and secure data and AI cloud. Dataproc, Dataflow, BigQuery, BigLake, and Vertex AI are used by thousands of clients in many industries across the globe for data-to-AI operations. From data intake and preparation to analysis, exploration, and visualization to ML training and inference, it presents BigQuery Studio, a unified, collaborative workspace for Google Cloud’s data analytics suite that speeds up data to AI workflows. It enables data professionals to:
Utilize BigQuery’s built-in SQL, Python, Spark, or natural language capabilities to leverage code assets across Vertex AI and other products for specific workflows.
Improve cooperation by applying best practices for software development, like CI/CD, version history, and source control, to data assets.
Enforce security standards consistently and obtain governance insights within BigQuery by using data lineage, profiling, and quality.
The following features of BigQuery Studio assist you in finding, examining, and drawing conclusions from data in BigQuery:
Code completion, query validation, and byte processing estimation are all features of this powerful SQL editor.
Colab Enterprise-built embedded Python notebooks. Notebooks come with built-in support for BigQuery DataFrames and one-click Python development runtimes.
You can create stored Python procedures for Apache Spark using this PySpark editor.
Dataform-based asset management and version history for code assets, including notebooks and stored queries.
Gemini generative AI (Preview)-based assistive code creation in notebooks and the SQL editor.
Dataplex includes for data profiling, data quality checks, and data discovery.
The option to view work history by project or by user.
The capability of exporting stored query results for use in other programs and analyzing them by linking to other tools like Looker and Google Sheets.
Follow the guidelines under Enable BigQuery Studio for Asset Management to get started with BigQuery Studio. The following APIs are made possible by this process:
To use Python functions in your project, you must have access to the Compute Engine API.
Code assets, such as notebook files, must be stored via the Dataform API.
In order to run Colab Enterprise Python notebooks in BigQuery, the Vertex AI API is necessary.
Single interface for all data teams
Analytics experts must use various connectors for data intake, switch between coding languages, and transfer data assets between systems due to disparate technologies, which results in inconsistent experiences. The time-to-value of an organization’s data and AI initiatives is greatly impacted by this.
By providing an end-to-end analytics experience on a single, specially designed platform, BigQuery Studio tackles these issues. Data engineers, data analysts, and data scientists can complete end-to-end tasks like data ingestion, pipeline creation, and predictive analytics using the coding language of their choice with its integrated workspace, which consists of a notebook interface and SQL (powered by Colab Enterprise, which is in preview right now).
For instance, data scientists and other analytics users can now analyze and explore data at the petabyte scale using Python within BigQuery in the well-known Colab notebook environment. The notebook environment of BigQuery Studio facilitates data querying and transformation, autocompletion of datasets and columns, and browsing of datasets and schema. Additionally, Vertex AI offers access to the same Colab Enterprise notebook for machine learning operations including MLOps, deployment, and model training and customisation.
Additionally, BigQuery Studio offers a single pane of glass for working with structured, semi-structured, and unstructured data of all types across cloud environments like Google Cloud, AWS, and Azure by utilizing BigLake, which has built-in support for Apache Parquet, Delta Lake, and Apache Iceberg.
One of the top platforms for commerce, Shopify, has been investigating how BigQuery Studio may enhance its current BigQuery environment.
Maximize productivity and collaboration
By extending software development best practices like CI/CD, version history, and source control to analytics assets like SQL scripts, Python scripts, notebooks, and SQL pipelines, BigQuery Studio enhances cooperation among data practitioners. To ensure that their code is always up to date, users will also have the ability to safely link to their preferred external code repositories.
BigQuery Studio not only facilitates human collaborations but also offers an AI-powered collaborator for coding help and contextual discussion. BigQuery’s Duet AI can automatically recommend functions and code blocks for Python and SQL based on the context of each user and their data. The new chat interface eliminates the need for trial and error and document searching by allowing data practitioners to receive specialized real-time help on specific tasks using natural language.
Unified security and governance
By assisting users in comprehending data, recognizing quality concerns, and diagnosing difficulties, BigQuery Studio enables enterprises to extract reliable insights from reliable data. To assist guarantee that data is accurate, dependable, and of high quality, data practitioners can profile data, manage data lineage, and implement data-quality constraints. BigQuery Studio will reveal tailored metadata insights later this year, such as dataset summaries or suggestions for further investigation.
Additionally, by eliminating the need to copy, move, or exchange data outside of BigQuery for sophisticated workflows, BigQuery Studio enables administrators to consistently enforce security standards for data assets. Policies are enforced for fine-grained security with unified credential management across BigQuery and Vertex AI, eliminating the need to handle extra external connections or service accounts. For instance, Vertex AI’s core models for image, video, text, and language translations may now be used by data analysts for tasks like sentiment analysis and entity discovery over BigQuery data using straightforward SQL in BigQuery, eliminating the need to share data with outside services.
Read more on Govindhtech.com
#BigQueryStudio#BigLake#AIcloud#VertexAI#BigQueryDataFrames#generativeAI#ApacheSpark#MLOps#news#technews#technology#technologynews#technologytrends#govindhtech
0 notes
Text
Chipsy.io Backend Development: Unleashing the Power of Modern Technology
In the fast-evolving world of technology, businesses need robust, scalable, and secure backend systems to support their digital transformation. At Chipsy.io, we specialize in backend development, harnessing the power of cutting-edge technologies to build systems that drive your business forward.
Key Technologies
AWS: Leveraging Amazon Web Services (AWS), we provide scalable and flexible solutions that meet the demands of your business. From EC2 instances to Lambda functions, our expertise ensures your applications run smoothly and efficiently.
Azure: With Microsoft Azure, we deliver enterprise-grade solutions that integrate seamlessly with your existing infrastructure. Our services include everything from Azure App Services to Azure Functions, enabling rapid development and deployment.
Google Cloud Platform (GCP): Utilizing the power of GCP, we build highly scalable and resilient backend systems. Our capabilities include using Google Kubernetes Engine (GKE) for container orchestration and BigQuery for real-time analytics.
Best Practices
At Chipsy.io, we adhere to industry best practices to ensure the quality and reliability of our backend systems:
Microservices Architecture: We design our systems using a microservices architecture, allowing for independent development, deployment, and scaling of each service.
Continuous Integration/Continuous Deployment (CI/CD): Our CI/CD pipelines automate the testing and deployment process, ensuring rapid and reliable releases.
Security: We implement robust security measures, including data encryption, secure APIs, and regular security audits, to protect your sensitive information.
Monitoring and Logging: Our systems include comprehensive monitoring and logging solutions, providing real-time insights and facilitating quick issue resolution.
Future Trends
We stay ahead of the curve by continuously exploring emerging trends and technologies:
Serverless Computing: Our expertise in serverless architectures allows for building highly scalable applications without the need for server management.
Artificial Intelligence and Machine Learning: We integrate AI and ML capabilities into backend systems to provide advanced analytics and automation.
Edge Computing: By processing data closer to the source, we reduce latency and improve performance, especially for IoT applications.
Why Choose Chipsy.io?
Partnering with Chipsy.io for your backend development needs means gaining access to a team of experts dedicated to delivering high-quality, future-proof solutions. Our commitment to excellence and innovation ensures your business stays competitive in a digital-first world.
Ready to transform your backend systems? Contact Chipsy.io today and let us help you unleash the power of modern technology.
#backend development#aws#microsoft azure#mobile app design#artificial intelligence#machinelearning#google cloud platform#google cloud services
0 notes
Text
You Need to Know About Modern Data Warehousing in 2024
In 2024, modern data warehousing leverages cloud-based platforms for scalability, agility, and cost-efficiency. Technologies like Snowflake, Google BigQuery, and Amazon Redshift dominate, offering seamless integration with data lakes, real-time analytics, and AI/ML capabilities. Data warehousing now emphasizes schema-on-read flexibility, enabling rapid adaptation to evolving business needs and diverse data sources. Automated data pipelines and serverless computing further streamline operations, reducing maintenance overhead. Security and compliance remain paramount, with robust encryption and governance features. Overall, modern data warehousing empowers organizations to harness vast data volumes for actionable insights, driving innovation and competitive advantage in today's data-driven landscape. Read more:
0 notes
Text
Oracle to GCP PostgreSQL Migration Strategies With Newt Global Expertise
When you Migrate Oracle to GCP PostgreSQL, it's crucial to understand the differences between the two database systems. PostgreSQL offers an open-source environment with a rich set of features and active community support, making it an attractive alternative to Oracle. During the migration, tools like Google Cloud Database Migration Service and Ora2Pg can help automate schema conversion and data transfer, ensuring a seamless transition. Plan for optimization and tuning in the new environment to achieve optimal performance. This blog explores best practices, the advantages of this migration, and how it positions businesses for ongoing success. Seamless Oracle to GCP PostgreSQL Migration When planning to Migrate Oracle to GCP PostgreSQL, careful planning and consideration are fundamental to guarantee a successful transition. First, conduct a comprehensive assessment of your current Oracle database environment. Identify the data, applications, and dependencies that need to be migrated. This assessment helps in understanding the complexity and scope of the migration. Next, focus on schema compatibility. Oracle and PostgreSQL have diverse data types and functionalities, so it's vital to map Oracle data types to their PostgreSQL equivalents and address any incompatibilities. Another critical consideration is the volume of data to be migrated. Large datasets may require more time and resources, so plan accordingly to minimize downtime and disruption. Choosing the right migration tools is also important. Tools like Google Cloud Database Migration Service, Ora2Pg, and pgLoader can automate and streamline the migration process, ensuring data integrity and consistency. Additionally, consider the need for thorough testing and validation post-migration to ensure the new PostgreSQL database functions correctly and meets performance expectations. Finally, plan for optimization and tuning in the new environment to achieve optimal performance. By addressing these key considerations, organizations can effectively manage the complexities of migrating Oracle to GCP PostgreSQL. Best Practices for Future-Proofing Your Migration
1. Incremental Migration: Consider migrating in phases, Begin with non-critical data. This approach minimizes risk and allows for easier troubleshooting and adjustments. 2. Comprehensive Documentation: Maintain detailed documentation of the migration process, including configuration settings, scripts used, and any issues encountered. 3. Continuous Monitoring and Maintenance: Implement robust monitoring tools to track database execution, resource utilization, and potential issues. Schedule regular maintenance tasks such as updating statistics and vacuuming tables.
GCP PostgreSQL: Powering Growth through Seamless Integration Migrating to GCP PostgreSQL offers several key advantages that make it a forward-looking choice:
Open-Source Innovation: PostgreSQL is a leading open-source database known for its robustness, feature richness, and active community. It continuously evolves with new features and advancements.
Integration with Google Ecosystem: GCP PostgreSQL integrates seamlessly with other Google Cloud services, such as BigQuery for analytics, AI and machine learning tools, and Kubernetes for container orchestration.
Cost Management: This model helps in better cost management and budgeting compared to traditional on-premises solutions.
Evolving with GCP PostgreSQL- Strategic Migration Insights with Newt Global In conclusion, migrating Oracle to GCP PostgreSQL represents a strategic evolution for businesses, facilitated by the expertise and partnership opportunities with Newt Global. This transition goes beyond mere database migration. Post-migration, thorough testing, and continuous optimization are crucial to fully leverage PostgreSQL's capabilities and ensure high performance. By migrating Oracle to GCP PostgreSQL, organizations can reduce the total cost of ownership and benefit from an open-source environment that integrates smoothly with other Google Cloud services. This positions businesses to better respond to market demands, drive innovation, and achieve greater operational efficiency. Ultimately, migrating to GCP PostgreSQL not only addresses current database needs but also sets the foundation for future growth and technological advancement in an increasingly digital world. Ultimately, migrating Oracle to GCP PostgreSQL through Newt Global signifies a proactive approach to staying competitive, driving growth, and achieving sustained success in the digital world. Thanks For Reading
For More Information, Visit Our Website: https://newtglobal.com/
0 notes
Text
Data Engineer Training in Hyderabad
Data Engineering Training in Hyderabad by RS Trainings: Learn from Industry IT Experts
Introduction to Data Engineering
Data Engineering is the backbone of modern data-driven organizations, focusing on the collection, storage, and processing of massive datasets. It involves the design, construction, and maintenance of scalable data infrastructures and pipelines, enabling the seamless flow of data across various systems. With the explosion of big data, skilled data engineers are in high demand, making data engineering one of the most sought-after professions in the IT industry.
Why Choose RS Trainings for Data Engineering Training?
RS Trainings in Hyderabad is a premier institute for learning Data Engineering. Here are several reasons why RS Trainings is considered the best place for data engineering training:
Expert Instructors: RS Trainings boasts a team of highly experienced instructors who are seasoned industry professionals. They bring a wealth of real-world knowledge and practical experience, ensuring that you learn the most current and applicable skills.
Comprehensive Curriculum: The Data Engineering course at RS Trainings covers all essential topics, from foundational concepts to advanced techniques. The curriculum is designed to equip you with the skills needed to excel in the data engineering field.
Hands-On Experience: The program emphasizes practical, hands-on learning. You'll work on real-world projects and use industry-standard tools and technologies, gaining the practical experience necessary to succeed in the job market.
Flexible Learning Options: RS Trainings understands the diverse needs of its learners and offers flexible learning schedules, including weekend and evening batches, as well as online training options for remote learners.
Post-Training Support: RS Trainings provides robust post-training support, including access to a network of professionals, additional learning resources, and job placement assistance.
Course Content
The Data Engineering training at RS Trainings is meticulously designed to ensure a comprehensive learning experience. Here’s an outline of the topics covered:
1. Introduction to Data Engineering
Overview of Data Engineering
Importance in Modern Data Ecosystems
Roles and Responsibilities of a Data Engineer
2. Data Modeling and Database Design
Fundamentals of Data Modeling
Relational and Non-Relational Databases
Designing Scalable Data Architectures
3. ETL (Extract, Transform, Load) Processes
Understanding ETL Pipelines
Data Extraction from Various Sources
Data Transformation Techniques
Data Loading into Data Warehouses
4. Big Data Technologies
Introduction to Big Data
Apache Hadoop Ecosystem
Apache Spark for Data Processing
5. Data Warehousing Solutions
Designing Data Warehouses
Implementing Data Lakes
Using Cloud Data Warehousing Solutions (e.g., AWS Redshift, Google BigQuery)
6. Data Pipelines and Workflow Orchestration
Building Data Pipelines
Workflow Orchestration with Apache Airflow
Managing and Monitoring Data Workflows
7. Data Security and Governance
Data Privacy and Compliance
Implementing Data Security Measures
Data Governance Best Practices
8. Real-World Applications and Projects
Data Integration Projects
Real-Time Data Processing
Data Quality and Validation
End-to-End Data Engineering Solutions
Benefits of Data Engineering Training at RS Trainings
Career Advancement: Acquiring skills in data engineering opens up a wide array of career opportunities in data-centric roles such as Data Engineer, Data Architect, and Data Analyst.
Industry-Relevant Skills: The training ensures you are up-to-date with the latest technologies and methodologies used in the industry, making you job-ready from day one.
Networking Opportunities: Training with industry experts and peers provides valuable networking opportunities that can be beneficial for your career growth.
Conclusion
If you're looking to build a career in data engineering and want to gain cutting-edge skills from the best in the industry, RS Trainings in Hyderabad is the ideal place to start. With expert instructors, a comprehensive and practical curriculum, and excellent post-training support, RS Trainings ensures you are well-equipped to meet the demands of the data engineering profession. Enroll today and embark on your journey to becoming a proficient data engineer with RS Trainings.
#data engineer training#data engineer training online#data engineer online training#data engineer training institute in Hyderabad#data engineering training with placement
0 notes
Text
Your Guide to GCP Certification Training Courses: "Mastering the Cloud"
In today's dynamic digital environment, cloud computing has become an essential element for companies looking for flexibility, scalability, and creativity. Google Cloud Platform (GCP), one of the top suppliers in this field, offers a range of effective tools and services to fully utilize the cloud. As more and more companies move their operations to the cloud, there is a growing need for qualified individuals who are knowledgeable about, gcp courses can help with this by giving people the knowledge and certifications they need to succeed in this fast-paced industry.
Unlocking Google Cloud Platform's Potential
A complete cloud computing platform, Google Cloud Platform (GCP) provides a multitude of services for data analytics, machine learning, computing, storage, and other areas. Using Google's extensive global infrastructure and state-of-the-art technology, GCP enables companies of all sizes to develop, launch, and grow applications with ease.
Compute Engine, Kubernetes Engine, BigQuery, TensorFlow, and other services from GCP give professionals the tools they need to take on challenging projects and spur innovation in their fields. GCP offers the essential components for success, whether it comes to developing scalable online apps, carrying out sophisticated data analysis, or putting machine learning models into practice.
GCP Certification's Significance
Having a GCP certification will greatly improve your professional prospects in today's cutthroat job market. GCP certificates attest to your proficiency in cloud computing and certify your ability to design, build, and manage solutions on Google Cloud Platform.
Google provides a variety of certification tests suited to various positions and skill levels, such as:
For those with practical experience installing, monitoring, and maintaining projects on GCP, the Associate Cloud Engineer position is ideal.
Professional Cloud Architect: Designed for seasoned cloud workers in charge of creating and overseeing highly available, secure, and scalable systems on GCP.
Professional Data Engineer: Designed for professionals with a background in data engineering who can show that they can plan, develop, and implement analytics solutions and data processing systems on GCP.
Professional Machine Learning Engineer: Targeting experts in data science and machine learning, this program highlights their prowess in developing and implementing machine learning models on GCP.
Starting Your Path to GCP Certification
Although earning a GCP certification has many advantages, passing these tests needs commitment, knowledge, and in-depth training. In order to succeed in GCP environments, prospective professionals need to have the knowledge, abilities, and practical experience that GCP Certification Training Courses offer.
A top-notch GCP certification training program addresses a broad range of subjects in line with the goals of the test, such as:
Knowing the basic features and services offered by GCP
Deploying and overseeing containers and virtual machines
Constructing dependable and scalable architectures
Putting data processing and storage systems into place
Protecting GCP networks and resources
Creating and implementing models for machine learning
Maximizing GCP's cost control and performance
To reinforce learning and get students ready for the demands of certification tests, these courses frequently incorporate practical labs, real-world projects, and practice exams.
Selecting the Appropriate GCP Certification Course
Choosing the best GCP certification training course might be difficult given the abundance of options available. Take into account the following elements when assessing courses:
Content Quality: Verify that the course covers all pertinent material and complies with the exam's requirements.
Instructor Expertise: Seek out classes instructed by licensed instructors or GCP experts with a lot of experience.
Practical Experience: To enhance learning, choose courses that include hands-on labs and real-world projects.
Flexibility: Select a course mode (instructor-led, self-paced, etc.) based on your learning style and schedule.
Student Testimonials and Achievements: To evaluate the efficacy and applicability of the course, read through reviews and testimonies from previous participants.
In summary
To sum up, gcp cloud training Courses provide a platform for people to become experts on Google Cloud Platform and verify their knowledge with certificates accepted by the industry. In the current digital environment, professionals may promote creativity, scalability, and efficiency by utilizing the extensive tools and services offered by GCP. GCP certification training courses give you the tools and direction you need to be successful in this fast-paced industry, regardless of how experienced you are with cloud computing. With GCP certification training, you can maximize your cloud computing capabilities and advance your career to new heights.
0 notes
Text
Navigating the Cloud: Specialized Cloud Computing Courses in Bangalore
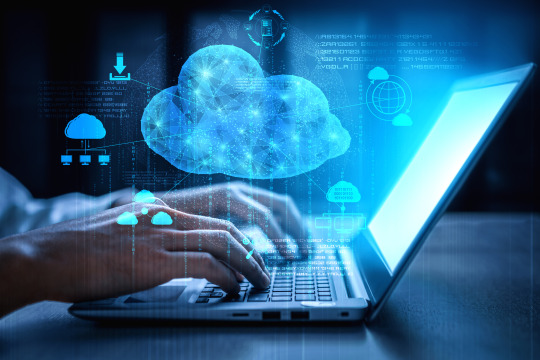
Bangalore, known as the Silicon Valley of India, is at the forefront of technological innovation and is home to a thriving ecosystem of tech companies, startups, and educational institutions. With the increasing adoption of cloud computing technologies across industries, there is a growing demand for skilled professionals who can design, deploy, and manage cloud-based solutions effectively. To meet this demand, several specialized cloud computing courses are available in Bangalore, offering in-depth training in emerging technologies, industry best practices, and practical skills development. In this article, we'll explore the specialized cloud computing courses available in Bangalore and how they equip students with the expertise needed to succeed in this dynamic field.
Advanced Cloud Architectures and Solutions: Advanced cloud computing courses in Bangalore focus on designing and implementing complex cloud architectures and solutions tailored to specific business needs. These courses delve into advanced topics such as multi-cloud and hybrid cloud deployments, microservices architecture, containerization (e.g., Docker, Kubernetes), serverless computing, and advanced networking and security concepts. Students learn how to leverage cutting-edge cloud technologies and best practices to architect scalable, resilient, and secure cloud-based solutions that meet the demands of modern enterprises.
DevOps and Continuous Integration/Continuous Deployment (CI/CD): DevOps has become integral to the software development lifecycle, facilitating collaboration between development and operations teams and enabling rapid and reliable software delivery. Specialized cloud computing courses in Bangalore focus on DevOps principles, practices, and tools, including version control systems (e.g., Git), automated testing, continuous integration/continuous deployment (CI/CD) pipelines, infrastructure as code (IaC), and configuration management tools (e.g., Ansible, Terraform). Students gain hands-on experience building, deploying, and managing cloud-native applications using DevOps methodologies and tools, preparing them for roles such as DevOps engineer, site reliability engineer (SRE), or cloud automation specialist.
Cloud Security and Compliance: With the increasing adoption of cloud computing, ensuring the security and compliance of cloud-based systems has become a top priority for organizations. Specialized cloud computing courses in Bangalore focus on cloud security fundamentals, threat detection and response, data encryption, identity and access management (IAM), compliance frameworks (e.g., GDPR, HIPAA), and cloud security best practices. Students learn how to design and implement robust security controls and measures to protect cloud-based assets and sensitive data, mitigate risks, and ensure compliance with industry regulations and standards.
Big Data Analytics and Machine Learning on the Cloud: Big data analytics and machine learning (ML) are transforming industries by enabling organizations to derive actionable insights, automate decision-making processes, and drive innovation. Specialized cloud computing courses in Bangalore cover topics such as big data processing frameworks (e.g., Apache Hadoop, Spark), distributed data storage and processing (e.g., Amazon S3, Google BigQuery), data visualization tools (e.g., Tableau, Power BI), and machine learning algorithms and techniques. Students learn how to leverage cloud-based platforms and services to build scalable, high-performance data analytics and ML solutions that unlock the value of big data and drive business growth.
Cloud-native Application Development: Cloud-native application development courses in Bangalore focus on building and deploying cloud-native applications that leverage cloud-native principles, architectures, and services. Students learn how to design, develop, deploy, and manage cloud-native applications using modern development frameworks (e.g., Spring Boot, Node.js), container orchestration platforms (e.g., Kubernetes), serverless computing services (e.g., AWS Lambda, Azure Functions), and cloud-native databases (e.g., Amazon DynamoDB, Google Cloud Firestore). These courses emphasize principles such as scalability, resilience, elasticity, and observability, preparing students for roles as cloud-native developers, architects, or engineers.
In conclusion, specialized cloud computing courses in Bangalore cater to the diverse needs and interests of students seeking to advance their careers in cloud computing and related fields. Whether you're interested in architecting complex cloud solutions, implementing DevOps practices, ensuring cloud security and compliance, leveraging big data analytics and ML, or building cloud-native applications, there are specialized courses available in Bangalore to help you acquire the skills and knowledge needed to succeed in this rapidly evolving industry. By enrolling in these courses, students can gain a competitive edge in the job market and contribute to the innovation and growth of the cloud computing ecosystem in Bangalore and beyond.
0 notes
Text
I've just finished Module 3 in the Data Engineering Zoomcamp #dezoomcamp. DWH fundamentals and BigQuery. External and materialized tables. BigQuery partitioning and clustering. Internals, architecture, performance, cost, and best practices. My progress is here: https://github.com/kirill505/data-engineering-zoomcamp/tree/main/03-data-warehouse
1 note
·
View note