#gedit is an engineer
Explore tagged Tumblr posts
Text
Breaking my tumblr break for a moment because I need someone else to appreciate that my Guild Wars 2 asuras are named "Vimvi" and "Gedit."
#guild wars 2#asura#explaining the joke for non programmers they're named after text editors#vimvi is a mesmer#gedit is an engineer#neither are my main but I like them enough they still get to hang around#also yeah along with a tumblr break I've been taking a break from ffxiv so I don't burn out before dawntrail#and I've picked gw2 back up to scratch the mmo itch#I've been playing on and off for seven years and this weekend finally just finished the base game#the benefit of taking that long I guess is now I get to do living world season 1 in the proper order
6 notes
·
View notes
Text
Install gedit linux
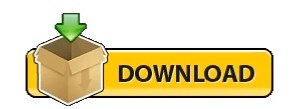
#INSTALL GEDIT LINUX HOW TO#
#INSTALL GEDIT LINUX UPDATE#
#INSTALL GEDIT LINUX SOFTWARE#
This will provide you with an overview of all the commands that this editor provides as well as its features. This is done by running searches from the terminal such as Gedit with the command 'help'. O Search Online: Google and other common search engines offer the ability to search online using the desktop version of Gnome Editor. You can also integrate it with the programming languages that are commonly used for application development and editing. It integrates seamlessly with the default text editor to set up in gnome and provides basic functionality for text processing including: spell checking, searching and replace. O Advanced integration with the Gnome Default Text Editor: Text editing in the gnome desktop environment can be as simple or as complex as you want it to be thanks to the extensive plugins available for this free software. The latest release of this text editor is a 6.3 which comes with lots of new features such as:
#INSTALL GEDIT LINUX SOFTWARE#
This free software can be used as a replacement for Microsoft Word or Open Office for all your office needs. It offers basic editing capabilities as well as advanced features for creating, editing and combining documents and files.
#INSTALL GEDIT LINUX HOW TO#
In this guide, we learned how to install gEdit on Ubuntu and extend it using Plugins.A free, open source text editor designed for the Linux desktop is gnome-text-editor. $ sudo apt remove gedit-pluginsĪfter the successful removal of all the packages, the software will be removed from your system. Run the following commands to get it done. We can uninstall it using the standard apt commands. We used Ubuntu repositories to install the gEdit text editor. These plugins will make the editor robust for programming in any language. Use the following command to install pre-developed plugins for your editor. It can be used for programming thus we can install plugins. It has a clean and sleek user interface.Īs we discussed before that the gEdit can be extended to the best of availability. Once located you are ready to launch the application, it will look something like below. If you want to see gEdit presence in your system via dashboard then press the super key and search for the gedit as shown below.availabilit圓. You will see the latest version available on your system. Go to the terminal and write the following command to see if it is installed. We can confirm the installation using both the command line and graphical user interface. When the packages are installed, the application will be available on your system. $ sudo apt updateĮnter your password and do not make a mistake while entering it. Check the following commands and they will help you to install the software. This helps us to install an up to date software.
#INSTALL GEDIT LINUX UPDATE#
Install gEdit on Ubuntu 20.04 LTSįirst, we update the repositories available on our system. The gEdit text editor does not require any prerequisites. The text editor has an enormous number of functions and can be used for programming purposes as well. I am using Ubuntu 20.04 Mate edition, and it does not come preinstalled. It is developed by Gnome and can be installed from standard Ubuntu repositories. There are several text editors available for Linux and one of them is gEdit.
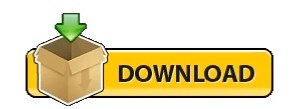
0 notes
Text
Linux online training || Linux online course - iteducationalexperts.com
Linux Online Training || Linux Online CourseABOUT LINUX
This article is mainly written for the people who are not familiar with the Linux operating system. I hope this article will help to understand the history behind the UNIX/LINUX Operating system. Before getting into the topic, we will see some important points of the Linux system:
Most of the computers are using Linux as an operating system as it is a Command line interface.
LINUX is very popular and stable because computers running LINUX almost never crash.
LINUX can smoothly manage a large amount of data as it is very efficient.
LINUX administration is a server-based technology. Linux was developed by Linux Torvalds in 1991. It is an open source operating system or kernel similar to Windows XP, Windows 7 and Windows 8. Linux operating system is used everywhere from smartphones to cars, supercomputers, laptops, desktop and home appliances. We can view and edit the code of the Linux as it is free unlike UNIX and available to the public.
What are the benefits of Linux Operating system?
As Linux is an open source operating system, it can modify by anyone who has a little bit knowledge of programming.
After installing the Linux kernel, there is no use of antivirus because it is a highly secure system. Also, there is a global development community tries many ways to improve its security and unfortunately, it is becoming more e secure and robust for each upgrade.
Due to its reliability and stability, many companies like Google, Facebook, Amazon are using Linux as their servers.
Who are eligible for Linux training course?
There are no requirements for learning Linux course. Anyone who has a passion to work as an administrator or web developer can learn this course. Also, Graduates from Engineering, BSc, BCA, and MCA can go through it. This is apart as necessary to imply learners to possess a high intensity in identifying and turn they career by themselves more.
Any Graduates
Software Engineers and administrators.
Linux developers and Linux administrators.
IT professionals
Certification:
ITeducationalexperts course completion certificate is awarded on the completion of the real-time project. We provide practical examples and assignments in a training course with real time experts. We make you best in your Linux professional career with IT organizations in Linux and UNIX tutorial and commands. At the time of preparation, you will like to allow in specified Linux trades which are required for creating a tremendous lead in Linux.
Training Summary
Linux online training with ITEducationalexperts is planned for beginners with step by step process in all required to make best in their Linux professional career with IT organizations by Linux and UNIX tutorial and commands. These plans are solidly for IT traditional in a perfect way on Linux. At the time of preparation, you will like to allow in specified Linux trades which are required for creating a tremendous lead in Linux
History of Linux operating system:
The UNIX story starts at AT & T Bell laboratory in 1969. Ken Thompson and Dennis Ritchie ported a game, known as “Space travel” in Multics project. Thompson wrote a simple file system for porting a game. In 1973, The UNIX was rewritten in C by Thompson and Ritchie. In 1979, portable version of UNIX (Version 7) was released for general use.
In 1991, Linux Torvalds who is a student of Helsinki University developed a UNIX system called Linux, to run on the Intel microprocessor. The Linux distributions, developed from 1993 are based on Torvalds kernel. Different versions of Linux distributions include Red Hat, Slackware, Caldera, Debian, Mandrake and so on.
The Linux can be downloaded at free of charge. Today, Linux has become the fastest growing part of the UNIX operating system.
OPERATING SYSTEM OF A LINUX:
As we all know that the operating system is an interface between a user and computer hardware. The operating system is a collection of software which manages computer hardware and provides services for the programs.
Linux is a layered operating system. The services provided to the operating system in Linux are the innermost layer called hardware.
The “Kernel” which is the operating system in Linux interacts directly with the hardware and provides services to all the user programs. For the user programs, it is enough to know how to interact with the kernel and no need to know anything about the hardware.
Multi-user Multi-tasking system:
Linux is a multi-user Multitasking operating system, which means many users can log in with each running multi programs at a time. The kernel will keep each process and user separately and manages the hardware of the system along with CPU, I/O devices.
As we know that the source code of the Linux is freely available, means anyone can add features and corrects the deficiencies of a Linux source code. Therefore, Linux is known as a free open-source operating system.
The Architecture of Linux Operating system:
The main important components of the Linux operating system are:
Kernel:
The kernel is the core part of the Linux operating system, as it manages the hardware devices of a PC and keeps track of the disks, printers, tapes and many other devices. The latest versions of the kernel can be downloaded from http://www.kernel.org.
Shell:
The Shell is an interface between user and kernel, known as Command Interpreter. Even though it is only a utility program, and is not a proper part of the system, it is a part which user sees. The shell translates our requests into actions by listening to the terminal on the part of a kernel.
The shell is divided into two forms – Command line shells and Graphical shells. The command line shell provides Command line interfaces and a Graphical line shell provides Graphical user interfaces. The Graphical shell performs operations slower than the Command line shells.
Hardware:
The hardware layer of the Linux operating system includes peripheral devices like RAM, CPU and so on.
It is easy to remember the architecture of the Linux system as a series of concentric circles. The innermost layer is the hardware and kernel including with the next layer as a shell and the outermost layer is the Application programs and utilities.
Features of the Linux Operating system:
Portable and Open source:
The Linux kernel supports all types of hardware installations and can work with different types of hardware. The source code of the Linux is also available free.
Multi-user multiprogramming:
As already said, the Linux operating system is a multi-user and multiprogramming system.
Security:
The Linux operating system provides security systems for the users by using authentication features such as password protection, encryption of data, controlling access to particular files and so on.
Hierarchical File system:
The Linux operating system provides a standard structure of the file where system or user files are arranged.
Advantages of Linux operating system:
Low cost: There is no need to spend more time and money for the license of Linux as it is available for free and software has GNU (General Public License).
Stability: There is no need of rebooting the system periodically to maintain the performance.
Performance: Linux can easily handle the bulk number of users simultaneously.
Flexibility: It is easy to save the disk space by installing only selected and wanted components.
Compatibility: Linux can run all UNIX software packages.
Applications of Linux operating system:
Due to its reliability and stability, many companies like Google, Facebook, Amazon and so on are using Linux as their servers. Some of the major application programs that use Linux are:
Abiword
Firefox
Apache
Gnumeric
GQview
Gedit
PHP
Python
My SQL
Open office
Rosegarden
Some of the electronic devices which are using Linux are:
Dell Inspiron Mini 9 and 12
HP Mini 1000
Google Android
Sony Reader
Lenovo Ipad
TiVo Digital video recorder.
#Linuxonlinetraining#Linuxonlinecourse#Linuxonlinetraininginkurnool#Linuxonlinecourseinkurnool#Linuxcertification#Linux#Linuxoperatingsystem#Linuxadministration#Bestlinuxonlinetraininginstituteinkurnool#Linuxonlinetraininginhyderabad#Linuxonlinecourseinhyderabad
0 notes
Text
Help! I can’t reproduce a machine learning project!
Have you ever sat down with the code and data for an existing machine learning project, trained the same model, checked your results… and found that they were different from the original results?
Not being able to reproduce someone else’s results is super frustrating. Not being able to reproduce your own results is frustrating and embarrassing. And tracking down the exact reason that you aren’t able to reproduce results can take ages; it took me a solid week to reproduce this NLP paper, even with the original authors’ exact code and data.
But there's good news: Reproducibility breaks down in three main places: the code, the data and the environment. I’ve put together this guide to help you narrow down where your reproducibility problems are, so you can focus on fixing them. Let’s go through the three potential offenders one by one, talk about what kind of problems arise and then see how to fix them.
Non-deterministic code
I’ve called this section “non-deterministic code” rather than “differences in code” because in a lot of machine learning or statistical applications you can end up with completely different results from the same code. This is because many machine learning and statistical algorithms are non-deterministic: randomness is an essential part of how they work.
If you come from a more traditional computer science or software engineering background, this can be pretty surprising: imagine if a sorting algorithm intentionally returned inputs in a slightly different order every time you ran it! In machine learning and statistical computing, however, randomness shows up in many places, including:
Bagging, where you train many small models on different randomly-sampled subsets of your dataset; and boosting, where only the first data subset is completely random and the rest are dependent on it
Initializing weights in a neural network, where the initial weights are sampled from a specific distribution (generally using the method proposed by He et al, 2015 for networks using ReLU activation)
Splitting data into testing, training and validation subsets
Any methods that rely on sampling, like Markov chain Monte Carlo-based methods used for Bayesian inference or Gaussian mixture models
Pretty much any method that talks about “random”, “stochastic”, “sample”, “normal” or "distribution" somewhere in the documentation is likely to have some random element in it
Randomness is your friend when it comes to things like escaping local minima, but it can throw a wrench in the works when you’re trying to reproduce those same results later. In order to make machine learning results reproducible, you need to make sure that the random elements are the same every time you run your code. In other words, you need to make sure you “randomly” generate the same set of random numbers. You can do this by making sure to set every random seed that your code relies on.
Random seed: The number used by a pseudorandom generator to determine the order in which numbers are generated. If the same generator is given the same seed, it will generate the same sequence every time it restarts.
Unfortunately, if you’re working from a project where the random seed was never set in the first place, you probably won’t be able to get those same results again. In that case, your best bet is to retrain a new model that does have a seed set. Here’s a quick primer on how to do that:
In R: Most packages depend on the global random seed, which you can set using `set.seed()`. The exceptions are packages that are actually wrappers around software written in other languages, like XGBoost or some of the packages that rely heavily on rcpp.
In Python: You can set the global random seed in Python using `seed()` from the `random` module. Unfortunately, most packages in the Python data science ecosystem tend to have their own internal random seed. My best piece of advice is to quickly search the documentation for each package you’re using and see if it has a function for setting the random seed. (I’ve compiled a list of the methods for some of the most common packages in the “Controlling randomness” section of this notebook.)
Differences in Data
Another thing that can potentially break reproducibility is differences in the data. While this happens less often, it can be especially difficult to pin down. (This is one of the reasons Kaggle datasets have versioning and why we’ve recently added notes on what’s changed between versions. This dataset is a good example: scroll down to the “History” section.)
You might not be lucky enough to be working from a versioned datasets, however. If you have access to the original data files, there are some ways you can check to make sure that you’re working with the same data the original project used:
You can use cmp in Bash to make sure that all the bytes two files are exactly the same.
You can hash the files and then compare the hashes. You can do this with the hashlib library in Python or either UNF package (for tabular data) or the md5sum() function in R. (Do note that there’s a small chance that two different files might create the same hash.)
Another thing that can be helpful is knowing what can introduce differences in your data files. By far the biggest culprit here is opening data in word processing or spreadsheet software. I’ve personally been bitten in the butt by this one more than once. A lot of the nice helpful changes made to improve the experience of working with data files for humans can be just enough to end up breaking reproducibility. Here’s are two of the biggest sneaky problem areas.
Automatic date formatting: This is actually a huge problem for scientific researchers. One study found that gene names have been automatically converted to dates in one-fifth of published genomics papers. In addition to changing non-dates into dates like that, the format of your dates will sometimes be edited to be more in line with your computer locale, like 6/4/2018 being changed to 4/6/2018.
Character encodings: This is an especially sneaky one because a lot of text editors will open files with different character encodings with no problems… but then save them using whatever your system default character encoding is. That means that your text might not look any different in the editor, but all the underlying bytes have been completely changed. Of course if you always use UTF-8 this isn’t generally a problem, but that’s not always an option.
Because of these problems, I strongly recommend that you don’t check or edit your data files in word processors or spreadsheet software. Or, if you do, do it with a second copy of your data that you can discard later. I tend to use a text editor to check out datasets instead. (I like Gedit or Notepad++ but I know better than to wade into an argument about which text editor is better than the other. 😉 If you’re comfortable working in the command line, you can also check your data there.
Differences in environments
So you’ve triple-double-checked your code and data, and you’re 100% sure that they aren’t accounting for differences between your runs. What’s left? The answer is the computational environment. This includes everything needed to run the code, including things like what packages and package versions were used to run the code and, if you reference them, file directory structures.
Getting a lot of “File not found” errors? They’re probably showing up because you’re trying to run code that references a specific directory structure in a directory with a different structure. Problems related to the file directory structures are pretty easy to fix: just make sure you’re using relative rather than absolute paths and configure your . (If you’re unsure what that means, this notebook goes into more details.) You may have to go back and redo some things by hand, but it’s a pretty easy fix once you know what you’re looking for.
It’s much harder to fix problems that show up because of dependency mismatches. If you’re trying to reproduce your own work, hopefully you still have access to the environment you originally ran your code in. If so, check out the “Computing environment” section of this notebook to learn how to get you can quickly get information on what packages and their versions you used to run code. You can then use that list to make sure you’re using the same packages versions in your current environment.
Pro tip: Make sure you check the language version too! While major versions will definitely break reproducibility (looking at you, Python 2 vs. Python 3), even subversion updates can introduce problems. In particular, differences in the subversion can make it difficult or impossible to load serialized data formats, like pickles in Python or .RData files in R.
The amount of information you have about the environment used to run the code will determine how difficult is to reproduce. You might have...
Information on what was in the environment using something like a requirements or init file. This takes the most work to reproduce, since you need to handle getting the environment set up yourself.
A complete environment using a container or virtual machine. These bundle together all the necessary information, and you just need to get it set up and run it.
A complete hosted runnable environment (like, say, a Kaggle Kernel ;). These are the easiest to use; you just need to point your browser at the address and run the code.
(This taxonomy is discussed in depth in this paper, if you’re curious. )
But what if you don’t already have access to any information about the original environment? If it’s your own project and you don’t have access to it anymore because you dropped it into a lake or something, you may be out of luck. If you’re trying to reproduce someone else’s work, through, the best advice I can give you is to reach out to the person whose work you’re trying to reproduce. I’ve actually had a fair amount of success reaching out to researchers on this front, especially if I’m very polite and specific about what I’m asking.
_____
In the majority of cases, you should be able to track down problems in reproducibility to one of these three places: the code, data or environment. It can take a while to laser in on the exact problems with your project, but having a rough guide should help you narrow down your search.
That said, there are a small number of cases where it’s literally impossible to reproduce modeling results. Perhaps the best example is deep-learning projects that rely on the cuDNN library, which is part of the NVIDIA Deep Learning SDK. Some key methods used for CNNs and bi-directional LSTMs are currently non-deterministic. (But check the documentation for up-to-date information). My advice is to consider not using CUDA if reproducibility is a priority. The tradeoff here is that not using CUDA is that your models might take much longer to train, so if you’re prioritizing speed instead this will be an important consideration for you.
Reproducibility is one of those areas where the whole “an ounce of prevention is worth a pound of cure” idea is very applicable. The easiest way to avoid running into problems with reproducibility is for everyone to make sure their work is reproductible from the get-go. If this is something you’re willing to invest some time in, I’ve written a notebook that walks through best practices for reproducible research here to get you started.
No Free Hunch published first on No Free Hunch
0 notes