#and then i found a channel that used various sources and analysed the reliability of each source and i went đ TRAINED HISTORIAN
Explore tagged Tumblr posts
Text
this might be very niche but i love when you start watching a new youtube channel and you can immediately tell that the person is very smart and know what they're talking about and can formulate an argument!! there are so many dumb people on youtube pretending to be smart and god does it really show in contrast to people who are actually smart
#started watching this new channel that (from their vid titles) mostly react to tiktoks#and i was like hmmm. do i really need to see someone reacting to tiktoks. is this going to be really dumb.#and then in the first five minutes she's talking about veblen's theory of conspicuous consumerism and i was like PHEW#btw i don't just mean people who reference academic theories like that bc dumb people definitely do that too#but 1) if you understand these things you can tell when someone else actually understands them#and 2) it's about the way they formulate an argument and frame their comments that really shows it!!#ie i also enjoy true crime (ik i'm sorry) and there are so many channels that find an account of a case and just basically recount it#and then i found a channel that used various sources and analysed the reliability of each source and i went đ TRAINED HISTORIAN#and i was right!#idk there are just so many shitty video essays out there it's really refreshing when u find a channel that is actually smart!!#đ§
4 notes
·
View notes
Text
Creating Captivating Content With The Royal Botanic Gardens Sydneyâs Digital Marketing And Media Coordinator, Victoria Ngu
Creating Captivating Content With The Royal Botanic Gardens Sydneyâs Digital Marketing And Media Coordinator, Victoria Ngu
Dream Job
Sasha Gattermayr
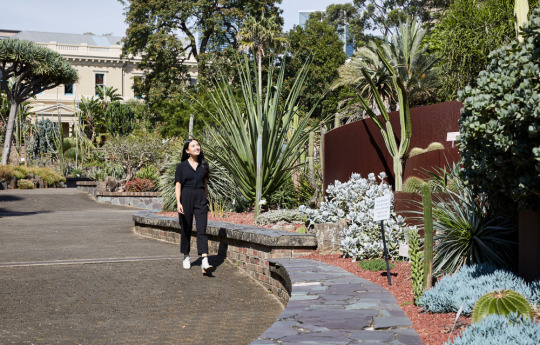
This has got to be one of the most picturesque offices in the world! Photo â Alisha Gore for The Design Files.
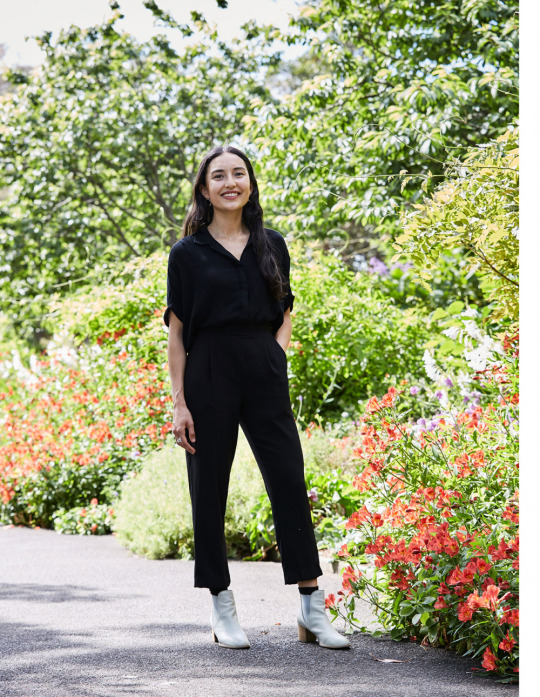
28-year-old Victoria Ngu, Digital Marketing and Media Coordinator at the Royal Botanic Gardens, Sydney. Photo â Alisha Gore for The Design Files.
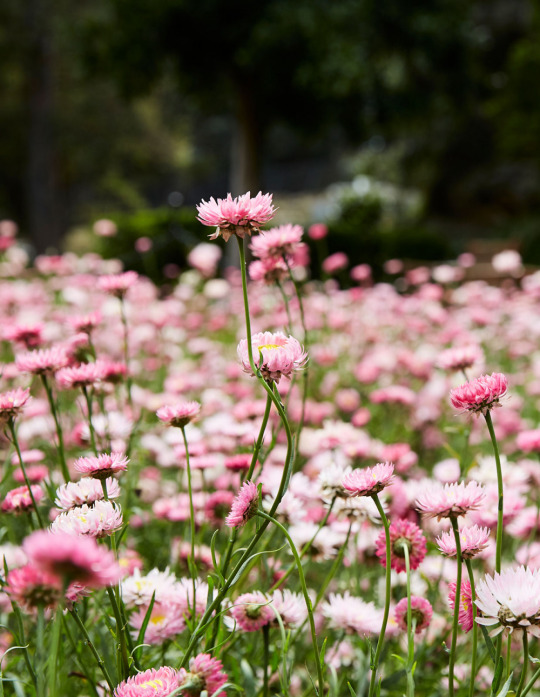
The Gardens span scientific, educational and cultural fields as well as the obvious â horticultural! Photo â Alisha Gore for The Design Files.

The Sydney skyline can be seen peeping above the gardens, which were established in 1816. Photo â Alisha Gore for The Design Files.

Victoria manages a team of content producers across the Gardensâ team, but she always makes a point to eat lunch outside! Photo â Alisha Gore for The Design Files.
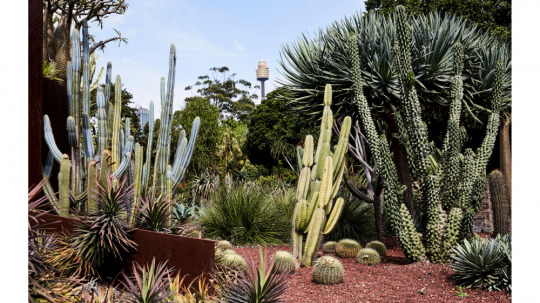
The succulents garden is her location of choice for taking her lunch break. Photo â Alisha Gore for The Design Files.
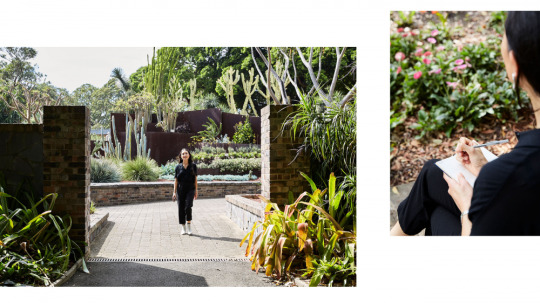
Imagine having this kind of botanical energy at work every day? Photo â Alisha Gore for The Design Files.
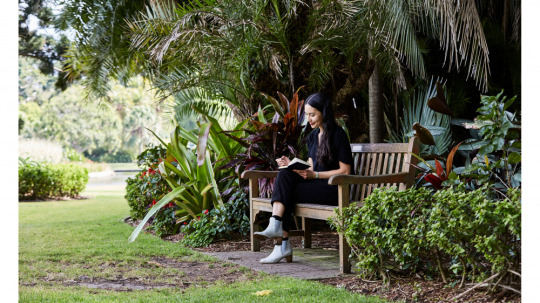
âI love learning plant names and traditional uses of plants by the Cadigal people, the traditional owners of the land of the Royal Botanic Garden Sydney,â says Victoria. Photo â Alisha Gore for The Design Files.
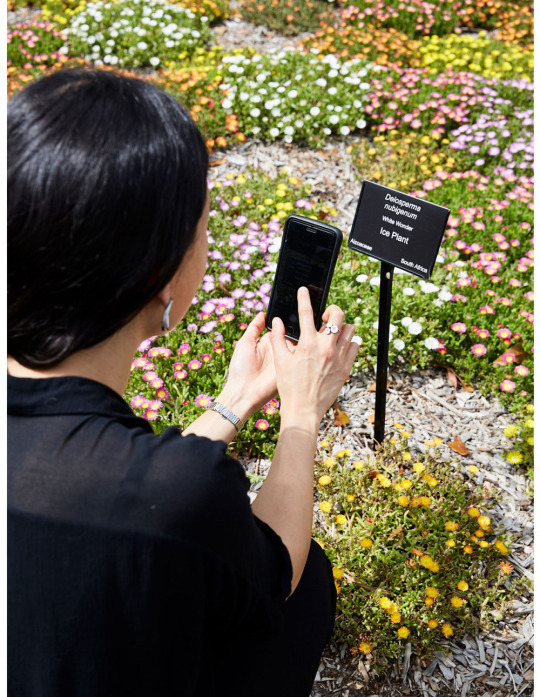
Never not content creating! Photo â Alisha Gore for The Design Files.

âSome people might not be aware that our Gardens comprise Australiaâs oldest living scientific institution, and there is so much to learn about the important work often done behind the scenes by our scientists,â says Victoria. Photo â Alisha Gore for The Design Files.
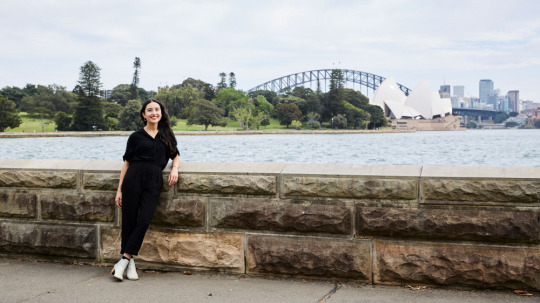
âI love how diverse my role can be. In 2019, I put together the Gardenâs first-ever Lunar New Year program to celebrate the year of the Pig.â Photo â Alisha Gore for The Design Files.
Suffering the classic high school graduate uncertainty, Victoria Ngu approached her tertiary education by applying for the uni course that would give her the most breadth in job choices later down the track: a Bachelor of Business. Here is a case for hedging your bets! She ended up majoring in marketing and law, and graduated knowing two things for sure: 1) she had zero interest in finance, 2) the diversity of communications and creative campaigns was totally her jam!
While she performed the classic study/retail work juggle during her undergraduate years, Victoria also completed a slew of internships on the side. Itâs this variety of experiences that led her first to The Sydney Opera House and then to Bangarra Dance Theatre as her first jobs out of uni. This string of incredible opportunities eventually wound up at the Royal Botanic Gardens Sydney, where she has held the role of digital marketing and media coordinator since 2017.
Victoriaâs position is hugely multifaceted, and spans the broad range of fields that fall under the Gardensâ umbrella. From horticulture to education to venue management to events, she is in constant liaison with various different stakeholders at all times. Not to mention keeping the companyâs public-facing image pristine!
The most important verb in the get-your-dream-job lexicon isâŠ
Apply! It sounds absurd, but I almost didnât apply for this job because I assumed I wouldnât have a chance. My partner convinced me to just go for it and now, looking back, it seems ridiculous that I wouldnât even try. If I had succumbed to my insecurities, I wouldnât be where I am today!
I landed this job byâŠÂ
Putting all my energy into my application. Once I ran out of reasons to not apply, I began to update my resume and write a cover letter. The application involved a series of written tasks for different digital channels and I carefully crafted responses I was happy with. I was genuinely surprised to get the call up for an interview and discovered I would also need to prepare a 10-minute presentation on top of the interview itself.
Work for me in 2020 has beenâŠ
Surreal. Iâve been working remotely since March and, while I do enjoy the improved focus that working from home allows, I do miss the Gardens themselves. Ordinarily I am based in the office at the Royal Botanic Garden Sydney, and work a few days from the Australian Botanic Garden in Mount Annan and the Blue Mountains Botanic Garden in Mount Tomah every few weeks, so working from home full-time has been a huge adjustment.
The work itself also pivoted from selling tickets to public programs and events, to focusing on promoting our at-home gardening series, being a source for inspiring content for our global audience, and a reliable outlet for gorgeous imagery. Itâs been quite a mentality shift, which required flexibility and patience to navigate this new and uncertain world.
A typical day for me involvesâŠ
Moderating the Gardensâ social media platforms (Facebook, Twitter, Instagram, YouTube and LinkedIn) is a big part of the job, especially now when we have engagement from all over the world. The three Gardens are public green spaces with millions of visitors every year, so we receive feedback, general questions and a myriad of plant-related questions that our Horticulture team help to answer.
A big part of my day is writing and editing blogs, web copy and social media captions that I schedule for the week ahead. We have so many content producers in Education, Horticulture and Science â itâs a huge number of images, videos and news to translate into engaging digital content. I also produce and analyse reports on the performance of digital channels to inform future campaigns.
The most rewarding part of my job isâŠ
Learning about plants. Itâs no exaggeration to say that Iâve learned something new about plants, horticulture or science every day Iâve been in this role. I love learning plant names and traditional uses of plants by the Cadigal people, the traditional owners of the land of the Royal Botanic Garden Sydney. Some people might not be aware that our Gardens comprise Australiaâs oldest living scientific institution, and there is so much to learn about the important work often done behind the scenes by our scientists.
I also love how diverse my role can be. In 2019, I put together the Gardenâs first-ever Lunar New Year program to celebrate the year of the Pig. It was fulfilling for me to try my hand at programming and to work on the events side, as well as the marketing and communications side of a campaign, and a special way for me to connect to my Chinese heritage.
On the other hand, the most challenging aspect isâŠ
Competing priorities. Marketing and communications support many teams in our organisation including science, education, horticulture, major events, venue services and more, and between the three Gardens, there are numerous events, projects, and stakeholders to support. My team is always busy and multi-tasking.
Something I thrive off at work isâŠ
Spending time in nature. I go for a walk every day, aiming to explore different pockets of the Garden throughout the week. One spot I return to daily is the Succulent Garden.
A piece of advice/a lesson Iâve found useful isâŠ
Where possible, eat lunch outside. Too often I find myself eating lunch at my desk on busy days. I find that by taking a break from my laptop and going outside, even if itâs a short break, I will have a more productive afternoon.
0 notes
Text
Larry Cuban on School Reform and Classroom Practice: Donors Reform Schooling: Evaluating Teachers (Part 2)
Larry Cuban on School Reform and Classroom Practice: Donors Reform Schooling: Evaluating Teachers (Part 2)
In Part 1, I described a Gates Foundation initiative aimed at identifying effective teachers as measured in part by their studentsâ test scores, rewarding such stellar teachers with cash, and giving poor and minority children access to their classrooms. Called Institute Program for Effective Teaching, the Foundation had mobilized sufficient political support for the huge grant to find and fund three school districts and four charter school networks across the nation. IPET launched in 2009 and closed it doors (and funding) in 2016.
A brief look at the largest partner in the project, Floridaâs Hillsborough County district, over the span of the grant gives a peek at how early exhilaration over the project morphed into opposition over rising program costs that had to be absorbed by the districtâs regular budget, and then key district and school staffâs growing disillusion over the projectâs direction and disappointing results for students. Consider what the Tampa Bay Times, a local paper, found in 2015 after a lengthy investigation into the grant. [i]
The Gates-funded program â which required Hillsborough to raise its own $100 million â ballooned beyond the districtâs ability to afford it, creating a new bureaucracy of mentors and âpeer evaluatorsâ who no longer work with students.
Nearly 3,000 employees got one-year raises of more than $8,000. Some were as high as $15,000, or 25 percent.
Raises went to a wider group than envisioned, including close to 500 people who donât work in the classroom full time, if at all.
The greatest share of large raises went to veteran teachers in stable suburban schools, despite the programâs stated goal of channeling better and better-paid teachers into high-needs schools.
More than $23 million of the Gates money went to consultants.
The programâs total cost has risen from $202 million to $271 million when related projects are factored in, with some of the money coming from private foundations in addition to Gates. The districtâs share now comes to $124 million.
Millions of dollars were pledged to parts of the program that educators now doubt. After investing in an elaborate system of peer evaluations to improve teaching, district leaders are considering a retreat from that model. And Gates is withholding $20 million after deciding it does not, after all, favor the idea of teacher performance bonuses â a major change in philosophy.
The end product â results in the classroom â is a mixed bag.
Hillsboroughâs graduation rate still lags behind other large school districts. Racial and economic achievement gaps remain pronounced, especially in middle school.
And poor schools still wind up with the newest, greenest teachers.
Not a pretty picture. RANDâs formal evaluation covering the life of the grant and across the three districts and four charter networks used less judgmental language but reached a similar conclusion on school outcomes that the Tampa Bay Times had for these county schools.
Overall, the initiative did not achieve its stated goals for students, particularly LIM [low-income minority students. By the end of 2014â2015, student outcomes were not dramatically better than outcomes in similar sites that did not participate in the IP initiative. Furthermore, in the sites where these analyses could be conducted, we did not find improvement in the effectiveness of newly hired teachers relative to experienced teachers; we found very few instances of improvement in the effectiveness of the teaching force overall; we found no evidence that LIM students had greater access than non-LIM students to effective teaching; and we found no increase in the retention of effective teachers, although we did find declines in the retention of ineffective teachers in most sites. [ii]
As with the history of such innovative projects in public schools over the past century, RAND evaluators found that districts and charter school networks fell short in achieving IPET because of uneven and incomplete implementation of the program.
We also examined variation in implementation and outcomes across sites. Although sites varied in context and in the ways in which they approached the levers, these differences did not translate into differences in ultimate outcomes. Although the sites implemented the same levers, they gave different degrees of emphasis to different levers, and none of the sites achieved strong implementation or outcomes across the board. [iii]
But the absolutist judgment of âfailureâ in achieving aims of this donor-funded initiative hides the rippling effects of this effort to reform teaching and learning in these districts and charter networks. For example, during the Obama administration, U.S. Secretary of Education Arne Duncanâs initiative of Race to the Top invited states to compete for grants of millions of dollars if they committed themselves to the Common Core standardsâanother Gates-funded initiativeâand included, as did IPET, different ways of evaluating teachers. [iv]
Now over 40 states and the District of Columbia have adopted plans to evaluate teachers on the basis of student test scores. How much student test scores should weigh in the overall determination of a teacherâs effectiveness varies by state and local districts as does the autonomy local districts have in putting their signature on state requirements in evaluating teachers. For example, from half of the total judgment of the teacher to one-third or one-fourth, test scores have become a significant variable in assessing a teacherâs effectiveness. Even as testing experts and academic evaluators have raised significant flags about the instability, inaccuracy, and unfairness of such district and state evaluation policies based upon student scores being put into practice, they remain on the books and have been implemented in various districts. Because the amount of time is such an important factor in putting these policies into practice, states will go through trial and error as they implement these policies possibly leading to more (or less) political acceptance from teachers and principals, key participants in the venture.[v]
While there has been a noticeable dulling of the reform glow for evaluating teachers on the basis of student performanceânote the Gates Foundation pulling back on their use in evaluating teachers as part of the half-billion dollar Intensive Partnerships for Effective Teachingâthe rise and fall in enthusiasm in using test scores, intentionally or unintentionally, has focused policy discussions on teachers as the source of school âfailureâ and inequalities among students. In pressing for teachers to be held accountable, policy elites have largely ignored other factors that influence both teacher and student performance that are deeply connected to economic and social inequalities outside the school such as poverty, neighborhood crime, discriminatory labor and housing practices, and lack of access to health centers.
By donors helping to frame an agenda for turning around âfailingâ U.S. schools or, more generously, improving equal opportunity for children and youth, these philanthropists âunaccountable to anyone and receiving tax subsidies from the federal governmentâas members of policy elites spotlight teachers as both the problem and solution to school improvement. Surely, teachers are the most important in-school factorâperhaps 10 percent of the variation in student achievement. Yet over 60 percent of the variation in student academic performance is attributed to out-of-school factors such as the family. [vi]
This Gates-funded Intensive Partnerships for Effective Teaching is an example, then, of policy elites shaping a reform agenda for the nationâs schools using teacher effectiveness as a primary criterion and having enormous direct and indirect influence in advocating and enacting other pet reforms.
Did, then, Intensive Partnerships for Effective Teaching âfail?â Part 3 answers that question.
__________________________
[i]Â (Marlene Sokol, âSticker Shock: How Hillsborough Countyâs Gates Grant Became a Budget Buster,â October 23, 2015 )
[ii]Â RAND evaluation; implementation quote, p. 488.
[iii] William Howell, âResults of President Obamaâs Race to the Top,â Education Next, 2015, 15 (4), at: https://www.educationnext.org/results-president-obama-race-to-the-top-reform/
[iv] ibid.
[v] Eduardo Porter, âGrading Teachers by the Test,â New York Times, March 24, 2015; Rachel Cohen, âTeachers Tests Test Teachers,â American Prospect, July 18, 2017; Kaitlin Pennington and Sara Mead, For Good Measure? Teacher Evaluation Policy in the ESSA Era, Bellwether Education Partners, December 2016; Edward Haertel, âReliability and Validity of Inferences about Teachers Based on Student Test Scores,â William Angoff Memorial Lecture, Washington D.C., March 22, 2013; Matthew Di Carlo, âWhy Teacher evaluation Reform Is Not a Failure,â August 23, 2018 at: http://www.shankerinstitute.org/blog/why-teacher-evaluation-reform-not-failure
[vi] Edward Haertel, âReliability and Validity of Inferences about Teachers Based on Student Test Scores,â William Angoff Memorial Lecture, Washington D.C., March 22, 2013
elaine January 7, 2019
Source
Larry Cuban on School Reform and Classroom Practice
Larry Cuban on School Reform and Classroom Practice: Donors Reform Schooling: Evaluating Teachers (Part 2) published first on https://buyessayscheapservice.tumblr.com/
0 notes
Link
NEXT TO HAVING your doctor ask you to say âaah,â having your blood examined is one of the most common diagnostic rituals. Some specific blood tests, such as that for diabetes, need just a drop from a finger stick, but broader evaluations can require a teaspoon or more of blood drawn through a needle inserted into a vein in your arm.
No one enjoys the process and some truly fear it. It is worth the pain only because blood tests can reveal conditions you and your doctor need to know about, from your bodyâs chemical balance to signs of disease. Besides the distress, there is the cost. One recent US study found a median cost of $100 for a basic blood test, with much higher costs for more sophisticated analyses. This adds up to a global blood-testing market in the tens of billions of dollars.
So when 19-year-old Elizabeth Holmes dropped out of Stanford in 2003 to realize her vision of less painful, faster, and cheaper blood testing, she quickly found eager investors. An admirer of Steve Jobs, she pitched her startup company Theranos (a portmanteau of âtherapyâ and âdiagnosisâ) as the âiPod of health care.â But instead it became the Enron of health care, a fount of corporate deceit that finally led to a federal criminal indictment of Holmes for fraud. She now awaits trial.
Bad Blood: Secrets and Lies in a Silicon Valley Startup is John Carreyrouâs gripping story of how Holmesâs great idea led to Silicon Valley stardom and then into an ethical quagmire. Carreyrou is the Wall Street Journal reporter who first revealed that Theranos was not actually achieving what Holmes claimed, though her company had been valued at $9 billion. Her net worth neared $5 billion, and her deals with Walgreens and Safeway could have put her technology into thousands of stores, thus measuring the health of millions, until Carreyrou showed that the whole impressive edifice rested on lies.
€
It didnât start that way. Impressed by her creativity and drive, Holmesâs faculty mentor at Stanford, writes Carreyrou, told her to âgo out and pursue her dream.â That required advanced technology, whereas Holmesâs scientific background consisted of a year at Stanford and an internship in a medical testing lab. Nevertheless, she conceived and patented the TheraPatch. Affixed to a patientâs arm, it would take blood painlessly through tiny needles, analyze the sample, and deliver an appropriate drug dosage. Her idea was good enough to raise $6 million from investors by the end of 2004, but it soon became clear that developing the patch was not feasible.
Holmes didnât quit. Her next idea was to have a patient prick a finger and put a drop of blood into a cartridge the size of a credit card but thicker. This would go into a âreader,â where pumps propelled the blood through a filter to hold back the red and white cells; the pumps then pushed the remaining liquid plasma into wells, where chemical reactions would provide the data to evaluate the sample. The results would quickly be sent wirelessly to the patientâs doctor. Compact and easy to use, the device could be kept in a personâs home.
In 2006, Holmes hired Edmond Ku, a Silicon Valley engineer known for solving hard problems, to turn a sketchy prototype of a Theranos 1.0 card and reader into a real product. Running a tiny volume of fluid through minute channels and into wells containing test reagents was a huge challenge in microfluidics, hardly a Silicon Valley field of expertise. As Carreyrou describes it:
All these fluids needed to flow through the cartridge in a meticulously choreographed sequence, so the cartridge contained little valves that opened and shut at precise intervals. Ed and his engineers tinkered with the design and the timing of the valves and the speed at which the various fluids were pumped through the cartridge.
But Ku never did get the system to perform reliably. Holmes was unhappy with his progress and insisted that his engineers work around the clock. Ku protested that this would only burn them out. According to Carreyrou, Holmes retorted, âI donât care. We can change people in and out. The company is all that matters.â Finally, she hired a second competing engineering team, sidelining Ku. (Later, she fired him.) She also pushed the unproven Theranos 1.0 into clinical testing before it was ready. In 2007, she persuaded the Pfizer drug company to try it at an oncology clinic in Tennessee. Ku fiddled with the device to get it working well enough to draw blood from two patients, but he was troubled by the use of this imperfect machine on actual cancer patients.
Meanwhile the second team jettisoned microfluidics, instead building a robotic arm that replicated what a human lab tech would do by taking a blood sample from a cartridge, processing it, and mixing it with test reagents. Holmes dubbed this relatively clunky device the âEdisonâ after the great inventor and immediately started showing off a prototype. Unease about the cancer test, however, had spread, and some employees wondered whether even the new Edison was reliable enough to use on patients.
As Carreyrou relates, Holmesâs management and her glowing revenue projections, which never seemed to materialize, were beginning to be questioned, in particular by Avie Tevanian, a retired Apple executive who sat on the Theranos board of directors. Holmes responded by threatening him with legal action. Tevanian resigned in 2007, and he warned the other board members that âby not going along 100% âwith the programâ they risk[ed] retribution from the Company/Elizabeth.â
He was right. Holmes was ruthless about perceived threats and obsessive about company security, and marginalized or fired anyone who failed to deliver or doubted her. Her management was backed up by Theranos chief operating officer and president Ramesh âSunnyâ Balwani. Much older than Holmes, he had prospered in the dotcom bubble and seemed to act as her mentor (it later emerged that they were in a secret relationship). To employees, his menacing management style made him Holmesâs âenforcer.â
Worst of all, Holmes continued to tout untested or nonexistent technology. Her lucrative deals with Safeway and Walgreens depended on her assurance that the Edison could perform over 200 different blood tests, whereas the device could really only do about a dozen. Holmes started a program in 2010 to develop the so-called âminiLabâ to perform what she had already promised. She told employees: âThe miniLab is the most important thing humanity has ever built.â The device ran into serious problems and in fact never worked.
Despite further whistleblowing efforts, Holmes and Balwani lied and maneuvered to keep the truth from investors, business partners, and government agencies. Eminent board members like former US Secretaries of State George Shultz and Henry Kissinger vouched for Holmes, and retired US Marine Corps General James Mattis (now President Trumpâs Secretary of Defense) praised her âmatureâ ethical sense. What the board could not verify was the validity of the technology: Holmes had not recruited any directors with the biomedical expertise to oversee and evaluate it. But others were doing just that, as Carreyrou relates in the last part of the book.
In 2014, Carreyrou received a tip from Adam Clapper, a pathologist in Missouri who had helped Carreyrou with an earlier story. Clapper had blogged about his doubts that Theranos could run many tests on just a drop of blood. He heard back from other skeptics and passed on their names to Carreyrou. After multiple tries, Carreyrou struck gold with one Alan Beam, who had just left his job as lab director at Theranos.
After Carreyrou promised him anonymity (âAlan Beamâ is a pseudonym), Beam dropped two bombshells. First, the Edisons were highly prone to error and regularly failed quality control tests. Second and more startling, most blood test results reported by Theranos in patient trials did not come from the Edisons but were secretly obtained from standard blood testing devices. Even these results were untrustworthy: the small Theranos samples had to be diluted to create the bigger liquid volumes required by conventional equipment. This changed the concentrations of the compounds the machines detected, which meant they could not be accurately measured. Beam was worried about the effects of these false results on physicians and patients who depended on them.
Carreyrou knew he had a big story if he could track down supporting evidence. In riveting detail, he recounts how he chased the evidence while Holmes and Balwani worked to derail his efforts. Theranos hired the famously effective and aggressive lawyer David Boies, who tried to stifle Carreyrou and his sources with legal threats and private investigators. Holmes also appealed directly to media magnate Rupert Murdoch, who owned the WSJ through its parent company and had invested $125 million in Theranos. Holmes told Murdoch that Carreyrou was using false information that would hurt Theranos, but Murdoch declined to intervene at the WSJ.
Carreyrouâs front page story in that newspaper, in October 2015, backed up Beamâs claims about the Edisons and the secret use of conventional testing. There was an immediate uproar, but Holmes and Balwani fought back, denying the allegations in press releases and personal appearances, and appealing to company loyalty. At one memorable meeting after the story broke, Balwani led hundreds of employees in a defiant chant: âFuck you, Carreyrou! Fuck you, Carreyrou!â
Problems arose faster than Holmes could deflect them. When Theranos submitted poor clinical data to the FDA, the agency banned the ânanotainer,â the tiny tube used for blood samples, from further use. The Centers for Medicare and Medicaid Services, the federal agency that monitors clinical labs, ran inspections that echoed Carreyrouâs findings, and banned Theranos from all blood testing. Eventually the company had to invalidate or fix nearly a million blood tests in California and Arizona. In another blow, on March 14, 2018, the Securities and Exchange Commission charged Theranos, Holmes, and Balwani with fraud. Holmes was required to relinquish control over the company and pay a $500,000 fine, and she was barred from holding any office in a public company for 10 years.
Carreyrou tells this intricate story in clear prose and with a momentum worthy of a crime novel. The only flaw, an unavoidable one, is that keeping track of the many characters is not easy â Carreyrou interviewed over 150 people. But he makes sure you know who the moral heroes are of this sad tale.
Two among them are Tyler Shultz, grandson of Theranos board member George Shultz, and Erika Cheung, both recent college grads in biology. While working at Theranos, they noticed severe problems with the blood tests and the companyâs claims about their accuracy. They got nowhere when they took their concerns to Holmes and Balwani, and to the elder Shultz. After resigning from the company out of conscience, they withstood Theranosâs attempts at intimidation and played crucial roles in uncovering what was really going on.
But many with a duty to ask questions did not. A board of directors supposedly exercises âdue diligence,â which means ensuring that a companyâs financial picture is sound and that the companyâs actions do not harm others. The Theranos board seemed little interested in either function, as Carreyrouâs story of Tyler Shultz and his grandfather shows. Later on, in a 2017 deposition for an investorâs lawsuit against Theranos, the elder Shultz finally did admit his inaction. He testified under oath that, despite escalating allegations, he had believed Holmesâs claims about her technology, saying, âThatâs what I assumed. I didnât probe into it. It didnât occur to me.â
Which brings us to the most fascinating part of the story: what power did Elizabeth Holmes have that kept people, experts or not, from simply asking, âDoes the technology work?â
Much of the answer comes from Holmes herself. In her appearances and interviews, she comes across as a smart and serious young woman. We learn that her commitment arose partly from her own fear of needles, which of course adds a compelling personal note. Many observers were also gratified that her success came in the notoriously male-oriented Silicon Valley world. Her magnetism was part of what Aswath Damodaran of the NYU Stern School of Business calls the âstoryâ of a business. Theranosâs story had the perfect protagonist â an appealing 19-year-old female Stanford dropout passionate about replacing a painful health test with a better, less painful one for the benefit of millions. âWith a story this good and a heroine this likable,â asks Damodaran in his book Narrative and Numbers, âwould you want to be the Grinch raising mundane questions about whether the product actually works?â
All this adds up to a combination of charisma and sincere belief in her goals. But Carreyrou has a darker and harsher view: that Holmesâs persuasive sincerity was a cover for a master manipulator. Noting her lies about the company finances and technology, her apparent lack of concern for those who might have been harmed by those lies, and her grandiose view of herself as âa modern-day Marie Curie,â he concludes his book with this:
Iâll leave it to the psychologists to decide whether Holmes fits the clinical profile [of a sociopath], but [âŠ] her moral compass was badly askew [âŠ] By all accounts, she had a vision that she genuinely believed in [âŠ] But in her all-consuming quest to be the second coming of Steve Jobs [âŠ] she stopped listening to sound advice and began to cut corners. Her ambition was voracious and it brooked no interference. If there was collateral damage on her way to riches and fame, so be it.
I would add one more thought. Holmes did not have the science to judge how hard it would be to realize her dream, then ignored the fact that the dream was failing. Instead she embraced Silicon Valley culture, which rewards at least the appearance of rapid disruptive change. That may not hurt anyone when the change is peripheral to peopleâs well-being, but it is dangerous when making real products that affect peopleâs health and lives. Facebookâs original motto, âMove fast and break things,â it seems, is a poor substitute for that old core tenet of medical ethics, âFirst, do no harm.â
€
Sidney Perkowitz is a professor emeritus of physics at Emory. He co-edited and contributed to Frankenstein: How a Monster Became an Icon(Pegasus Books, 2018), and is the author of Physics: A Very Short Introduction (Oxford University Press, 2019).
The post Bad Blood, Worse Ethics appeared first on Los Angeles Review of Books.
from Los Angeles Review of Books https://ift.tt/2wV1CCt
0 notes
Text
Don't Be Fooled by Data: 4 Data Analysis Pitfalls & How to Avoid Them
Posted by Tom.Capper
Digital marketing is a proudly data-driven field. Yet, as SEOs especially, we often have such incomplete or questionable data to work with, that we end up jumping to the wrong conclusions in our attempts to substantiate our arguments or quantify our issues and opportunities.
In this post, Iâm going to outline 4 data analysis pitfalls that are endemic in our industry, and how to avoid them.
1. Jumping to conclusions
Earlier this year, I conducted a ranking factor study around brand awareness, and I posted this caveat:
"...the fact that Domain Authority (or branded search volume, or anything else) is positively correlated with rankings could indicate that any or all of the following is likely:
Links cause sites to rank well
Ranking well causes sites to get links
Some third factor (e.g. reputation or age of site) causes sites to get both links and rankings" ~ Me
However, I want to go into this in a bit more depth and give you a framework for analyzing these yourself, because it still comes up a lot. Take, for example, this recent study by Stone Temple, which you may have seen in the Moz Top 10 or Randâs tweets, or this excellent article discussing SEMRushâs recent direct traffic findings. To be absolutely clear, Iâm not criticizing either of the studies, but I do want to draw attention to how we might interpret them.
Firstly, we do tend to suffer a little confirmation bias â weâre all too eager to call out the clichĂ© âcorrelation vs. causationâ distinction when we see successful sites that are keyword-stuffed, but all too approving when we see studies doing the same with something we think is or was effective, like links.
Secondly, we fail to critically analyze the potential mechanisms. The options arenât just causation or coincidence.
Before you jump to a conclusion based on a correlation, youâre obliged to consider various possibilities:
Complete coincidence
Reverse causation
Joint causation
Linearity
Broad applicability
If those donât make any sense, then thatâs fair enough â theyâre jargon. Letâs go through an example:
Before I warn you not to eat cheese because you may die in your bedsheets, Iâm obliged to check that it isnât any of the following:
Complete coincidence - Is it possible that so many datasets were compared, that some were bound to be similar? Why, thatâs exactly what Tyler Vigen did! Yes, this is possible.
Reverse causation - Is it possible that we have this the wrong way around? For example, perhaps your relatives, in mourning for your bedsheet-related death, eat cheese in large quantities to comfort themselves? This seems pretty unlikely, so letâs give it a pass. No, this is very unlikely.
Joint causation - Is it possible that some third factor is behind both of these? Maybe increasing affluence makes you healthier (so you donât die of things like malnutrition), and also causes you to eat more cheese? This seems very plausible. Yes, this is possible.
Linearity - Are we comparing two linear trends? A linear trend is a steady rate of growth or decline. Any two statistics which are both roughly linear over time will be very well correlated. In the graph above, both our statistics are trending linearly upwards. If the graph was drawn with different scales, they might look completely unrelated, like this, but because they both have a steady rate, theyâd still be very well correlated. Yes, this looks likely.
Broad applicability - Is it possible that this relationship only exists in certain niche scenarios, or, at least, not in my niche scenario? Perhaps, for example, cheese does this to some people, and thatâs been enough to create this correlation, because there are so few bedsheet-tangling fatalities otherwise? Yes, this seems possible.
So we have 4 âYesâ answers and one âNoâ answer from those 5 checks.
If your example doesnât get 5 âNoâ answers from those 5 checks, itâs a fail, and you donât get to say that the study has established either a ranking factor or a fatal side effect of cheese consumption.
A similar process should apply to case studies, which are another form of correlation â the correlation between you making a change, and something good (or bad!) happening. For example, ask:
Have I ruled out other factors (e.g. external demand, seasonality, competitors making mistakes)?
Did I increase traffic by doing the thing I tried to do, or did I accidentally improve some other factor at the same time?
Did this work because of the unique circumstance of the particular client/project?
This is particularly challenging for SEOs, because we rarely have data of this quality, but Iâd suggest an additional pair of questions to help you navigate this minefield:
If I were Google, would I do this?
If I were Google, could I do this?
Direct traffic as a ranking factor passes the âcouldâ test, but only barely â Google could use data from Chrome, Android, or ISPs, but itâd be sketchy. It doesnât really pass the âwouldâ test, though â itâd be far easier for Google to use branded search traffic, which would answer the same questions you might try to answer by comparing direct traffic levels (e.g. how popular is this website?).
2. Missing the context
If I told you that my traffic was up 20% week on week today, what would you say? Congratulations?
What if it was up 20% this time last year?
What if I told you it had been up 20% year on year, up until recently?
Itâs funny how a little context can completely change this. This is another problem with case studies and their evil inverted twin, traffic drop analyses.
If we really want to understand whether to be surprised at something, positively or negatively, we need to compare it to our expectations, and then figure out what deviation from our expectations is ânormal.â If this is starting to sound like statistics, thatâs because it is statistics â indeed, I wrote about a statistical approach to measuring change way back in 2015.
If you want to be lazy, though, a good rule of thumb is to zoom out, and add in those previous years. And if someone shows you data that is suspiciously zoomed in, you might want to take it with a pinch of salt.
3. Trusting our tools
Would you make a multi-million dollar business decision based on a number that your competitor could manipulate at will? Well, chances are you do, and the number can be found in Google Analytics. Iâve covered this extensively in other places, but there are some major problems with most analytics platforms around:
How easy they are to manipulate externally
How arbitrarily they group hits into sessions
How vulnerable they are to ad blockers
How they perform under sampling, and how obvious they make this
For example, did you know that the Google Analytics API v3 can heavily sample data whilst telling you that the data is unsampled, above a certain amount of traffic (~500,000 within date range)? Neither did I, until we ran into it whilst building Distilled ODN.
Similar problems exist with many âSearch Analyticsâ tools. My colleague Sam Nemzer has written a bunch about this â did you know that most rank tracking platforms report completely different rankings? Or how about the fact that the keywords grouped by Google (and thus tools like SEMRush and STAT, too) are not equivalent, and donât necessarily have the volumes quoted?
Itâs important to understand the strengths and weaknesses of tools that we use, so that we can at least know when theyâre directionally accurate (as in, their insights guide you in the right direction), even if not perfectly accurate. All I can really recommend here is that skilling up in SEO (or any other digital channel) necessarily means understanding the mechanics behind your measurement platforms â which is why all new starts at Distilled end up learning how to do analytics audits.
One of the most common solutions to the root problem is combining multiple data sources, butâŠ
4. Combining data sources
There are numerous platforms out there that will âdefeat (not provided)â by bringing together data from two or more of:
Analytics
Search Console
AdWords
Rank tracking
The problems here are that, firstly, these platforms do not have equivalent definitions, and secondly, ironically, (not provided) tends to break them.
Letâs deal with definitions first, with an example â letâs look at a landing page with a channel:
In Search Console, these are reported as clicks, and can be vulnerable to heavy, invisible sampling when multiple dimensions (e.g. keyword and page) or filters are combined.
In Google Analytics, these are reported using last non-direct click, meaning that your organic traffic includes a bunch of direct sessions, time-outs that resumed mid-session, etc. Thatâs without getting into dark traffic, ad blockers, etc.
In AdWords, most reporting uses last AdWords click, and conversions may be defined differently. In addition, keyword volumes are bundled, as referenced above.
Rank tracking is location specific, and inconsistent, as referenced above.
Fine, though â it may not be precise, but you can at least get to some directionally useful data given these limitations. However, about that â(not provided)â...
Most of your landing pages get traffic from more than one keyword. Itâs very likely that some of these keywords convert better than others, particularly if they are branded, meaning that even the most thorough click-through rate model isnât going to help you. So how do you know which keywords are valuable?
The best answer is to generalize from AdWords data for those keywords, but itâs very unlikely that you have analytics data for all those combinations of keyword and landing page. Essentially, the tools that report on this make the very bold assumption that a given page converts identically for all keywords. Some are more transparent about this than others.
Again, this isnât to say that those tools arenât valuable â they just need to be understood carefully. The only way you could reliably fill in these blanks created by ânot providedâ would be to spend a ton on paid search to get decent volume, conversion rate, and bounce rate estimates for all your keywords, and even then, youâve not fixed the inconsistent definitions issues.
Bonus peeve: Average rank
I still see this way too often. Three questions:
Do you care more about losing rankings for ten very low volume queries (10 searches a month or less) than for one high volume query (millions plus)? If the answer isnât âyes, I absolutely care more about the ten low-volume queriesâ, then this metric isnât for you, and you should consider a visibility metric based on click through rate estimates.
When you start ranking at 100 for a keyword you didnât rank for before, does this make you unhappy? If the answer isnât âyes, I hate ranking for new keywords,â then this metric isnât for you â because that will lower your average rank. You could of course treat all non-ranking keywords as position 100, as some tools allow, but is a drop of 2 average rank positions really the best way to express that 1/50 of your landing pages have been de-indexed? Again, use a visibility metric, please.
Do you like comparing your performance with your competitors? If the answer isnât âno, of course not,â then this metric isnât for you â your competitors may have more or fewer branded keywords or long-tail rankings, and these will skew the comparison. Again, use a visibility metric.
Conclusion
Hopefully, youâve found this useful. To summarize the main takeaways:
Critically analyse correlations & case studies by seeing if you can explain them as coincidences, as reverse causation, as joint causation, through reference to a third mutually relevant factor, or through niche applicability.
Donât look at changes in traffic without looking at the context â what would you have forecasted for this period, and with what margin of error?
Remember that the tools we use have limitations, and do your research on how that impacts the numbers they show. âHow has this number been produced?â is an important component in âWhat does this number mean?â
If you end up combining data from multiple tools, remember to work out the relationship between them â treat this information as directional rather than precise.
Let me know what data analysis fallacies bug you, in the comments below.
Sign up for The Moz Top 10, a semimonthly mailer updating you on the top ten hottest pieces of SEO news, tips, and rad links uncovered by the Moz team. Think of it as your exclusive digest of stuff you don't have time to hunt down but want to read!
http://ift.tt/2nAc68g
0 notes
Text
Don't Be Fooled by Data: 4 Data Analysis Pitfalls & How to Avoid Them
Posted by Tom.Capper
Digital marketing is a proudly data-driven field. Yet, as SEOs especially, we often have such incomplete or questionable data to work with, that we end up jumping to the wrong conclusions in our attempts to substantiate our arguments or quantify our issues and opportunities.
In this post, Iâm going to outline 4 data analysis pitfalls that are endemic in our industry, and how to avoid them.
1. Jumping to conclusions
Earlier this year, I conducted a ranking factor study around brand awareness, and I posted this caveat:
"...the fact that Domain Authority (or branded search volume, or anything else) is positively correlated with rankings could indicate that any or all of the following is likely:
Links cause sites to rank well
Ranking well causes sites to get links
Some third factor (e.g. reputation or age of site) causes sites to get both links and rankings" ~ Me
However, I want to go into this in a bit more depth and give you a framework for analyzing these yourself, because it still comes up a lot. Take, for example, this recent study by Stone Temple, which you may have seen in the Moz Top 10 or Randâs tweets, or this excellent article discussing SEMRushâs recent direct traffic findings. To be absolutely clear, Iâm not criticizing either of the studies, but I do want to draw attention to how we might interpret them.
Firstly, we do tend to suffer a little confirmation bias â weâre all too eager to call out the clichĂ© âcorrelation vs. causationâ distinction when we see successful sites that are keyword-stuffed, but all too approving when we see studies doing the same with something we think is or was effective, like links.
Secondly, we fail to critically analyze the potential mechanisms. The options arenât just causation or coincidence.
Before you jump to a conclusion based on a correlation, youâre obliged to consider various possibilities:
Complete coincidence
Reverse causation
Joint causation
Linearity
Broad applicability
If those donât make any sense, then thatâs fair enough â theyâre jargon. Letâs go through an example:
Before I warn you not to eat cheese because you may die in your bedsheets, Iâm obliged to check that it isnât any of the following:
Complete coincidence - Is it possible that so many datasets were compared, that some were bound to be similar? Why, thatâs exactly what Tyler Vigen did! Yes, this is possible.
Reverse causation - Is it possible that we have this the wrong way around? For example, perhaps your relatives, in mourning for your bedsheet-related death, eat cheese in large quantities to comfort themselves? This seems pretty unlikely, so letâs give it a pass. No, this is very unlikely.
Joint causation - Is it possible that some third factor is behind both of these? Maybe increasing affluence makes you healthier (so you donât die of things like malnutrition), and also causes you to eat more cheese? This seems very plausible. Yes, this is possible.
Linearity - Are we comparing two linear trends? A linear trend is a steady rate of growth or decline. Any two statistics which are both roughly linear over time will be very well correlated. In the graph above, both our statistics are trending linearly upwards. If the graph was drawn with different scales, they might look completely unrelated, like this, but because they both have a steady rate, theyâd still be very well correlated. Yes, this looks likely.
Broad applicability - Is it possible that this relationship only exists in certain niche scenarios, or, at least, not in my niche scenario? Perhaps, for example, cheese does this to some people, and thatâs been enough to create this correlation, because there are so few bedsheet-tangling fatalities otherwise? Yes, this seems possible.
So we have 4 âYesâ answers and one âNoâ answer from those 5 checks.
If your example doesnât get 5 âNoâ answers from those 5 checks, itâs a fail, and you donât get to say that the study has established either a ranking factor or a fatal side effect of cheese consumption.
A similar process should apply to case studies, which are another form of correlation â the correlation between you making a change, and something good (or bad!) happening. For example, ask:
Have I ruled out other factors (e.g. external demand, seasonality, competitors making mistakes)?
Did I increase traffic by doing the thing I tried to do, or did I accidentally improve some other factor at the same time?
Did this work because of the unique circumstance of the particular client/project?
This is particularly challenging for SEOs, because we rarely have data of this quality, but Iâd suggest an additional pair of questions to help you navigate this minefield:
If I were Google, would I do this?
If I were Google, could I do this?
Direct traffic as a ranking factor passes the âcouldâ test, but only barely â Google could use data from Chrome, Android, or ISPs, but itâd be sketchy. It doesnât really pass the âwouldâ test, though â itâd be far easier for Google to use branded search traffic, which would answer the same questions you might try to answer by comparing direct traffic levels (e.g. how popular is this website?).
2. Missing the context
If I told you that my traffic was up 20% week on week today, what would you say? Congratulations?
What if it was up 20% this time last year?
What if I told you it had been up 20% year on year, up until recently?
Itâs funny how a little context can completely change this. This is another problem with case studies and their evil inverted twin, traffic drop analyses.
If we really want to understand whether to be surprised at something, positively or negatively, we need to compare it to our expectations, and then figure out what deviation from our expectations is ânormal.â If this is starting to sound like statistics, thatâs because it is statistics â indeed, I wrote about a statistical approach to measuring change way back in 2015.
If you want to be lazy, though, a good rule of thumb is to zoom out, and add in those previous years. And if someone shows you data that is suspiciously zoomed in, you might want to take it with a pinch of salt.
3. Trusting our tools
Would you make a multi-million dollar business decision based on a number that your competitor could manipulate at will? Well, chances are you do, and the number can be found in Google Analytics. Iâve covered this extensively in other places, but there are some major problems with most analytics platforms around:
How easy they are to manipulate externally
How arbitrarily they group hits into sessions
How vulnerable they are to ad blockers
How they perform under sampling, and how obvious they make this
For example, did you know that the Google Analytics API v3 can heavily sample data whilst telling you that the data is unsampled, above a certain amount of traffic (~500,000 within date range)? Neither did I, until we ran into it whilst building Distilled ODN.
Similar problems exist with many âSearch Analyticsâ tools. My colleague Sam Nemzer has written a bunch about this â did you know that most rank tracking platforms report completely different rankings? Or how about the fact that the keywords grouped by Google (and thus tools like SEMRush and STAT, too) are not equivalent, and donât necessarily have the volumes quoted?
Itâs important to understand the strengths and weaknesses of tools that we use, so that we can at least know when theyâre directionally accurate (as in, their insights guide you in the right direction), even if not perfectly accurate. All I can really recommend here is that skilling up in SEO (or any other digital channel) necessarily means understanding the mechanics behind your measurement platforms â which is why all new starts at Distilled end up learning how to do analytics audits.
One of the most common solutions to the root problem is combining multiple data sources, butâŠ
4. Combining data sources
There are numerous platforms out there that will âdefeat (not provided)â by bringing together data from two or more of:
Analytics
Search Console
AdWords
Rank tracking
The problems here are that, firstly, these platforms do not have equivalent definitions, and secondly, ironically, (not provided) tends to break them.
Letâs deal with definitions first, with an example â letâs look at a landing page with a channel:
In Search Console, these are reported as clicks, and can be vulnerable to heavy, invisible sampling when multiple dimensions (e.g. keyword and page) or filters are combined.
In Google Analytics, these are reported using last non-direct click, meaning that your organic traffic includes a bunch of direct sessions, time-outs that resumed mid-session, etc. Thatâs without getting into dark traffic, ad blockers, etc.
In AdWords, most reporting uses last AdWords click, and conversions may be defined differently. In addition, keyword volumes are bundled, as referenced above.
Rank tracking is location specific, and inconsistent, as referenced above.
Fine, though â it may not be precise, but you can at least get to some directionally useful data given these limitations. However, about that â(not provided)â...
Most of your landing pages get traffic from more than one keyword. Itâs very likely that some of these keywords convert better than others, particularly if they are branded, meaning that even the most thorough click-through rate model isnât going to help you. So how do you know which keywords are valuable?
The best answer is to generalize from AdWords data for those keywords, but itâs very unlikely that you have analytics data for all those combinations of keyword and landing page. Essentially, the tools that report on this make the very bold assumption that a given page converts identically for all keywords. Some are more transparent about this than others.
Again, this isnât to say that those tools arenât valuable â they just need to be understood carefully. The only way you could reliably fill in these blanks created by ânot providedâ would be to spend a ton on paid search to get decent volume, conversion rate, and bounce rate estimates for all your keywords, and even then, youâve not fixed the inconsistent definitions issues.
Bonus peeve: Average rank
I still see this way too often. Three questions:
Do you care more about losing rankings for ten very low volume queries (10 searches a month or less) than for one high volume query (millions plus)? If the answer isnât âyes, I absolutely care more about the ten low-volume queriesâ, then this metric isnât for you, and you should consider a visibility metric based on click through rate estimates.
When you start ranking at 100 for a keyword you didnât rank for before, does this make you unhappy? If the answer isnât âyes, I hate ranking for new keywords,â then this metric isnât for you â because that will lower your average rank. You could of course treat all non-ranking keywords as position 100, as some tools allow, but is a drop of 2 average rank positions really the best way to express that 1/50 of your landing pages have been de-indexed? Again, use a visibility metric, please.
Do you like comparing your performance with your competitors? If the answer isnât âno, of course not,â then this metric isnât for you â your competitors may have more or fewer branded keywords or long-tail rankings, and these will skew the comparison. Again, use a visibility metric.
Conclusion
Hopefully, youâve found this useful. To summarize the main takeaways:
Critically analyse correlations & case studies by seeing if you can explain them as coincidences, as reverse causation, as joint causation, through reference to a third mutually relevant factor, or through niche applicability.
Donât look at changes in traffic without looking at the context â what would you have forecasted for this period, and with what margin of error?
Remember that the tools we use have limitations, and do your research on how that impacts the numbers they show. âHow has this number been produced?â is an important component in âWhat does this number mean?â
If you end up combining data from multiple tools, remember to work out the relationship between them â treat this information as directional rather than precise.
Let me know what data analysis fallacies bug you, in the comments below.
Sign up for The Moz Top 10, a semimonthly mailer updating you on the top ten hottest pieces of SEO news, tips, and rad links uncovered by the Moz team. Think of it as your exclusive digest of stuff you don't have time to hunt down but want to read!
http://ift.tt/2nAc68g
0 notes
Text
Don't Be Fooled by Data: 4 Data Analysis Pitfalls & How to Avoid Them
Posted by Tom.Capper
Digital marketing is a proudly data-driven field. Yet, as SEOs especially, we often have such incomplete or questionable data to work with, that we end up jumping to the wrong conclusions in our attempts to substantiate our arguments or quantify our issues and opportunities.
In this post, Iâm going to outline 4 data analysis pitfalls that are endemic in our industry, and how to avoid them.
1. Jumping to conclusions
Earlier this year, I conducted a ranking factor study around brand awareness, and I posted this caveat:
"...the fact that Domain Authority (or branded search volume, or anything else) is positively correlated with rankings could indicate that any or all of the following is likely:
Links cause sites to rank well
Ranking well causes sites to get links
Some third factor (e.g. reputation or age of site) causes sites to get both links and rankings" ~ Me
However, I want to go into this in a bit more depth and give you a framework for analyzing these yourself, because it still comes up a lot. Take, for example, this recent study by Stone Temple, which you may have seen in the Moz Top 10 or Randâs tweets, or this excellent article discussing SEMRushâs recent direct traffic findings. To be absolutely clear, Iâm not criticizing either of the studies, but I do want to draw attention to how we might interpret them.
Firstly, we do tend to suffer a little confirmation bias â weâre all too eager to call out the clichĂ© âcorrelation vs. causationâ distinction when we see successful sites that are keyword-stuffed, but all too approving when we see studies doing the same with something we think is or was effective, like links.
Secondly, we fail to critically analyze the potential mechanisms. The options arenât just causation or coincidence.
Before you jump to a conclusion based on a correlation, youâre obliged to consider various possibilities:
Complete coincidence
Reverse causation
Joint causation
Linearity
Broad applicability
If those donât make any sense, then thatâs fair enough â theyâre jargon. Letâs go through an example:
Before I warn you not to eat cheese because you may die in your bedsheets, Iâm obliged to check that it isnât any of the following:
Complete coincidence - Is it possible that so many datasets were compared, that some were bound to be similar? Why, thatâs exactly what Tyler Vigen did! Yes, this is possible.
Reverse causation - Is it possible that we have this the wrong way around? For example, perhaps your relatives, in mourning for your bedsheet-related death, eat cheese in large quantities to comfort themselves? This seems pretty unlikely, so letâs give it a pass. No, this is very unlikely.
Joint causation - Is it possible that some third factor is behind both of these? Maybe increasing affluence makes you healthier (so you donât die of things like malnutrition), and also causes you to eat more cheese? This seems very plausible. Yes, this is possible.
Linearity - Are we comparing two linear trends? A linear trend is a steady rate of growth or decline. Any two statistics which are both roughly linear over time will be very well correlated. In the graph above, both our statistics are trending linearly upwards. If the graph was drawn with different scales, they might look completely unrelated, like this, but because they both have a steady rate, theyâd still be very well correlated. Yes, this looks likely.
Broad applicability - Is it possible that this relationship only exists in certain niche scenarios, or, at least, not in my niche scenario? Perhaps, for example, cheese does this to some people, and thatâs been enough to create this correlation, because there are so few bedsheet-tangling fatalities otherwise? Yes, this seems possible.
So we have 4 âYesâ answers and one âNoâ answer from those 5 checks.
If your example doesnât get 5 âNoâ answers from those 5 checks, itâs a fail, and you donât get to say that the study has established either a ranking factor or a fatal side effect of cheese consumption.
A similar process should apply to case studies, which are another form of correlation â the correlation between you making a change, and something good (or bad!) happening. For example, ask:
Have I ruled out other factors (e.g. external demand, seasonality, competitors making mistakes)?
Did I increase traffic by doing the thing I tried to do, or did I accidentally improve some other factor at the same time?
Did this work because of the unique circumstance of the particular client/project?
This is particularly challenging for SEOs, because we rarely have data of this quality, but Iâd suggest an additional pair of questions to help you navigate this minefield:
If I were Google, would I do this?
If I were Google, could I do this?
Direct traffic as a ranking factor passes the âcouldâ test, but only barely â Google could use data from Chrome, Android, or ISPs, but itâd be sketchy. It doesnât really pass the âwouldâ test, though â itâd be far easier for Google to use branded search traffic, which would answer the same questions you might try to answer by comparing direct traffic levels (e.g. how popular is this website?).
2. Missing the context
If I told you that my traffic was up 20% week on week today, what would you say? Congratulations?
What if it was up 20% this time last year?
What if I told you it had been up 20% year on year, up until recently?
Itâs funny how a little context can completely change this. This is another problem with case studies and their evil inverted twin, traffic drop analyses.
If we really want to understand whether to be surprised at something, positively or negatively, we need to compare it to our expectations, and then figure out what deviation from our expectations is ânormal.â If this is starting to sound like statistics, thatâs because it is statistics â indeed, I wrote about a statistical approach to measuring change way back in 2015.
If you want to be lazy, though, a good rule of thumb is to zoom out, and add in those previous years. And if someone shows you data that is suspiciously zoomed in, you might want to take it with a pinch of salt.
3. Trusting our tools
Would you make a multi-million dollar business decision based on a number that your competitor could manipulate at will? Well, chances are you do, and the number can be found in Google Analytics. Iâve covered this extensively in other places, but there are some major problems with most analytics platforms around:
How easy they are to manipulate externally
How arbitrarily they group hits into sessions
How vulnerable they are to ad blockers
How they perform under sampling, and how obvious they make this
For example, did you know that the Google Analytics API v3 can heavily sample data whilst telling you that the data is unsampled, above a certain amount of traffic (~500,000 within date range)? Neither did I, until we ran into it whilst building Distilled ODN.
Similar problems exist with many âSearch Analyticsâ tools. My colleague Sam Nemzer has written a bunch about this â did you know that most rank tracking platforms report completely different rankings? Or how about the fact that the keywords grouped by Google (and thus tools like SEMRush and STAT, too) are not equivalent, and donât necessarily have the volumes quoted?
Itâs important to understand the strengths and weaknesses of tools that we use, so that we can at least know when theyâre directionally accurate (as in, their insights guide you in the right direction), even if not perfectly accurate. All I can really recommend here is that skilling up in SEO (or any other digital channel) necessarily means understanding the mechanics behind your measurement platforms â which is why all new starts at Distilled end up learning how to do analytics audits.
One of the most common solutions to the root problem is combining multiple data sources, butâŠ
4. Combining data sources
There are numerous platforms out there that will âdefeat (not provided)â by bringing together data from two or more of:
Analytics
Search Console
AdWords
Rank tracking
The problems here are that, firstly, these platforms do not have equivalent definitions, and secondly, ironically, (not provided) tends to break them.
Letâs deal with definitions first, with an example â letâs look at a landing page with a channel:
In Search Console, these are reported as clicks, and can be vulnerable to heavy, invisible sampling when multiple dimensions (e.g. keyword and page) or filters are combined.
In Google Analytics, these are reported using last non-direct click, meaning that your organic traffic includes a bunch of direct sessions, time-outs that resumed mid-session, etc. Thatâs without getting into dark traffic, ad blockers, etc.
In AdWords, most reporting uses last AdWords click, and conversions may be defined differently. In addition, keyword volumes are bundled, as referenced above.
Rank tracking is location specific, and inconsistent, as referenced above.
Fine, though â it may not be precise, but you can at least get to some directionally useful data given these limitations. However, about that â(not provided)â...
Most of your landing pages get traffic from more than one keyword. Itâs very likely that some of these keywords convert better than others, particularly if they are branded, meaning that even the most thorough click-through rate model isnât going to help you. So how do you know which keywords are valuable?
The best answer is to generalize from AdWords data for those keywords, but itâs very unlikely that you have analytics data for all those combinations of keyword and landing page. Essentially, the tools that report on this make the very bold assumption that a given page converts identically for all keywords. Some are more transparent about this than others.
Again, this isnât to say that those tools arenât valuable â they just need to be understood carefully. The only way you could reliably fill in these blanks created by ânot providedâ would be to spend a ton on paid search to get decent volume, conversion rate, and bounce rate estimates for all your keywords, and even then, youâve not fixed the inconsistent definitions issues.
Bonus peeve: Average rank
I still see this way too often. Three questions:
Do you care more about losing rankings for ten very low volume queries (10 searches a month or less) than for one high volume query (millions plus)? If the answer isnât âyes, I absolutely care more about the ten low-volume queriesâ, then this metric isnât for you, and you should consider a visibility metric based on click through rate estimates.
When you start ranking at 100 for a keyword you didnât rank for before, does this make you unhappy? If the answer isnât âyes, I hate ranking for new keywords,â then this metric isnât for you â because that will lower your average rank. You could of course treat all non-ranking keywords as position 100, as some tools allow, but is a drop of 2 average rank positions really the best way to express that 1/50 of your landing pages have been de-indexed? Again, use a visibility metric, please.
Do you like comparing your performance with your competitors? If the answer isnât âno, of course not,â then this metric isnât for you â your competitors may have more or fewer branded keywords or long-tail rankings, and these will skew the comparison. Again, use a visibility metric.
Conclusion
Hopefully, youâve found this useful. To summarize the main takeaways:
Critically analyse correlations & case studies by seeing if you can explain them as coincidences, as reverse causation, as joint causation, through reference to a third mutually relevant factor, or through niche applicability.
Donât look at changes in traffic without looking at the context â what would you have forecasted for this period, and with what margin of error?
Remember that the tools we use have limitations, and do your research on how that impacts the numbers they show. âHow has this number been produced?â is an important component in âWhat does this number mean?â
If you end up combining data from multiple tools, remember to work out the relationship between them â treat this information as directional rather than precise.
Let me know what data analysis fallacies bug you, in the comments below.
Sign up for The Moz Top 10, a semimonthly mailer updating you on the top ten hottest pieces of SEO news, tips, and rad links uncovered by the Moz team. Think of it as your exclusive digest of stuff you don't have time to hunt down but want to read!
http://ift.tt/2nAc68g
0 notes
Text
Don't Be Fooled by Data: 4 Data Analysis Pitfalls & How to Avoid Them
Posted by Tom.Capper
Digital marketing is a proudly data-driven field. Yet, as SEOs especially, we often have such incomplete or questionable data to work with, that we end up jumping to the wrong conclusions in our attempts to substantiate our arguments or quantify our issues and opportunities.
In this post, Iâm going to outline 4 data analysis pitfalls that are endemic in our industry, and how to avoid them.
1. Jumping to conclusions
Earlier this year, I conducted a ranking factor study around brand awareness, and I posted this caveat:
"...the fact that Domain Authority (or branded search volume, or anything else) is positively correlated with rankings could indicate that any or all of the following is likely:
Links cause sites to rank well
Ranking well causes sites to get links
Some third factor (e.g. reputation or age of site) causes sites to get both links and rankings" ~ Me
However, I want to go into this in a bit more depth and give you a framework for analyzing these yourself, because it still comes up a lot. Take, for example, this recent study by Stone Temple, which you may have seen in the Moz Top 10 or Randâs tweets, or this excellent article discussing SEMRushâs recent direct traffic findings. To be absolutely clear, Iâm not criticizing either of the studies, but I do want to draw attention to how we might interpret them.
Firstly, we do tend to suffer a little confirmation bias â weâre all too eager to call out the clichĂ© âcorrelation vs. causationâ distinction when we see successful sites that are keyword-stuffed, but all too approving when we see studies doing the same with something we think is or was effective, like links.
Secondly, we fail to critically analyze the potential mechanisms. The options arenât just causation or coincidence.
Before you jump to a conclusion based on a correlation, youâre obliged to consider various possibilities:
Complete coincidence
Reverse causation
Joint causation
Linearity
Broad applicability
If those donât make any sense, then thatâs fair enough â theyâre jargon. Letâs go through an example:
Before I warn you not to eat cheese because you may die in your bedsheets, Iâm obliged to check that it isnât any of the following:
Complete coincidence - Is it possible that so many datasets were compared, that some were bound to be similar? Why, thatâs exactly what Tyler Vigen did! Yes, this is possible.
Reverse causation - Is it possible that we have this the wrong way around? For example, perhaps your relatives, in mourning for your bedsheet-related death, eat cheese in large quantities to comfort themselves? This seems pretty unlikely, so letâs give it a pass. No, this is very unlikely.
Joint causation - Is it possible that some third factor is behind both of these? Maybe increasing affluence makes you healthier (so you donât die of things like malnutrition), and also causes you to eat more cheese? This seems very plausible. Yes, this is possible.
Linearity - Are we comparing two linear trends? A linear trend is a steady rate of growth or decline. Any two statistics which are both roughly linear over time will be very well correlated. In the graph above, both our statistics are trending linearly upwards. If the graph was drawn with different scales, they might look completely unrelated, like this, but because they both have a steady rate, theyâd still be very well correlated. Yes, this looks likely.
Broad applicability - Is it possible that this relationship only exists in certain niche scenarios, or, at least, not in my niche scenario? Perhaps, for example, cheese does this to some people, and thatâs been enough to create this correlation, because there are so few bedsheet-tangling fatalities otherwise? Yes, this seems possible.
So we have 4 âYesâ answers and one âNoâ answer from those 5 checks.
If your example doesnât get 5 âNoâ answers from those 5 checks, itâs a fail, and you donât get to say that the study has established either a ranking factor or a fatal side effect of cheese consumption.
A similar process should apply to case studies, which are another form of correlation â the correlation between you making a change, and something good (or bad!) happening. For example, ask:
Have I ruled out other factors (e.g. external demand, seasonality, competitors making mistakes)?
Did I increase traffic by doing the thing I tried to do, or did I accidentally improve some other factor at the same time?
Did this work because of the unique circumstance of the particular client/project?
This is particularly challenging for SEOs, because we rarely have data of this quality, but Iâd suggest an additional pair of questions to help you navigate this minefield:
If I were Google, would I do this?
If I were Google, could I do this?
Direct traffic as a ranking factor passes the âcouldâ test, but only barely â Google could use data from Chrome, Android, or ISPs, but itâd be sketchy. It doesnât really pass the âwouldâ test, though â itâd be far easier for Google to use branded search traffic, which would answer the same questions you might try to answer by comparing direct traffic levels (e.g. how popular is this website?).
2. Missing the context
If I told you that my traffic was up 20% week on week today, what would you say? Congratulations?
What if it was up 20% this time last year?
What if I told you it had been up 20% year on year, up until recently?
Itâs funny how a little context can completely change this. This is another problem with case studies and their evil inverted twin, traffic drop analyses.
If we really want to understand whether to be surprised at something, positively or negatively, we need to compare it to our expectations, and then figure out what deviation from our expectations is ânormal.â If this is starting to sound like statistics, thatâs because it is statistics â indeed, I wrote about a statistical approach to measuring change way back in 2015.
If you want to be lazy, though, a good rule of thumb is to zoom out, and add in those previous years. And if someone shows you data that is suspiciously zoomed in, you might want to take it with a pinch of salt.
3. Trusting our tools
Would you make a multi-million dollar business decision based on a number that your competitor could manipulate at will? Well, chances are you do, and the number can be found in Google Analytics. Iâve covered this extensively in other places, but there are some major problems with most analytics platforms around:
How easy they are to manipulate externally
How arbitrarily they group hits into sessions
How vulnerable they are to ad blockers
How they perform under sampling, and how obvious they make this
For example, did you know that the Google Analytics API v3 can heavily sample data whilst telling you that the data is unsampled, above a certain amount of traffic (~500,000 within date range)? Neither did I, until we ran into it whilst building Distilled ODN.
Similar problems exist with many âSearch Analyticsâ tools. My colleague Sam Nemzer has written a bunch about this â did you know that most rank tracking platforms report completely different rankings? Or how about the fact that the keywords grouped by Google (and thus tools like SEMRush and STAT, too) are not equivalent, and donât necessarily have the volumes quoted?
Itâs important to understand the strengths and weaknesses of tools that we use, so that we can at least know when theyâre directionally accurate (as in, their insights guide you in the right direction), even if not perfectly accurate. All I can really recommend here is that skilling up in SEO (or any other digital channel) necessarily means understanding the mechanics behind your measurement platforms â which is why all new starts at Distilled end up learning how to do analytics audits.
One of the most common solutions to the root problem is combining multiple data sources, butâŠ
4. Combining data sources
There are numerous platforms out there that will âdefeat (not provided)â by bringing together data from two or more of:
Analytics
Search Console
AdWords
Rank tracking
The problems here are that, firstly, these platforms do not have equivalent definitions, and secondly, ironically, (not provided) tends to break them.
Letâs deal with definitions first, with an example â letâs look at a landing page with a channel:
In Search Console, these are reported as clicks, and can be vulnerable to heavy, invisible sampling when multiple dimensions (e.g. keyword and page) or filters are combined.
In Google Analytics, these are reported using last non-direct click, meaning that your organic traffic includes a bunch of direct sessions, time-outs that resumed mid-session, etc. Thatâs without getting into dark traffic, ad blockers, etc.
In AdWords, most reporting uses last AdWords click, and conversions may be defined differently. In addition, keyword volumes are bundled, as referenced above.
Rank tracking is location specific, and inconsistent, as referenced above.
Fine, though â it may not be precise, but you can at least get to some directionally useful data given these limitations. However, about that â(not provided)â...
Most of your landing pages get traffic from more than one keyword. Itâs very likely that some of these keywords convert better than others, particularly if they are branded, meaning that even the most thorough click-through rate model isnât going to help you. So how do you know which keywords are valuable?
The best answer is to generalize from AdWords data for those keywords, but itâs very unlikely that you have analytics data for all those combinations of keyword and landing page. Essentially, the tools that report on this make the very bold assumption that a given page converts identically for all keywords. Some are more transparent about this than others.
Again, this isnât to say that those tools arenât valuable â they just need to be understood carefully. The only way you could reliably fill in these blanks created by ânot providedâ would be to spend a ton on paid search to get decent volume, conversion rate, and bounce rate estimates for all your keywords, and even then, youâve not fixed the inconsistent definitions issues.
Bonus peeve: Average rank
I still see this way too often. Three questions:
Do you care more about losing rankings for ten very low volume queries (10 searches a month or less) than for one high volume query (millions plus)? If the answer isnât âyes, I absolutely care more about the ten low-volume queriesâ, then this metric isnât for you, and you should consider a visibility metric based on click through rate estimates.
When you start ranking at 100 for a keyword you didnât rank for before, does this make you unhappy? If the answer isnât âyes, I hate ranking for new keywords,â then this metric isnât for you â because that will lower your average rank. You could of course treat all non-ranking keywords as position 100, as some tools allow, but is a drop of 2 average rank positions really the best way to express that 1/50 of your landing pages have been de-indexed? Again, use a visibility metric, please.
Do you like comparing your performance with your competitors? If the answer isnât âno, of course not,â then this metric isnât for you â your competitors may have more or fewer branded keywords or long-tail rankings, and these will skew the comparison. Again, use a visibility metric.
Conclusion
Hopefully, youâve found this useful. To summarize the main takeaways:
Critically analyse correlations & case studies by seeing if you can explain them as coincidences, as reverse causation, as joint causation, through reference to a third mutually relevant factor, or through niche applicability.
Donât look at changes in traffic without looking at the context â what would you have forecasted for this period, and with what margin of error?
Remember that the tools we use have limitations, and do your research on how that impacts the numbers they show. âHow has this number been produced?â is an important component in âWhat does this number mean?â
If you end up combining data from multiple tools, remember to work out the relationship between them â treat this information as directional rather than precise.
Let me know what data analysis fallacies bug you, in the comments below.
Sign up for The Moz Top 10, a semimonthly mailer updating you on the top ten hottest pieces of SEO news, tips, and rad links uncovered by the Moz team. Think of it as your exclusive digest of stuff you don't have time to hunt down but want to read!
http://ift.tt/2nAc68g
0 notes
Text
Don't Be Fooled by Data: 4 Data Analysis Pitfalls & How to Avoid Them
Posted by Tom.Capper
Digital marketing is a proudly data-driven field. Yet, as SEOs especially, we often have such incomplete or questionable data to work with, that we end up jumping to the wrong conclusions in our attempts to substantiate our arguments or quantify our issues and opportunities.
In this post, Iâm going to outline 4 data analysis pitfalls that are endemic in our industry, and how to avoid them.
1. Jumping to conclusions
Earlier this year, I conducted a ranking factor study around brand awareness, and I posted this caveat:
"...the fact that Domain Authority (or branded search volume, or anything else) is positively correlated with rankings could indicate that any or all of the following is likely:
Links cause sites to rank well
Ranking well causes sites to get links
Some third factor (e.g. reputation or age of site) causes sites to get both links and rankings" ~ Me
However, I want to go into this in a bit more depth and give you a framework for analyzing these yourself, because it still comes up a lot. Take, for example, this recent study by Stone Temple, which you may have seen in the Moz Top 10 or Randâs tweets, or this excellent article discussing SEMRushâs recent direct traffic findings. To be absolutely clear, Iâm not criticizing either of the studies, but I do want to draw attention to how we might interpret them.
Firstly, we do tend to suffer a little confirmation bias â weâre all too eager to call out the clichĂ© âcorrelation vs. causationâ distinction when we see successful sites that are keyword-stuffed, but all too approving when we see studies doing the same with something we think is or was effective, like links.
Secondly, we fail to critically analyze the potential mechanisms. The options arenât just causation or coincidence.
Before you jump to a conclusion based on a correlation, youâre obliged to consider various possibilities:
Complete coincidence
Reverse causation
Joint causation
Linearity
Broad applicability
If those donât make any sense, then thatâs fair enough â theyâre jargon. Letâs go through an example:
Before I warn you not to eat cheese because you may die in your bedsheets, Iâm obliged to check that it isnât any of the following:
Complete coincidence - Is it possible that so many datasets were compared, that some were bound to be similar? Why, thatâs exactly what Tyler Vigen did! Yes, this is possible.
Reverse causation - Is it possible that we have this the wrong way around? For example, perhaps your relatives, in mourning for your bedsheet-related death, eat cheese in large quantities to comfort themselves? This seems pretty unlikely, so letâs give it a pass. No, this is very unlikely.
Joint causation - Is it possible that some third factor is behind both of these? Maybe increasing affluence makes you healthier (so you donât die of things like malnutrition), and also causes you to eat more cheese? This seems very plausible. Yes, this is possible.
Linearity - Are we comparing two linear trends? A linear trend is a steady rate of growth or decline. Any two statistics which are both roughly linear over time will be very well correlated. In the graph above, both our statistics are trending linearly upwards. If the graph was drawn with different scales, they might look completely unrelated, like this, but because they both have a steady rate, theyâd still be very well correlated. Yes, this looks likely.
Broad applicability - Is it possible that this relationship only exists in certain niche scenarios, or, at least, not in my niche scenario? Perhaps, for example, cheese does this to some people, and thatâs been enough to create this correlation, because there are so few bedsheet-tangling fatalities otherwise? Yes, this seems possible.
So we have 4 âYesâ answers and one âNoâ answer from those 5 checks.
If your example doesnât get 5 âNoâ answers from those 5 checks, itâs a fail, and you donât get to say that the study has established either a ranking factor or a fatal side effect of cheese consumption.
A similar process should apply to case studies, which are another form of correlation â the correlation between you making a change, and something good (or bad!) happening. For example, ask:
Have I ruled out other factors (e.g. external demand, seasonality, competitors making mistakes)?
Did I increase traffic by doing the thing I tried to do, or did I accidentally improve some other factor at the same time?
Did this work because of the unique circumstance of the particular client/project?
This is particularly challenging for SEOs, because we rarely have data of this quality, but Iâd suggest an additional pair of questions to help you navigate this minefield:
If I were Google, would I do this?
If I were Google, could I do this?
Direct traffic as a ranking factor passes the âcouldâ test, but only barely â Google could use data from Chrome, Android, or ISPs, but itâd be sketchy. It doesnât really pass the âwouldâ test, though â itâd be far easier for Google to use branded search traffic, which would answer the same questions you might try to answer by comparing direct traffic levels (e.g. how popular is this website?).
2. Missing the context
If I told you that my traffic was up 20% week on week today, what would you say? Congratulations?
What if it was up 20% this time last year?
What if I told you it had been up 20% year on year, up until recently?
Itâs funny how a little context can completely change this. This is another problem with case studies and their evil inverted twin, traffic drop analyses.
If we really want to understand whether to be surprised at something, positively or negatively, we need to compare it to our expectations, and then figure out what deviation from our expectations is ânormal.â If this is starting to sound like statistics, thatâs because it is statistics â indeed, I wrote about a statistical approach to measuring change way back in 2015.
If you want to be lazy, though, a good rule of thumb is to zoom out, and add in those previous years. And if someone shows you data that is suspiciously zoomed in, you might want to take it with a pinch of salt.
3. Trusting our tools
Would you make a multi-million dollar business decision based on a number that your competitor could manipulate at will? Well, chances are you do, and the number can be found in Google Analytics. Iâve covered this extensively in other places, but there are some major problems with most analytics platforms around:
How easy they are to manipulate externally
How arbitrarily they group hits into sessions
How vulnerable they are to ad blockers
How they perform under sampling, and how obvious they make this
For example, did you know that the Google Analytics API v3 can heavily sample data whilst telling you that the data is unsampled, above a certain amount of traffic (~500,000 within date range)? Neither did I, until we ran into it whilst building Distilled ODN.
Similar problems exist with many âSearch Analyticsâ tools. My colleague Sam Nemzer has written a bunch about this â did you know that most rank tracking platforms report completely different rankings? Or how about the fact that the keywords grouped by Google (and thus tools like SEMRush and STAT, too) are not equivalent, and donât necessarily have the volumes quoted?
Itâs important to understand the strengths and weaknesses of tools that we use, so that we can at least know when theyâre directionally accurate (as in, their insights guide you in the right direction), even if not perfectly accurate. All I can really recommend here is that skilling up in SEO (or any other digital channel) necessarily means understanding the mechanics behind your measurement platforms â which is why all new starts at Distilled end up learning how to do analytics audits.
One of the most common solutions to the root problem is combining multiple data sources, butâŠ
4. Combining data sources
There are numerous platforms out there that will âdefeat (not provided)â by bringing together data from two or more of:
Analytics
Search Console
AdWords
Rank tracking
The problems here are that, firstly, these platforms do not have equivalent definitions, and secondly, ironically, (not provided) tends to break them.
Letâs deal with definitions first, with an example â letâs look at a landing page with a channel:
In Search Console, these are reported as clicks, and can be vulnerable to heavy, invisible sampling when multiple dimensions (e.g. keyword and page) or filters are combined.
In Google Analytics, these are reported using last non-direct click, meaning that your organic traffic includes a bunch of direct sessions, time-outs that resumed mid-session, etc. Thatâs without getting into dark traffic, ad blockers, etc.
In AdWords, most reporting uses last AdWords click, and conversions may be defined differently. In addition, keyword volumes are bundled, as referenced above.
Rank tracking is location specific, and inconsistent, as referenced above.
Fine, though â it may not be precise, but you can at least get to some directionally useful data given these limitations. However, about that â(not provided)â...
Most of your landing pages get traffic from more than one keyword. Itâs very likely that some of these keywords convert better than others, particularly if they are branded, meaning that even the most thorough click-through rate model isnât going to help you. So how do you know which keywords are valuable?
The best answer is to generalize from AdWords data for those keywords, but itâs very unlikely that you have analytics data for all those combinations of keyword and landing page. Essentially, the tools that report on this make the very bold assumption that a given page converts identically for all keywords. Some are more transparent about this than others.
Again, this isnât to say that those tools arenât valuable â they just need to be understood carefully. The only way you could reliably fill in these blanks created by ânot providedâ would be to spend a ton on paid search to get decent volume, conversion rate, and bounce rate estimates for all your keywords, and even then, youâve not fixed the inconsistent definitions issues.
Bonus peeve: Average rank
I still see this way too often. Three questions:
Do you care more about losing rankings for ten very low volume queries (10 searches a month or less) than for one high volume query (millions plus)? If the answer isnât âyes, I absolutely care more about the ten low-volume queriesâ, then this metric isnât for you, and you should consider a visibility metric based on click through rate estimates.
When you start ranking at 100 for a keyword you didnât rank for before, does this make you unhappy? If the answer isnât âyes, I hate ranking for new keywords,â then this metric isnât for you â because that will lower your average rank. You could of course treat all non-ranking keywords as position 100, as some tools allow, but is a drop of 2 average rank positions really the best way to express that 1/50 of your landing pages have been de-indexed? Again, use a visibility metric, please.
Do you like comparing your performance with your competitors? If the answer isnât âno, of course not,â then this metric isnât for you â your competitors may have more or fewer branded keywords or long-tail rankings, and these will skew the comparison. Again, use a visibility metric.
Conclusion
Hopefully, youâve found this useful. To summarize the main takeaways:
Critically analyse correlations & case studies by seeing if you can explain them as coincidences, as reverse causation, as joint causation, through reference to a third mutually relevant factor, or through niche applicability.
Donât look at changes in traffic without looking at the context â what would you have forecasted for this period, and with what margin of error?
Remember that the tools we use have limitations, and do your research on how that impacts the numbers they show. âHow has this number been produced?â is an important component in âWhat does this number mean?â
If you end up combining data from multiple tools, remember to work out the relationship between them â treat this information as directional rather than precise.
Let me know what data analysis fallacies bug you, in the comments below.
Sign up for The Moz Top 10, a semimonthly mailer updating you on the top ten hottest pieces of SEO news, tips, and rad links uncovered by the Moz team. Think of it as your exclusive digest of stuff you don't have time to hunt down but want to read!
http://ift.tt/2nAc68g
0 notes
Text
Don't Be Fooled by Data: 4 Data Analysis Pitfalls & How to Avoid Them
Posted by Tom.Capper
Digital marketing is a proudly data-driven field. Yet, as SEOs especially, we often have such incomplete or questionable data to work with, that we end up jumping to the wrong conclusions in our attempts to substantiate our arguments or quantify our issues and opportunities.
In this post, Iâm going to outline 4 data analysis pitfalls that are endemic in our industry, and how to avoid them.
1. Jumping to conclusions
Earlier this year, I conducted a ranking factor study around brand awareness, and I posted this caveat:
"...the fact that Domain Authority (or branded search volume, or anything else) is positively correlated with rankings could indicate that any or all of the following is likely:
Links cause sites to rank well
Ranking well causes sites to get links
Some third factor (e.g. reputation or age of site) causes sites to get both links and rankings" ~ Me
However, I want to go into this in a bit more depth and give you a framework for analyzing these yourself, because it still comes up a lot. Take, for example, this recent study by Stone Temple, which you may have seen in the Moz Top 10 or Randâs tweets, or this excellent article discussing SEMRushâs recent direct traffic findings. To be absolutely clear, Iâm not criticizing either of the studies, but I do want to draw attention to how we might interpret them.
Firstly, we do tend to suffer a little confirmation bias â weâre all too eager to call out the clichĂ© âcorrelation vs. causationâ distinction when we see successful sites that are keyword-stuffed, but all too approving when we see studies doing the same with something we think is or was effective, like links.
Secondly, we fail to critically analyze the potential mechanisms. The options arenât just causation or coincidence.
Before you jump to a conclusion based on a correlation, youâre obliged to consider various possibilities:
Complete coincidence
Reverse causation
Joint causation
Linearity
Broad applicability
If those donât make any sense, then thatâs fair enough â theyâre jargon. Letâs go through an example:
Before I warn you not to eat cheese because you may die in your bedsheets, Iâm obliged to check that it isnât any of the following:
Complete coincidence - Is it possible that so many datasets were compared, that some were bound to be similar? Why, thatâs exactly what Tyler Vigen did! Yes, this is possible.
Reverse causation - Is it possible that we have this the wrong way around? For example, perhaps your relatives, in mourning for your bedsheet-related death, eat cheese in large quantities to comfort themselves? This seems pretty unlikely, so letâs give it a pass. No, this is very unlikely.
Joint causation - Is it possible that some third factor is behind both of these? Maybe increasing affluence makes you healthier (so you donât die of things like malnutrition), and also causes you to eat more cheese? This seems very plausible. Yes, this is possible.
Linearity - Are we comparing two linear trends? A linear trend is a steady rate of growth or decline. Any two statistics which are both roughly linear over time will be very well correlated. In the graph above, both our statistics are trending linearly upwards. If the graph was drawn with different scales, they might look completely unrelated, like this, but because they both have a steady rate, theyâd still be very well correlated. Yes, this looks likely.
Broad applicability - Is it possible that this relationship only exists in certain niche scenarios, or, at least, not in my niche scenario? Perhaps, for example, cheese does this to some people, and thatâs been enough to create this correlation, because there are so few bedsheet-tangling fatalities otherwise? Yes, this seems possible.
So we have 4 âYesâ answers and one âNoâ answer from those 5 checks.
If your example doesnât get 5 âNoâ answers from those 5 checks, itâs a fail, and you donât get to say that the study has established either a ranking factor or a fatal side effect of cheese consumption.
A similar process should apply to case studies, which are another form of correlation â the correlation between you making a change, and something good (or bad!) happening. For example, ask:
Have I ruled out other factors (e.g. external demand, seasonality, competitors making mistakes)?
Did I increase traffic by doing the thing I tried to do, or did I accidentally improve some other factor at the same time?
Did this work because of the unique circumstance of the particular client/project?
This is particularly challenging for SEOs, because we rarely have data of this quality, but Iâd suggest an additional pair of questions to help you navigate this minefield:
If I were Google, would I do this?
If I were Google, could I do this?
Direct traffic as a ranking factor passes the âcouldâ test, but only barely â Google could use data from Chrome, Android, or ISPs, but itâd be sketchy. It doesnât really pass the âwouldâ test, though â itâd be far easier for Google to use branded search traffic, which would answer the same questions you might try to answer by comparing direct traffic levels (e.g. how popular is this website?).
2. Missing the context
If I told you that my traffic was up 20% week on week today, what would you say? Congratulations?
What if it was up 20% this time last year?
What if I told you it had been up 20% year on year, up until recently?
Itâs funny how a little context can completely change this. This is another problem with case studies and their evil inverted twin, traffic drop analyses.
If we really want to understand whether to be surprised at something, positively or negatively, we need to compare it to our expectations, and then figure out what deviation from our expectations is ânormal.â If this is starting to sound like statistics, thatâs because it is statistics â indeed, I wrote about a statistical approach to measuring change way back in 2015.
If you want to be lazy, though, a good rule of thumb is to zoom out, and add in those previous years. And if someone shows you data that is suspiciously zoomed in, you might want to take it with a pinch of salt.
3. Trusting our tools
Would you make a multi-million dollar business decision based on a number that your competitor could manipulate at will? Well, chances are you do, and the number can be found in Google Analytics. Iâve covered this extensively in other places, but there are some major problems with most analytics platforms around:
How easy they are to manipulate externally
How arbitrarily they group hits into sessions
How vulnerable they are to ad blockers
How they perform under sampling, and how obvious they make this
For example, did you know that the Google Analytics API v3 can heavily sample data whilst telling you that the data is unsampled, above a certain amount of traffic (~500,000 within date range)? Neither did I, until we ran into it whilst building Distilled ODN.
Similar problems exist with many âSearch Analyticsâ tools. My colleague Sam Nemzer has written a bunch about this â did you know that most rank tracking platforms report completely different rankings? Or how about the fact that the keywords grouped by Google (and thus tools like SEMRush and STAT, too) are not equivalent, and donât necessarily have the volumes quoted?
Itâs important to understand the strengths and weaknesses of tools that we use, so that we can at least know when theyâre directionally accurate (as in, their insights guide you in the right direction), even if not perfectly accurate. All I can really recommend here is that skilling up in SEO (or any other digital channel) necessarily means understanding the mechanics behind your measurement platforms â which is why all new starts at Distilled end up learning how to do analytics audits.
One of the most common solutions to the root problem is combining multiple data sources, butâŠ
4. Combining data sources
There are numerous platforms out there that will âdefeat (not provided)â by bringing together data from two or more of:
Analytics
Search Console
AdWords
Rank tracking
The problems here are that, firstly, these platforms do not have equivalent definitions, and secondly, ironically, (not provided) tends to break them.
Letâs deal with definitions first, with an example â letâs look at a landing page with a channel:
In Search Console, these are reported as clicks, and can be vulnerable to heavy, invisible sampling when multiple dimensions (e.g. keyword and page) or filters are combined.
In Google Analytics, these are reported using last non-direct click, meaning that your organic traffic includes a bunch of direct sessions, time-outs that resumed mid-session, etc. Thatâs without getting into dark traffic, ad blockers, etc.
In AdWords, most reporting uses last AdWords click, and conversions may be defined differently. In addition, keyword volumes are bundled, as referenced above.
Rank tracking is location specific, and inconsistent, as referenced above.
Fine, though â it may not be precise, but you can at least get to some directionally useful data given these limitations. However, about that â(not provided)â...
Most of your landing pages get traffic from more than one keyword. Itâs very likely that some of these keywords convert better than others, particularly if they are branded, meaning that even the most thorough click-through rate model isnât going to help you. So how do you know which keywords are valuable?
The best answer is to generalize from AdWords data for those keywords, but itâs very unlikely that you have analytics data for all those combinations of keyword and landing page. Essentially, the tools that report on this make the very bold assumption that a given page converts identically for all keywords. Some are more transparent about this than others.
Again, this isnât to say that those tools arenât valuable â they just need to be understood carefully. The only way you could reliably fill in these blanks created by ânot providedâ would be to spend a ton on paid search to get decent volume, conversion rate, and bounce rate estimates for all your keywords, and even then, youâve not fixed the inconsistent definitions issues.
Bonus peeve: Average rank
I still see this way too often. Three questions:
Do you care more about losing rankings for ten very low volume queries (10 searches a month or less) than for one high volume query (millions plus)? If the answer isnât âyes, I absolutely care more about the ten low-volume queriesâ, then this metric isnât for you, and you should consider a visibility metric based on click through rate estimates.
When you start ranking at 100 for a keyword you didnât rank for before, does this make you unhappy? If the answer isnât âyes, I hate ranking for new keywords,â then this metric isnât for you â because that will lower your average rank. You could of course treat all non-ranking keywords as position 100, as some tools allow, but is a drop of 2 average rank positions really the best way to express that 1/50 of your landing pages have been de-indexed? Again, use a visibility metric, please.
Do you like comparing your performance with your competitors? If the answer isnât âno, of course not,â then this metric isnât for you â your competitors may have more or fewer branded keywords or long-tail rankings, and these will skew the comparison. Again, use a visibility metric.
Conclusion
Hopefully, youâve found this useful. To summarize the main takeaways:
Critically analyse correlations & case studies by seeing if you can explain them as coincidences, as reverse causation, as joint causation, through reference to a third mutually relevant factor, or through niche applicability.
Donât look at changes in traffic without looking at the context â what would you have forecasted for this period, and with what margin of error?
Remember that the tools we use have limitations, and do your research on how that impacts the numbers they show. âHow has this number been produced?â is an important component in âWhat does this number mean?â
If you end up combining data from multiple tools, remember to work out the relationship between them â treat this information as directional rather than precise.
Let me know what data analysis fallacies bug you, in the comments below.
Sign up for The Moz Top 10, a semimonthly mailer updating you on the top ten hottest pieces of SEO news, tips, and rad links uncovered by the Moz team. Think of it as your exclusive digest of stuff you don't have time to hunt down but want to read!
http://ift.tt/2nAc68g
0 notes
Text
Don't Be Fooled by Data: 4 Data Analysis Pitfalls & How to Avoid Them
Posted by Tom.Capper
Digital marketing is a proudly data-driven field. Yet, as SEOs especially, we often have such incomplete or questionable data to work with, that we end up jumping to the wrong conclusions in our attempts to substantiate our arguments or quantify our issues and opportunities.
In this post, Iâm going to outline 4 data analysis pitfalls that are endemic in our industry, and how to avoid them.
1. Jumping to conclusions
Earlier this year, I conducted a ranking factor study around brand awareness, and I posted this caveat:
"...the fact that Domain Authority (or branded search volume, or anything else) is positively correlated with rankings could indicate that any or all of the following is likely:
Links cause sites to rank well
Ranking well causes sites to get links
Some third factor (e.g. reputation or age of site) causes sites to get both links and rankings" ~ Me
However, I want to go into this in a bit more depth and give you a framework for analyzing these yourself, because it still comes up a lot. Take, for example, this recent study by Stone Temple, which you may have seen in the Moz Top 10 or Randâs tweets, or this excellent article discussing SEMRushâs recent direct traffic findings. To be absolutely clear, Iâm not criticizing either of the studies, but I do want to draw attention to how we might interpret them.
Firstly, we do tend to suffer a little confirmation bias â weâre all too eager to call out the clichĂ© âcorrelation vs. causationâ distinction when we see successful sites that are keyword-stuffed, but all too approving when we see studies doing the same with something we think is or was effective, like links.
Secondly, we fail to critically analyze the potential mechanisms. The options arenât just causation or coincidence.
Before you jump to a conclusion based on a correlation, youâre obliged to consider various possibilities:
Complete coincidence
Reverse causation
Joint causation
Linearity
Broad applicability
If those donât make any sense, then thatâs fair enough â theyâre jargon. Letâs go through an example:
Before I warn you not to eat cheese because you may die in your bedsheets, Iâm obliged to check that it isnât any of the following:
Complete coincidence - Is it possible that so many datasets were compared, that some were bound to be similar? Why, thatâs exactly what Tyler Vigen did! Yes, this is possible.
Reverse causation - Is it possible that we have this the wrong way around? For example, perhaps your relatives, in mourning for your bedsheet-related death, eat cheese in large quantities to comfort themselves? This seems pretty unlikely, so letâs give it a pass. No, this is very unlikely.
Joint causation - Is it possible that some third factor is behind both of these? Maybe increasing affluence makes you healthier (so you donât die of things like malnutrition), and also causes you to eat more cheese? This seems very plausible. Yes, this is possible.
Linearity - Are we comparing two linear trends? A linear trend is a steady rate of growth or decline. Any two statistics which are both roughly linear over time will be very well correlated. In the graph above, both our statistics are trending linearly upwards. If the graph was drawn with different scales, they might look completely unrelated, like this, but because they both have a steady rate, theyâd still be very well correlated. Yes, this looks likely.
Broad applicability - Is it possible that this relationship only exists in certain niche scenarios, or, at least, not in my niche scenario? Perhaps, for example, cheese does this to some people, and thatâs been enough to create this correlation, because there are so few bedsheet-tangling fatalities otherwise? Yes, this seems possible.
So we have 4 âYesâ answers and one âNoâ answer from those 5 checks.
If your example doesnât get 5 âNoâ answers from those 5 checks, itâs a fail, and you donât get to say that the study has established either a ranking factor or a fatal side effect of cheese consumption.
A similar process should apply to case studies, which are another form of correlation â the correlation between you making a change, and something good (or bad!) happening. For example, ask:
Have I ruled out other factors (e.g. external demand, seasonality, competitors making mistakes)?
Did I increase traffic by doing the thing I tried to do, or did I accidentally improve some other factor at the same time?
Did this work because of the unique circumstance of the particular client/project?
This is particularly challenging for SEOs, because we rarely have data of this quality, but Iâd suggest an additional pair of questions to help you navigate this minefield:
If I were Google, would I do this?
If I were Google, could I do this?
Direct traffic as a ranking factor passes the âcouldâ test, but only barely â Google could use data from Chrome, Android, or ISPs, but itâd be sketchy. It doesnât really pass the âwouldâ test, though â itâd be far easier for Google to use branded search traffic, which would answer the same questions you might try to answer by comparing direct traffic levels (e.g. how popular is this website?).
2. Missing the context
If I told you that my traffic was up 20% week on week today, what would you say? Congratulations?
What if it was up 20% this time last year?
What if I told you it had been up 20% year on year, up until recently?
Itâs funny how a little context can completely change this. This is another problem with case studies and their evil inverted twin, traffic drop analyses.
If we really want to understand whether to be surprised at something, positively or negatively, we need to compare it to our expectations, and then figure out what deviation from our expectations is ânormal.â If this is starting to sound like statistics, thatâs because it is statistics â indeed, I wrote about a statistical approach to measuring change way back in 2015.
If you want to be lazy, though, a good rule of thumb is to zoom out, and add in those previous years. And if someone shows you data that is suspiciously zoomed in, you might want to take it with a pinch of salt.
3. Trusting our tools
Would you make a multi-million dollar business decision based on a number that your competitor could manipulate at will? Well, chances are you do, and the number can be found in Google Analytics. Iâve covered this extensively in other places, but there are some major problems with most analytics platforms around:
How easy they are to manipulate externally
How arbitrarily they group hits into sessions
How vulnerable they are to ad blockers
How they perform under sampling, and how obvious they make this
For example, did you know that the Google Analytics API v3 can heavily sample data whilst telling you that the data is unsampled, above a certain amount of traffic (~500,000 within date range)? Neither did I, until we ran into it whilst building Distilled ODN.
Similar problems exist with many âSearch Analyticsâ tools. My colleague Sam Nemzer has written a bunch about this â did you know that most rank tracking platforms report completely different rankings? Or how about the fact that the keywords grouped by Google (and thus tools like SEMRush and STAT, too) are not equivalent, and donât necessarily have the volumes quoted?
Itâs important to understand the strengths and weaknesses of tools that we use, so that we can at least know when theyâre directionally accurate (as in, their insights guide you in the right direction), even if not perfectly accurate. All I can really recommend here is that skilling up in SEO (or any other digital channel) necessarily means understanding the mechanics behind your measurement platforms â which is why all new starts at Distilled end up learning how to do analytics audits.
One of the most common solutions to the root problem is combining multiple data sources, butâŠ
4. Combining data sources
There are numerous platforms out there that will âdefeat (not provided)â by bringing together data from two or more of:
Analytics
Search Console
AdWords
Rank tracking
The problems here are that, firstly, these platforms do not have equivalent definitions, and secondly, ironically, (not provided) tends to break them.
Letâs deal with definitions first, with an example â letâs look at a landing page with a channel:
In Search Console, these are reported as clicks, and can be vulnerable to heavy, invisible sampling when multiple dimensions (e.g. keyword and page) or filters are combined.
In Google Analytics, these are reported using last non-direct click, meaning that your organic traffic includes a bunch of direct sessions, time-outs that resumed mid-session, etc. Thatâs without getting into dark traffic, ad blockers, etc.
In AdWords, most reporting uses last AdWords click, and conversions may be defined differently. In addition, keyword volumes are bundled, as referenced above.
Rank tracking is location specific, and inconsistent, as referenced above.
Fine, though â it may not be precise, but you can at least get to some directionally useful data given these limitations. However, about that â(not provided)â...
Most of your landing pages get traffic from more than one keyword. Itâs very likely that some of these keywords convert better than others, particularly if they are branded, meaning that even the most thorough click-through rate model isnât going to help you. So how do you know which keywords are valuable?
The best answer is to generalize from AdWords data for those keywords, but itâs very unlikely that you have analytics data for all those combinations of keyword and landing page. Essentially, the tools that report on this make the very bold assumption that a given page converts identically for all keywords. Some are more transparent about this than others.
Again, this isnât to say that those tools arenât valuable â they just need to be understood carefully. The only way you could reliably fill in these blanks created by ânot providedâ would be to spend a ton on paid search to get decent volume, conversion rate, and bounce rate estimates for all your keywords, and even then, youâve not fixed the inconsistent definitions issues.
Bonus peeve: Average rank
I still see this way too often. Three questions:
Do you care more about losing rankings for ten very low volume queries (10 searches a month or less) than for one high volume query (millions plus)? If the answer isnât âyes, I absolutely care more about the ten low-volume queriesâ, then this metric isnât for you, and you should consider a visibility metric based on click through rate estimates.
When you start ranking at 100 for a keyword you didnât rank for before, does this make you unhappy? If the answer isnât âyes, I hate ranking for new keywords,â then this metric isnât for you â because that will lower your average rank. You could of course treat all non-ranking keywords as position 100, as some tools allow, but is a drop of 2 average rank positions really the best way to express that 1/50 of your landing pages have been de-indexed? Again, use a visibility metric, please.
Do you like comparing your performance with your competitors? If the answer isnât âno, of course not,â then this metric isnât for you â your competitors may have more or fewer branded keywords or long-tail rankings, and these will skew the comparison. Again, use a visibility metric.
Conclusion
Hopefully, youâve found this useful. To summarize the main takeaways:
Critically analyse correlations & case studies by seeing if you can explain them as coincidences, as reverse causation, as joint causation, through reference to a third mutually relevant factor, or through niche applicability.
Donât look at changes in traffic without looking at the context â what would you have forecasted for this period, and with what margin of error?
Remember that the tools we use have limitations, and do your research on how that impacts the numbers they show. âHow has this number been produced?â is an important component in âWhat does this number mean?â
If you end up combining data from multiple tools, remember to work out the relationship between them â treat this information as directional rather than precise.
Let me know what data analysis fallacies bug you, in the comments below.
Sign up for The Moz Top 10, a semimonthly mailer updating you on the top ten hottest pieces of SEO news, tips, and rad links uncovered by the Moz team. Think of it as your exclusive digest of stuff you don't have time to hunt down but want to read!
http://ift.tt/2nAc68g
0 notes
Text
Don't Be Fooled by Data: 4 Data Analysis Pitfalls & How to Avoid Them
Posted by Tom.Capper
Digital marketing is a proudly data-driven field. Yet, as SEOs especially, we often have such incomplete or questionable data to work with, that we end up jumping to the wrong conclusions in our attempts to substantiate our arguments or quantify our issues and opportunities.
In this post, Iâm going to outline 4 data analysis pitfalls that are endemic in our industry, and how to avoid them.
1. Jumping to conclusions
Earlier this year, I conducted a ranking factor study around brand awareness, and I posted this caveat:
"...the fact that Domain Authority (or branded search volume, or anything else) is positively correlated with rankings could indicate that any or all of the following is likely:
Links cause sites to rank well
Ranking well causes sites to get links
Some third factor (e.g. reputation or age of site) causes sites to get both links and rankings" ~ Me
However, I want to go into this in a bit more depth and give you a framework for analyzing these yourself, because it still comes up a lot. Take, for example, this recent study by Stone Temple, which you may have seen in the Moz Top 10 or Randâs tweets, or this excellent article discussing SEMRushâs recent direct traffic findings. To be absolutely clear, Iâm not criticizing either of the studies, but I do want to draw attention to how we might interpret them.
Firstly, we do tend to suffer a little confirmation bias â weâre all too eager to call out the clichĂ© âcorrelation vs. causationâ distinction when we see successful sites that are keyword-stuffed, but all too approving when we see studies doing the same with something we think is or was effective, like links.
Secondly, we fail to critically analyze the potential mechanisms. The options arenât just causation or coincidence.
Before you jump to a conclusion based on a correlation, youâre obliged to consider various possibilities:
Complete coincidence
Reverse causation
Joint causation
Linearity
Broad applicability
If those donât make any sense, then thatâs fair enough â theyâre jargon. Letâs go through an example:
Before I warn you not to eat cheese because you may die in your bedsheets, Iâm obliged to check that it isnât any of the following:
Complete coincidence - Is it possible that so many datasets were compared, that some were bound to be similar? Why, thatâs exactly what Tyler Vigen did! Yes, this is possible.
Reverse causation - Is it possible that we have this the wrong way around? For example, perhaps your relatives, in mourning for your bedsheet-related death, eat cheese in large quantities to comfort themselves? This seems pretty unlikely, so letâs give it a pass. No, this is very unlikely.
Joint causation - Is it possible that some third factor is behind both of these? Maybe increasing affluence makes you healthier (so you donât die of things like malnutrition), and also causes you to eat more cheese? This seems very plausible. Yes, this is possible.
Linearity - Are we comparing two linear trends? A linear trend is a steady rate of growth or decline. Any two statistics which are both roughly linear over time will be very well correlated. In the graph above, both our statistics are trending linearly upwards. If the graph was drawn with different scales, they might look completely unrelated, like this, but because they both have a steady rate, theyâd still be very well correlated. Yes, this looks likely.
Broad applicability - Is it possible that this relationship only exists in certain niche scenarios, or, at least, not in my niche scenario? Perhaps, for example, cheese does this to some people, and thatâs been enough to create this correlation, because there are so few bedsheet-tangling fatalities otherwise? Yes, this seems possible.
So we have 4 âYesâ answers and one âNoâ answer from those 5 checks.
If your example doesnât get 5 âNoâ answers from those 5 checks, itâs a fail, and you donât get to say that the study has established either a ranking factor or a fatal side effect of cheese consumption.
A similar process should apply to case studies, which are another form of correlation â the correlation between you making a change, and something good (or bad!) happening. For example, ask:
Have I ruled out other factors (e.g. external demand, seasonality, competitors making mistakes)?
Did I increase traffic by doing the thing I tried to do, or did I accidentally improve some other factor at the same time?
Did this work because of the unique circumstance of the particular client/project?
This is particularly challenging for SEOs, because we rarely have data of this quality, but Iâd suggest an additional pair of questions to help you navigate this minefield:
If I were Google, would I do this?
If I were Google, could I do this?
Direct traffic as a ranking factor passes the âcouldâ test, but only barely â Google could use data from Chrome, Android, or ISPs, but itâd be sketchy. It doesnât really pass the âwouldâ test, though â itâd be far easier for Google to use branded search traffic, which would answer the same questions you might try to answer by comparing direct traffic levels (e.g. how popular is this website?).
2. Missing the context
If I told you that my traffic was up 20% week on week today, what would you say? Congratulations?
What if it was up 20% this time last year?
What if I told you it had been up 20% year on year, up until recently?
Itâs funny how a little context can completely change this. This is another problem with case studies and their evil inverted twin, traffic drop analyses.
If we really want to understand whether to be surprised at something, positively or negatively, we need to compare it to our expectations, and then figure out what deviation from our expectations is ânormal.â If this is starting to sound like statistics, thatâs because it is statistics â indeed, I wrote about a statistical approach to measuring change way back in 2015.
If you want to be lazy, though, a good rule of thumb is to zoom out, and add in those previous years. And if someone shows you data that is suspiciously zoomed in, you might want to take it with a pinch of salt.
3. Trusting our tools
Would you make a multi-million dollar business decision based on a number that your competitor could manipulate at will? Well, chances are you do, and the number can be found in Google Analytics. Iâve covered this extensively in other places, but there are some major problems with most analytics platforms around:
How easy they are to manipulate externally
How arbitrarily they group hits into sessions
How vulnerable they are to ad blockers
How they perform under sampling, and how obvious they make this
For example, did you know that the Google Analytics API v3 can heavily sample data whilst telling you that the data is unsampled, above a certain amount of traffic (~500,000 within date range)? Neither did I, until we ran into it whilst building Distilled ODN.
Similar problems exist with many âSearch Analyticsâ tools. My colleague Sam Nemzer has written a bunch about this â did you know that most rank tracking platforms report completely different rankings? Or how about the fact that the keywords grouped by Google (and thus tools like SEMRush and STAT, too) are not equivalent, and donât necessarily have the volumes quoted?
Itâs important to understand the strengths and weaknesses of tools that we use, so that we can at least know when theyâre directionally accurate (as in, their insights guide you in the right direction), even if not perfectly accurate. All I can really recommend here is that skilling up in SEO (or any other digital channel) necessarily means understanding the mechanics behind your measurement platforms â which is why all new starts at Distilled end up learning how to do analytics audits.
One of the most common solutions to the root problem is combining multiple data sources, butâŠ
4. Combining data sources
There are numerous platforms out there that will âdefeat (not provided)â by bringing together data from two or more of:
Analytics
Search Console
AdWords
Rank tracking
The problems here are that, firstly, these platforms do not have equivalent definitions, and secondly, ironically, (not provided) tends to break them.
Letâs deal with definitions first, with an example â letâs look at a landing page with a channel:
In Search Console, these are reported as clicks, and can be vulnerable to heavy, invisible sampling when multiple dimensions (e.g. keyword and page) or filters are combined.
In Google Analytics, these are reported using last non-direct click, meaning that your organic traffic includes a bunch of direct sessions, time-outs that resumed mid-session, etc. Thatâs without getting into dark traffic, ad blockers, etc.
In AdWords, most reporting uses last AdWords click, and conversions may be defined differently. In addition, keyword volumes are bundled, as referenced above.
Rank tracking is location specific, and inconsistent, as referenced above.
Fine, though â it may not be precise, but you can at least get to some directionally useful data given these limitations. However, about that â(not provided)â...
Most of your landing pages get traffic from more than one keyword. Itâs very likely that some of these keywords convert better than others, particularly if they are branded, meaning that even the most thorough click-through rate model isnât going to help you. So how do you know which keywords are valuable?
The best answer is to generalize from AdWords data for those keywords, but itâs very unlikely that you have analytics data for all those combinations of keyword and landing page. Essentially, the tools that report on this make the very bold assumption that a given page converts identically for all keywords. Some are more transparent about this than others.
Again, this isnât to say that those tools arenât valuable â they just need to be understood carefully. The only way you could reliably fill in these blanks created by ânot providedâ would be to spend a ton on paid search to get decent volume, conversion rate, and bounce rate estimates for all your keywords, and even then, youâve not fixed the inconsistent definitions issues.
Bonus peeve: Average rank
I still see this way too often. Three questions:
Do you care more about losing rankings for ten very low volume queries (10 searches a month or less) than for one high volume query (millions plus)? If the answer isnât âyes, I absolutely care more about the ten low-volume queriesâ, then this metric isnât for you, and you should consider a visibility metric based on click through rate estimates.
When you start ranking at 100 for a keyword you didnât rank for before, does this make you unhappy? If the answer isnât âyes, I hate ranking for new keywords,â then this metric isnât for you â because that will lower your average rank. You could of course treat all non-ranking keywords as position 100, as some tools allow, but is a drop of 2 average rank positions really the best way to express that 1/50 of your landing pages have been de-indexed? Again, use a visibility metric, please.
Do you like comparing your performance with your competitors? If the answer isnât âno, of course not,â then this metric isnât for you â your competitors may have more or fewer branded keywords or long-tail rankings, and these will skew the comparison. Again, use a visibility metric.
Conclusion
Hopefully, youâve found this useful. To summarize the main takeaways:
Critically analyse correlations & case studies by seeing if you can explain them as coincidences, as reverse causation, as joint causation, through reference to a third mutually relevant factor, or through niche applicability.
Donât look at changes in traffic without looking at the context â what would you have forecasted for this period, and with what margin of error?
Remember that the tools we use have limitations, and do your research on how that impacts the numbers they show. âHow has this number been produced?â is an important component in âWhat does this number mean?â
If you end up combining data from multiple tools, remember to work out the relationship between them â treat this information as directional rather than precise.
Let me know what data analysis fallacies bug you, in the comments below.
Sign up for The Moz Top 10, a semimonthly mailer updating you on the top ten hottest pieces of SEO news, tips, and rad links uncovered by the Moz team. Think of it as your exclusive digest of stuff you don't have time to hunt down but want to read!
http://ift.tt/2nAc68g
0 notes
Text
Don't Be Fooled by Data: 4 Data Analysis Pitfalls & How to Avoid Them
Posted by Tom.Capper
Digital marketing is a proudly data-driven field. Yet, as SEOs especially, we often have such incomplete or questionable data to work with, that we end up jumping to the wrong conclusions in our attempts to substantiate our arguments or quantify our issues and opportunities.
In this post, Iâm going to outline 4 data analysis pitfalls that are endemic in our industry, and how to avoid them.
1. Jumping to conclusions
Earlier this year, I conducted a ranking factor study around brand awareness, and I posted this caveat:
"...the fact that Domain Authority (or branded search volume, or anything else) is positively correlated with rankings could indicate that any or all of the following is likely:
Links cause sites to rank well
Ranking well causes sites to get links
Some third factor (e.g. reputation or age of site) causes sites to get both links and rankings" ~ Me
However, I want to go into this in a bit more depth and give you a framework for analyzing these yourself, because it still comes up a lot. Take, for example, this recent study by Stone Temple, which you may have seen in the Moz Top 10 or Randâs tweets, or this excellent article discussing SEMRushâs recent direct traffic findings. To be absolutely clear, Iâm not criticizing either of the studies, but I do want to draw attention to how we might interpret them.
Firstly, we do tend to suffer a little confirmation bias â weâre all too eager to call out the clichĂ© âcorrelation vs. causationâ distinction when we see successful sites that are keyword-stuffed, but all too approving when we see studies doing the same with something we think is or was effective, like links.
Secondly, we fail to critically analyze the potential mechanisms. The options arenât just causation or coincidence.
Before you jump to a conclusion based on a correlation, youâre obliged to consider various possibilities:
Complete coincidence
Reverse causation
Joint causation
Linearity
Broad applicability
If those donât make any sense, then thatâs fair enough â theyâre jargon. Letâs go through an example:
Before I warn you not to eat cheese because you may die in your bedsheets, Iâm obliged to check that it isnât any of the following:
Complete coincidence - Is it possible that so many datasets were compared, that some were bound to be similar? Why, thatâs exactly what Tyler Vigen did! Yes, this is possible.
Reverse causation - Is it possible that we have this the wrong way around? For example, perhaps your relatives, in mourning for your bedsheet-related death, eat cheese in large quantities to comfort themselves? This seems pretty unlikely, so letâs give it a pass. No, this is very unlikely.
Joint causation - Is it possible that some third factor is behind both of these? Maybe increasing affluence makes you healthier (so you donât die of things like malnutrition), and also causes you to eat more cheese? This seems very plausible. Yes, this is possible.
Linearity - Are we comparing two linear trends? A linear trend is a steady rate of growth or decline. Any two statistics which are both roughly linear over time will be very well correlated. In the graph above, both our statistics are trending linearly upwards. If the graph was drawn with different scales, they might look completely unrelated, like this, but because they both have a steady rate, theyâd still be very well correlated. Yes, this looks likely.
Broad applicability - Is it possible that this relationship only exists in certain niche scenarios, or, at least, not in my niche scenario? Perhaps, for example, cheese does this to some people, and thatâs been enough to create this correlation, because there are so few bedsheet-tangling fatalities otherwise? Yes, this seems possible.
So we have 4 âYesâ answers and one âNoâ answer from those 5 checks.
If your example doesnât get 5 âNoâ answers from those 5 checks, itâs a fail, and you donât get to say that the study has established either a ranking factor or a fatal side effect of cheese consumption.
A similar process should apply to case studies, which are another form of correlation â the correlation between you making a change, and something good (or bad!) happening. For example, ask:
Have I ruled out other factors (e.g. external demand, seasonality, competitors making mistakes)?
Did I increase traffic by doing the thing I tried to do, or did I accidentally improve some other factor at the same time?
Did this work because of the unique circumstance of the particular client/project?
This is particularly challenging for SEOs, because we rarely have data of this quality, but Iâd suggest an additional pair of questions to help you navigate this minefield:
If I were Google, would I do this?
If I were Google, could I do this?
Direct traffic as a ranking factor passes the âcouldâ test, but only barely â Google could use data from Chrome, Android, or ISPs, but itâd be sketchy. It doesnât really pass the âwouldâ test, though â itâd be far easier for Google to use branded search traffic, which would answer the same questions you might try to answer by comparing direct traffic levels (e.g. how popular is this website?).
2. Missing the context
If I told you that my traffic was up 20% week on week today, what would you say? Congratulations?
What if it was up 20% this time last year?
What if I told you it had been up 20% year on year, up until recently?
Itâs funny how a little context can completely change this. This is another problem with case studies and their evil inverted twin, traffic drop analyses.
If we really want to understand whether to be surprised at something, positively or negatively, we need to compare it to our expectations, and then figure out what deviation from our expectations is ânormal.â If this is starting to sound like statistics, thatâs because it is statistics â indeed, I wrote about a statistical approach to measuring change way back in 2015.
If you want to be lazy, though, a good rule of thumb is to zoom out, and add in those previous years. And if someone shows you data that is suspiciously zoomed in, you might want to take it with a pinch of salt.
3. Trusting our tools
Would you make a multi-million dollar business decision based on a number that your competitor could manipulate at will? Well, chances are you do, and the number can be found in Google Analytics. Iâve covered this extensively in other places, but there are some major problems with most analytics platforms around:
How easy they are to manipulate externally
How arbitrarily they group hits into sessions
How vulnerable they are to ad blockers
How they perform under sampling, and how obvious they make this
For example, did you know that the Google Analytics API v3 can heavily sample data whilst telling you that the data is unsampled, above a certain amount of traffic (~500,000 within date range)? Neither did I, until we ran into it whilst building Distilled ODN.
Similar problems exist with many âSearch Analyticsâ tools. My colleague Sam Nemzer has written a bunch about this â did you know that most rank tracking platforms report completely different rankings? Or how about the fact that the keywords grouped by Google (and thus tools like SEMRush and STAT, too) are not equivalent, and donât necessarily have the volumes quoted?
Itâs important to understand the strengths and weaknesses of tools that we use, so that we can at least know when theyâre directionally accurate (as in, their insights guide you in the right direction), even if not perfectly accurate. All I can really recommend here is that skilling up in SEO (or any other digital channel) necessarily means understanding the mechanics behind your measurement platforms â which is why all new starts at Distilled end up learning how to do analytics audits.
One of the most common solutions to the root problem is combining multiple data sources, butâŠ
4. Combining data sources
There are numerous platforms out there that will âdefeat (not provided)â by bringing together data from two or more of:
Analytics
Search Console
AdWords
Rank tracking
The problems here are that, firstly, these platforms do not have equivalent definitions, and secondly, ironically, (not provided) tends to break them.
Letâs deal with definitions first, with an example â letâs look at a landing page with a channel:
In Search Console, these are reported as clicks, and can be vulnerable to heavy, invisible sampling when multiple dimensions (e.g. keyword and page) or filters are combined.
In Google Analytics, these are reported using last non-direct click, meaning that your organic traffic includes a bunch of direct sessions, time-outs that resumed mid-session, etc. Thatâs without getting into dark traffic, ad blockers, etc.
In AdWords, most reporting uses last AdWords click, and conversions may be defined differently. In addition, keyword volumes are bundled, as referenced above.
Rank tracking is location specific, and inconsistent, as referenced above.
Fine, though â it may not be precise, but you can at least get to some directionally useful data given these limitations. However, about that â(not provided)â...
Most of your landing pages get traffic from more than one keyword. Itâs very likely that some of these keywords convert better than others, particularly if they are branded, meaning that even the most thorough click-through rate model isnât going to help you. So how do you know which keywords are valuable?
The best answer is to generalize from AdWords data for those keywords, but itâs very unlikely that you have analytics data for all those combinations of keyword and landing page. Essentially, the tools that report on this make the very bold assumption that a given page converts identically for all keywords. Some are more transparent about this than others.
Again, this isnât to say that those tools arenât valuable â they just need to be understood carefully. The only way you could reliably fill in these blanks created by ânot providedâ would be to spend a ton on paid search to get decent volume, conversion rate, and bounce rate estimates for all your keywords, and even then, youâve not fixed the inconsistent definitions issues.
Bonus peeve: Average rank
I still see this way too often. Three questions:
Do you care more about losing rankings for ten very low volume queries (10 searches a month or less) than for one high volume query (millions plus)? If the answer isnât âyes, I absolutely care more about the ten low-volume queriesâ, then this metric isnât for you, and you should consider a visibility metric based on click through rate estimates.
When you start ranking at 100 for a keyword you didnât rank for before, does this make you unhappy? If the answer isnât âyes, I hate ranking for new keywords,â then this metric isnât for you â because that will lower your average rank. You could of course treat all non-ranking keywords as position 100, as some tools allow, but is a drop of 2 average rank positions really the best way to express that 1/50 of your landing pages have been de-indexed? Again, use a visibility metric, please.
Do you like comparing your performance with your competitors? If the answer isnât âno, of course not,â then this metric isnât for you â your competitors may have more or fewer branded keywords or long-tail rankings, and these will skew the comparison. Again, use a visibility metric.
Conclusion
Hopefully, youâve found this useful. To summarize the main takeaways:
Critically analyse correlations & case studies by seeing if you can explain them as coincidences, as reverse causation, as joint causation, through reference to a third mutually relevant factor, or through niche applicability.
Donât look at changes in traffic without looking at the context â what would you have forecasted for this period, and with what margin of error?
Remember that the tools we use have limitations, and do your research on how that impacts the numbers they show. âHow has this number been produced?â is an important component in âWhat does this number mean?â
If you end up combining data from multiple tools, remember to work out the relationship between them â treat this information as directional rather than precise.
Let me know what data analysis fallacies bug you, in the comments below.
Sign up for The Moz Top 10, a semimonthly mailer updating you on the top ten hottest pieces of SEO news, tips, and rad links uncovered by the Moz team. Think of it as your exclusive digest of stuff you don't have time to hunt down but want to read!
http://ift.tt/2nAc68g
0 notes
Text
Larry Cuban on School Reform and Classroom Practice: Donors Reform Schooling: Evaluating Teachers (Part 2)
Larry Cuban on School Reform and Classroom Practice: Donors Reform Schooling: Evaluating Teachers (Part 2)
In Part 1, I described a Gates Foundation initiative aimed at identifying effective teachers as measured in part by their studentsâ test scores, rewarding such stellar teachers with cash, and giving poor and minority children access to their classrooms. Called Institute Program for Effective Teaching, the Foundation had mobilized sufficient political support for the huge grant to find and fund three school districts and four charter school networks across the nation. IPET launched in 2009 and closed it doors (and funding) in 2016.
A brief look at the largest partner in the project, Floridaâs Hillsborough County district, over the span of the grant gives a peek at how early exhilaration over the project morphed into opposition over rising program costs that had to be absorbed by the districtâs regular budget, and then key district and school staffâs growing disillusion over the projectâs direction and disappointing results for students. Consider what the Tampa Bay Times, a local paper, found in 2015 after a lengthy investigation into the grant. [i]
The Gates-funded program â which required Hillsborough to raise its own $100 million â ballooned beyond the districtâs ability to afford it, creating a new bureaucracy of mentors and âpeer evaluatorsâ who no longer work with students.
Nearly 3,000 employees got one-year raises of more than $8,000. Some were as high as $15,000, or 25 percent.
Raises went to a wider group than envisioned, including close to 500 people who donât work in the classroom full time, if at all.
The greatest share of large raises went to veteran teachers in stable suburban schools, despite the programâs stated goal of channeling better and better-paid teachers into high-needs schools.
More than $23 million of the Gates money went to consultants.
The programâs total cost has risen from $202 million to $271 million when related projects are factored in, with some of the money coming from private foundations in addition to Gates. The districtâs share now comes to $124 million.
Millions of dollars were pledged to parts of the program that educators now doubt. After investing in an elaborate system of peer evaluations to improve teaching, district leaders are considering a retreat from that model. And Gates is withholding $20 million after deciding it does not, after all, favor the idea of teacher performance bonuses â a major change in philosophy.
The end product â results in the classroom â is a mixed bag.
Hillsboroughâs graduation rate still lags behind other large school districts. Racial and economic achievement gaps remain pronounced, especially in middle school.
And poor schools still wind up with the newest, greenest teachers.
Not a pretty picture. RANDâs formal evaluation covering the life of the grant and across the three districts and four charter networks used less judgmental language but reached a similar conclusion on school outcomes that the Tampa Bay Times had for these county schools.
Overall, the initiative did not achieve its stated goals for students, particularly LIM [low-income minority students. By the end of 2014â2015, student outcomes were not dramatically better than outcomes in similar sites that did not participate in the IP initiative. Furthermore, in the sites where these analyses could be conducted, we did not find improvement in the effectiveness of newly hired teachers relative to experienced teachers; we found very few instances of improvement in the effectiveness of the teaching force overall; we found no evidence that LIM students had greater access than non-LIM students to effective teaching; and we found no increase in the retention of effective teachers, although we did find declines in the retention of ineffective teachers in most sites. [ii]
As with the history of such innovative projects in public schools over the past century, RAND evaluators found that districts and charter school networks fell short in achieving IPET because of uneven and incomplete implementation of the program.
We also examined variation in implementation and outcomes across sites. Although sites varied in context and in the ways in which they approached the levers, these differences did not translate into differences in ultimate outcomes. Although the sites implemented the same levers, they gave different degrees of emphasis to different levers, and none of the sites achieved strong implementation or outcomes across the board. [iii]
But the absolutist judgment of âfailureâ in achieving aims of this donor-funded initiative hides the rippling effects of this effort to reform teaching and learning in these districts and charter networks. For example, during the Obama administration, U.S. Secretary of Education Arne Duncanâs initiative of Race to the Top invited states to compete for grants of millions of dollars if they committed themselves to the Common Core standardsâanother Gates-funded initiativeâand included, as did IPET, different ways of evaluating teachers. [iv]
Now over 40 states and the District of Columbia have adopted plans to evaluate teachers on the basis of student test scores. How much student test scores should weigh in the overall determination of a teacherâs effectiveness varies by state and local districts as does the autonomy local districts have in putting their signature on state requirements in evaluating teachers. For example, from half of the total judgment of the teacher to one-third or one-fourth, test scores have become a significant variable in assessing a teacherâs effectiveness. Even as testing experts and academic evaluators have raised significant flags about the instability, inaccuracy, and unfairness of such district and state evaluation policies based upon student scores being put into practice, they remain on the books and have been implemented in various districts. Because the amount of time is such an important factor in putting these policies into practice, states will go through trial and error as they implement these policies possibly leading to more (or less) political acceptance from teachers and principals, key participants in the venture.[v]
While there has been a noticeable dulling of the reform glow for evaluating teachers on the basis of student performanceânote the Gates Foundation pulling back on their use in evaluating teachers as part of the half-billion dollar Intensive Partnerships for Effective Teachingâthe rise and fall in enthusiasm in using test scores, intentionally or unintentionally, has focused policy discussions on teachers as the source of school âfailureâ and inequalities among students. In pressing for teachers to be held accountable, policy elites have largely ignored other factors that influence both teacher and student performance that are deeply connected to economic and social inequalities outside the school such as poverty, neighborhood crime, discriminatory labor and housing practices, and lack of access to health centers.
By donors helping to frame an agenda for turning around âfailingâ U.S. schools or, more generously, improving equal opportunity for children and youth, these philanthropists âunaccountable to anyone and receiving tax subsidies from the federal governmentâas members of policy elites spotlight teachers as both the problem and solution to school improvement. Surely, teachers are the most important in-school factorâperhaps 10 percent of the variation in student achievement. Yet over 60 percent of the variation in student academic performance is attributed to out-of-school factors such as the family. [vi]
This Gates-funded Intensive Partnerships for Effective Teaching is an example, then, of policy elites shaping a reform agenda for the nationâs schools using teacher effectiveness as a primary criterion and having enormous direct and indirect influence in advocating and enacting other pet reforms.
Did, then, Intensive Partnerships for Effective Teaching âfail?â Part 3 answers that question.
__________________________
[i]Â (Marlene Sokol, âSticker Shock: How Hillsborough Countyâs Gates Grant Became a Budget Buster,â October 23, 2015 )
[ii]Â RAND evaluation; implementation quote, p. 488.
[iii] William Howell, âResults of President Obamaâs Race to the Top,â Education Next, 2015, 15 (4), at: https://www.educationnext.org/results-president-obama-race-to-the-top-reform/
[iv] ibid.
[v] Eduardo Porter, âGrading Teachers by the Test,â New York Times, March 24, 2015; Rachel Cohen, âTeachers Tests Test Teachers,â American Prospect, July 18, 2017; Kaitlin Pennington and Sara Mead, For Good Measure? Teacher Evaluation Policy in the ESSA Era, Bellwether Education Partners, December 2016; Edward Haertel, âReliability and Validity of Inferences about Teachers Based on Student Test Scores,â William Angoff Memorial Lecture, Washington D.C., March 22, 2013; Matthew Di Carlo, âWhy Teacher evaluation Reform Is Not a Failure,â August 23, 2018 at: http://www.shankerinstitute.org/blog/why-teacher-evaluation-reform-not-failure
[vi] Edward Haertel, âReliability and Validity of Inferences about Teachers Based on Student Test Scores,â William Angoff Memorial Lecture, Washington D.C., March 22, 2013
elaine January 7, 2019
Source
Larry Cuban on School Reform and Classroom Practice
Larry Cuban on School Reform and Classroom Practice: Donors Reform Schooling: Evaluating Teachers (Part 2) published first on https://buyessayscheapservice.tumblr.com/
0 notes
Text
Don't Be Fooled by Data: 4 Data Analysis Pitfalls & How to Avoid Them
Posted by Tom.Capper
Digital marketing is a proudly data-driven field. Yet, as SEOs especially, we often have such incomplete or questionable data to work with, that we end up jumping to the wrong conclusions in our attempts to substantiate our arguments or quantify our issues and opportunities.
In this post, Iâm going to outline 4 data analysis pitfalls that are endemic in our industry, and how to avoid them.
1. Jumping to conclusions
Earlier this year, I conducted a ranking factor study around brand awareness, and I posted this caveat:
"...the fact that Domain Authority (or branded search volume, or anything else) is positively correlated with rankings could indicate that any or all of the following is likely:
Links cause sites to rank well
Ranking well causes sites to get links
Some third factor (e.g. reputation or age of site) causes sites to get both links and rankings" ~ Me
However, I want to go into this in a bit more depth and give you a framework for analyzing these yourself, because it still comes up a lot. Take, for example, this recent study by Stone Temple, which you may have seen in the Moz Top 10 or Randâs tweets, or this excellent article discussing SEMRushâs recent direct traffic findings. To be absolutely clear, Iâm not criticizing either of the studies, but I do want to draw attention to how we might interpret them.
Firstly, we do tend to suffer a little confirmation bias â weâre all too eager to call out the clichĂ© âcorrelation vs. causationâ distinction when we see successful sites that are keyword-stuffed, but all too approving when we see studies doing the same with something we think is or was effective, like links.
Secondly, we fail to critically analyze the potential mechanisms. The options arenât just causation or coincidence.
Before you jump to a conclusion based on a correlation, youâre obliged to consider various possibilities:
Complete coincidence
Reverse causation
Joint causation
Linearity
Broad applicability
If those donât make any sense, then thatâs fair enough â theyâre jargon. Letâs go through an example:
Before I warn you not to eat cheese because you may die in your bedsheets, Iâm obliged to check that it isnât any of the following:
Complete coincidence - Is it possible that so many datasets were compared, that some were bound to be similar? Why, thatâs exactly what Tyler Vigen did! Yes, this is possible.
Reverse causation - Is it possible that we have this the wrong way around? For example, perhaps your relatives, in mourning for your bedsheet-related death, eat cheese in large quantities to comfort themselves? This seems pretty unlikely, so letâs give it a pass. No, this is very unlikely.
Joint causation - Is it possible that some third factor is behind both of these? Maybe increasing affluence makes you healthier (so you donât die of things like malnutrition), and also causes you to eat more cheese? This seems very plausible. Yes, this is possible.
Linearity - Are we comparing two linear trends? A linear trend is a steady rate of growth or decline. Any two statistics which are both roughly linear over time will be very well correlated. In the graph above, both our statistics are trending linearly upwards. If the graph was drawn with different scales, they might look completely unrelated, like this, but because they both have a steady rate, theyâd still be very well correlated. Yes, this looks likely.
Broad applicability - Is it possible that this relationship only exists in certain niche scenarios, or, at least, not in my niche scenario? Perhaps, for example, cheese does this to some people, and thatâs been enough to create this correlation, because there are so few bedsheet-tangling fatalities otherwise? Yes, this seems possible.
So we have 4 âYesâ answers and one âNoâ answer from those 5 checks.
If your example doesnât get 5 âNoâ answers from those 5 checks, itâs a fail, and you donât get to say that the study has established either a ranking factor or a fatal side effect of cheese consumption.
A similar process should apply to case studies, which are another form of correlation â the correlation between you making a change, and something good (or bad!) happening. For example, ask:
Have I ruled out other factors (e.g. external demand, seasonality, competitors making mistakes)?
Did I increase traffic by doing the thing I tried to do, or did I accidentally improve some other factor at the same time?
Did this work because of the unique circumstance of the particular client/project?
This is particularly challenging for SEOs, because we rarely have data of this quality, but Iâd suggest an additional pair of questions to help you navigate this minefield:
If I were Google, would I do this?
If I were Google, could I do this?
Direct traffic as a ranking factor passes the âcouldâ test, but only barely â Google could use data from Chrome, Android, or ISPs, but itâd be sketchy. It doesnât really pass the âwouldâ test, though â itâd be far easier for Google to use branded search traffic, which would answer the same questions you might try to answer by comparing direct traffic levels (e.g. how popular is this website?).
2. Missing the context
If I told you that my traffic was up 20% week on week today, what would you say? Congratulations?
What if it was up 20% this time last year?
What if I told you it had been up 20% year on year, up until recently?
Itâs funny how a little context can completely change this. This is another problem with case studies and their evil inverted twin, traffic drop analyses.
If we really want to understand whether to be surprised at something, positively or negatively, we need to compare it to our expectations, and then figure out what deviation from our expectations is ânormal.â If this is starting to sound like statistics, thatâs because it is statistics â indeed, I wrote about a statistical approach to measuring change way back in 2015.
If you want to be lazy, though, a good rule of thumb is to zoom out, and add in those previous years. And if someone shows you data that is suspiciously zoomed in, you might want to take it with a pinch of salt.
3. Trusting our tools
Would you make a multi-million dollar business decision based on a number that your competitor could manipulate at will? Well, chances are you do, and the number can be found in Google Analytics. Iâve covered this extensively in other places, but there are some major problems with most analytics platforms around:
How easy they are to manipulate externally
How arbitrarily they group hits into sessions
How vulnerable they are to ad blockers
How they perform under sampling, and how obvious they make this
For example, did you know that the Google Analytics API v3 can heavily sample data whilst telling you that the data is unsampled, above a certain amount of traffic (~500,000 within date range)? Neither did I, until we ran into it whilst building Distilled ODN.
Similar problems exist with many âSearch Analyticsâ tools. My colleague Sam Nemzer has written a bunch about this â did you know that most rank tracking platforms report completely different rankings? Or how about the fact that the keywords grouped by Google (and thus tools like SEMRush and STAT, too) are not equivalent, and donât necessarily have the volumes quoted?
Itâs important to understand the strengths and weaknesses of tools that we use, so that we can at least know when theyâre directionally accurate (as in, their insights guide you in the right direction), even if not perfectly accurate. All I can really recommend here is that skilling up in SEO (or any other digital channel) necessarily means understanding the mechanics behind your measurement platforms â which is why all new starts at Distilled end up learning how to do analytics audits.
One of the most common solutions to the root problem is combining multiple data sources, butâŠ
4. Combining data sources
There are numerous platforms out there that will âdefeat (not provided)â by bringing together data from two or more of:
Analytics
Search Console
AdWords
Rank tracking
The problems here are that, firstly, these platforms do not have equivalent definitions, and secondly, ironically, (not provided) tends to break them.
Letâs deal with definitions first, with an example â letâs look at a landing page with a channel:
In Search Console, these are reported as clicks, and can be vulnerable to heavy, invisible sampling when multiple dimensions (e.g. keyword and page) or filters are combined.
In Google Analytics, these are reported using last non-direct click, meaning that your organic traffic includes a bunch of direct sessions, time-outs that resumed mid-session, etc. Thatâs without getting into dark traffic, ad blockers, etc.
In AdWords, most reporting uses last AdWords click, and conversions may be defined differently. In addition, keyword volumes are bundled, as referenced above.
Rank tracking is location specific, and inconsistent, as referenced above.
Fine, though â it may not be precise, but you can at least get to some directionally useful data given these limitations. However, about that â(not provided)â...
Most of your landing pages get traffic from more than one keyword. Itâs very likely that some of these keywords convert better than others, particularly if they are branded, meaning that even the most thorough click-through rate model isnât going to help you. So how do you know which keywords are valuable?
The best answer is to generalize from AdWords data for those keywords, but itâs very unlikely that you have analytics data for all those combinations of keyword and landing page. Essentially, the tools that report on this make the very bold assumption that a given page converts identically for all keywords. Some are more transparent about this than others.
Again, this isnât to say that those tools arenât valuable â they just need to be understood carefully. The only way you could reliably fill in these blanks created by ânot providedâ would be to spend a ton on paid search to get decent volume, conversion rate, and bounce rate estimates for all your keywords, and even then, youâve not fixed the inconsistent definitions issues.
Bonus peeve: Average rank
I still see this way too often. Three questions:
Do you care more about losing rankings for ten very low volume queries (10 searches a month or less) than for one high volume query (millions plus)? If the answer isnât âyes, I absolutely care more about the ten low-volume queriesâ, then this metric isnât for you, and you should consider a visibility metric based on click through rate estimates.
When you start ranking at 100 for a keyword you didnât rank for before, does this make you unhappy? If the answer isnât âyes, I hate ranking for new keywords,â then this metric isnât for you â because that will lower your average rank. You could of course treat all non-ranking keywords as position 100, as some tools allow, but is a drop of 2 average rank positions really the best way to express that 1/50 of your landing pages have been de-indexed? Again, use a visibility metric, please.
Do you like comparing your performance with your competitors? If the answer isnât âno, of course not,â then this metric isnât for you â your competitors may have more or fewer branded keywords or long-tail rankings, and these will skew the comparison. Again, use a visibility metric.
Conclusion
Hopefully, youâve found this useful. To summarize the main takeaways:
Critically analyse correlations & case studies by seeing if you can explain them as coincidences, as reverse causation, as joint causation, through reference to a third mutually relevant factor, or through niche applicability.
Donât look at changes in traffic without looking at the context â what would you have forecasted for this period, and with what margin of error?
Remember that the tools we use have limitations, and do your research on how that impacts the numbers they show. âHow has this number been produced?â is an important component in âWhat does this number mean?â
If you end up combining data from multiple tools, remember to work out the relationship between them â treat this information as directional rather than precise.
Let me know what data analysis fallacies bug you, in the comments below.
Sign up for The Moz Top 10, a semimonthly mailer updating you on the top ten hottest pieces of SEO news, tips, and rad links uncovered by the Moz team. Think of it as your exclusive digest of stuff you don't have time to hunt down but want to read!
http://ift.tt/2nAc68g
0 notes
Text
Don't Be Fooled by Data: 4 Data Analysis Pitfalls & How to Avoid Them
Posted by Tom.Capper
Digital marketing is a proudly data-driven field. Yet, as SEOs especially, we often have such incomplete or questionable data to work with, that we end up jumping to the wrong conclusions in our attempts to substantiate our arguments or quantify our issues and opportunities.
In this post, Iâm going to outline 4 data analysis pitfalls that are endemic in our industry, and how to avoid them.
1. Jumping to conclusions
Earlier this year, I conducted a ranking factor study around brand awareness, and I posted this caveat:
"...the fact that Domain Authority (or branded search volume, or anything else) is positively correlated with rankings could indicate that any or all of the following is likely:
Links cause sites to rank well
Ranking well causes sites to get links
Some third factor (e.g. reputation or age of site) causes sites to get both links and rankings" ~ Me
However, I want to go into this in a bit more depth and give you a framework for analyzing these yourself, because it still comes up a lot. Take, for example, this recent study by Stone Temple, which you may have seen in the Moz Top 10 or Randâs tweets, or this excellent article discussing SEMRushâs recent direct traffic findings. To be absolutely clear, Iâm not criticizing either of the studies, but I do want to draw attention to how we might interpret them.
Firstly, we do tend to suffer a little confirmation bias â weâre all too eager to call out the clichĂ© âcorrelation vs. causationâ distinction when we see successful sites that are keyword-stuffed, but all too approving when we see studies doing the same with something we think is or was effective, like links.
Secondly, we fail to critically analyze the potential mechanisms. The options arenât just causation or coincidence.
Before you jump to a conclusion based on a correlation, youâre obliged to consider various possibilities:
Complete coincidence
Reverse causation
Joint causation
Linearity
Broad applicability
If those donât make any sense, then thatâs fair enough â theyâre jargon. Letâs go through an example:
Before I warn you not to eat cheese because you may die in your bedsheets, Iâm obliged to check that it isnât any of the following:
Complete coincidence - Is it possible that so many datasets were compared, that some were bound to be similar? Why, thatâs exactly what Tyler Vigen did! Yes, this is possible.
Reverse causation - Is it possible that we have this the wrong way around? For example, perhaps your relatives, in mourning for your bedsheet-related death, eat cheese in large quantities to comfort themselves? This seems pretty unlikely, so letâs give it a pass. No, this is very unlikely.
Joint causation - Is it possible that some third factor is behind both of these? Maybe increasing affluence makes you healthier (so you donât die of things like malnutrition), and also causes you to eat more cheese? This seems very plausible. Yes, this is possible.
Linearity - Are we comparing two linear trends? A linear trend is a steady rate of growth or decline. Any two statistics which are both roughly linear over time will be very well correlated. In the graph above, both our statistics are trending linearly upwards. If the graph was drawn with different scales, they might look completely unrelated, like this, but because they both have a steady rate, theyâd still be very well correlated. Yes, this looks likely.
Broad applicability - Is it possible that this relationship only exists in certain niche scenarios, or, at least, not in my niche scenario? Perhaps, for example, cheese does this to some people, and thatâs been enough to create this correlation, because there are so few bedsheet-tangling fatalities otherwise? Yes, this seems possible.
So we have 4 âYesâ answers and one âNoâ answer from those 5 checks.
If your example doesnât get 5 âNoâ answers from those 5 checks, itâs a fail, and you donât get to say that the study has established either a ranking factor or a fatal side effect of cheese consumption.
A similar process should apply to case studies, which are another form of correlation â the correlation between you making a change, and something good (or bad!) happening. For example, ask:
Have I ruled out other factors (e.g. external demand, seasonality, competitors making mistakes)?
Did I increase traffic by doing the thing I tried to do, or did I accidentally improve some other factor at the same time?
Did this work because of the unique circumstance of the particular client/project?
This is particularly challenging for SEOs, because we rarely have data of this quality, but Iâd suggest an additional pair of questions to help you navigate this minefield:
If I were Google, would I do this?
If I were Google, could I do this?
Direct traffic as a ranking factor passes the âcouldâ test, but only barely â Google could use data from Chrome, Android, or ISPs, but itâd be sketchy. It doesnât really pass the âwouldâ test, though â itâd be far easier for Google to use branded search traffic, which would answer the same questions you might try to answer by comparing direct traffic levels (e.g. how popular is this website?).
2. Missing the context
If I told you that my traffic was up 20% week on week today, what would you say? Congratulations?
What if it was up 20% this time last year?
What if I told you it had been up 20% year on year, up until recently?
Itâs funny how a little context can completely change this. This is another problem with case studies and their evil inverted twin, traffic drop analyses.
If we really want to understand whether to be surprised at something, positively or negatively, we need to compare it to our expectations, and then figure out what deviation from our expectations is ânormal.â If this is starting to sound like statistics, thatâs because it is statistics â indeed, I wrote about a statistical approach to measuring change way back in 2015.
If you want to be lazy, though, a good rule of thumb is to zoom out, and add in those previous years. And if someone shows you data that is suspiciously zoomed in, you might want to take it with a pinch of salt.
3. Trusting our tools
Would you make a multi-million dollar business decision based on a number that your competitor could manipulate at will? Well, chances are you do, and the number can be found in Google Analytics. Iâve covered this extensively in other places, but there are some major problems with most analytics platforms around:
How easy they are to manipulate externally
How arbitrarily they group hits into sessions
How vulnerable they are to ad blockers
How they perform under sampling, and how obvious they make this
For example, did you know that the Google Analytics API v3 can heavily sample data whilst telling you that the data is unsampled, above a certain amount of traffic (~500,000 within date range)? Neither did I, until we ran into it whilst building Distilled ODN.
Similar problems exist with many âSearch Analyticsâ tools. My colleague Sam Nemzer has written a bunch about this â did you know that most rank tracking platforms report completely different rankings? Or how about the fact that the keywords grouped by Google (and thus tools like SEMRush and STAT, too) are not equivalent, and donât necessarily have the volumes quoted?
Itâs important to understand the strengths and weaknesses of tools that we use, so that we can at least know when theyâre directionally accurate (as in, their insights guide you in the right direction), even if not perfectly accurate. All I can really recommend here is that skilling up in SEO (or any other digital channel) necessarily means understanding the mechanics behind your measurement platforms â which is why all new starts at Distilled end up learning how to do analytics audits.
One of the most common solutions to the root problem is combining multiple data sources, butâŠ
4. Combining data sources
There are numerous platforms out there that will âdefeat (not provided)â by bringing together data from two or more of:
Analytics
Search Console
AdWords
Rank tracking
The problems here are that, firstly, these platforms do not have equivalent definitions, and secondly, ironically, (not provided) tends to break them.
Letâs deal with definitions first, with an example â letâs look at a landing page with a channel:
In Search Console, these are reported as clicks, and can be vulnerable to heavy, invisible sampling when multiple dimensions (e.g. keyword and page) or filters are combined.
In Google Analytics, these are reported using last non-direct click, meaning that your organic traffic includes a bunch of direct sessions, time-outs that resumed mid-session, etc. Thatâs without getting into dark traffic, ad blockers, etc.
In AdWords, most reporting uses last AdWords click, and conversions may be defined differently. In addition, keyword volumes are bundled, as referenced above.
Rank tracking is location specific, and inconsistent, as referenced above.
Fine, though â it may not be precise, but you can at least get to some directionally useful data given these limitations. However, about that â(not provided)â...
Most of your landing pages get traffic from more than one keyword. Itâs very likely that some of these keywords convert better than others, particularly if they are branded, meaning that even the most thorough click-through rate model isnât going to help you. So how do you know which keywords are valuable?
The best answer is to generalize from AdWords data for those keywords, but itâs very unlikely that you have analytics data for all those combinations of keyword and landing page. Essentially, the tools that report on this make the very bold assumption that a given page converts identically for all keywords. Some are more transparent about this than others.
Again, this isnât to say that those tools arenât valuable â they just need to be understood carefully. The only way you could reliably fill in these blanks created by ânot providedâ would be to spend a ton on paid search to get decent volume, conversion rate, and bounce rate estimates for all your keywords, and even then, youâve not fixed the inconsistent definitions issues.
Bonus peeve: Average rank
I still see this way too often. Three questions:
Do you care more about losing rankings for ten very low volume queries (10 searches a month or less) than for one high volume query (millions plus)? If the answer isnât âyes, I absolutely care more about the ten low-volume queriesâ, then this metric isnât for you, and you should consider a visibility metric based on click through rate estimates.
When you start ranking at 100 for a keyword you didnât rank for before, does this make you unhappy? If the answer isnât âyes, I hate ranking for new keywords,â then this metric isnât for you â because that will lower your average rank. You could of course treat all non-ranking keywords as position 100, as some tools allow, but is a drop of 2 average rank positions really the best way to express that 1/50 of your landing pages have been de-indexed? Again, use a visibility metric, please.
Do you like comparing your performance with your competitors? If the answer isnât âno, of course not,â then this metric isnât for you â your competitors may have more or fewer branded keywords or long-tail rankings, and these will skew the comparison. Again, use a visibility metric.
Conclusion
Hopefully, youâve found this useful. To summarize the main takeaways:
Critically analyse correlations & case studies by seeing if you can explain them as coincidences, as reverse causation, as joint causation, through reference to a third mutually relevant factor, or through niche applicability.
Donât look at changes in traffic without looking at the context â what would you have forecasted for this period, and with what margin of error?
Remember that the tools we use have limitations, and do your research on how that impacts the numbers they show. âHow has this number been produced?â is an important component in âWhat does this number mean?â
If you end up combining data from multiple tools, remember to work out the relationship between them â treat this information as directional rather than precise.
Let me know what data analysis fallacies bug you, in the comments below.
Sign up for The Moz Top 10, a semimonthly mailer updating you on the top ten hottest pieces of SEO news, tips, and rad links uncovered by the Moz team. Think of it as your exclusive digest of stuff you don't have time to hunt down but want to read!
http://ift.tt/2nAc68g
0 notes