#it's wild how intelligent commentary just stays relevant
Explore tagged Tumblr posts
Text
youtube
Helmet - Aftertaste - Driving Nowhere
#helmet#aftertaste#driving nowhere#it's wild how intelligent commentary just stays relevant#this song is from 1997 and was in response to the oklahoma city bombing#i think specifically the reaction to it based on the quote from page hamilton#Youtube
2 notes
¡
View notes
Note
I'm so freaking tired of anti Mary fans. I was in a heated argument with some of them (no hate, we're all on good terms with each other), & it's just, they don't wanna understand, they think that the writers had NO REASON to bring back Mary (like, wut?), & that she was a bad mom & HOW DARE she leave her grown ass children that she doesn't even know. & ofc the writers are out to destroy my fave tv show & j2m know their characters best & should teach the writers there job apparently *sigh*
*fart noise* Iâm sorry youâre running into that with your friends :(Â
If it can help at all, can you go with the angle that it seemed clear that Carverâs vision was to bring back Mary all along, stretching way back, and itâs possible they were always wondering if it was possible but this was how they found to do it. There was a lot of meta after season 10 and in the beginning of season 11 about Mary and her relevance to the current arc⌠For example without going delving into more complicated places, I trawled my 1x01 tag and found these posts about Carverâs loops back to the start which show an increasing awareness that with hindsight you can see that we were pretty bang on with understanding the thematic reasons, but largely assumed that if Dean was going to confront Mary it would be in a symbolic way - e.g. the photos in 10x23, Amara as a *spectre* of the Original Fridged Woman without actually getting back to her. In fact as late as 11x22 we were writing about how Amara came across as The Original Fridged Woman and her angry ranting was exactly a commentary on what had happened to Mary - and we were in no way ready for her to actually come back and put money where their mouth was on this theme! So a year later Berens got to utterly rip our hearts out by having the actual words spoken to the actual Mary instead of via symbolismâŚ
https://elizabethrobertajones.tumblr.com/post/94087411528/dustydreamsanddirtyscars-starborndean-crowley
https://elizabethrobertajones.tumblr.com/post/127722435903/rainbofiction-rainbofiction-but-i-cant-stop
https://elizabethrobertajones.tumblr.com/post/128772693689/themegalosaurus-sam-not-knowing-his-mother
https://elizabethrobertajones.tumblr.com/post/130898528313/dustydreamsanddirtyscars-season-1-vs-season-11
https://elizabethrobertajones.tumblr.com/post/131013833113/oh-i-just-thought
https://elizabethrobertajones.tumblr.com/post/132800927293/green-circles-101-vs-1105
https://elizabethrobertajones.tumblr.com/post/133128335053/postmodernmulticoloredcloak
After Mary came back there were a lot more posts making the actual connections with the hindsight and explaining it all over again but with the context that Carver era had been a build up to bring her back for the real emotional conflict that needed to be played out, and those posts were in my tag too but I picked posts from before that specifically because itâs so intriguing to me how close we were in some ways about the thematic reasons she would come back, or for events which were linked to her and her story, and how none of what has happened with her since she returned is some new wild thing, but all addressing stuff based on past canon, themes built up for years beforehand and always rooted in the very based of the show (hence pulling these posts from my 1x01 tag just to be most petty when I could have gone 10x23/11x01 and found a lot more).Â
Knowing the history of the themes of the show feels really important to me in the sense that I never think old meta is wasted or outdated just because more canon has come out, because saying something really astute without knowing that Mary is coming back is often in some ways more evidence for the worth of bringing her back than the actual argument of why she should stay now that she is back from the POV of knowing it happened and arguing with the decision about if it was the right one or not.Â
When I say that Mary is really firmly embedded in the themes of the show, I know she is because weâd been explaining them for years, and years before I got here as well into fandom, and so when she arrived, everything that she stood for was ALREADY understood by those of us whoâd thought about it already and nothing thatâs happened with her has actually been that wild, and all of it has been covering long over-due character interactions and reckonings and whatnot. Stuff that was built deep into the DNA of the show. I can think of dozens of examples off the top of my head which I wonât go into that reference Mary in season 11 that might not have been in the tag I looked through but I can tell you where else she âwasâ in season 11. Because they were building up to her arrival, and it was all done in Sam and Deanâs characterisation.Â
And this is all just rambling very specifically about themes and not really going into other stuff like the intelligence of bringing her back and using Amara to set up her arc with the story of her as the original fridged woman and the inherent misogyny in denying her her story, and of course Amaraâs sympathy to that as she chooses to bring Mary back to give Dean his own reckoning just as she had needed to confront her brother, so Dean needs to confront his mother. It wasnât about GIVING her to Dean just as a trophy, but in the character building it would give him to address everything about what Mary had become to him.Â
So even viewing Mary through a very narrow lens of what she gives to Dean or Sam in their missing parent they want back sheâs actually doing exactly what sheâs supposed to and the show is approaching it with the correct themes. In order for Mary and Sam to come to an understanding, they brought back yellow eyed demons and then moved on to Jack, both of which confront Samâs original arc, but allow Mary a chance to experience this stuff for herself, and what she did to Sam.Â
Itâs inherently thematically solid, even if people donât like the character, or the writing when it comes to dialogue and decisions, or whatever else. As a part of the story, she BELONGS, and it is being well-told on a structural, thematic level, which more than justified why they would bring her back and why they needed to, years before she ever showed up.Â
I think Iâve made more than enough rants about how to sympathise with her, but maybe this will help with the whole reason for bringing her back and how she actually does have a purpose and is a real part of the story and fits comfortably if you let her. :)Â
57 notes
¡
View notes
Photo
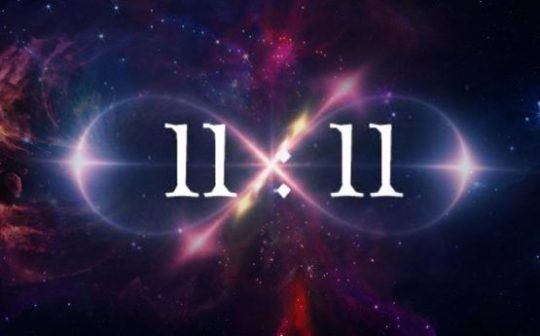
New Post has been published on https://lovehaswonangelnumbers.org/the-white-of-novembers-1111-gateway/
The White of November's 11:11 Gateway!!
The White of Novemberâs 11:11 Gateway!!
By Lisa Gawlas
This has been a truly wild ride these last few months. No doubt, we are not about to slow down any time soon. (I really wish it would though lol.) I have not put out any sharings 1, because there was nothing new for me to share and 2, I have been babysitting my grandson while my daughter recovers from surgery and her husband works day-shift. I am out of the house by 4:50 am on most days.
My voice came back just in time for November to start. And what a weird November it is already. The first day of November all I could see in what I call âpreviewsâ (imagery before the call happens) is what looks like a spotlight shining down on the earth and flooding it with light. The light is so bright I cannot see thru it. This was consistent for every person on my schedule. The next day, we got an addition within the preview⌠a left arm (physical reach for life) with a wristwatch on it. The numbers of the watch were blobby and blurry, there were no minute or hour hands. The only thing I can understand about this consistent imagery is⌠we tend to look at the clock, at the time, the time it takes (for anything) and now, time is becoming less and less relevant in our reach for life. What we want or need.
Yesterday in the previews (no one has had an actual reading yet) it really became interesting because each person added a different element to the preview (unlike the previous 2 days.)
My first ladâs preview was an opening of a curtain, very much like the opening of a play. The curtains themselves were extremely white and what was thru them was another layer of (a softer) white light. Nothing I could see thru tho.
My next lady offered a silhouette pacing back and forth behind the softer white light. So my guess is that the softer while the light is a secondary curtain to the first one. Like a set of shears behind the brighter ones.
I am now wondering if the pacing is us⌠waiting, wondering and yet, the time is blurred, irrelevant to our desires, for now, information lol. And trust me, I want to know NOW!! lol
My third lady offered something incredible⌠the only thing of color besides white and silhouette. It was a ring/crown of brilliantly colored flowers. Flowers reminiscent of Hawaiian leis. I could feel the thickness of their petals. This ring of flowers was about the side around as a Frisbee (going to scale of my vision of course) and hung in the center of the stage a couple of feet above where this image of pacing was.
I kept feeling the energy of the crowning of the virgin mary (why I have no idea lol..) Maybe it is the purity of the light and the brilliance (flower colors) of what is to come of the whiteness. Of course, we are heading into and through the most significant 11:11 gateway ever.
Well, my day has already started with readings and I am only getting the finger wag⌠meaning, not seeing anything. I have a deep inner feeling each day closer to 11:11 the frequency of light is getting more and more intense. Which alone feels exciting, but not when looking directly into it.
Sadly, with the chaos of my days driving to my daughters, not having a voice, babysitting and days not getting home until after 7 pm, I have been really really negligent in my emails and many other things. The times I am at home I tend to take very long (and needed) naps. And just so no one worries, my daughter is fine, she got new boobs put in and cannot lift anything until a week or so from now, including her 26-pound chunky monkey lol. So I did not block off the moon periods or even 11:11, and those days are all booked up. I would strongly advise rescheduling coming into and on the 11:11 (I have a feeling beyond that will be ok) or stay there and we can try and see what we can see.
A few days before m voice came back, I was sitting on the couch and tilted my head to the left and it felt like I dunked my head underwater and my right ear filled up. The next day, the same thing happened with my left ear, Both are still pressurized, with my right ear being super intense and both ringing like crazy. It will be 2 weeks Thursday this âear thingâ has been consistent. My doc gave me antibiotics which helped everything except the ears.
I actually broke down and begged my team to help me understand what is happening. They gave me a dream just before November started. I was painting someoneâs ceiling white. In this dream, all I could think about is how great it would be if my son painted my ceilings before I move (I move out by the end of this month.)
We are all working to purify the ceilings of life, raising the frequency for ALL. When work is being done, it is not a time to understand but allow. This is what all the previews in November are reminding us of⌠allow. Time is irrelevant (unless you have bills to pay lol.) and life is getting a new coat of paint at the highest level for all of us.
On my car ride from daycare to home this morning, I was equally reminded of how incredibly intelligent and communicative our cellular body is and will do all it can to push us where we need to go.
I got insurance on the 1st of Oct and had not yet contacted an ENT to explore my chronic, persistent voice loss. Well, with my ears in the condition they are now in, I called yesterday. The first thing they asked me is if I had an MRI done on my throat and head at all. Nope. My appointment is for Nov 20th!!
I am also being asked to remind all of us to stop thinking we are doing something wrong, or out of alignment, or not working on issues. Sometimes the light itself is so intense, the body reacts the only way it can as it undergoes change.
WE ARE UNDERGOING CHANGE!!!
On that note⌠I love you all so much and for those of you willing to endure my rescheduling, thank you from the deepest part of my heart and soul.
Oh, and we have decided to change the day and time of our Nations class so we do not have to cancel it. Right now we are looking at Wednesdays at 3 pm and will be confirmed tonight when we meet. So the Nations Tuesday night classes live on (only on another day and time lol.)
Big big big ((((HUGZ)))) filled with light, love and pure excitement to and thru ALL!!
Lisa Gawlas
~~~~~~~~~
LoveHasWon.org is a Non-Profit Charity, Heartfully Associated with the âWorld Blessing Church Trustâ for the Benefit of Mother Earth
Share Our Messages with Love and Gratitude
LOVE US @ MeWe mewe.com/join/lovehaswon
Visit Our Online Store for Higher Consciousness Alternative Medicine, Products and Tools: Gaiaâs Whole Healing Essentials
Visit Our Sister Site for Angel Number guidance and astrology: LoveHasWon Angel Numbers
Commentary from The First Contact Ground Crew 5dSpiritual Healing Team:
Feel Blocked, Drained, Fatigued, Restless, Nausea, Achy, Ready to Give Up? We Can Help! We are preparing everyone for a Full Planetary Ascension, and provide you with the tools and techniques to assist you Home Into The Light. The First Contact Ground Crew Team, Will Help to Get You Ready For Ascension which is Underway. New Spiritual Sessions have now been created for an Entire Family, including the Crystal Children; Group Family Healing & Therapy. We have just began these and they are incredible. Highly recommend for any families struggling together in these times of intense changes. Email:Â [email protected]Â for more information or to schedule an emergency spiritual session. We can Assist You into Awakening into 5d Reality, where your experience is one of Constant Joy, Wholeness of Being, Whole Health, Balanced, Happy and Abundant. Lets DO THIS! Schedule Your Session Below by following the Link! Visit:Â Â http://www.lovehaswon.org/awaken-to-5d/
Introducing our New LoveHasWon Twin Flame Spiritual Intuitive Ascension Session. Visit the link below:
https://lovehaswon.org/lovehaswon-twin-flame-spiritual-intuitive-ascension-session/
Request an Astonishing Personal Ascension Assessment Report or Astrology Reading, visit the link below for more information:
https://lovehaswon.org/lovehaswon-ascension-assessment-report
https://lovehaswon.org/lovehaswon-astrology/
           Experiencing DeAscension Symptoms, Energy Blockages, Disease and more? Book a Holistic Healing Session
https://lovehaswon.org/lovehaswon-holistic-healing-session/
To read our Testimonials you can follow this link:Â http://www.lovehaswon.org/testimonials
Connect with MotherGod~Mother of All Creation on Skype @ mothergoddess8
Request a copy of our Book:Â The Tree of Life ~ Light of The Immortals Book
Order a copy of Our LoveHasWon Ascension Guide: https://lovehaswon.org/lovehaswon-ascension-guide/
Gaiaâs Whole Healing Essentials ~ Higher Consciousness Products and Tools to Support Inner Healing, Self-Empowerment, Expansion and Spiritual Growth https://gaiaswholehealingessentials.org/
**If you do not have a Paypal account, click on the button below:
If you wish to donate and receive a Tax Receipt, click the button below:
Donate with Paypal
 Use Cash App with Our code and weâll each get $5! FKMPGLH
Cash App Tag: $lovehaswon1111
Cash -App
Donate with Venmo
VENMO
Thank you so much for Supporting Our Gaiaâs Whole Healing Gofundme CampaignÂ
https://www.gofundme.com/f/gaias-whole-healing-essentialsÂ
 Support Us Through Our LoveHasWon Wish List
LoveHasWon Wish List
We also accept Western Union and Moneygram. You may send an email to [email protected] for more information.
***If you wish to send Donations by mail or other methods, email us at [email protected]  or  [email protected]***
**** We Do Not Refund Donations****
MeWe ~ Youtube ~ Facebook ~ Apple News ~ Linkedin ~ Twitter ~ Tumblr ~ GAB ~ Minds ~ Google+ ~ Medium ~ Mix ~ Reddit ~ BlogLovin ~ Pinterest ~ Instagram ~ Snapchat
0 notes
Text
4 trends in security data science for 2018

A glimpse into what lies ahead for response automation, model compliance, and repeatable experiments.
This is the third consecutive year Iâve tried to read the tea leaves for security analytics. Last yearâs trends post manifested well: from a rise in adversarial machine learning (ML) to the deep learning craze (such that entire conferences are now dedicated to this subject). This year, Hyrum Anderson, technical director of data science from Endgame, joins me in calling out the trends in security data science for 2018. We present a 360-degree view of the security data science landscapeâfrom unicorn startups to established enterprises.
The format remains mostly remains the same: four trends to map to each quarter of the year. For each trend, we provide a rationale about why the time is right to capitalize on the trend, offer practical tips on what you can do now to join the conversation, and include links to papers, GitHub repositories, tools, and tutorials. We also added a new section âWhat wonât happen in 2018â to help readers look beyond the marketing material and stay clear of hype.
1. Machine learning for response (semi-)automation
In 2016, we predicted a shift from detection to intelligent investigation. In 2018, weâre predicting a shift from rich investigative information toward distilled recommended actions, backed by information-rich incident reports. Infosec analysts have long stopped clamoring for âmore alerts!â from security providers. In the coming year, weâll see increased customer appetite for products to recommend actions based on solid evidence. Machine learning has, in large part, proven itself a valuable tool for detecting evidence of threats used to compile an incident report. Security professionals subconsciously train themselves to respond to (or ignore) the evidence of an incident in a certain way. The linchpin to scale in information security rests still on the information security analyst, and many of the knee-jerk responses can be automated. In some cases, the response might be ML-automated, but in many others it will be at least ML-recommended.
Why now?
The information overload pain point is as old as IDS technologyânot a new problem for machine learning to tackleâand some in the industry have invested in ML-based (semi-) automated remediation. However, there are a few pressures driving more widespread application of ML to simplify response through ML distillation rather than complicate with additional evidence: (1) market pressure to optimize workflows instead of alertsâto scale human response, (2) diminishing returns on reducing time-to-detect compared to time-to-remediate.
What can you do?
Assess remediation workflows of security analysts in your organization: (1) What pieces of evidence related to the incident provide high enough confidence to respond? (2) What evidence determines how to respond? (3) For a typical incident, how many decisions must be made during remediation? (4) How long does remediation take for a typical incident? (5) What is currently being automated reliably? (6) What tasks could still be automated?
Donât force a solution on security analystsâchances are, they are creating custom remediation scripts in powershell or bash. You may already be using a mixed-bag of commercial and open source tools for remediation (e.g., Ansible to task commands to different groups, or open source @davehullâs Kansa).
Assess how existing solutions can help simplify and automate remediation steps. Check out Demisto, or Endgameâs Artemis.
2. Machine learning for attack automation
âInvest in adversarial machine learningâ was listed in our previous two yearly trends because of the tremendous uptick in research activity. In 2018, weâre predicting that one manifestation of this is now ripe for adoption in the mainstream: ML for attack automation. A caveat: although we believe that 2018 will be the year that ML begins to be adopted for automatingâfor example, social engineering phishing attacks or bypassing CAPTCHAâwe donât think itâs necessarily the year weâll see evidence in the wild of sophisticated methods to subvert your machine learning malware detection, or to discover and exploit vulnerabilities in your network. Thatâs still research, and today, there are still easier methods for attackers.
Why now?
Thereâs been significant research activity to demonstrate how, at least theoretically, AI can scale digital attacks in an unprecedented way. Tool sets are making the barrier to entry quite low. And there are economic drivers to do things like bypass CAPTCHA. Incidentally, todayâs security risks and exploits are often more embarrassing than sophisticated, so that even sophisticated adversaries may not require machine learning to be effective, instead relying on unpatched deficiencies in networks that the attacker understands and exploits. So, itâs important to not be an alarmist. Think of ML for attack automation as just an algorithmic wrinkle that adds dynamism and efficiency to automatically discovering or exploiting during an attack.
What can you do?
Protect your users by more than simple image/audio CAPTCHA-like techniques that can be solved trivially by a human. Chances are that if itâs trivially solved by a human, then itâs a target for machine learning automation. There are no easy alternativesâbut, moderate success has been obtained in image recognition showing fragments of a single image (say, a scene on the road), and asking to pick out those pieces that have a desired object (say, a red car).
Calmly prepare for even the unlikely. Ask yourself: how would you discover whether an attack on your network was automated by machine learning or by old-fashioned enumeration in a script? (Might you see exploration-turn-to-exploitation in an ML attack? Would it matter?)
Familiarize yourself with pen testing and red-teaming tools like Caldera, Innuendo, and Atomic Red Team, which can simulate advanced manual attacks, but would also give you a leg-up on automated attacks in years to come.
3. Model compliance
Global compliance laws affect the design, engineering, and operational costs of security data science solutions. The laws themselves provide strict guidelines around data handling, movement (platform constraints), as well as model-building constraints such as explainability and the âright to be forgotten.â Model compliance is not a one-time investment: privacy laws change with the political landscape. For instance, it is not clear how Britain leaving the European Union might affect the privacy laws in the UK. In some cases, privacy laws do not agreeâfor instance, Irish DPA considers IP addresses to be personally identifiable information, which is not the case across the world. More concretely, if you have an anomalous logins detection based on IP addresses, in some parts of the world the detection would not work because IP addresses would be scrubbed/removed. This means that the same machine learning model for detecting these anomalous logins would not work across different geographic regions.
Why now?
Building models that are respectful of compliance laws is important because failure to do so not only brings with it crippling monetary costsâfor instance, failure to adhere to the new European General Data Protection Regulation (GDPR), set to take effect in May 2018, can result in a fine of up to 20 million Euros, or 4% of annual global turnoverâbut also the negative press associated for the business.
What can you do?
As an end consumer, you would need to audit your data and tag it appropriately. Those who are on AWS are lucky, with Amazonâs Macie service. If your data set is small, it is best to bite the bullet and do it by hand.
Many countries prevent cloud providers from merging locality-specific data outside regional boundaries. We recommend tiered modeling: each geographic region is modeled separately, and the results are scrubbed and sent to a global model. Differentially private ensembles are particularly relevant here.
Figure 1. Tiered modeling models each geographic region separately. Source: Ram Shankar Siva Kumar. 4. Rigor and repeatable experiments
The biggest buzz of the NIPS 2017 conference was when Ali Rahimi claimed current ML methods are akin to alchemy (read commentary from @fhuszar on this subject). At the core of Rahimiâs talk was how the machine learning field is progressing on non-rigorous methods that are not widely understood and, in some cases, not repeatable. For instance, researchers showed how the same reinforcement algorithm from two different code bases on the same data set, had vastly different results. Jason Brownleeâs blog breaks down the different ways an ML experiment can produce random results: from randomization introduced by libraries to GPU quirks.
Why now?
We are at a time where there is a deluge of buzzwords in the security worldâartificial intelligence, advanced persistent threats, and machine deception. As a field, we have matured to know there are limitations to every solution; there is no omnipotent solutionâeven if it were to use the latest methods. So, this one is less of a trend and more a call to action.
What can you do?
Whenever you publish your work, at an academic conference or a security con, please release your code and the data set you used for training. The red team is very good at doing this; we defenders need to step up our game.
Eschew publishing your detection results on the KDD 1999 data setâclaiming state-of-the-art results on a data set that was popular during the times of Internet Explorer 5 and Napster is unhygienic. (âMNIST is the new unit test,â suggested Ian Goodfellow, but it doesnât convey a successful result.) Consider using a more realistic data set like Splunkâs Boss of the SOC curated by Ryan Kovar.
We understand that in some cases there are no publicly available benchmarks and there is a constraint to release the data set as isâin that case, consider generating evaluation data in a simulated environment using @subteeâs Red Canary framework.
When you present a method at a conference, highlight the weakness and failures of the methodâgo beyond false positive and false negative rates, and highlight the tradeoffs. Let the audience know what kinds of attacks you will miss and how you compensate for them. If you need inspiration, I will be at the Strata Data Conference in San Jose this March talking about the different security experiments that spectacularly failed and how we fixed them.
Your efforts to bring rigor to the security analytics field are going to benefit us allâthe rising tide does raise all boats.
What wonât happen in 2018
To temper some of the untempered excitement (and sometimes hype) about machine learning in information security, we conclude with a few suggestions for things that we arenât likely to see in 2018.
Reinforcement learning (RL) for offense in the wild
RL has been used to train agents that demonstrate superhuman performance at very narrow tasks, like AlphaGo and Atari. In infosec, it has been demonstrated in research settings to, for example, discover weaknesses of next-gen AV at very modest rates. However, itâs not yet in the âit just worksâ category, and we forecast another one to two years before infosec realizes interesting offensive or defensive automation via RL.
Generative adversarial networks (GANs) in an infosec product
Generally speaking, GANs continue to see a ton of research activity with impressive resultsâthe excitement is totally warranted. Unfortunately, thereâs also been a lack of systematic and objective evaluation metrics in their development. This is a cool hammer that has yet to find its respective killer application in infosec.
Machine learning displacing security jobs
In fact, we think the assimilation causality may go in reverse: because of ever-improving accessibility of machine learning, many more infosec professionals will begin to adopt machine learning in traditional security tasks.
Hype around AI in infosec
It is a fact that, especially in infosec, those talking about âAIâ usually mean âML.â Despite our best efforts, in 2018, the loaded buzzwords about AI in security arenât going away. We still need to educate customers about how to cut through the hype by asking the right questions. And frankly, a consumer shouldnât care if itâs AI, ML, or hand-crafted rules. The real question should be, âdoes it protect me?â
Parting thoughts
The year 2018 is going to bring ML-for-response, as well as the milder forms of attack automation, into the mainstream. As an industry, compliance laws for machine learning will affect a more general shift toward data privacy. The ML community will self-correct toward rigor and repeatability. At the same time, this year we will not see security products infused with RL or GANsâdespite popularity in ongoing research. Your infosec job is here to stay, despite more use of ML. Finally, weâll see this year that ML is mature enough to stand on its own, with no need to be propped up with imaginative buzzwords or hype.
We would love to hear your thoughtsâreach out to us (@ram_ssk and @drhyrum) and join the conversation!
Continue reading 4 trends in security data science for 2018.
from FEED 10 TECHNOLOGY http://ift.tt/2BrjDeB
0 notes
Text
4 trends in security data science for 2018
4 trends in security data science for 2018
A glimpse into what lies ahead for response automation, model compliance, and repeatable experiments.
This is the third consecutive year Iâve tried to read the tea leaves for security analytics. Last yearâs trends post manifested well: from a rise in adversarial machine learning (ML) to the deep learning craze (such that entire conferences are now dedicated to this subject). This year, Hyrum Anderson, technical director of data science from Endgame, joins me in calling out the trends in security data science for 2018. We present a 360-degree view of the security data science landscapeâfrom unicorn startups to established enterprises.
The format remains mostly remains the same: four trends to map to each quarter of the year. For each trend, we provide a rationale about why the time is right to capitalize on the trend, offer practical tips on what you can do now to join the conversation, and include links to papers, GitHub repositories, tools, and tutorials. We also added a new section âWhat wonât happen in 2018â to help readers look beyond the marketing material and stay clear of hype.
1. Machine learning for response (semi-)automation
In 2016, we predicted a shift from detection to intelligent investigation. In 2018, weâre predicting a shift from rich investigative information toward distilled recommended actions, backed by information-rich incident reports. Infosec analysts have long stopped clamoring for âmore alerts!â from security providers. In the coming year, weâll see increased customer appetite for products to recommend actions based on solid evidence. Machine learning has, in large part, proven itself a valuable tool for detecting evidence of threats used to compile an incident report. Security professionals subconsciously train themselves to respond to (or ignore) the evidence of an incident in a certain way. The linchpin to scale in information security rests still on the information security analyst, and many of the knee-jerk responses can be automated. In some cases, the response might be ML-automated, but in many others it will be at least ML-recommended.
Why now?
The information overload pain point is as old as IDS technologyânot a new problem for machine learning to tackleâand some in the industry have invested in ML-based (semi-) automated remediation. However, there are a few pressures driving more widespread application of ML to simplify response through ML distillation rather than complicate with additional evidence: (1) market pressure to optimize workflows instead of alertsâto scale human response, (2) diminishing returns on reducing time-to-detect compared to time-to-remediate.
What can you do?
Assess remediation workflows of security analysts in your organization: (1) What pieces of evidence related to the incident provide high enough confidence to respond? (2) What evidence determines how to respond? (3) For a typical incident, how many decisions must be made during remediation? (4) How long does remediation take for a typical incident? (5) What is currently being automated reliably? (6) What tasks could still be automated?
Donât force a solution on security analystsâchances are, they are creating custom remediation scripts in powershell or bash. You may already be using a mixed-bag of commercial and open source tools for remediation (e.g., Ansible to task commands to different groups, or open source @davehullâs Kansa).
Assess how existing solutions can help simplify and automate remediation steps. Check out Demisto, or Endgameâs Artemis.
2. Machine learning for attack automation
âInvest in adversarial machine learningâ was listed in our previous two yearly trends because of the tremendous uptick in research activity. In 2018, weâre predicting that one manifestation of this is now ripe for adoption in the mainstream: ML for attack automation. A caveat: although we believe that 2018 will be the year that ML begins to be adopted for automatingâfor example, social engineering phishing attacks or bypassing CAPTCHAâwe donât think itâs necessarily the year weâll see evidence in the wild of sophisticated methods to subvert your machine learning malware detection, or to discover and exploit vulnerabilities in your network. Thatâs still research, and today, there are still easier methods for attackers.
Why now?
Thereâs been significant research activity to demonstrate how, at least theoretically, AI can scale digital attacks in an unprecedented way. Tool sets are making the barrier to entry quite low. And there are economic drivers to do things like bypass CAPTCHA. Incidentally, todayâs security risks and exploits are often more embarrassing than sophisticated, so that even sophisticated adversaries may not require machine learning to be effective, instead relying on unpatched deficiencies in networks that the attacker understands and exploits. So, itâs important to not be an alarmist. Think of ML for attack automation as just an algorithmic wrinkle that adds dynamism and efficiency to automatically discovering or exploiting during an attack.
What can you do?
Protect your users by more than simple image/audio CAPTCHA-like techniques that can be solved trivially by a human. Chances are that if itâs trivially solved by a human, then itâs a target for machine learning automation. There are no easy alternativesâbut, moderate success has been obtained in image recognition showing fragments of a single image (say, a scene on the road), and asking to pick out those pieces that have a desired object (say, a red car).
Calmly prepare for even the unlikely. Ask yourself: how would you discover whether an attack on your network was automated by machine learning or by old-fashioned enumeration in a script? (Might you see exploration-turn-to-exploitation in an ML attack? Would it matter?)
Familiarize yourself with pen testing and red-teaming tools like Caldera, Innuendo, and Atomic Red Team, which can simulate advanced manual attacks, but would also give you a leg-up on automated attacks in years to come.
3. Model compliance
Global compliance laws affect the design, engineering, and operational costs of security data science solutions. The laws themselves provide strict guidelines around data handling, movement (platform constraints), as well as model-building constraints such as explainability and the âright to be forgotten.â Model compliance is not a one-time investment: privacy laws change with the political landscape. For instance, it is not clear how Britain leaving the European Union might affect the privacy laws in the UK. In some cases, privacy laws do not agreeâfor instance, Irish DPA considers IP addresses to be personally identifiable information, which is not the case across the world. More concretely, if you have an anomalous logins detection based on IP addresses, in some parts of the world the detection would not work because IP addresses would be scrubbed/removed. This means that the same machine learning model for detecting these anomalous logins would not work across different geographic regions.
Why now?
Building models that are respectful of compliance laws is important because failure to do so not only brings with it crippling monetary costsâfor instance, failure to adhere to the new European General Data Protection Regulation (GDPR), set to take effect in May 2018, can result in a fine of up to 20 million Euros, or 4% of annual global turnoverâbut also the negative press associated for the business.
What can you do?
As an end consumer, you would need to audit your data and tag it appropriately. Those who are on AWS are lucky, with Amazonâs Macie service. If your data set is small, it is best to bite the bullet and do it by hand.
Many countries prevent cloud providers from merging locality-specific data outside regional boundaries. We recommend tiered modeling: each geographic region is modeled separately, and the results are scrubbed and sent to a global model. Differentially private ensembles are particularly relevant here.
Figure 1. Tiered modeling models each geographic region separately. Source: Ram Shankar Siva Kumar. 4. Rigor and repeatable experiments
The biggest buzz of the NIPS 2017 conference was when Ali Rahimi claimed current ML methods are akin to alchemy (read commentary from @fhuszar on this subject). At the core of Rahimiâs talk was how the machine learning field is progressing on non-rigorous methods that are not widely understood and, in some cases, not repeatable. For instance, researchers showed how the same reinforcement algorithm from two different code bases on the same data set, had vastly different results. Jason Brownleeâs blog breaks down the different ways an ML experiment can produce random results: from randomization introduced by libraries to GPU quirks.
Why now?
We are at a time where there is a deluge of buzzwords in the security worldâartificial intelligence, advanced persistent threats, and machine deception. As a field, we have matured to know there are limitations to every solution; there is no omnipotent solutionâeven if it were to use the latest methods. So, this one is less of a trend and more a call to action.
What can you do?
Whenever you publish your work, at an academic conference or a security con, please release your code and the data set you used for training. The red team is very good at doing this; we defenders need to step up our game.
Eschew publishing your detection results on the KDD 1999 data setâclaiming state-of-the-art results on a data set that was popular during the times of Internet Explorer 5 and Napster is unhygienic. (âMNIST is the new unit test,â suggested Ian Goodfellow, but it doesnât convey a successful result.) Consider using a more realistic data set like Splunkâs Boss of the SOC curated by Ryan Kovar.
We understand that in some cases there are no publicly available benchmarks and there is a constraint to release the data set as isâin that case, consider generating evaluation data in a simulated environment using @subteeâs Red Canary framework.
When you present a method at a conference, highlight the weakness and failures of the methodâgo beyond false positive and false negative rates, and highlight the tradeoffs. Let the audience know what kinds of attacks you will miss and how you compensate for them. If you need inspiration, I will be at the Strata Data Conference in San Jose this March talking about the different security experiments that spectacularly failed and how we fixed them.
Your efforts to bring rigor to the security analytics field are going to benefit us allâthe rising tide does raise all boats.
What wonât happen in 2018
To temper some of the untempered excitement (and sometimes hype) about machine learning in information security, we conclude with a few suggestions for things that we arenât likely to see in 2018.
Reinforcement learning (RL) for offense in the wild
RL has been used to train agents that demonstrate superhuman performance at very narrow tasks, like AlphaGo and Atari. In infosec, it has been demonstrated in research settings to, for example, discover weaknesses of next-gen AV at very modest rates. However, itâs not yet in the âit just worksâ category, and we forecast another one to two years before infosec realizes interesting offensive or defensive automation via RL.
Generative adversarial networks (GANs) in an infosec product
Generally speaking, GANs continue to see a ton of research activity with impressive resultsâthe excitement is totally warranted. Unfortunately, thereâs also been a lack of systematic and objective evaluation metrics in their development. This is a cool hammer that has yet to find its respective killer application in infosec.
Machine learning displacing security jobs
In fact, we think the assimilation causality may go in reverse: because of ever-improving accessibility of machine learning, many more infosec professionals will begin to adopt machine learning in traditional security tasks.
Hype around AI in infosec
It is a fact that, especially in infosec, those talking about âAIâ usually mean âML.â Despite our best efforts, in 2018, the loaded buzzwords about AI in security arenât going away. We still need to educate customers about how to cut through the hype by asking the right questions. And frankly, a consumer shouldnât care if itâs AI, ML, or hand-crafted rules. The real question should be, âdoes it protect me?â
Parting thoughts
The year 2018 is going to bring ML-for-response, as well as the milder forms of attack automation, into the mainstream. As an industry, compliance laws for machine learning will affect a more general shift toward data privacy. The ML community will self-correct toward rigor and repeatability. At the same time, this year we will not see security products infused with RL or GANsâdespite popularity in ongoing research. Your infosec job is here to stay, despite more use of ML. Finally, weâll see this year that ML is mature enough to stand on its own, with no need to be propped up with imaginative buzzwords or hype.
We would love to hear your thoughtsâreach out to us (@ram_ssk and @drhyrum) and join the conversation!
Continue reading 4 trends in security data science for 2018.
http://ift.tt/2BrjDeB
0 notes
Text
4 trends in security data science for 2018
4 trends in security data science for 2018
A glimpse into what lies ahead for response automation, model compliance, and repeatable experiments.
This is the third consecutive year Iâve tried to read the tea leaves for security analytics. Last yearâs trends post manifested well: from a rise in adversarial machine learning (ML) to the deep learning craze (such that entire conferences are now dedicated to this subject). This year, Hyrum Anderson, technical director of data science from Endgame, joins me in calling out the trends in security data science for 2018. We present a 360-degree view of the security data science landscapeâfrom unicorn startups to established enterprises.
The format remains mostly remains the same: four trends to map to each quarter of the year. For each trend, we provide a rationale about why the time is right to capitalize on the trend, offer practical tips on what you can do now to join the conversation, and include links to papers, GitHub repositories, tools, and tutorials. We also added a new section âWhat wonât happen in 2018â to help readers look beyond the marketing material and stay clear of hype.
1. Machine learning for response (semi-)automation
In 2016, we predicted a shift from detection to intelligent investigation. In 2018, weâre predicting a shift from rich investigative information toward distilled recommended actions, backed by information-rich incident reports. Infosec analysts have long stopped clamoring for âmore alerts!â from security providers. In the coming year, weâll see increased customer appetite for products to recommend actions based on solid evidence. Machine learning has, in large part, proven itself a valuable tool for detecting evidence of threats used to compile an incident report. Security professionals subconsciously train themselves to respond to (or ignore) the evidence of an incident in a certain way. The linchpin to scale in information security rests still on the information security analyst, and many of the knee-jerk responses can be automated. In some cases, the response might be ML-automated, but in many others it will be at least ML-recommended.
Why now?
The information overload pain point is as old as IDS technologyânot a new problem for machine learning to tackleâand some in the industry have invested in ML-based (semi-) automated remediation. However, there are a few pressures driving more widespread application of ML to simplify response through ML distillation rather than complicate with additional evidence: (1) market pressure to optimize workflows instead of alertsâto scale human response, (2) diminishing returns on reducing time-to-detect compared to time-to-remediate.
What can you do?
Assess remediation workflows of security analysts in your organization: (1) What pieces of evidence related to the incident provide high enough confidence to respond? (2) What evidence determines how to respond? (3) For a typical incident, how many decisions must be made during remediation? (4) How long does remediation take for a typical incident? (5) What is currently being automated reliably? (6) What tasks could still be automated?
Donât force a solution on security analystsâchances are, they are creating custom remediation scripts in powershell or bash. You may already be using a mixed-bag of commercial and open source tools for remediation (e.g., Ansible to task commands to different groups, or open source @davehullâs Kansa).
Assess how existing solutions can help simplify and automate remediation steps. Check out Demisto, or Endgameâs Artemis.
2. Machine learning for attack automation
âInvest in adversarial machine learningâ was listed in our previous two yearly trends because of the tremendous uptick in research activity. In 2018, weâre predicting that one manifestation of this is now ripe for adoption in the mainstream: ML for attack automation. A caveat: although we believe that 2018 will be the year that ML begins to be adopted for automatingâfor example, social engineering phishing attacks or bypassing CAPTCHAâwe donât think itâs necessarily the year weâll see evidence in the wild of sophisticated methods to subvert your machine learning malware detection, or to discover and exploit vulnerabilities in your network. Thatâs still research, and today, there are still easier methods for attackers.
Why now?
Thereâs been significant research activity to demonstrate how, at least theoretically, AI can scale digital attacks in an unprecedented way. Tool sets are making the barrier to entry quite low. And there are economic drivers to do things like bypass CAPTCHA. Incidentally, todayâs security risks and exploits are often more embarrassing than sophisticated, so that even sophisticated adversaries may not require machine learning to be effective, instead relying on unpatched deficiencies in networks that the attacker understands and exploits. So, itâs important to not be an alarmist. Think of ML for attack automation as just an algorithmic wrinkle that adds dynamism and efficiency to automatically discovering or exploiting during an attack.
What can you do?
Protect your users by more than simple image/audio CAPTCHA-like techniques that can be solved trivially by a human. Chances are that if itâs trivially solved by a human, then itâs a target for machine learning automation. There are no easy alternativesâbut, moderate success has been obtained in image recognition showing fragments of a single image (say, a scene on the road), and asking to pick out those pieces that have a desired object (say, a red car).
Calmly prepare for even the unlikely. Ask yourself: how would you discover whether an attack on your network was automated by machine learning or by old-fashioned enumeration in a script? (Might you see exploration-turn-to-exploitation in an ML attack? Would it matter?)
Familiarize yourself with pen testing and red-teaming tools like Caldera, Innuendo, and Atomic Red Team, which can simulate advanced manual attacks, but would also give you a leg-up on automated attacks in years to come.
3. Model compliance
Global compliance laws affect the design, engineering, and operational costs of security data science solutions. The laws themselves provide strict guidelines around data handling, movement (platform constraints), as well as model-building constraints such as explainability and the âright to be forgotten.â Model compliance is not a one-time investment: privacy laws change with the political landscape. For instance, it is not clear how Britain leaving the European Union might affect the privacy laws in the UK. In some cases, privacy laws do not agreeâfor instance, Irish DPA considers IP addresses to be personally identifiable information, which is not the case across the world. More concretely, if you have an anomalous logins detection based on IP addresses, in some parts of the world the detection would not work because IP addresses would be scrubbed/removed. This means that the same machine learning model for detecting these anomalous logins would not work across different geographic regions.
Why now?
Building models that are respectful of compliance laws is important because failure to do so not only brings with it crippling monetary costsâfor instance, failure to adhere to the new European General Data Protection Regulation (GDPR), set to take effect in May 2018, can result in a fine of up to 20 million Euros, or 4% of annual global turnoverâbut also the negative press associated for the business.
What can you do?
As an end consumer, you would need to audit your data and tag it appropriately. Those who are on AWS are lucky, with Amazonâs Macie service. If your data set is small, it is best to bite the bullet and do it by hand.
Many countries prevent cloud providers from merging locality-specific data outside regional boundaries. We recommend tiered modeling: each geographic region is modeled separately, and the results are scrubbed and sent to a global model. Differentially private ensembles are particularly relevant here.
Figure 1. Tiered modeling models each geographic region separately. Source: Ram Shankar Siva Kumar. 4. Rigor and repeatable experiments
The biggest buzz of the NIPS 2017 conference was when Ali Rahimi claimed current ML methods are akin to alchemy (read commentary from @fhuszar on this subject). At the core of Rahimiâs talk was how the machine learning field is progressing on non-rigorous methods that are not widely understood and, in some cases, not repeatable. For instance, researchers showed how the same reinforcement algorithm from two different code bases on the same data set, had vastly different results. Jason Brownleeâs blog breaks down the different ways an ML experiment can produce random results: from randomization introduced by libraries to GPU quirks.
Why now?
We are at a time where there is a deluge of buzzwords in the security worldâartificial intelligence, advanced persistent threats, and machine deception. As a field, we have matured to know there are limitations to every solution; there is no omnipotent solutionâeven if it were to use the latest methods. So, this one is less of a trend and more a call to action.
What can you do?
Whenever you publish your work, at an academic conference or a security con, please release your code and the data set you used for training. The red team is very good at doing this; we defenders need to step up our game.
Eschew publishing your detection results on the KDD 1999 data setâclaiming state-of-the-art results on a data set that was popular during the times of Internet Explorer 5 and Napster is unhygienic. (âMNIST is the new unit test,â suggested Ian Goodfellow, but it doesnât convey a successful result.) Consider using a more realistic data set like Splunkâs Boss of the SOC curated by Ryan Kovar.
We understand that in some cases there are no publicly available benchmarks and there is a constraint to release the data set as isâin that case, consider generating evaluation data in a simulated environment using @subteeâs Red Canary framework.
When you present a method at a conference, highlight the weakness and failures of the methodâgo beyond false positive and false negative rates, and highlight the tradeoffs. Let the audience know what kinds of attacks you will miss and how you compensate for them. If you need inspiration, I will be at the Strata Data Conference in San Jose this March talking about the different security experiments that spectacularly failed and how we fixed them.
Your efforts to bring rigor to the security analytics field are going to benefit us allâthe rising tide does raise all boats.
What wonât happen in 2018
To temper some of the untempered excitement (and sometimes hype) about machine learning in information security, we conclude with a few suggestions for things that we arenât likely to see in 2018.
Reinforcement learning (RL) for offense in the wild
RL has been used to train agents that demonstrate superhuman performance at very narrow tasks, like AlphaGo and Atari. In infosec, it has been demonstrated in research settings to, for example, discover weaknesses of next-gen AV at very modest rates. However, itâs not yet in the âit just worksâ category, and we forecast another one to two years before infosec realizes interesting offensive or defensive automation via RL.
Generative adversarial networks (GANs) in an infosec product
Generally speaking, GANs continue to see a ton of research activity with impressive resultsâthe excitement is totally warranted. Unfortunately, thereâs also been a lack of systematic and objective evaluation metrics in their development. This is a cool hammer that has yet to find its respective killer application in infosec.
Machine learning displacing security jobs
In fact, we think the assimilation causality may go in reverse: because of ever-improving accessibility of machine learning, many more infosec professionals will begin to adopt machine learning in traditional security tasks.
Hype around AI in infosec
It is a fact that, especially in infosec, those talking about âAIâ usually mean âML.â Despite our best efforts, in 2018, the loaded buzzwords about AI in security arenât going away. We still need to educate customers about how to cut through the hype by asking the right questions. And frankly, a consumer shouldnât care if itâs AI, ML, or hand-crafted rules. The real question should be, âdoes it protect me?â
Parting thoughts
The year 2018 is going to bring ML-for-response, as well as the milder forms of attack automation, into the mainstream. As an industry, compliance laws for machine learning will affect a more general shift toward data privacy. The ML community will self-correct toward rigor and repeatability. At the same time, this year we will not see security products infused with RL or GANsâdespite popularity in ongoing research. Your infosec job is here to stay, despite more use of ML. Finally, weâll see this year that ML is mature enough to stand on its own, with no need to be propped up with imaginative buzzwords or hype.
We would love to hear your thoughtsâreach out to us (@ram_ssk and @drhyrum) and join the conversation!
Continue reading 4 trends in security data science for 2018.
http://ift.tt/2BrjDeB
0 notes