#seismometer strong motion
Explore tagged Tumblr posts
Text
#geotechnical#geotechnical instrumentation and monitoring market#geoteknik#instrumengeoteknik#seismometer#seismometer product#seismometer digital#seismometer strong motion#jual seismometer
1 note
·
View note
Text
The only way to know firsthand would be to take a real Journey to the Center of the Earth—but for now, a new study suggests our planet’s inner core might be spinning more slowly than it used to.
The Earth’s inner core, a solid ball of iron and nickel about 70 percent of the size of the moon, rotates along with the rest of the planet, but scientists disagree about whether it might spin at a slightly slower, faster or equal speed, compared to the outer layers. The study, published in June in the journal Nature, uses seismic waves to add evidence for the idea that the inner core switches between spinning faster and slower than Earth’s crust.
Such changes in the inner core’s spin could have a small impact on the Earth’s magnetic field—and they could, very marginally, alter the length of days up on the surface.
The inner core is “a planet within a planet, so how it moves is obviously very important,” Xiaodong Song, a seismologist at Peking University in China, told the New York Times’ Robin George Andrews last year.
But scientists have been struggling to pin down the specifics of the inner core’s motion. The controversy of whether or not the heart of the planet spins differently than the rest of the Earth reignited in January 2023, after Song and others proposed in Nature Geoscience that the core’s rotation oscillates in a 70-year cycle, alternating between leading and lagging the spin of the planet every 35 years. The most recent switch, from faster to slower than the outer part of the Earth, occurred about 15 years ago, according to those researchers.
“This paper shows that the evidence for [faster] rotation is strong before about 2009 and basically dies off in subsequent years.” Paul Richards, a seismologist at Columbia University who was not involved with either paper, told the Washington Post’sCarolyn Y. Johnson in 2023.
Not all scientists who study the interior of the planet agreed, however. Dongdong Tian, a seismologist at the China University of Geosciences, and Lianxing Wen, a geoscientist at Stony Brook University, wrote in a comment to the journal Geophysical Research Letters that fluctuations on the surface of the inner core could explain the observed changes, even if its spin was constant.
Now, the new study provides support in favor of last year’s paper, suggesting the inner core has been decreasing its speed since about 2008—and it fuels further debates on the nature of Earth’s center.
Researchers have no way of directly observing the planet’s innermost layers, so they instead rely on tracking the energy released by earthquakes. These seismic events generate waves of energy that pass through the Earth’s crust, mantle and core.
To view these waves, geologists use a tool called a seismometer to record the shaking of the Earth’s surface that’s generated by an earthquake. The instrument produces wiggles on a line graph, which they call waveforms. The researchers understood that if they tracked waveforms from seismic waves that passed along the same path through the center of the Earth in different years, they might be able to see how the inner structure of the planet is evolving over time.
For the study, they observed patterns in the waveforms from 121 earthquakes that originated in the South Sandwich Islands near Antarctica from 1991 to 2023. These were recorded on a seismometer in Alaska after traveling through the Earth’s interior. If the inner core is rotating at a different speed than the layers above it, then the repeated seismic waves should have passed through different areas of the inner core during the 32-year period, leading to differences in the detected waveforms, even while they take the same overall path through the planet, per Science News’ Nikk Ogasa.
But if the inner core was shifting its speed in a cycle, some of these waveforms would repeat. And sure enough, the team was able to match more than 25 patterns before and after 2008, showing a symmetry they say is explained by a recent slowing of the inner core’s speed.
“When I first saw the seismograms that hinted at this change, I was stumped,” study co-author John Vidale, an earth scientist at the University of Southern California, says in a statement. “But when we found two dozen more observations signaling the same pattern, the result was inescapable. The inner core had slowed down for the first time in many decades.”
Still, not everyone is convinced. Wen tells Science News that even with the new study, “nothing has changed”—he maintains that the expanding and contracting of the inner core’s surface is significant enough to explain the patterns observed in the waveforms.
Debates like these tend to polarize the scientific community. But Hrvoje Tkalčić, a geophysicist at the Australian National University who was not involved with the study, tells Science News “it is very likely the truth is somewhere in between.” To try to understand the unreachable depths of Earth’s interior, scientists tend to make a lot of assumptions, he says. “We need more data to find the ultimate truth.”
0 notes
Photo
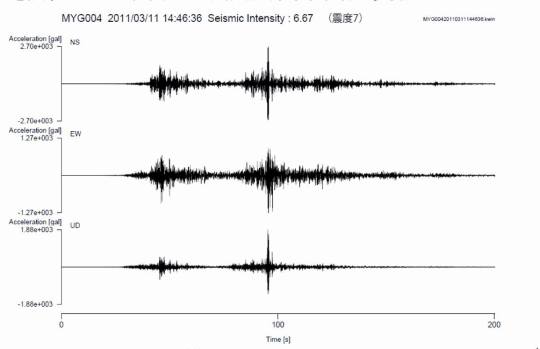
Peak Ground Acceleration When you look at a seismogram, a classic one, what are the units on the vertical axis? That’s the earthquake, right? But what are we actually measuring?
A classic seismogram is built based on a pen, held in place by a mass, suspended over a turning drum. During an earthquake, that drum vibrates side to side and the pen records the shaking of the earthquake on it, right? While this simple model does record some details on an earthquake, the real world is much more complicated. Even in this simple case, the pen would be held in place by a mass and its own inertia, right? But if the whole room is shaking, will the mass really stay fixed in position over a long time? Although this isn’t a perfect example, picture the classic “Newton’s cradle” experiment, of balls on strings bouncing back and forth. If you start the balls swinging and they bounce off seemingly elastically, eventually smaller effects build and the balls that were previously stationary will swing from side to side. Inertia only gets you so far if there are lots of different things going on. Trying to record an earthquake is a very complicated problem. If the mass holding our pen in place moves at all, then suddenly the mark on the page doesn’t just show horizontal movement, it is a combination of the horizontal movement of the drum and whatever movement happens to the pen. Even in the case of the best classic seismographs this will be a problem – your pen is never truly fixed, just like the Newton’s cradle is not truly elastic, so the final record doesn’t only show the motion of the ground, it’s instead records a summation of the different motions. This problem actually can get even worse. Earthquakes aren’t just a simple back and forth shaking; they’re a summation of different waves with different frequencies all piled on top of each other. If you try to make your seismograph work so that the pen is fixed for some frequencies, it might shake at others, so the final recorded seismogram depends on which frequencies you measure. It’s kind of like audio speakers – you put low frequencies through a subwoofer and you put other frequencies through standard speakers to produce a more complete sound. If you put two seismographs right next to each other but design them differently, they will produce different records of the same earthquake! Thankfully, we don’t use pen and paper any more to record earthquakes; modern seismographs are electronic. They measure how masses move by converting those motions into electronic signals, which means that we can pull apart these problems if we build an instrument correctly. The instrument needs to respond to different frequencies and getting seismometers to do this has been complicated. It took decades of work by electronic engineers to build seismic instruments that could overcome all of those complexities to produce a standard, regularized signal. During an earthquake, what is actually being measured is the change in electric current associated with motion of a mass inside a seismograph. The instrument will always have some limitations; some frequencies will be filtered or undetectable due to noise within the instrument, but with electronics you can design different systems to capture most of the motion at a variety of different frequencies. That whole discussion finally lets me answer the question I posed at the beginning; what a seismogram is actually showing. A modern seismogram starts off as an electronic response to motion on a mass. In other words, it is directly related to the force the mass experiences. The force on a mass can be directly converted to “acceleration” thanks to Isaac Newton, so for modern seismic instruments, you often see the units presented as “Acceleration”. Plotting acceleration recorded by a seismic instrument versus time gives you a seismogram like this one, recorded during the 2011 Tohoku Earthquake in Japan. Ground acceleration is a useful parameter to measure because it directly relates to the force on an object. During an earthquake there will be lots of different waves with different frequencies passing, the ground will shake in all 3 directions and the shaking will change during the quake due to all the different, complex features of the Earth. However, if you measure the peak ground acceleration, you’re getting a measurement of the maximum force felt by any object during that quake. The peak acceleration on this graph is the point where the absolute value is the largest - where the line gets the farthest away from the center. Peak ground acceleration is a way to take all the complexities of an earthquake, the changes in frequency and intensity, and put a single number on it. In fact, that number can be expressed as a fraction of the acceleration due to gravity a percent of “g” since gravity is roughly constant. If you can get a single number out of an earthquake, that can be really helpful for the public because you can use that as your basic standard for building earthquake-safe buildings. Peak Ground Acceleration is therefore one of the parameters used to develop building safety standards in this country. If you’ve got a building hooked directly to the ground, the Peak Ground Acceleration is the strongest force it will feel during the quake. If you build your building strong enough to survive that moment, then the building could be in good shape. Those are the numbers the U.S. government puts out. The US Geological Survey put out updated seismic hazard maps in 2014 and the most commonly displayed maps showed the probability of feeling an earthquake with a peak acceleration that was a certain fraction of the acceleration of gravity. However, since an earthquake is a complex mixture of waves, designing an earthquake-safe building isn’t quite that simple. Taller buildings sway with different frequencies, so the response of the building is important as well. A building that hits its own resonant frequency during an earthquake could suffer heavy damage even if it survives the peak ground acceleration. The USGS therefore also put out maps of the spectral acceleration for certain frequencies – that’s the maximum acceleration which would be experienced by a structure with a natural vibration frequency in that range. These parameters can be directly related to damage during an earthquake, but that’s not the whole story. Ready for some calculus? We can also integrate acceleration to get “velocity” and plot up “peak ground velocity”. That’s another commonly shown parameter on seismic instruments. If you try to come up with an expression for damage risk during an earthquake, you’ll find that every building has its own properties. Some buildings are most likely damaged during the peak acceleration; some buildings actually are damaged most at the peak velocity. And you know what? It can be even more complicated than that. Some buildings show increases in damage during longer earthquakes even when the peak acceleration or peak velocity doesn’t change – somehow the damage works its way into the structure with multiple passes. To develop a truly quake-safe building code therefore you need to know both the way your structure behaves and the way the Earth behaves. An earthquake is a complicated mix of shaking at different frequencies and over different times. A seismograph can record that in a certain way, but interpreting that data requires a lot of math involving frequencies, wavelengths, travel times, etc. We can convert those complicated functions into single numbers useful in design, but in doing so we lose some constraints on the behavior of the system. Developing earthquake safe buildings therefore isn’t easy. Engineers are constantly testing building designs using large shaketables to see how they hold up for exactly these reasons (http://tmblr.co/Zyv2Js1NJfTeC); the ground can shake in many different ways and some buildings will be strong against one way of shaking and weak against another. These are the kinds of tasks that seismic engineers are faced with in quake-prone areas every day, and they’re not easy puzzles to solve. -JBB Image credit: http://geophysics.eas.gatech.edu/people/zpeng/Japan_20110311/ http://eandt.theiet.org/.../2011/04/basics-of-seismology.cfm Read more: http://www.ipgp.fr/~brunet/Seismometers.pdf
#earthquake#seismometer#seismograph#shaking#wave#science#frequency#math#velocity#acceleration#physics#structural geology#geophysics#geology#the earth story
40 notes
·
View notes
Text
A new way to identify a rare type of earthquake in time to issue lifesaving tsunami warnings
by Valerie Sahakian
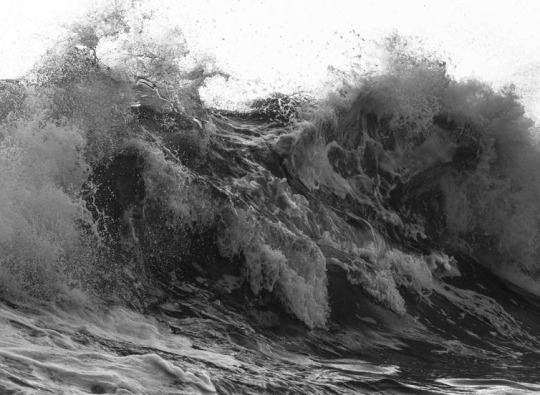
This unusual earthquake type generates an outsized tsunami. camila castillo/Unsplash, CC BY-ND
Just a few times in a century, somewhere on the globe, a rare “tsunami earthquake” occurs. These are mysterious because, while they’re just medium-sized as earthquakes go, they cause disproportionately large and devastating tsunamis. This type of midsized earthquake is very different than an event like the 2004 earthquake in Sumatra – a very big magnitude 9.2 event which unsurprisingly produced a huge tsunami.
The most recent tsunami earthquake happened in 2010. A magnitude 7.8 earthquake off the Mentawai Islands in Indonesia set off a tsunami that was over 50 feet in height in some places – much greater than seismologists would predict based just on the earthquake’s size. 509 people were killed, and 15,000 more were displaced or left homeless.
Tsunami earthquakes are particularly destructive and dangerous because the massive tsunami waves can hit local coastal communities within just five to 15 minutes – before officials can issue a warning. But based on our analysis of previously unavailable closeup observations of the 2010 Mentawai event, my colleagues and I think there is a way to determine that an event is a tsunami earthquake in time to warn people that an unexpectedly large wave is on the way.
Earthquakes under the ocean
The Earth’s surface is made up of floating tectonic plates that fit together like a slightly imperfect jigsaw puzzle. These plates are moving next to, under or away from each other.
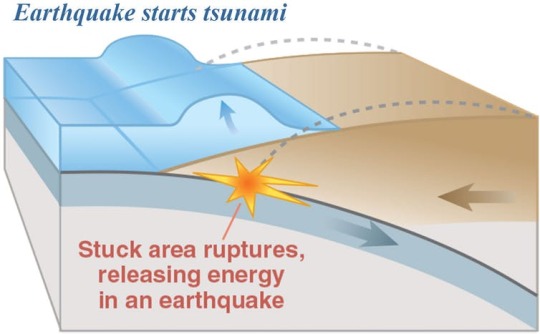
An earthquake along a subduction zone happens when the leading edge of the overriding plate breaks free and springs seaward, raising the seafloor and the water above it. This uplift starts a tsunami. USGS
In a subduction zone, one tectonic plate is sinking beneath another. This builds up stresses over time and will eventually create an earthquake. Most typical subduction-zone earthquakes occur roughly 10 to 30 miles down, in an area where the rocks are rigid and strong on the fault between the two tectonic plates.
Meanwhile, the shallowest area of a subduction zone, closest to the seafloor, is made up of soft sediments that are not very strong. Earthquakes rarely occur only here, because stresses mostly don’t build up in these soft, weak rocks.
Geoscientists define an earthquake’s overall size with its magnitude. Earthquake magnitude describes how much “work” is accomplished by the earthquake moving the fault – more work for either more movement, or for moving more rigid rock.
Very large earthquakes, like the magnitude 9 Tohoku earthquake in Japan in 2011, are so big that they break the deeper part of the subduction zone, but also continue upwards to break the shallow part of a subduction zone. This rapid earthquake motion moves the seafloor and creates a tsunami.
Cartoon depicting the amount of movement in five earthquakes. The 2010 Mentawai tsunami earthquake moved the fault much more – over 65 feet compared to about 15 feet for the others – and the movement occurred much closer to the seafloor than in any of the other earthquakes. Sahakian et al. (2019), GRL
What sets tsunami quakes apart
“Tsunami earthquakes” are strange in that they happen almost entirely in the soft, weak section of the fault.
Because tsunami earthquakes break such soft rock, they happen slower, and create much more movement on or near the seafloor in comparison to a normal subduction-zone earthquake of the same size that happens in rigid rock. This in turn creates a much larger tsunami than expected.
Seismograms showing how much the ground shook from six similarly sized earthquakes, at seismometers all about the same distance from their earthquake. You’d expect the shaking to be comparable. The 2010 Mentawai earthquake seismogram is at the bottom in orange, and shows significantly less shaking than any of the others. Modified from Sahakian et al. (2019), GRL.
A tsunami earthquake might have the same magnitude as an earthquake that occurs in rigid rock but produces much less of what seismologists call high-frequency energy.
Think of breaking a thick slab of concrete – which is strong and would produce an audible bang with both low and high-pitched noise – versus breaking a loaf of bread, which makes almost no sound at all. In the Earth, “sound” is the shaking you feel under your feet. The soft bread break is like a tsunami earthquake that doesn’t release a lot of high-frequency energy, and thus doesn’t create as much shaking as we would expect for its magnitude.
Sensing quakes in time to warn
Currently, officials rely on knowing an earthquake’s magnitude and location to issue tsunami warnings within tens of minutes. But this doesn’t work in the case of tsunami earthquakes, because the earthquake’s magnitude doesn’t match up with the size of the tsunami it produces.
Instead, to figure out whether an earthquake is in fact a tsunami earthquake, scientists compare its seismic magnitude measured from afar with the amount of high frequency radiated energy it produced, as recorded by far away stations.
If the ratio of energy to magnitude is very low, it’s a tsunami earthquake – basically, its shaking was far too weak for its magnitude because it was breaking soft rock. Instead, its energy is of the low-frequency type: Rather than strong shaking, its energy goes into large slow movement of the seafloor and the ensuing tsunami.
The problem is that in the past, scientists had never recorded one of these elusive earthquakes closeup in what we call the near-field – within about 180 miles (300 kilometers) or so. Instead, scientists have had to find an earthquake’s energy-to-magnitude ratio using seismic waves that have traveled all the way from the epicenter of the earthquake across the world to where researchers can measure them. This process is relatively slow, so we haven’t been able to identify tsunami earthquakes quickly enough to warn people in time, before the wave hits the coast.
Now my colleagues and I have for the first time analyzed data recorded by seismic stations that happened to be near the epicenter of the 2010 Mentawai earthquake. We think we have figured out a new way to identify the danger of a future tsunami earthquake, faster.
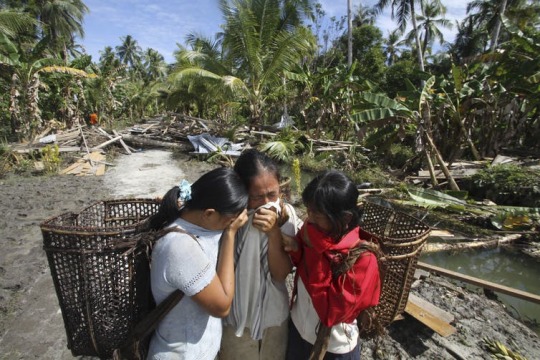
Tsunamis can take a terrible toll, as for this Indonesian family that lost their father and their home in the Mentawai disaster. AP Photo/Tundra Laksamana
Closer and quicker proxies
Our new study used the same concept of comparing the energy released by an earthquake to its seismic magnitude – but based on data from geographically close to the event. Instead of looking at energy measurements recorded at a distance, we used two proxies.
To look directly at how much the ground shook, we used seismic stations onshore near the epicenters of 16 earthquakes, including the Mentawai one in 2010. Because the amount the ground accelerates when seismic waves pass through illustrates how much high frequency energy is in the earthquake, this information was a stand-in for the data we would traditionally get from the far-flung teleseismic stations. Low accelerations mean little high frequency energy.
For the normal earthquakes we looked at, the accelerations from near-field seismometers were close to what we’d expect for each earthquake’s magnitude. In comparison, the 2010 Mentawai earthquake’s accelerations were closer to what we would expect for a magnitude 6.3 earthquake – whereas the earthquake was actually a magnitude 7.8, and produced a tsunami we’d expect for an event of greater than magnitude 8.
We also looked at GPS stations close to the earthquakes. They can show us how much the ground actually moved or was displaced, and measure the earthquake magnitude itself.
Using these measurements together allowed us to compare the amount of energy in the earthquake with respect to its magnitude – without waiting for the seismic waves to travel across the globe. Instead, we would have been able to identify a tsunami earthquake immediately by looking at how low the accelerations were on local seismometers in comparison to the magnitude of the earthquake based on GPS readings.
We think our finding is really promising because these near-field measurements are available immediately – even while an earthquake is happening. Seismologists could use this approach in the future, to identify a tsunami earthquake right after it happens, and provide warning to the nearby coast before the tsunami wave arrives.
About The Author:
Valerie Sahakian is an Assistant Professor of Geophysics at the University of Oregon
This article is republished from our content partners over at The Conversation.
17 notes
·
View notes
Photo

2019 June 4
SEIS: Listening for Marsquakes Image Credit: NASA, JPL-Caltech, Mars Insight
Explanation: If you put your ear to Mars, what would you hear? To find out, and to explore the unknown interior of Mars, NASA's Insight Lander deployed SEIS late last year, a sensitive seismometer that can detect marsquakes. In early April, after hearing the wind and motions initiated by the lander itself, SEIS recorded an unprecedented event that matches what was expected for a marsquake. This event can be heard on this YouTube video. Although Mars is not thought to have tectonic plates like the Earth, numerous faults are visible on the Martian surface which likely occurred as the hot interior of Mars cooled -- and continues to cool. Were strong enough marsquakes to occur, SEIS could hear their rumbles reflected from large structures internal to Mars, like a liquid core, if one exists. Pictured last week, SEIS sits quietly on the Martian surface, taking in some Sun while light clouds are visible over the horizon.
∞ Source: apod.nasa.gov/apod/ap190604.html
113 notes
·
View notes
Photo

SEIS: Listening for Marsquakes ⠀ ⠀ Image Credit: NASA, JPL-Caltech, Mars Insight⠀ ⠀ Explanation: If you put your ear to Mars, what would you hear? To find out, and to explore the unknown interior of Mars, NASA's Insight Lander deployed SEIS late last year, a sensitive seismometer that can detect marsquakes. In early April, after hearing the wind and motions initiated by the lander itself, SEIS recorded an unprecedented event that matches what was expected for a marsquake. This event can be heard on this YouTube video. Although Mars is not thought to have tectonic plates like the Earth, numerous faults are visible on the Martian surface which likely occurred as the hot interior of Mars cooled -- and continues to cool. Were strong enough marsquakes to occur, SEIS could hear their rumbles reflected from large structures internal to Mars, like a liquid core, if one exists. Pictured last week, SEIS sits quietly on the Martian surface, taking in some Sun while light clouds are visible over the horizon.⠀ ⠀ NASA APOD (https://go.nasa.gov/2F2DGBp) via Instagram http://bit.ly/2KuYJ33
7 notes
·
View notes
Text
Prediction of Strong Ground Motion Using Fuzzy Inference Systems Based on Adaptive Networks
Authored by Mostafa Allameh Zadeh*
Abstract
Peak ground acceleration (PGA) estimates have been calculated in order to predict the devastation potential resulting from earthquakes in reconstruction sites. In this research, a training algorithm based on gradient descent were developed and employed by using strong ground motion records. The Artificial Neural Networks (ANN) algorithm indicated that the fitting between the predicted strong ground motion by the networks and the observed PGA values were able to yield high correlation coefficients of 0.78 for PGA. We attempt to provide a suitable prediction of the large acceleration peak from ground gravity acceleration in different areas. Methods are defined by using fuzzy inference systems based on adaptive networks, feed-forward neural networks (FFBP)by four basic parameters as input variables which influence an earthquake in regional studied. The affected indices of an earthquake include the moment magnitude, rupture distance, fault mechanism and site class. The ANFIS network — with an average error of 0.012 — is a more precise network than FFBP neural networks. The FFBP network has a mean square error of 0.017 accordingly. Nonetheless, these two networks can have a suitable estimation of probable acceleration peaks (PGA) in this area.
Keywords: Adaptive-network-based fuzzy inference systems; Feed-forward back propagation error of a neural network; Peak ground acceleration; Rupture distance
Abbreviations: PGA: Peak ground acceleration; ANN: Artificial Neural Networks; FFBP: Feed-Forward Neural Networks; FIS: Fuzzy Inference System; Mw: Moment Magnitude
Introduction
Peak ground acceleration is a very important factor that must be considered in any construction site in order to examine the potential damage that can result from earthquakes. The actual records by seismometers at nearby stations may be considered as a basis. But a reliable method of estimation may be useful for providing more detailed information of the earthquake’s characteristics and motion [1]. The peak ground acceleration parameter is often estimated by the attenuation of relationships and also by using regression analysis. PGA is one of the most important parameters, often analyzed in studies related to damages caused by earthquakes [2]. It is mostly estimated by the attenuation of equations and is developed by a regression analysis of powerful motion data. Powerful motions relating to a ground have basic effects on the structure of that region [3]. Peak ground acceleration is mostly estimated by attenuation relationships [4]. The input variables in the constructed artificial neural network model are the magnitude, the source-to-site distance and the site’s conditions. The output is the PGA. The generalization capability of ANN algorithms was tested with the same training data. Results indicated that there is a high correlation coefficient (R2) for the fitting that is between the predicted PGA values by the networks and those of the observed ones. Furthermore, comparisons between the correlations by the ANN and the regression method showed that the ANN approach performed better than the regression. Developed ANN models can be conservatively utilized to achieve a better understanding of the input parameters and their influence, and thus reach PGA predictions.
Kerh & Chaw [1] used software calculation techniques to remove the lack of certainties in declining relations. They used the mixed gradient training algorithm of Fletcher-Reeves’ back propagation error [5]. They applied three neural network models with different inputs including epicentric distance, focal depth and magnitude of the earthquakes. These records were trained and then the output results were compared with available nonlinear regression analysis. The comparisons demonstrated that the present neural network model did have a better performance than that of the other methods. From a deterministic point of view, determining the strongest level of shaking- that can potentially happen at a site- has long been an important topic in earthquake science. Also, the maximum level of shaking defines the maximum load which ultimately affects urban structures.
From a probabilistic point of view, knowledge of the greatest ground motions that can possibly occur would allow a meaningful truncation of the distribution of ground motion residuals, and thus lead to a reduction in the computed values pertaining to probabilistic seismic hazard analyses. Particularly, it points to the low annual frequencies that exceed norms which are considered for critical facilities [6,7]. Empirical recordings of ground motions that feature large amplitudes of acceleration or velocity play a key role in defining the maximum levels of ground motion, which outline the design of engineering projects, given the potentially destructive nature of motions. They also provide valuable insights into the nature of the tails that further distribute the ground motions.
Feed-forward, back propagation error in neural networks
Artificial neural networks are a set of non-linear optimizer methods which do not need certain mathematical models in order to solve problems. In regression analysis, PGA is calculated as a function of earthquake magnitude, distance from the source of the earthquake to the site under study, local condition of the site and other characteristics that are linked to the earthquake source such as slippery length and reverse, normal or wave propagation. In non-linear regression methods, non-linear relations which exist between input and output parameters are expressed as estimations, through statistical calculations within a specified relationship [8]. One of the most popular neural networks is the back propagation algorithms. It is particularly useful for data modeling and the application of predictions [9] (Equations 1, 2 and 3). It is a supervised learning technique which was first described by Werbos [10] and further developed by Rumelhart et al. [11]. Furthermore, its most useful function is for feed forward neural networks where the information moves in one direction only, forward, beginning from the input nodes through to the hidden nodes, and then to the output nodes. There are no cycles or loops in the network.
In (1), one instance of iteration is written for the back propagation algorithm. Where Xk is a vector of current weights and biases, gk is the current gradient and a is the learning rate.
In (2), where F is the performance function of error (mean square error),'t' is the target and 'a' is the real output
In (3), 'a' is the net output,(n) is the net input and 'f' is the activation function of the neuron model
In (4), the error of energy is calculated by the least squares estimate for back-propagation learning algorithm. Where N is the number of training patterns, m is the number of neurons in the output layer. And tjk is the target value of processing the neuron. Therefore, this algorithm changes synaptic weights along with the negative gradient of the error energy function. Furthermore, it mostly benefits feed-forward neural networks where the information moves in only one direction, forward, beginning from the input nodes, through to the hidden nodes, and then to the output nodes. There are no cycles or loops in the network. The basic back-propagation algorithm adjusts the weights in the steepest direction of descent wherein the performance function decreases most rapidly. This network is a general figure of a multi-layer Prospectron network with one or several occasions of connectivity. Theoretically, it can prove every theorem that can be proven by the feed-forward network. Also, problems can be solved more accurately by testing general feed-forward networks.
Results of FFBP neural network
In Figure 1, testing the output of feed-forward neural networks against the true output is demonstrated. In Figure 2, the correlation coefficient of training, testing and validating general feed-forward neural networks is shown. In Table 1, testing the output of a feed-forward network against its true output has been compared. In Figure 3, training and validating the error graph against the feed-forward neural network is shown. Mean square error versus epoch is shown in Figure 4 with the aim of training and checking the general feed-forward
network. The sensitivity factor was obtained by training the feed-forward network (Figure 5). The sensitivity factor for input parameters is shown in Table 2. The performance error function was obtained by testing the FFBP neural network (Table 3).
Data processing
The datasets of records by large amplitude considered in this study involves one sets of accelerogram selected based on their value of PGA. These records are described below in terms of the variables generally considered to control the behavior of ground motions in general i.e. Magnitude, Rupture distance, style of faulting and site classification. The dataset includes recordings from events with Moment Magnitude ranging from (5.2-7.7) and rupture distance from (0.3-51.7 km). The SC values in the models were used as (1 to 5) for S-Wave velocity (For (1), Vs>1500 m/s and (5) Vs<180m/s). One Model was developed for each ANN method. This model was developed for estimation of maximum PGA values of the three components. The Focal Mechanism values in this model were used as (1 to 5) that (1: Strike Slip, 2:Reverse, 3:Normal, 4:Reverse oblique and 5: Normal oblique). A program includes MATLAB Neural Network toolbox was coded to train and test the models for each ANN method. All recordings from crustal events correspond to rupture distances shorter than 25km. The horizontal dataset shows a predominance of records from strike-slip and reverse earthquakes. Ground motions recorded on early strong-motions instruments often required a correction to be applied to retrieve the peak motions, Filtering generally eliminates the highest frequencies for motions recorded on modern accelerographs, and thus reduces the observed PGA values. The training of networks was performed using 60 sets of data. Testing of networks was done using 14 datasets that were randomely selected among the whole data. As shown in Figure 6 (a,b), the Mw and RD values of test and train data varied in the range of (5.2-7.7) and (0.3-52 km), respectively, the fault mechanism values were given in the Figure 6c. Figure 6d illustrated the site conditions of train and test data. As seen in this figure the site conditions were commonly soft and stiff soil types. Figure 6e showed the maximum PGA of records of the three components. In ANFIS model, training and the testing of records are shown in Figures 7a,b. Final decision surfaces are shown in Figure 7c. Final quiver surfaces are shown in Figure 7d.
Adaptive network based fuzzy inference system
The fuzzy logic appeared parallel to the growth in evolution of neural networks theory. The definition of being fuzzy can be found in human decision-making. These definitions can be searched by methods related to processing information [12].ANFIS is one of hybrid neuro-fuzzy inference expert systems and it works like the Takagi-Sugeno-type fuzzy inference system, which was developed by Jang [13]. ANFIS has a similar structure to a multilayer feed-forward neural network, but the links in an ANFIS can only indicate the flow direction of signals between nodes. No weights are associated with the links [14]. ANFIS
architecture consists of five layers of nodes. Out of the five layers, the first and the fourth layers consist of adaptive nodes while the second, third and fifth layers consist of fixed nodes. The adaptive nodes are associated with respective parameters, while the fixed nodes are devoid of any parameters [15-17]. For simplicity, we assume that the fuzzy inference system under consideration has two inputs x, y and one output called z. Supposing that the rule base contains two fuzzy if-then rules(6 and 7) of the Takagi & Sugenos [18], then the type-1 ANFIS structure can be illustrated as in Figure 6.
Rule 1: If (x is A1) and (y is B1) then (f = plx + qly + r1) (5)
Rule 2: If(x is A2) and (y is B2) then (f2 = p2 x + q2 y + r2) (6)
Where x and y are the inputs, A, Bi are the fuzzy sets and fi is the output within the fuzzy region specified by the fuzzy rule. Then pi , qiand r are the design parameters that are determined during the training process, in which a circle indicates fixed nodes, whereas a square indicates adaptive nodes.
The node functions which are in the same layer are of the same function family as described below:
In Figure 8, layer (1), every node (i) is a square node with a node function like this: O1i=μAi(x)
The outputs of this layer constitute the fuzzy membership grade of the inputs, which are presented as:
Where x and y are the inputs that enter node (i), A is a linguistic label and m (x),mBi (y) can adapt any fuzzy membership function.(a,b and c) are the parameters of the membership function. As the values of these parameters change, the bell shaped function varies accordingly. In layer 2 (Figure 8), every node is a circle node labeled n. The outputs of this layer can be presented as a firing strength of rule. In layer 3, every node is a circle node labeled N. The 'th’ node calculates the ratio of the ‘ith ’ rules' firing strength to the sum of all rules belonging to the firing strength. For convenience, outputs of this layer will be termed as normalized firing strengths. In layer 4, the defuzzification layer is an adaptive node with one node. The output of each node in this layer is simply a first order polynomial.
Where Wi is the output of layer 3, {pi * * ri} is the parameter set. Parameters in this layer will be referred to as consequent parameters. In layer 5,the summation neuron is a fixed node which computes the overall output as the summation of all incoming signals. The single node in this layer is a circle node labeled E that computes the overall output as the summation of all incoming signals.
Functionally, there are almost no constraints on the node functions of an adaptive network except in the case of a piecewise differentiability. Structurally, the only limitation of network configuration is that it should be of the feed-forward type. Due to minimal restrictions, the applications of adaptive networks are immediate and immense in various areas. In this section, we propose a class of adaptive networks which are functionally equivalent to fuzzy inference systems. The targeted architecture is referred to as ANFIS, which stands for Adaptive Network-based Fuzzy Inference System. ANFIS utilizes a strategy of hybrid training algorithm to tune all parameters. It takes a given input/output data set and constructs a fuzzy inference system which has membership function parameters that are tuned, or adjusted, using a back-propagation algorithm in combination with the least-squares type of method (NAZMY .T.M, 2009). Fuzzy inference systems are also known as fuzzy- rule-based systems, fuzzy models, fuzzy associative memories or fuzzy controllers, when used as controllers. Basically, a fuzzy inference system is comprised of five functional blocks.
a. A rule base containing a number of fuzzy if-then rules.
b. A database which defines the membership functions of the fuzzy sets used in the fuzzy rules.
c. A decision-making unit which performs inference operations on the rules.
d. A fuzzification interface which transforms the crisp inputs into degrees of match with linguistic values.
e. A defuzzification interface which transform the fuzzy results of the inference into a crisp output.
Usually the rule base and database are jointly referred to as the knowledge base. The steps of fuzzy reasoning performed by fuzzy inference systems are:
a. To compare the input variables with the membership functions on the premise part so as to obtain the membership values. (That is the fuzzification step).
b. To combine multiplications or minimizations of the membership values on the premise part so as to yield the firing strength of each rule.
c. To generate the qualified consequence— either fuzzy or crisp — of each rule depending on the firing strength.
d. To aggregate the qualified consequences so as to produce a crisp output. (That is the defuzzification step).
Results ofANFIS network for maximum PGA simulation
In this research, an adaptive neuro-fuzzy inference method was applied to simulate non-linear mapping among acceleration peak conditions. The neuro-fuzzy model included an approximate fuzzy reasoning through a sugeno fuzzy inference system (FIS). The input space was fuzzified by a grid-partitioning technique. A hybrid learning algorithm was selected in order to adapt the model's parameters. Furthermore, a linear-nonlinear regression analyses and neural network model were employed to observe the relative performances. Based on our findings, it can be concluded that the neuro-fuzzy control system exhibits a superior performance, compared to the other employed methods [19,20]. In the developed ANFIS model, input-space fuzzification was carried out via the grid-partitioning technique. Fuzzy variables were divided into four triangular membership functions *i>*2> x3* 3. The 625 fuzzy ‘if-then’ rules were set up where in the fuzzy variables were connected by the T-Norm (AND) apparatus. First order sugeno FIS was selected for the approximate reasoning process. The adjustment of independent parameters was made according to the batch mode based on the hybrid learning algorithm. The ANFIS model was trained for 50epochs until the observed error ceased to fluctuate. The resultant neuro-fuzzy Simulink model structure is illustrated in Figure 9.
The input space contains four parameters- moment magnitude (Mw), rupture distance, fault mechanism and site class. The output contains vertical components of PGA, including 40 records from different regions of the world, 24 records for training, 6 records for checking and 10 records for testing the selected ANFIS network. Sixty training data and sixty checking data pairs were obtained at first. The one used here contains 625 rules, with four membership functions being assigned to each input variable, having total number of 3185 fitting parameters which are composed of 60 premise parameters and 3125 consequent parameters. This section presents the simulation results of the proposed type-3 ANFIS with both batch (off-line) and pattern (on-line) learning. In the first example, ANFIS is used to model highly nonlinear functions, where by results are compared with the neural network approach and also with relevant earlier work. In the second example, the FIS name is PGA1 and the FIS type is sugeno. We used the 'and-or' method for input partitioning. Furthermore, we used ‘wtsum’ and 'wtaver' functions for defuzzification. The ranges of input and output variables- in other words, the target variables- are Mw=(5-8), R=(1.50-80 km), fault mechanism type =(1-5),site class=(1-5) and target range (PGA)=(0.5-2.50). The number of MFs={5 5 5 5}, the MF type=Trimf and G-bell MFs (Figure 9 $10). The result of this simulation is LSE: 0.002 and the final epoch error equals to 0.0000002.
Results of the ANFIS network
The input MFs for initial fuzzy inference system and the MFs of trained FIS are shown in Figure 11 & 12. The rule base for the designed ANFIS is shown in Figure 13. Finally, a trained FIS structure is created from the initial FIS by using the ANFIS GUI editor, which is depicted in Figure 14. Also, by testing the results, one can interpret Table 4. Fuzzy parameters used for training ANFIS are shown in Table 5. Also two membership functions for ANFIS training are shown in Figure 10.
Discussion on Results
Empirical recordings have had a significant influence on the estimation of the maximum physical ground motions that can be possible. Peak ground acceleration is an important factor which needs to be investigated before testing devastation potentials that can result from earthquakes in rebuilding sites. One of the problems that deserve attention by seismologists is the occurrence of earthquakes where of the ground motion acceleration peak unexpectedly appears to be more than 1g (Figure 15-18). Valuable data on some earthquakes have been used by Strasser [6] to investigate the earthquakes' physical processes and their consequences.
Figure 15a &b shows the acceleration and velocity traces of the horizontal components falling into this category for which the recordings were available. Spectra of pseudo-acceleration response, pertaining to damping by 5%, are also shown. All the examined traces are characterized by a very pronounced peak in the short-period (T<0.3s) range of the spectrum. The peak velocities that are associated with these recordings are less than 50cm/s. Slip distribution of focal mechanism for tohoko earthquake in Japan are shown in Figure 15c. The results of Gullo and Ercelebi's [2] research (2007) indicated that the fitting between the predicted PGA values by the networks and the observed ones yielded high correlation coefficients (R2). Furthermore, comparisons between the correlations by the ANN and the regression method showed that the ANN approach performed better than the regression method (Table 6). The developed ANN models can be used conservatively so as to establish a good understanding of the influence of input parameters for the PGA predictions.
In Strasser and Bommer's [6] research, a dataset of recordings was examined. It was characterized by the recordings' large amplitudes of PGA (1g) (Figure 15). A number of physical processes have been proposed in the literature to explain these large ground motions, which are commonly divided into source, path and site related effects. While it is often a matter of convention whether these are considered to be predominantly linked to ground motion generation (source effects) or propagation (path and site effects), particularly in the nearsource region, it is important to distinguish between factors that are event-specific, station-specific and record-specific, in terms of implications for ground motion predictions and thus seismic hazard assessment. This is because only site-specific effects can be predicted for certain, in advance. In the present paper, the ANN algorithm indicated that the fitting between the predicted PGA values by the networks and the observed PGA values could yield high correlation coefficients of 0.851for PGA ( 3). Moreover, comparisons between the correlations obtained by the ANN and the regression method demonstrated that the ANN algorithm performed better than the regressions. The Levenberg-Marquart gradient method which we applied on the training algorithm contributed dominantly to fitting the results well.
It had the potential to carry out training very quickly. Moreover, the network models developed in this paper offer new insights into attenuation studies for the purpose of estimating the PGA. In this study, ANFIS and FFBP models were developed to forecast the PGA in different regions of the world. The results of two models and the observed values were compared and evaluated based on their training and validation performance (Figures 2 & 4). The results demonstrated that ANFIS and FFBP models can be applied successfully to establish accurate and reliable PGA forecasting, when comparing the results of the two networks. It was observed that the value of R belonging to the FFBP models is high (0.78) (Figure 3). Moreover, the LSE values of the ANFIS model — which is 0.012 — were lower than that of the FFBP model (Table 4). Therefore, the ANFIS model could be more accurate than the FFBP model. However, a significant advantage is evident when predicting the PGAvia ANFIS, compared to the FFBP model (Figures 16 & 17). The simulations show that the ANFIS network is good for predicting maximum peak ground acceleration in some regions of the world. Finally, the minimum testing error- obtained for the ANFIS network- is 0.002 and the ultimate epoch error is 0.012 (Table 4). This conclusion shows that the ANFIS network can be suitable and useful for predicting values of peak ground acceleration for future earthquakes. PGA-predicted values versus record numbers for three neural networks are shown in Figure 18 & 19.
Conclusion
In this study, FFBP neural networks and ANFIS were trained so as to estimate peak ground acceleration in an area. The input variables in the ANN model were the magnitude, the rupture distance, the focal mechanism and site classification. The output was the PGA only. In the end, the minimum testing error was obtained for the ANFIS network, which equaled 0.002, and the mean square error for the FFBP neural network equaled 0.017. This conclusion shows that the ANFIS network can be suitable and useful in predicting peak ground acceleration for future earthquakes.
Acknowledgement
I am very grateful to the editors and anonymous reviewers, for their suggestions aimed at improving the quality of this manuscript. I also appreciate professors Strasser and Bommer for granting necessary data to this work.
To Know More About Biostatistics and Biometrics Open Access Journal Please Click on: https://juniperpublishers.com/bboaj/index.php
To Know More About Open Access Journals Publishers Please Click on: Juniper Publishers
0 notes
Link

If you put your ear to Mars, what would you hear? To find out, and to explore the unknown interior of Mars, NASA's Insight Lander deployed SEIS late last year, a sensitive seismometer that can detect marsquakes. In early April, after hearing the wind and motions initiated by the lander itself, SEIS recorded an unprecedented event that matches what was expected for a marsquake. This event can be heard on this YouTube video. Although Mars is not thought to have tectonic plates like the Earth, numerous faults are visible on the Martian surface which likely occurred as the hot interior of Mars cooled -- and continues to cool. Were strong enough marsquakes to occur, SEIS could hear their rumbles reflected from large structures internal to Mars, like a liquid core, if one exists. Pictured last week, SEIS sits quietly on the Martian surface, taking in some Sun while light clouds are visible over the horizon. via NASA https://go.nasa.gov/2F2DGBp
0 notes
Photo

Astronomy Picture of the Day: June 04, 2019 If you put your ear to Mars, what would you hear? To find out, and to explore the unknown interior of Mars, NASA's Insight Lander deployed SEIS late last year, a sensitive seismometer that can detect marsquakes. In early April, after hearing the wind and motions initiated by the lander itself, SEIS recorded an unprecedented event that matches what was expected for a marsquake. This event can be heard on this YouTube video. Although Mars is not thought to have tectonic plates like the Earth, numerous faults are visible on the Martian surface which likely occurred as the hot interior of Mars cooled -- and continues to cool. Were strong enough marsquakes to occur, SEIS could hear their rumbles reflected from large structures internal to Mars, like a liquid core, if one exists. Pictured last week, SEIS sits quietly on the Martian surface, taking in some Sun while light clouds are visible over the horizon. SEIS: Listening for Marsquakes via NASA https://go.nasa.gov/2F2DGBp
0 notes
Text
NASA's InSight Detects First Likely 'Quake' On Mars
NASA's Mars InSight lander has measured and recorded for the first time ever a likely "marsquake."

The faint seismic signal, detected by the lander's Seismic Experiment for Interior Structure (SEIS) instrument, was recorded on April 6, the lander's 128th Martian day, or sol. This is the first recorded trembling that appears to have come from inside the planet, as opposed to being caused by forces above the surface, such as wind. Scientists still are examining the data to determine the exact cause of the signal. "InSight's first readings carry on the science that began with NASA's Apollo missions," said InSight Principal Investigator Bruce Banerdt of NASA's Jet Propulsion Laboratory (JPL) in Pasadena, California. "We've been collecting background noise up until now, but this first event officially kicks off a new field: Martian seismology!" The new seismic event was too small to provide solid data on the Martian interior, which is one of InSight's main objectives. The Martian surface is extremely quiet, allowing SEIS, InSight's specially designed seismometer, to pick up faint rumbles. In contrast, Earth's surface is quivering constantly from seismic noise created by oceans and weather. An event of this size in Southern California would be lost among dozens of tiny crackles that occur every day. "The Martian Sol 128 event is exciting because its size and longer duration fit the profile of moonquakes detected on the lunar surface during the Apollo missions," said Lori Glaze, Planetary Science Division director at NASA Headquarters. NASA's Apollo astronauts installed five seismometers that measured thousands of quakes while operating on the Moon between 1969 and 1977, revealing seismic activity on the Moon. Different materials can change the speed of seismic waves or reflect them, allowing scientists to use these waves to learn about the interior of the Moon and model its formation. NASA currently is planning to return astronauts to the Moon by 2024, laying the foundation that will eventually enable human exploration of Mars. InSight's seismometer, which the lander placed on the planet's surface on Dec. 19, 2018, will enable scientists to gather similar data about Mars. By studying the deep interior of Mars, they hope to learn how other rocky worlds, including Earth and the Moon, formed. Three other seismic signals occurred on March 14 (Sol 105), April 10 (Sol 132) and April 11 (Sol 133). Detected by SEIS' more sensitive Very Broad Band sensors, these signals were even smaller than the Sol 128 event and more ambiguous in origin. The team will continue to study these events to try to determine their cause. Regardless of its cause, the Sol 128 signal is an exciting milestone for the team. "We've been waiting months for a signal like this," said Philippe Lognonné, SEIS team lead at the Institut de Physique du Globe de Paris (IPGP) in France. "It's so exciting to finally have proof that Mars is still seismically active. We're looking forward to sharing detailed results once we've had a chance to analyze them." Most people are familiar with quakes on Earth, which occur on faults created by the motion of tectonic plates. Mars and the Moon do not have tectonic plates, but they still experience quakes -- in their cases, caused by a continual process of cooling and contraction that creates stress. This stress builds over time, until it is strong enough to break the crust, causing a quake. Detecting these tiny quakes required a huge feat of engineering. On Earth, high-quality seismometers often are sealed in underground vaults to isolate them from changes in temperature and weather. InSight's instrument has several ingenious insulating barriers, including a cover built by JPL called the Wind and Thermal Shield, to protect it from the planet's extreme temperature changes and high winds. SEIS has surpassed the team's expectations in terms of its sensitivity. The instrument was provided for InSight by the French space agency, Centre National d'Études Spatiales (CNES), while these first seismic events were identified by InSight's Marsquake Service team, led by the Swiss Federal Institute of Technology. "We are delighted about this first achievement and are eager to make many similar measurements with SEIS in the years to come," said Charles Yana, SEIS mission operations manager at CNES. JPL manages InSight for NASA's Science Mission Directorate. InSight is part of NASA's Discovery Program, managed by the agency's Marshall Space Flight Center in Huntsville, Alabama. Lockheed Martin Space in Denver built the InSight spacecraft, including its cruise stage and lander, and supports spacecraft operations for the mission. A number of European partners, including CNES and the German Aerospace Center (DLR), support the InSight mission. CNES provided the SEIS instrument to NASA, with the principal investigator at IPGP. Significant contributions for SEIS came from IPGP; the Max Planck Institute for Solar System Research in Germany; the Swiss Federal Institute of Technology (ETH Zurich) in Switzerland; Imperial College London and Oxford University in the United Kingdom; and JPL. DLR provided the Heat Flow and Physical Properties Package (HP3) instrument, with significant contributions from the Space Research Center of the Polish Academy of Sciences and Astronika in Poland. Spain's Centro de Astrobiología supplied the temperature and wind sensors. Read the full article
0 notes
Text
Fiber Optic Seismic Array in Pasadena Tracked the Rose Parade
In November, Caltech and the City of Pasadena unveiled a new citywide fiber optic earthquake detector capable of mapping how temblors are shaking the city at millimeter-scale resolution.
A month and a half later, while millions of spectators watched floats and bands parade down Colorado Boulevard, Caltech researchers watched the seismic signal of those floats and bands, captured in fine detail by the array.
“The Rose Parade is an amazing cultural event in Pasadena, and the floats and bands run right on top of our newly established fiber seismic array,” says Zhongwen Zhan (MS ’08, PhD ’13), assistant professor of geophysics and the lead researcher on the fiber optic project. Zhan is the corresponding author of a paper about the Rose Parade’s seismic signature, published on May 6 in Seismological Research Letters.
The Pasadena Array takes advantage of two currently unused, or “dark,” strands of the City of Pasadena’s fiber optic cable network, which is typically buried just below ground and stretches in a large loop around the city. Two laser emitters shoot beams of light through the cables. The cables have tiny imperfections every few meters that reflect back a minuscule portion of the light to the source, where it is tracked and recorded. In this manner, each imperfection acts as a trackable waypoint along the fiber optic cable. Seismic waves moving through the ground cause the cable to expand and contract slightly, which changes the travel time of light to and from these waypoints. Thus, the imperfections act like individual “virtual” seismometers that allow seismologists to observe the motion of seismic waves. The technique is known as Distributed Acoustic Sensing, or DAS.
The Pasadena Array has more than 5,000 of these virtual sensors; about 400 were positioned along the Rose Parade route. Zhan found that heavy floats—those weighing about 16,000 to 18,000 kilograms—produced distinct long-period signals, while marching bands produced weaker long-period signals but strong signals in the 1.0–10 Hz frequency band.
Digging into the data, Zhan was able to spot the most ground-shaking marching bands: those from Southern University and A&M College, and the hometown Pasadena City College Honor Band. He also detected the gap caused when the float “Mrs. Meyer’s Clean Day” got stuck at the corner of Colorado and Sierra Madre boulevards for six minutes.
“The 2020 Rose Parade provided a rare calibration opportunity with well-controlled unidirectional traffic and heavy, slow-moving vehicles right on top of a section of the array. The Pasadena Array performed as well as expected,” Zhan says.
The study is titled “Rose Parade seismology: signatures of floats and bands on optical fiber.” Caltech co-authors are postdoctoral scholar Xin Wang and graduate student Ethan F. Williams (MS ’19); other co-authors include Martin Karrenbach at OptaSense Inc.; Miguel González Herráez at the University of Alcalá, Spain; and Hugo Fidalgo Martins at CSIC, Spain. This research was supported by a five-year agreement with the City of Pasadena to use the dark fiber; a National Science Foundation CAREER Award; and the Caltech-JPL President’s and Director’s Research and Development Fund (PDRDF).
source https://scienceblog.com/516172/fiber-optic-seismic-array-in-pasadena-tracked-the-rose-parade/
0 notes
Text
The National Aeronautics and Space Administration shares this fantastic image: SEIS: Listening for Marsquakes
The National Aeronautics and Space Administration shares this fantastic image: SEIS: Listening for Marsquakes
Deep space is amazing, and NASA continuously deliver stunning images we can all enjoy.
SEIS: Listening for Marsquakes
by NASA
(more…)
View On WordPress
0 notes
Text
NASA's InSight detects first likely 'quake' on Mars
NASA's Mars InSight lander has measured and recorded for the first time ever a likely "marsquake."

The faint seismic signal, detected by the lander's Seismic Experiment for Interior Structure (SEIS) instrument, was recorded on April 6, the lander's 128th Martian day, or sol. This is the first recorded trembling that appears to have come from inside the planet, as opposed to being caused by forces above the surface, such as wind. Scientists still are examining the data to determine the exact cause of the signal.
youtube
"InSight's first readings carry on the science that began with NASA's Apollo missions," said InSight Principal Investigator Bruce Banerdt of NASA's Jet Propulsion Laboratory (JPL) in Pasadena, California. "We've been collecting background noise up until now, but this first event officially kicks off a new field: Martian seismology!"
The new seismic event was too small to provide solid data on the Martian interior, which is one of InSight's main objectives. The Martian surface is extremely quiet, allowing SEIS, InSight's specially designed seismometer, to pick up faint rumbles. In contrast, Earth's surface is quivering constantly from seismic noise created by oceans and weather. An event of this size in Southern California would be lost among dozens of tiny crackles that occur every day.
"The Martian Sol 128 event is exciting because its size and longer duration fit the profile of moonquakes detected on the lunar surface during the Apollo missions," said Lori Glaze, Planetary Science Division director at NASA Headquarters.
NASA's Apollo astronauts installed five seismometers that measured thousands of quakes while operating on the Moon between 1969 and 1977, revealing seismic activity on the Moon. Different materials can change the speed of seismic waves or reflect them, allowing scientists to use these waves to learn about the interior of the Moon and model its formation. NASA currently is planning to return astronauts to the Moon by 2024, laying the foundation that will eventually enable human exploration of Mars.
InSight's seismometer, which the lander placed on the planet's surface on Dec. 19, 2018, will enable scientists to gather similar data about Mars. By studying the deep interior of Mars, they hope to learn how other rocky worlds, including Earth and the Moon, formed.
Three other seismic signals occurred on March 14 (Sol 105), April 10 (Sol 132) and April 11 (Sol 133). Detected by SEIS' more sensitive Very Broad Band sensors, these signals were even smaller than the Sol 128 event and more ambiguous in origin. The team will continue to study these events to try to determine their cause.
Regardless of its cause, the Sol 128 signal is an exciting milestone for the team.
"We've been waiting months for a signal like this," said Philippe Lognonné, SEIS team lead at the Institut de Physique du Globe de Paris (IPGP) in France. "It's so exciting to finally have proof that Mars is still seismically active. We're looking forward to sharing detailed results once we've had a chance to analyze them."
Most people are familiar with quakes on Earth, which occur on faults created by the motion of tectonic plates. Mars and the Moon do not have tectonic plates, but they still experience quakes -- in their cases, caused by a continual process of cooling and contraction that creates stress. This stress builds over time, until it is strong enough to break the crust, causing a quake.
Detecting these tiny quakes required a huge feat of engineering. On Earth, high-quality seismometers often are sealed in underground vaults to isolate them from changes in temperature and weather. InSight's instrument has several ingenious insulating barriers, including a cover built by JPL called the Wind and Thermal Shield, to protect it from the planet's extreme temperature changes and high winds.
SEIS has surpassed the team's expectations in terms of its sensitivity. The instrument was provided for InSight by the French space agency, Centre National d'Études Spatiales (CNES), while these first seismic events were identified by InSight's Marsquake Service team, led by the Swiss Federal Institute of Technology.
"We are delighted about this first achievement and are eager to make many similar measurements with SEIS in the years to come," said Charles Yana, SEIS mission operations manager at CNES.
JPL manages InSight for NASA's Science Mission Directorate. InSight is part of NASA's Discovery Program, managed by the agency's Marshall Space Flight Center in Huntsville, Alabama. Lockheed Martin Space in Denver built the InSight spacecraft, including its cruise stage and lander, and supports spacecraft operations for the mission.
A number of European partners, including CNES and the German Aerospace Center (DLR), support the InSight mission. CNES provided the SEIS instrument to NASA, with the principal investigator at IPGP. Significant contributions for SEIS came from IPGP; the Max Planck Institute for Solar System Research in Germany; the Swiss Federal Institute of Technology (ETH Zurich) in Switzerland; Imperial College London and Oxford University in the United Kingdom; and JPL. DLR provided the Heat Flow and Physical Properties Package (HP3) instrument, with significant contributions from the Space Research Center of the Polish Academy of Sciences and Astronika in Poland. Spain's Centro de Astrobiología supplied the temperature and wind sensors.
#science#science news#Mars#Marsquake#Asteroseismology#seismology#featured#NASA#space exploration#insight mission
23 notes
·
View notes
Text
Assignment #3
Early warning system for Disaster management in rural area(2015)
Z.N. Khalil Wafi , Mohd Fareq Abd.Malek, Sateaa Hikmat alnajjar, R.Badlishah Ahmad
"The stakeholder of ICT Information & Communication Technology is facing problems and challenges in identifying the necessary requirements for the proper design of communications in rural areas, the need of early alarm system in these area has been increase to reduce the gab of communication problems, scientists and engineers start to do researches to cover the problem by using new communication methods to ensure the connection with people in rural area especially during disasters such as floods, fires, storms and other disasters [3]"
My study relates about giving the information in rural areas by giving information to the people in this areas through radio or sending sms warning for the upcoming disaster.
-------------------------------------------------------------------
Earthquake Early Warning System by IOT using Wireless Sensor Networks(2016)
Alphonsa A.,Ravi G.
"In this paper, we propose an earthquake early warning system by means of an IOT in WSN. The sensors are placed in the surface of the earth. When an earthquake occurs, both compression P wave and transverse S wave radiates outward the epicenter of the earth. The P wave, which travels fastest, trips the sensors, placed in the landscape. It causes early alert signals to be transfer ahead, giving humans and automated electronic system a warning to take precautionary actions. So that before the damage begins with the arrival of the slower but stronger S waves, the public are warned earlier.[4]"
In this study the case is the prediction in earthquake disaster, a warning system by IOT using WSN. My study is also related to my study that when the system detects a earth motion in the ground using a seismometer, the system then give the information annd providing an early warning before the earthquake arrive in the region.
-------------------------------------------------------------------
A Development and Execution Environment for Early Warning Systems for Natural Disasters(2015)
Bartosz Balis, Tomasz Bartynski, Marian Bubak, Grzegorz Dyk, Tomasz Gubala and Marek Kasztelnik
"Early Warning Systems (EWS) may become a powerful tool for mitigating the negative impact of natural disasters, especially when combined with advanced IT solutions – such as on-demand scenario simulations, semi-automatic impact assessment, or real-time analysis of measurements from in-situ sensors. However, such complex systems require a proper computing environment supporting their development and operation. We propose the Common Information Space (CIS), a software framework facilitating design, deployment and execution of early warning systems based on real-time monitoring of natural phenomena and computationally intensive, time-critical computations.[5]"
Their study have a function in monitoring the natural phenomenon and time-critical computations, and give predictions for natural disaster to occur in the specific place, it correlates in my study in terms of warning systems giving the awareness and preparedness for the people.
-------------------------------------------------------------------
A Self Adaptive Telemetry Station for Flash Flood EarlyWarning Systems(2017)
Autanan Wannachai, Paskorn Champrasert, Somrawee Aramkul
"This paper proposed a self adaptive telemetry station, SATS, that can automatically adjust its operation mode to the environmental conditions.An automatic environmental condition learning and evaluation mechanism, Auto-ELE, is also proposed in this paper.[6]"
This system also helps for flash flood disaster, it has significance to my study for it is both warning systems although this study is unique for it is using a self adaptive telemetry station, my study focuses on alarming and sending warning alarms to the resident.
-------------------------------------------------------------------
EARTHQUAKE EARLY WARNING SYSTEM USING REAL-TIIME SIGNAL PROCESSING(2002)
Richard R. Leach Jr., Farid U. Dowla
"The warning system is designed to analyze the first-arrival from the three components of an earthquake signal and instantaneously provide a profile of impending ground motion, in as little as 0.3 sec after first ground motion is felt at the sensors. For each new data sample, at a rate of 25 samples per second, the complete profile of the earthquake is updated.[7]"
Just like the other warning systems, its relates to my study for it gives an accurate and informative warning to mitigate catastrophic ground motion and to give an early warning the people and be prepared for the disaster to occur.
RRL
Early Warning System for Disaster by IOT using broadcasting.
Disaster is a big problem to the society and most of them are natural disaster which cannot be stopped, but can be predicted by the help of warning systems. Some of these systems are used in different kinds of disaster like hurricanes, tsuanmi, earthquakes these natural disaster can be predicted to prevent and reduce the damage of the disaster when it happens.Most current natural disaster warning systems are based on remote sensors that depend on certain characteristics of natural disasters[1]. There are some research project takes root in the making of a system of monitoring for early warning systems that generates a Transport Stream (TS) signals with the Emergency Warning Broadcast System (EWBS) code [2].In this cases, a Warning system composing of different sensors that predicts every different disaster in their specific characteristic behaviour will be a big help, and also using Emergency Warning Broadcast System(EWBS) and Transport Stream(TS) for alarming the residents in the place where the disaster takes place. This system will be helpfull not just to monitor the disaster and alsp giving the alarm to the people and to prevent life loss and great destruction of the society.
REFERENCES
[1] B.c. Ko, H.J. Hwang, and J.Y. Nam, "Nonparametric membership functions and fuzzy logic for vision sensor-based flame detection," Journal of Optical Engineering, vol. 49, pp.2-11, 23, 2010
[2] ARIB STANDARD, Service Information for Digital Broadcasting System, Version 4.6, 2008.
[3] Shibata, Y.; Sato, Y.; Ogasawara, N.; Chiba, G.; “A Disaster Information System by Ballooned Wireless Adhoc Network “,Complex, Intelligent and Software Intensive Systems, 2009. CISIS '09. International Conference on 16-19 March 2009, P 299 - 304 Fukuoka ,ISBN: 978-1-4244-3569-2 , Accession Number: 10702165
[4] Poslad S, Middleton S.E., Chaves F., Ran Tao Necmioglu O and Bugel U., “A Semantic IOT Early Warning System for Natural Environment Crisis Management”, IEEE Transaction on Emerging in Computing, vol. 3, Issue 6, pp. 246-257, 2015.
[5] B. Balis, M. Kasztelnik, M. Bubak, T. Bartynski, T. Gubała,P. Nowakowski, and J. Broekhuijsen, “The UrbanFlood Common Information Space for Early Warning Systems,” Procedia Computer Science,vol. 4, pp. 96–105, 2011, proceedings of the International Conference on Computational Science, ICCS 2011.
[6] M. Castillo-Effer, D. H. Quintela, W. Moreno, R. Jordan, and W. Westhoff. Wireless sensor networks for flash-flood alerting. In Devices, Circuits and Systems, 2004. Proceedings of the Fifth IEEE International Caracas Conference on, volume 1, pages 142–146. IEEE, 2004.
[7] Anderson, J. G., and Q. Chen (1995). Beginnings of Earthquakes in the Mexican Subduction Zone on Strong-Motion Accelerograms. Bull. Seism. Soc. Am. 85,1 107-1 11 5.
0 notes
Text
Is This Completion Of Nuclear Electrical Power Beyond China And Russia?
Stargazers have actually discovered almost 500 earthly devices along with a number of worlds. Food preparation in the New Moon is actually practically difficult, as well as the trip through the steep, strong winding hills ends up being everything however pleasant. This week-long stage the subsiding Moon rises at twelve o'clock at night (in the East) and is actually straight above at sunup. Stephen: Just like bein' a really good purchases person, the greatest purchases people use their ears greater than their mouth. It is two nights prior to the brand-new moon, the night of the brand-new moon, as well as 2 evenings after the brand-new moon. The Moon has long provided fascination for the mankind, from the myriad of lunar gods and goddesses to theological schedules found out due to the phases from the Moon because in the past created record. Similarly, if you were birthed during the course of the third quarter of the series of days in Capricorn, from January 7th by means of January 14th, your privileged moon days are those that drop throughout the Moon. Like many photo voltaic eclipses, this set are going to be greatest noticed off the slender band in the world's surface where the shade from the moon falls. A lot of women may synchronize along with the patterns of the moon in about three to four months by affecting the mild level while they sleep. fit-behappyblog.info Roy Rogers, the cowboy star, possesses his natal Moon listed here and he was showcased in over one hundred flicks along with his Palamino and German Guard. This is a really black-and-white, all-or-nothing moon with exceptionally taken care of ideas regarding factors. As they rotate, occasionally pair of or even more from the noticeable earths (and or even the moon) set all together or even line up in a little viewing location of the skies - a relatively popular occurrence. You could get hold of many covert spots that internet search engine continually cannot reveal you when searching for moon as well as celebrity designs. Where the Sunshine behaves, the Moon responds; the Moon is our casual as well as natural responses. Seismometers that were actually left on the Moon by Beauty rocketeers, documented the activity from the moon's inner parts up until 1977. Uncovering the moon is actually a burning need of human beings so the Lunar Exploration Orbiter (LRO), NASA's youngest moon probe, assists deliver our team some outstanding truths concerning the lunar body such as there are lots of water ice being actually discovered at the moon's north post and somewhere else. A person along with moon in Aquarius is actually finest satisfied to become an expert or business person, for he could swiftly conceptualize ideas to merely innovate or developed just about anything. When I was in university at Eastern Kentucky College in the overdue 1970s, my fascination with Moon Landrieu began. Early man looked on the moon as a grandma siren; they felt the moon moderated pregnancies and menstrual circulations. Thus visit HuffPost Good Headlines Listed below's wishing that triggers copycat actions of excellent news reporting around the media. Looks like a regression to the moon to me. If the S&P FIVE HUNDRED moves up 5%, down 5%, or stays unchanged, Netflix is going to perhaps ... effectively, I don't understand. Solar system in 10th and also aspected/associated through Moon might provide mass help to the spiritual motion from the applicant.
A remarkable trine between the moon in the primary sky sign of Libra and also Neptune in the taken care of air indicator of Aquarius on the morning from Thursday, September 1st, could develop mental and also mental consistency that is actually as close to excellent as you can easily obtain.
0 notes
Text
Want to pursue mechanical engineering? Know More About It.

Before starting off with Mechanical Engineering, I would like to tell you about an event called ABN Vidhya Jyothi, which is happening across Andhra Pradesh this year, where you will find a solution to every problem that you face as a student for FREE of cost. There will be exam tips, career counseling provided to all the students. Over 20 top colleges in the country under one roof and over 200 courses to choose from at ABN Vidhya Jyothi. Some of the best colleges in the country are taking part in the event.
Register now for ABN Vidhya Jyothi by clicking on the link below:-
http://www.vidhyajyothi.in/
Or you can register by calling
89706 36149, 97423 45039
The mechanical engineering field requires an understanding of core areas including mechanics, dynamics, thermodynamics, materials science, structural analysis, and electricity. In addition to these core principles, mechanical engineers use tools such as computer-aided design (CAD), and product lifecycle management to design and analyze manufacturing plants, industrial equipment and machinery, heating and cooling systems, transport systems, aircraft, watercraft, robotics, medical devices, weapons, and others. It is the branch of engineering that involves the design, production, and operation of machinery.
Mechanical engineering emerged as a field during the Industrial Revolution in Europe in the 18th century; however, its development can be traced back several thousand years around the world. In the 19th century, developments in physics led to the development of mechanical engineering science. The field has continually evolved to incorporate advancements; today mechanical engineers are pursuing developments in such areas as composites, mechatronics, and nanotechnology. It also overlaps with aerospace engineering, metallurgical engineering, civil engineering, electrical engineering, manufacturing engineering, chemical engineering, industrial engineering, and other engineering disciplines to varying amounts. Mechanical engineers may also work in the field of biomedical engineering, specifically with biomechanics, transport phenomena, biomechatronics, bionanotechnology, and modeling of biological systems.
The application of mechanical engineering can be seen in the archives of various ancient and medieval societies. In ancient Greece, the works of Archimedes (287–212 BC) influenced mechanics in the Western tradition and Heron of Alexandria (c. 10–70 AD) created the first steam engine (Aeolipile). In China, Zhang Heng (78–139 AD) improved a water clock and invented a seismometer, and Ma Jun (200–265 AD) invented a chariot with differential gears. The medieval Chinese horologist and engineer Su Song (1020–1101 AD) incorporated an escapement mechanism into his astronomical clock tower two centuries before escapement devices were found in medieval European clocks. He also invented the world's first known endless power-transmitting chain drive.
During the Islamic Golden Age (7th to 15th century), Muslim inventors made remarkable contributions in the field of mechanical technology. Al-Jazari, who was one of them, wrote his famous Book of Knowledge of Ingenious Mechanical Devices in 1206 and presented many mechanical designs. He is also considered to be the inventor of such mechanical devices which now form the very basic of mechanisms, such as the crankshaft and camshaft.
During the 17th century, important breakthroughs in the foundations of mechanical engineering occurred in England. Sir Isaac Newton formulated Newton's Laws of Motion and developed Calculus, the mathematical basis of physics. Newton was reluctant to publish his works for years, but he was finally persuaded to do so by his colleagues, such as Sir Edmond Halley, much to the benefit of all mankind. Gottfried Wilhelm Leibniz is also credited with creating Calculus during this time period.
During the early 19th century industrial revolution, machine tools were developed in England, Germany, and Scotland. This allowed mechanical engineering to develop as a separate field within engineering. They brought with them manufacturing machines and the engines to power them. The first British professional society of mechanical engineers was formed in 1847 Institution of Mechanical Engineers, thirty years after the civil engineers formed the first such professional society Institution of Civil Engineers. On the European continent, Johann von Zimmermann (1820–1901) founded the first factory for grinding machines in Chemnitz, Germany in 1848.
In the United States, the American Society of Mechanical Engineers (ASME) was formed in 1880, becoming the third such professional engineering society, after the American Society of Civil Engineers (1852) and the American Institute of Mining Engineers (1871). The first schools in the United States to offer an engineering education were the United States Military Academy in 1817, an institution now known as Norwich University in 1819, and Rensselaer Polytechnic Institute in 1825. Education in mechanical engineering has historically been based on a strong foundation in mathematics and science.
0 notes