#AlphaFold 3
Explore tagged Tumblr posts
Text
Why I Believe AlphaFold 3 is a Powerful Tool for the Future of Healthcare
Insights on a groundbreaking artificial intelligence tool for health sciences research Dear science and technology readers, Thanks for subscribing to Health Science Research By Dr Mike Broadly, where I curate important public health content. A few months ago, I wrote about AlphaFold 3, a groundbreaking AI tool that helps scientists understand protein structures, which are essential for…
#academic research#AI in healthcare#alphafold#AlphaFold 3#AlphaFold 3 represents a major leap in biological understanding#AlphaFold 3 to model proteins#AlphaFold Protein Structure Database#AlphaFold Server#Artificial Intelligence#Bioinformatics#biotechnology#Democratizing Science:#Drug discovery#future of artificial intelligence#future of science#future of technology#health#health science resarch#Health sciences research#Insights from Dr Michael Broadly#Isomorphic Labs#life lessons#Machine learning in medicine#Personalized medicine#Precision Predictions#protein resarch#Protein structure prediction#Real-World Applications#research#science
1 note
·
View note
Text
@hellsite-proteins
“Funny shapes”
“The analyses of each protein are hilarious even if you don’t know anything about proteins”
“AlphaFold is an incredibly powerful tool in bioinformatics and is a contender for the Nobel Prize in upcoming years, but is underappreciated by the general public. Seeing it put to use in such a silly and fun way is both refreshing and educational!”
“Turns posts into big squiggly lines :3”
@aroace-everyday
(no propaganda submitted)
178 notes
·
View notes
Note
i saw a talk by jonas adler from deepmind the other day about alphafold 3, unfortunately im far more of a biochemist than a computer understander but its definitely exciting. open source by the end of the year !
im also not much of a computer person but everything i've heard about it sounds awesome!
letter sequence in this ask matching protein-coding amino acids:
isawatalkynasadlerfrmdeepmindthetherdayatalphafldnfrtnatelyimfarmrefaichemistthanacmpternderstandertitsdefinitelyecitingpensrceytheendftheyear
protein guy analysis:
there is not too much making this protein notable compared to the rest of the structures on here. it has some secondary structure in the form of helices but there is no tertiary structure. the one distinguishing factor is the place where one helix seems to be interrupted with a bend going the opposite direction, before a new helix starts. (this is towards the back left of the image.) it is likely due to a proline residue, and facilitated by the small size of a nearby alanine. while it's not very productive to consider this structure specifically, this is an interesting demonstration of how different residues affect secondary structure formation!
predicted protein structure:
cartoon structure
residues between disconnected helices
#science#biochemistry#biology#chemistry#stem#proteins#protein structure#science side of tumblr#protein asks
15 notes
·
View notes
Text
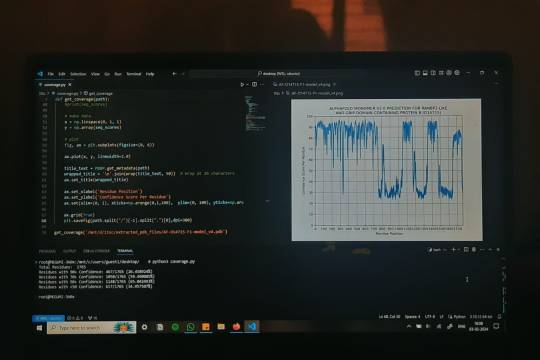
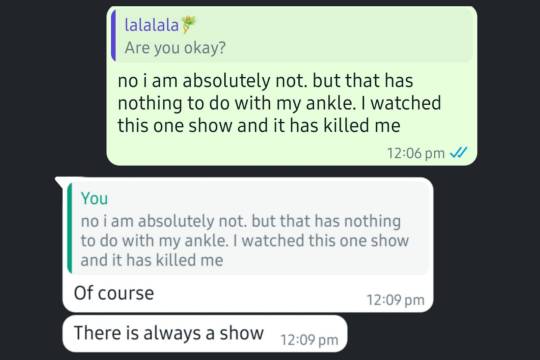
this week on megumi.fm ▸ the ankle incident
🏥 guess who twisted their ankle and ended up with a partial ligament tear and restrictions on mobility T-T I went to the doc and he gave me my meds and told me to sit my ass down for the whole month. so all I've been doing lately is throwing tantrums and zooming around the house in my trusty computer chair. it's gotten better oveer the days though, I can't wait until it fully recovers ahhhh :"
📋 Tasks
💻 Internship ↳ read papers on alphafold and it's coverage [3/3]✅ ↳ update tracker and alphafold notes ✅ ↳ complete ppt for lab presentation ✅ ↳ code to determine seq coverage depth of an AF.pdb file ✅ ↳ code to plot a per residue confidence of an AF.pdb file ✅ 🩺Radiomics Project ↳ call with PI to discuss about annotation limitations and radiomic feature selection✅ 📧 Application-related ↳ collected referee report from my profs ✅ ↳ submitted masters application for 1/1 Uni ✅
📅 Daily-s
🛌 consistent sleep [?/7] (i've been going to bed early enough but been in too much pain to actually sleep well) 💧 good water intake [7/7] 👟 exercise [/7] ankle recovery brrr
Fun Stuff this week
💗 my aunt and grandad came to visit! my cousin also showed up a couple days after, we spent a lot of time bickering with each other it was fun 💗 a completely different aunt and their family also came home this week for lunch! 📞 I've been on constant calls with a lot of my friends and talking to them. my besties and I also designed a cute little pinterest board for this story idea we have been discussing together [previous antics with said besties include painting mugs for christmas and getting hazelnut lattes for dinner] 📺 ongoing: Marry my Husband, Cherry Magic Th, Love for Love's Sake 📺 binged: My Man is Cupid, Our Dating Sim, Jun & Jun 📺 rewatch: Semantic Error 📊 on this note I also made a tier ranked list of all the asian tv (excluding anime/ghibli) I have consumed. it was fun xD 📹 This Serotonin Boost>> the leads from Love For Love's Sake being cute and clingy and affectionate with each other
📻 This week's soundtrack
Siren by Kailee Morgue All I really want is you, by the Marías
---
[Jan 29 to Feb 4 ; week 5/52 || not the most productive version of myself but given that I'm stuck cooped up in my room all day I've been doing the best I can. one of my uni applications is submitted! few more to go.
also. on a completely different note, Love for Love's Sake is such a good show?? i'm obsessed with the mechanics of the story universe and the cinematography and the narrative structure combined with the characters personalities and the parallels and that there's so much open to interpretation and. yea. it has changed me ]
#52wktracker#studyblr#study blog#studyspo#stemblr#stem student#study goals#student life#college student#studying#stem studyblr#adhd studyblr#adhd student#study motivation#100 days of productivity#study inspo#study inspiration#gradblr#uniblr#studyinspo#sciblr#study aesthetic#study blr#study motivator#100 days of self discipline#100 days of studying#stem academia#bio student#100 dop#100dop
28 notes
·
View notes
Link
DeepMind and Isomorphic Labs unveil AlphaFold 3, an AI model that predicts structures and interactions of all biomolecules like proteins, nucleic acids, ligands, and more. A protein structure predicting system, AlphaFold 2, launched in 2021, had an accuracy that was unprecedented before. With an updated diffusion-based architecture, AlphaFold 3 demonstrates significantly improved accuracy over specialized tools across protein-ligand, protein-nucleic acid, and antibody-antigen interactions. This unified deep learning framework enables high-precision modeling across the entire biomolecular space, ushering in new possibilities for structural biology.
AlphaFold 3 is powered by a deep learning system. This is AI that has been created in the likeness of the human brain both structurally and functionally. Consequently, for the construction of its deep learning model AlphaFold 3, a huge dataset was used; known protein structures were trained upon together with their corresponding amino acid sequences. Thus, it can define how individual units (amino acids) stack up into building cell blocks, forming any particular protein’s final three-dimensional shape.
Continue Reading
6 notes
·
View notes
Text
My First Blog
Today I briefly wrote about Alphafold 3 in my blogs. I plan to share more here. Here is the link to my blog post.
2 notes
·
View notes
Text
5 notes
·
View notes
Quote
Google DeepMindがAI系創薬企業のIsomorphic Labsと協力し、タンパク質やDNA、RNA、リガンドなど生命分子の構造と相互作用をきわめて正確に予測できるAIモデル「AlphaFold 3」をリリースしました。 Google DeepMind and Isomorphic Labs introduce AlphaFold 3 AI model https://blog.google/technology/ai/google-deepmind-isomorphic-alphafold-3-ai-model/ [snip] AlphaFold 3は新たに公開された研究ツールである「AlphaFold Server」を通じてアクセス可能で、非営利目的での利用であれば機能のほとんどを無料で使用可能。
Google DeepMindがすべての生命分子の構造と相互作用をきわめて正確に予測できるAIモデル「AlphaFold 3」を発表 - GIGAZINE
これが無料か。すごい時代になったもんだなー
2 notes
·
View notes
Note
also ayy fellow microbio buddy 🤝 what's your research on!
YEAH!!! 🤝 my research focuses on drug discovery for bacterial pathogens, specifically by 'disarming' the components that make them pathogens in the first place (i.e., not antibiotics).
the target protein and system i worked with are really specific, so i have to be vague for obvious reasons 😔 but my experimental research focused on neisseria gonorrhoeae! ish!
extra rambles under the cut - it got really REALLY long SORRY LOL
my research project was a two-parter with experimental and computational halves. basically - they're almost the same type of project, but one was virtual LOL. i say 'my research' in present tense because i continued on the 2nd project after graduating. we wrapped up very recently and are now... sitting on the manuscript. because of course. at least my supervisor is a wonderful person 😔
for the 1st project, we wanted to flesh out an inhibitory drug screening protocol that'd been developed by a previous student for a different organism. i spent a lot of time on bacterial transformations, protein purification, and assay optimization. a lot of time. our protein didn't want to cooperate until we switched model organisms 2 years into my program. then i spent too much time looking at 96-well plates. i have a picture somewhere of the 96-well plates surrounding my bench like a castle fortress. i know too much about e. coli lactose fermentation at this point. i can mix malachite green in my sleep. this was my personal hell for 3 years.
my 2nd project focused on molecular dynamic simulations and computational drug screening for the same system. a few groups have tried this before (AND GOT PUBLISHED IN HIGH IMPACT JOURNALS BUT ANYWAYS), but none of them were... good at thoughtfully and purposefully drug screening, much less simulating a complex protein.
basically, we made a 3D model of our protein and simulated how it would act under several artificial (but biologically relevant) conditions. afterwards, we feed the 3D models into a virtual screening workflow to predict if compounds have drug potential against the protein.
we started the simulations just before alphafold 2 made de novo protein folding popular, so the protein model isn't sexy but it's FINE and at least passed QA. such is life I GUESS 😑
anyways, after crying over 75 GB of sim data, i did the subsequent screening locally on a spare PC. and dear god, i killed that PC. it made my office hotter than hell for 4 days straight. but it was also sick as fuck!!!!
a lot of this project was parsing data while waiting for things to run for days at a time, but now we have leads on what might potentially work as a drug! and now my PC is bloated with over 200 GB of data!!
#answering this first bc i'm still cooking for dd2 😵💫#holy shit. this is so much I AM SO SORRY LMFAOOOOO i even cut out some ranty parts#can you tell which project i enjoyed more? very not obvious.#i WILL talk at length about this if anyone asks me in personal dms btw#infodumping? about MY research? more likely than you think#anyways if you wonder why i talk the way i do. it's because of academia. fuck academia btw .#i have 2 brain cells. 1 is for science the other is for [redacted]#themanwhomadeamonster#ask
5 notes
·
View notes
Text
Wanna see some REAL examples of AI in medicine?
Tired of seeing bad-faith posts on AI? Me too. Here’s how AI is actually truly helping advance medicine.
This new technology is AI powered, and allows researchers to discover new medicines to treat diseases such as cancer.
And this is AMIE, the AI healthcare worker who outperformed human doctors for conversational healthcare, and diagnostics.
Want a more comprehensive list that includes all the general ways AI is helpfully impacting the healthcare industry, including medicine? I got you! AI is also being used to help:
• claims processing
• clinical documentation
• revenue cycle management
• medical records management
• and more.
[Source]
All of which would otherwise be a continued strain on the already strained and overworked healthcare staff around the world.
…
But yeah, let’s always highlight the worst of AI, present it as the best we can do, and continue to push the narrative that human good, AI bad. We hate progress here. /s 🙄
16K notes
·
View notes
Text
AI in Omics Studies Market is valued at USD 614.8 million and is projected to grow at a compound annual growth rate (CAGR) of 31.45% over the forecast period, reaching approximately USD 5,480.47 million by 2032. Artificial Intelligence (AI) is revolutionizing the field of omics studies, which encompass genomics, proteomics, metabolomics, and other molecular data analyses. These disciplines, collectively known as "omics," allow scientists to investigate the roles, relationships, and actions of genes, proteins, and metabolic pathways. Integrating AI into omics studies has transformed the way researchers analyze complex biological data, offering unprecedented insights into disease mechanisms, drug discovery, and personalized medicine. This article explores the role of AI in the omics studies market, its applications, benefits, and the challenges that must be addressed to maximize its potential.Omics studies are essential in understanding diseases at the molecular level. By examining an organism's entire set of genes, proteins, metabolites, or other cellular molecules, scientists gain a holistic view of biological functions and their changes under specific conditions. This vast amount of data holds the potential for identifying biomarkers, developing new therapies, and personalizing treatment approaches. However, analyzing omics data is challenging due to its volume, complexity, and variability. This is where AI becomes invaluable, enabling researchers to derive meaningful insights from data that would otherwise be difficult to interpret.
Browse the full report https://www.credenceresearch.com/report/ai-in-omics-studies-market
AI's Role in the Omics Studies Market
AI has introduced advanced techniques like machine learning (ML) and deep learning (DL) that can process large datasets, recognize patterns, and make predictions based on the data. In the context of omics studies, AI supports tasks such as data pre-processing, pattern recognition, and predictive modeling. These tasks are particularly useful in genomics for identifying genetic mutations associated with diseases, in proteomics for analyzing protein interactions, and in metabolomics for studying metabolic pathways affected by disease states.
1. Genomics: Unraveling Genetic Information
In genomics, AI tools help analyze DNA sequences to identify gene variations that increase the risk of diseases like cancer, cardiovascular disorders, and rare genetic syndromes. AI algorithms can rapidly scan through enormous genomic datasets to detect mutations and predict their implications. Machine learning models, such as random forests and neural networks, have become essential for filtering out significant genetic markers from thousands of potential candidates, greatly aiding genetic diagnostics and treatment planning.
2. Proteomics: Exploring Protein Dynamics
Proteomics, which studies the structure and function of proteins, benefits from AI by aiding in protein identification and quantification. AI tools such as convolutional neural networks (CNNs) can predict protein structures and model protein-protein interactions, accelerating discoveries in fields like drug development. For example, AlphaFold, an AI developed by DeepMind, has made groundbreaking advances in predicting protein structures. By accurately modeling the shape of proteins, researchers can better understand disease mechanisms and design targeted therapies.
3. Metabolomics: Mapping Metabolic Changes
Metabolomics investigates the metabolic profiles of organisms, providing insights into how diseases affect cellular metabolism. AI-driven analytical tools help interpret complex metabolic data, identify biomarkers, and establish disease signatures. In cancer research, for instance, AI-based metabolomics analyses have identified unique metabolic alterations that distinguish cancer cells from normal cells. This information enables researchers to pinpoint metabolic vulnerabilities and develop interventions that target cancer-specific metabolic pathways.
Benefits of AI in Omics Studies
AI-driven advancements in omics studies offer numerous benefits for biomedical research and personalized medicine:
- Enhanced Data Processing: AI can handle vast, multidimensional datasets at a scale that traditional methods cannot, accelerating the discovery process and improving the accuracy of results. - Improved Precision Medicine: AI facilitates the identification of biomarkers and patient stratification, allowing for personalized treatments based on an individual’s genetic and molecular profile. - Reduced Costs and Time: Automation and data-driven insights reduce the time and cost of experimental trials, enabling researchers to prioritize promising targets for further exploration. - Predictive Modeling for Drug Discovery: AI models can predict drug efficacy and safety by analyzing omics data, expediting drug discovery and reducing the reliance on animal testing.
Challenges and Future Outlook
Despite its transformative potential, there are challenges to implementing AI in omics studies. Data privacy and security are significant concerns, particularly when dealing with sensitive genetic information. Regulatory frameworks are still catching up with the ethical implications of AI in healthcare, and there is a pressing need for standardized guidelines. Additionally, the lack of interpretability in some AI models, particularly deep learning algorithms, makes it difficult for researchers to fully understand the rationale behind certain predictions, which is essential for clinical applications.
Another challenge is data quality. Omics data is often noisy and incomplete, which can limit the effectiveness of AI algorithms. Developing robust AI systems that can work with imperfect data will be crucial for the future of AI in this field.
Looking forward, continued collaboration between data scientists, bioinformaticians, and healthcare professionals will be essential for overcoming these challenges. AI in omics studies will likely expand beyond research institutions into clinical settings, where it could become a fundamental part of diagnostics and treatment planning. Integrating AI into omics studies promises a future where personalized medicine is not only achievable but also affordable and accessible to a broader population.
Segmentation:
Based on Product Type:
Genomics
Proteomics
Metabolomics
Transcriptomics
Based on Technology:
Machine Learning
Natural Language Processing
Computer Vision
Deep Learning
Based on End-User:
Academic Institutions
Pharmaceutical Companies
Biotechnology Firms
Healthcare Providers
Research Organizations
Based on Region:
North America (United States, Canada)
Europe (Germany, United Kingdom, France, Italy)
Asia-Pacific (China, India, Japan, Australia)
Latin America (Brazil, Mexico, Argentina)
Middle East and Africa (South Africa, UAE, Saudi Arabia)
Browse the full report https://www.credenceresearch.com/report/ai-in-omics-studies-market
Contact:
Credence Research
Please contact us at +91 6232 49 3207
Email: [email protected]
Website: www.credenceresearch.com
0 notes
Note
WoLF PSORT is an extension of the PSORT II program for protein subcellular localization prediction, which is based on the PSORT principle. WoLF PSORT converts a protein's amino acid sequences into numerical localization features; based on sorting signals, amino acid composition and functional motifs. After conversion, a simple k-nearest neighbor classifier is used for prediction. To predict the subcellular localization of your target protein, simply paste your protein's amino acid sequence (single letter code) in the WoLF PSORT window below and click submit.
DeepLoc - 2.0
Prediction of eukaryotic protein subcellular localization using deep learning. DeepLoc 2.0 predicts the subcellular localization(s) of eukaryotic proteins. DeepLoc 2.0 is a multi-label predictor, which means that is able to predict one or more localizations for any given protein. It can differentiate between 10 different localizations: Nucleus, Cytoplasm, Extracellular, Mitochondrion, Cell membrane, Endoplasmic reticulum, Chloroplast, Golgi apparatus, Lysosome/Vacuole and Peroxisome. Additionally, DeepLoc 2.0 can predict the presence of the sorting signal(s) that had an influence on the prediction of the subcellular localization(s).
MembraneFold
About MembraneFold
MembraneFold combines two types of protein sequence prediction tools: protein structure (AlphaFold [1] and OmegaFold [2] and transmembrane protein topology (DeepTMHMM [3]. The Mol* toolkit [4] is used for visualisation.
The purpose of MembraneFold is to present membrane positioning and structure predictions of a given protein chain simultaneously. The tool allows to obtain a fast overview of this information and quickly toggle between the topology annotation and the structure confidence scores.
sorry it took me so long to get to this ask!
this is really neat, and i think its great to see more tools predicting what happens to certain structures. i did consider looking for localization signals in the proteins i am making for this blog, but ultimately decided against it to keep things easier for me. furthermore, i honestly don't entirely trust most of these to even make it through the translation step, and i shudder to think what a mess any sort of co-localization would make on top of the existing horror of my abominations. to be honest i'm also just not sure how to use these and don't really want to download any more software, at least not until i have more free time. however, its still really fun to mess around with tools like this, and i highly encourage anyone interested to look into it!
letter sequence in this ask matching protein-coding amino acids:
WLFPSRTisanetensinfthePSRTIIprgramfrprteinscelllarlcaliatinpredictinwhichisasednthePSRTprincipleWLFPSRTcnvertsaprteinsaminacidseqencesintnmericallcaliatinfeatresasednsrtingsignalsaminacidcmpsitinandfnctinalmtifsAftercnversinasimpleknearestneighrclassifierissedfrpredictinTpredictthescelllarlcaliatinfyrtargetprteinsimplypasteyrprteinsaminacidseqencesinglelettercdeintheWLFPSRTwindwelwandclicksmitDeepLcPredictinfekaryticprteinscelllarlcaliatinsingdeeplearningDeepLcpredictsthescelllarlcaliatinsfekaryticprteinsDeepLcisamltilaelpredictrwhichmeansthatisaletpredictnermrelcaliatinsfranygivenprteinItcandifferentiateetweendifferentlcaliatinsNclesCytplasmEtracelllarMitchndrinCellmemraneEndplasmicreticlmChlrplastGlgiapparatsLyssmeVacleandPerismeAdditinallyDeepLccanpredictthepresencefthesrtingsignalsthathadaninflencenthepredictinfthescelllarlcaliatinsMemraneFldAtMemraneFldMemraneFldcminestwtypesfprteinseqencepredictintlsprteinstrctreAlphaFldandmegaFldandtransmemraneprteintplgyDeepTMHMMTheMltlkitissedfrvisalisatinTheprpsefMemraneFldistpresentmemranepsitiningandstrctrepredictinsfagivenprteinchainsimltaneslyThetlallwsttainafastverviewfthisinfrmatinandqicklytggleetweenthetplgyanntatinandthestrctrecnfidencescres
protein guy analysis:
this one doesn't look great, with lots of loops that do not seem to stick together and are just floating loosely and horribly around the outside of the protein. while none of this is predicted with much confidence, the middle does look slightly better. it is made up of several alpha helices arranged in a way that almost resembles a protein channel if i don't think too hard about it and am blindingly optimistic.
predicted protein structure:
#science#biochemistry#biology#chemistry#stem#proteins#protein structure#science side of tumblr#protein asks#protein info
17 notes
·
View notes
Text
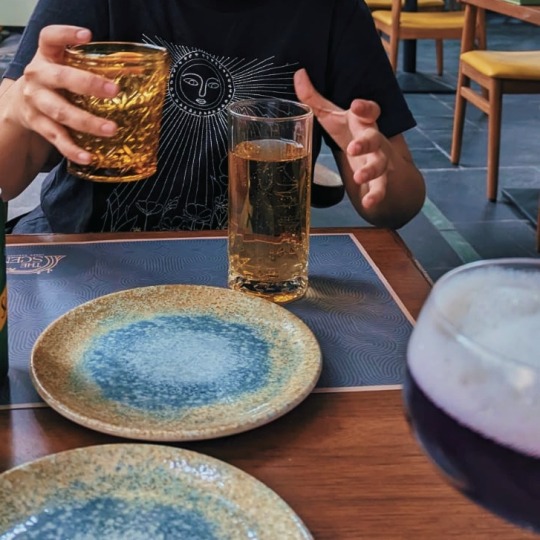
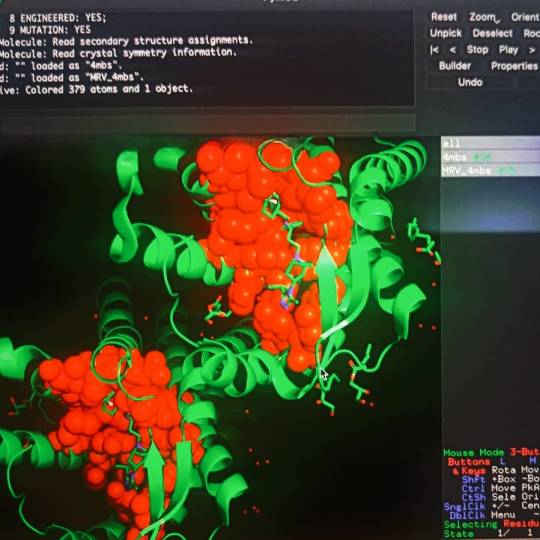
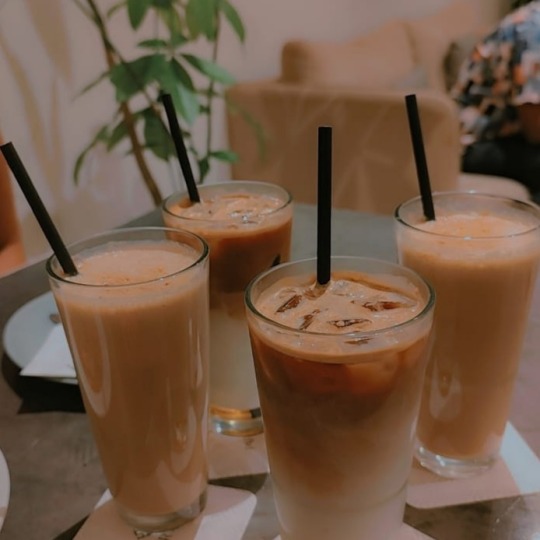
this week on megumi.fm ▸ coding and coffeeshops
📋 Tasks
💻 Internship ↳ lab meet!!! got to learn about the other projects in the lab ↳ got work from home approved!! ↳ optimize protein seq code // account for missing residues ✅ ↳ add on a binding site identifier function for code using 4.5A distance threshold ✅ ↳ optimize binding site code // reducing time complexity for large PDB file inputs ✅ ↳ download and extract alphafold human protein repository and analyze pdb file formats ↳ set up progress tracker and upload code on colab ✅ 🎓 Uni ↳ Final Project: update images quality according to changes mentioned ✅ ↳ renew uni email for extra credit classes ✅ ↳ extra credit classes started this week! 🩺Radiomics Projects ↳ call with teammates to discuss next steps ✅ 📧 Application-related ↳ finished masters application form for 1/1 Uni (waiting on my referee reports) ✅ ↳ finalize referee report from my profs ✅
📅 Daily-s
🛌 consistent sleep [7/7] 💧 good water intake [5/7] 👟 exercise [5/7]
Fun Stuff this week
🍻 met up with my bestie @muakrrr <3 it was a stressful tuesday so meeting him for lunch was super comforting! he bought this cute purple drink and I got myself some ginger ale and the waiter served us the wrong drinks (gender and expectations something something) and it was amusing to watch them get confused when we corrected them 🎂 mom's b'day this week!! went out for dinner with her!! 🛒 went shopping with relatives who I haven't seen in years. bought myself a book! (rip my bookshelf) ☕ went out for coffee and dinner with my girlies (the same besties who I exchanged mugs with). we're trying to spend as much time together as possible before we leave to different countries for our masters 🎮 continuing the beginner's guide 📺 ongoing: Marry my Husband, Cherry Magic Th, Last Twilight 📺 binged: KinnPorsche The Series
📻 This week's soundtrack
Love Wins All by IU (been crying over this music video for days now. it's beautiful) KinnPorsche theme by Slot Machine: Kinn's theme [aka Phiang Waichai; TH] | Porche's theme [aka Free Fall; Eng] (first of all this is one of the catchiest theme songs to exist second only to SPECIALZ aka the JJK s2 op i'm also particularly losing my mind over how the two themes are love letters to the main characters from each other... the narrative parallels of it all are driving me insane sldkhlaksjkshs) Dum Dum by Jeff Satur + the Live Unchained version where his vocals are heavenly (maybe im so drawn to this song because the chorus is similar to the melodic motifs of the KPTS themes/soundtrack, either way, the show introduced me to him and god. I've been voraciously consuming his discography.) Ghost by Jeff Satur (on repeat all week. thoroughly obsessed with this song- the lyricism, his voice, the storyline in the MV, his acting, everything. wow. truly.)
---
[Jan 22 to 28 ; week 4/52 || I. love. my. internship. like. I have been having the most fun time problem solving and troubleshooting. it's also super satisfying to see the outcome of my code. it's been a while since I used python (I've been coding on C) so I forget that python has a lot of inbuilt functions that would do the same tasks I inadvertently entrust my nested loops with, and finding out about them is always so joyous (although it means I have to scrap off several chunks of code). i am a bit annoyed though, because the other intern isn't really doing any work that we're entrusted with so I'm having to carry the team and it's taking me too much time. but oh well. I've suggested we split tasks from next week, hopefully that'll make things better.
I've also been procrastinating a lot when it comes to my masters applications and it really hit me this week when I had to run to uni several times to get things approved and completed. Now that I'll get to work from home I need to set up a proper schedule to get application work completed wayy in advance. also need to resume my GRE prep from next week.]
#52wktracker#studyblr#study blog#studyspo#stemblr#stem student#study goals#student life#college student#studying#stem studyblr#adhd studyblr#adhd student#study motivation#100 days of productivity#study inspo#study inspiration#gradblr#uniblr#studyinspo#sciblr#study aesthetic#study blr#study motivator#100 days of self discipline#100 days of studying#stem academia#bio student#100 dop#100dop
27 notes
·
View notes
Text
Short term view. Short term view. Short term view. Crazily short term view.
LLM are but a small part of AI, and if google is spending more money and more power it’s definitely NOT going to search…
Look at this :
This is the next frontier in biology. Medical science, nutrition, you name it, it has protein in it.
This is going to make shit tons of money.
And this:
If you’re not an engineer, and by this, I mean a real one: with physics and chemistry and mechanics or aeronautics. (Not software one. I mean. I love them but I am sorry, software engineering is often really not taught as engineering.) you have no idea what this means : the data collected about the weather are giving better predictions than the fluid dynamics equations we’re able to solve.
AND THAT MEANS THAT THE SOLUTION OF THE EQUATIONS ARE IN THE DATA, we just don’t know yet how to extract them. You know what that means ? F***ING LONG TERM PREDICTIONS FOR CLIMATE CHANGE. You know who would kill for this kind of numbers ? INSURANCE !!!
People see ChatGPT and say: meh, that’s not going to revolutionise anything. Yeah, we know. That is just a step needed to be able to interface data and build complex models.
It makes me crazy when people say : AI is a fad look, the bubble is going to explode.
AI is not a fad. AI is LONG TERM something that’s going to have on humans the same impact as mechanisation.
You know what the industrial revolution did? People before it were making food. Regarding of places/time/knowledge between 85-90% of the population were needed to just provide food for surviving. Your potters, clothes makers, blacksmiths, carpenters, doctors, clercs, soldiers, judges, teachers, philosophers ? In the 10-15%.
Fast forward 100 years later, late 19th, beginning of the 20st century, only 10% of people are working in agriculture, the rest is building bicycles, and cars, and inventing phones and radio.
Fast forward 100 years later, less than 3% of the population feed the 97%
From 90 to 3.
That’s a revolution. Automation is going to build on top of that. For all the rest.
And people are not ready.
There are telling that LLM is not going to be a trillion dollars industry. Nope. It’s not. But that’s not what AI is all about.
Sources: official figures for France: < 2.7% of all WORKERS. That means less than that for the whole population.
ed zitron, a tech beat reporter, wrote an article about a recent paper that came out from goldman-sachs calling AI, in nicer terms, a grift. it is a really interesting article; hearing criticism from people who are not ignorant of the tech and have no reason to mince words is refreshing. it also brings up points and asks the right questions:
if AI is going to be a trillion dollar investment, what trillion dollar problem is it solving?
what does it mean when people say that AI will "get better"? what does that look like and how would it even be achieved? the article makes a point to debunk talking points about how all tech is misunderstood at first by pointing out that the tech it gets compared to the most, the internet and smartphones, were both created over the course of decades with roadmaps and clear goals. AI does not have this.
the american power grid straight up cannot handle the load required to run AI because it has not been meaningfully developed in decades. how are they going to overcome this hurdle (they aren't)?
people who are losing their jobs to this tech aren't being "replaced". they're just getting a taste of how little their managers care about their craft and how little they think of their consumer base. ai is not capable of replacing humans and there's no indication they ever will because...
all of these models use the same training data so now they're all giving the same wrong answers in the same voice. without massive and i mean EXPONENTIALLY MASSIVE troves of data to work with, they are pretty much as a standstill for any innovation they're imagining in their heads
52K notes
·
View notes
Text
La inteligencia artificial frenará el envejecimiento: doctor De la Peña
El doctor Manuel de la Peña, reconocido cardiólogo y gurú de la longevidad, afirma que la inteligencia artificial como Alpha Fold 3 de Google y otras AI están compitiendo en el mercado para ser los primeros en frenar el envejecimiento Por Deyanira Vázquez | Reportera De hecho, AlphaFold 3 ya ha logrado determinar la estructura tridimensional de 200 millones de proteínas, por lo que esta…

View On WordPress
0 notes
Text
(0:00) Bestie intros!
(3:18) The science behind Hurricanes Helene and Milton
(14:59) The economics of intensifying natural disasters
(29:03) AlphaFold creators win Nobel Prize in Chemistry
(35:17) The Jayter's Ball
(38:53) Google antitrust update: DOJ is going for a breakup
(53:32) VC giveback: CRV will return ~$275M of a $500M fund to LPs
(1:03:44) New TikTok survey shows increased usage as a news source
(1:15:26) Election update: Are polling problems causing a strategy shift for Kamala Harris?
Follow the besties:
Follow on X:
Follow on Instagram:
Follow on TikTok:
Follow on LinkedIn:
Intro Music Credit:
Intro Video Credit:
Referenced in the show:
1 note
·
View note