#alexa what are the different types of drones
Explore tagged Tumblr posts
Photo

Top online trends to look forward to in 2020
Video content marketing dominated 2018, especially when it came to innovations from Facebook, Instagram and Snapchat. The same trend followed 2019 but with more changes that helped video content marketing. Artificial intelligence and social media marketing raised the bar for video content marketing with artificial intelligence also having its own significant breakouts. For 2020, video content marketing will still continue its streak in terms of advertisements for online shopping. Still, it would be innovations in artificial intelligence that would usher a new age of voice-powered search. To narrow down a long list of trends for 2020, here are some of the top emerging trends in the fields of e-commerce, visual content, technology and digital marketing.
On E-Commerce: Google Shopping
Over the past couple of years, Google experienced some setbacks with GDPR and other privacy issues. Still, they have shown in 2019 that they can remain on top not just as a search engine but as one of the world’s leading innovators in artificial intelligence and e-commerce. On the other hand, working hard on organic SEO and paid advertisements wouldn’t be enough in 2020. Following Google’s algorithm in ranking search queries and displaying ads, it’s only natural that their new product – Google Shopping – will also be included in this list.
As Google is becoming more and more an online marketplace, its developers created Google Shopping to accommodate the growing market and make shopping easier. Just like websites that compare flights or hotel fares, Google Shopping allows users to compare product prices from different e-commerce websites. Currently available via the Google web and the Google app, both enable users to search for products, view their shopping lists, track their order history and check their notification settings. Google also offers product guarantee and their customer service support for enquires on product changes, late shipping, returns and more.
With Google Shopping’s landing search, e-commerce is expected to rise, especially since a recent case study showed how ads listed in Google Shopping helped boost conversions by over 17%. Sellers only need to create a Google Merchant account to be able to be added on Google’s database for Google Shopping. Users who are looking to buy products online can type in their enquiries on Google Search or Google Shopping and then the search engine will show a list of products comparing the merchants that have the item. Depending on the account setting of the user, Google can also filter their product search based on your personal configuration and based on Google recommendations.
As this platform continues to develop and rise in 2020, more and more merchants are expected to join the community. Small businesses who only have an online store are expected to see an increase in products sold because of Google’s new approach for e-commerce.
On Visual Content: AR and VR
Like 2019, Augmented Reality or Virtual Reality will still stay on top in terms of visual content. With big brands like Amazon and IKEA using AR and VR to make their products more accessible to their customers, more and more businesses are expected to take part in this type of visual content to promote their products and services, genuinely creating a new trend for customer experience.
Online businesses with no physical stores like eyewear shops that ship directly to customers will now have the chance to compete with others in their trade by using AR/VR to help customers decide what to buy. Customers will be asked to take a photo of their face in various angles. Then the computer will process the image and detect multiple points on your face to capture your movements, allowing you to virtually try on various eyewear in different shapes, colours and sizes at the comfort of your home.
On Technology: 5G Cellular Network
As mobile usage becomes more prominent, it is only natural that the internet also upgrades its features to meet the soaring demands of small-screen internet usage. The 5G cellular network boosts faster internet connection that makes mobile internet usage faster and downloads quicker to finish. The fifth-generation cellular network was also designed to reduce congestion on mobile networks so that consumers can get the full quality-service of what they are paying for. With this innovation, 5G can make flying drones cover more extensive ranges and driverless cars more convenient to use since the fast cellular connection would allow all smart devices within the vicinity to interact with each other and avoid accidents.
On Digital Marketing: Voiced-Power Search
From voice commands on mobile phones which were designed to help the visually impaired, to voice commands for convenient use, voice input has come a long way. With more and more Voice Assistant devices popping up in the market, further innovations from voice assistant pioneers like Google, Apple, and Amazon will continue to rise, especially in terms of speech recognition. The ability to accurately detect voice will improve search queries and command prompts, especially when it comes to voice assistants, mainly Google’s Google Home, Amazon’s Alexa and Echo, and Apple’s HomePod.
Innovations in voice-powered search will also change the way SEO is written because search engines have started to rewrite their algorithms to give priority to searches that answer voice-powered queries. Since these voice searches are designed to be conversational, the language for which online content is written will also have to change to be able to rank in search engine pages. For example, people usually type the search ‘ABC Mall opening time’, but when it comes to voice-powered search people will say, “what time does ABC Mall open?�� and websites should answer this question well enough to be recognised and ranked under voice-powered enquiries. An excellent way to answer this would be to write how you would ordinarily answer a question posed to you by a friend; “ABC Mall opens at 10 AM today”.
As 2020 ushers in these technological developments that will surely influence how people do things in their everyday lives, it is vital for digital-dependent businesses to catch up to the world. That’s why at Bureauserv, we’ll make sure to adapt these changes to be able to compete in the ever-growing technological world especially when it comes to digital marketing.
We’ll adapt these new trends to improve our Digital Marketing services especially when it comes to Web Design and Development, (SEO) Search Engine Optimisation, and Social Media Marketing.
#2020#trends#voice assistant#content marketing#digital marketing#bureauserv global inc#virtual reality#blog post
2 notes
·
View notes
Text
Wednesday 11th September- 2 Kings 3:15
“Now bring me a musician”. While the musician played, the Lord’s hand came on Elisha.
2 Kings 3:15
Or in the New Glasgow Translation, “Alexa, play me some Hillsong”...
Here the musician starts to play, God’s presence comes and He speaks.
If you’ve been to pre-service prayer, you’ll know that the band plays the whole time. We also play whilst the person leading the service prays and reads scripture. The aim is not to emotionally manipulate the congregation or add a bit of pizazz to the prayer. We play because throughout the Bible, worship acts as a catalyst for the presence of God, it sets God in His rightful place (on the throne), it realigns our spirit with His and as Elisha shows us, it makes way for God to speak.
It strikes me as weird that Elisha responds to the frustration of corrupt people being bossy and demanding he hears from God by adding more noise, rather than going off for some peace and quiet. It’s just as odd when Saul, over in 1 Samuel 16, responds to intense spiritual torment by bringing in David (who we can all agree was an anointed worshipper, he literally wrote the book on it) to play his lyre. However, I realise from these passages that music is not a drone used to drown everything else out, it is a noise that connects our spirit with God’s and being caught up in that diminishes everything else, making it easier to meet with Him and hear from Him.
These examples show us that worship music is different to other music. Music made or played with the intention of glorifying God and bringing us closer to him can bring revelation, peace and deeper intimacy with Him. That’s why I (a very non-feelingsy person) don’t cry at Adele songs, but I weep when I hear the intro to In Christ Alone, or feel like dancing when I listen to Lecrae rap, or fall to my knees in repentance (just in my own flat…) when I hear a Jonathan McReynolds song. It’s not just music, it’s for God and from God and His Holy Spirit loves to move through it.
In Elisha’s story, we are taught that worship sets us up to hear from God. In Saul’s story, it drives out a tormenting spirit. Both of these show us that worship changes the atmosphere and ushers in the Spirit of God.
Are you struggling to hear from God about something? Start off by worshipping.
What atmosphere would you like the Holy Spirit to change? Your home? Your work place? Find a way of worshipping God in that space (I mean actually, physically… like go to your office early and blast the tunes).
Do you feel tormented and need your spirit to be realigned with God’s? Listen to worship music. Don’t be limited by what we sing in church. Here is a list of some of my favourite worshipers to get you started (just type them into YouTube): Jonathan McReynolds, People + Songs, Worship Mob, Brooklyn Tabernacle Choir, Celtic Worship.
Naomi Stirrat
2 notes
·
View notes
Text
What is Deep Learning and How Does Deep Learning Work?
In the past few years, we’ve seen a surge of research and development in artificial intelligence. This has resulted in a variety of new AI applications, such as speech recognition and computer vision. However, no subfield of AI research has seen more progress than Deep Learning.

These days, almost every article on AI seems to mention Deep Learning. You might be wondering: What is Deep Learning? How Does Deep Learning Work? And what are some examples of Deep Learning technologies? In this blog post, we will answer all your questions about Deep Learning and its role in the future of AI research.
What is Deep Learning?
Deep Learning is a subfield of machine learning that uses neural networks to build AI systems. Neural networks are computer systems modeled after the human brain, including the structure and function of neurons. Neural networks have been used in computer science for a long time.
In recent years, an advancement in technology and a surge in machine learning research have led to major improvements in neural network technology. This has paved the way for a new type of neural network called deep learning. Deep learning neural networks have many layers, allowing them to learn complex, multi-step tasks like image recognition.
Deep learning is inspired by the architecture of the human brain. It’s made up of multiple layers, each of which performs a different type of computation. This makes it well-suited to problems such as image recognition, where there are many different types of information and each has a different technical term.
Why Is Deep Learning Important?
Currently, Deep Learning is the state-of-the-art in machine learning and artificial intelligence. It is the most powerful technology that exists today for building AI systems. It has the ability to solve many problems that were previously thought to be impossible for computers to solve.
This sets the stage for ground breaking progress in AI research. Deep Learning’s recent rise to prominence is due to several factors. First, the past decade of progress in computer hardware has resulted in a massive increase in computing power. This has made it possible to train more complex neural networks than ever before. Second, the emergence of new, easy-to-use software platforms has made it much easier for non-experts to build and deploy Deep Learning systems.
This has allowed researchers and businesses to experiment with Deep Learning on a much larger scale. Finally, the availability of large sets of training data has made it possible to train much more complex neural networks than previously possible.
Some examples of Deep Learning AI
Speech recognition
This system tries to translate sounds that you make into written words. This technology is used in digital assistants like Apple’s Siri, Amazon’s Alexa, and Google’s Home.
Computer vision
This system tries to understand the content of visual images. This technology is used in image and video recognition, image tagging, and image filtering.
Natural language processing
This system tries to understand the meaning of written language. This technology is used in machine translation, sentiment analysis, and automatic writing.
Recommendation systems
This system tries to predict what a person will like. This technology is used in shopping carts, social media feeds, and Netflix.
Robotics
This system tries to control the movement of robots. This technology is used in self-driving cars, automated warehouses, and autonomous drones.
Healthcare
This system tries to assist doctors and nurses in diagnosis and treatment. This technology is used in medical imaging, drug discovery, and genetic research.
How Does Deep Learning Work?
To understand how deep learning works, let’s first take a look at how traditional machine learning works. In traditional machine learning, you have an algorithm with certain parameters.Image Source By: quantib
The algorithm takes in some data as input and it produces some output, such as a prediction or a diagnosis. What’s important is that the algorithm has been programmed to work in a certain way. The algorithm is what engineers call a black box. It’s a system that you can’t see inside of because there’s no way to get inside of it.
The way deep learning works is that you take a neural network and you train the neural network to do a certain task. So let’s say that you’re training the neural network to do image recognition. What you do is you take a bunch of images, you feed the images into the network, and then you tell the network, “Hey! For each one of these images, tell me what objects are in the image.”
The Road Ahead for Deep Learning
As we’ve seen, deep learning has revolutionized the field of AI research. It has enabled scientists to build powerful AI systems that can solve problems that were previously thought to be impossible for computers to solve. However, there is still much room for improvement.
Deep learning networks have high error rates. The best systems have error rates that are 10-20% lower than human error rates on standardized tasks. This makes them useful for many applications, but not perfect. This presents an opportunity to improve the technology, as well as an important challenge. How will researchers overcome these limitations? The answer will determine the future of deep learning research.
Key takeaway
Deep learning is a subfield of machine learning that uses neural networks to build AI systems. Its recent rise to prominence is due to several factors, including the availability of large sets of training data and the availability of easy-to-use software platforms. Deep learning has revolutionized the field of AI and enabled scientists to build powerful AI systems. There is still room for improvement, and the future of deep learning research will be determined by how researchers overcome these limitations.
#artificial intelligence#technology#techalertx#tech news#AI research#Deep Learning#future of deep learning#How Does It Work?#Neural networks#Robotics technology#What is Deep Learning?
0 notes
Text
Artificial Intelligence : where are we headed for ?
The world is set to see a sea change in the manner technology operates and responds to our need. As per an internal report, more than 80% of businesses would be using Artificial Intelligence, one way or the other by 2020,
Defining strategies based on AI for competitive advantage and intelligence will serve to be the basics when planning for a smart and agile enterprise in the coming times. Self-serving automated devices would prove to be catalysts ushering into a new era that would become mainstream. whether it is the automotive industry or the healthcare industry, artificial intelligence would prove to be the flag-bearer in decision making.
Transformation in personal lifestyle would definitely bring about the eventual adoption an everyday affair. This is primarily based on man and machine relationship and therefore an opportunity for a transformative potential for a next-gen organization. Whilst Machine learning can solve linear problems, Artificial Intelligence helps distillation of automation. We are talking about disruptive technologies based on design thinking that lead to the simplification of tasks and better decision making structures.
There is a tender inflection point between data and artificial intelligence that would prove to be deciduous for today's CIO to prioritize and adapt. Tangible and value-added insights in the business if delivered to address a pre-defined challenge can reap true benefits
So what does it take to become an AI-driven organization?
What is the impact of AI and eventually machine learning on our daily lives? An interesting case study that I saw on the economist TV channel that details the role of technology in the coming decades and how it would impact
By 2050 worlds 2/3 population would live in urban areas. In order to better our living conditions owing to congestion on account of population, artificial intelligence, and predictive technologies would have a major role to contribute to daily lives. Consider this, The subway system in North Korea in Seoul is generally managed through a sophisticated technology system that monitors daily traffic or footfall over the stations through data technologies. The administrators manage to pre-arrange and adjust the speed of trams and stoppage times based on the technology and the data gathering.
This way they gather in advance the number of passengers and the time needed for them to board at different stations.Not only that, they keep citizens informed through social media and internet channels about the arrival of trains and stoppage times. Whats even more interesting is, that machine learning helps the administrators find faults and any kind of discrepancies in the tram equipments in advance. Thus, saving time and balancing processes at such a scale is only enabled through machine learning and artificial intelligence meeting scale and state-of-the-art infrastructure
This is a typical example of data management models sitting at the core of AI.
Ai and Digital Marketing
In a digital landscape, a great user experience or user journey is where AI adapts to and makes payments and transactions much faster and eases decision-making algorithms.
AI, therefore, holds a huge potential in the field of Digital marketing, predictive analytics, and targeted marketing and would surely provide for a smarter future and consequently, a better customer experience.
We have advanced applications based on the same technology, This would also imply towards a change in the way we analyze and assess. Assessing current data to extrapolate future trends and benchmarking business excellence is a major key result area that we may for-see and it could be social media data, purchase pattern of customers or clinical data of customers mapped in a hospital. we would be able to predict our best customers and also offer them tailor-made answers to their questions which could be in the form of products and services.
Automation in data and knowledge work is another major stakeholder. Insights and value-driven inferences would lead to broader perspectives.
There would be a time in the near future where every company would increase their investments in artificial intelligence whether it is retail, healthcare, government and more. Inferences and value led insights would navigate the ever-evolving and rapidly changing the technological landscape.
Think about precision targeting that is based on behaviors and backed by intelligence and a Marketers quickest response would be Artificial Intelligence.
Mining customers based on their individual choices and preferences, and thereby Collecting and analyzing data to make specific decisions based on predictive technologies can leverage the effectiveness of a marketing campaign. Gone are the days of IP address based predictions, now AI maps customer journeys and targets based on customer choices and preferences.
A great CRM tool coupled with analytics can be a very promising combination today but would soon be a hygiene. The intersection of AI and marketing enables Personalizes User Experience (UX). Artificial Intelligence tracks search queries generated through search engines. Hence the search engine today is smarter.
Purchase behavior and multiple touch points lead to a pre-defined path based on search strings to get an exact match to the specifics that are custom generated through Artificial Intelligence. This automatically reduces complexity and noise leading to value-added conversions based on an exact match on demand and supply.
Hello "Chatbots"
Chatbots would be a common feature soon. Apps lead to bots and bots ultimately integrate with data and machine learning models.
"A chatbot is a computer program or an artificial intelligence powered program which conducts a conversation via auditory or textual methods ; chatbots are typically used in customer service or information acquisition and many systems scan for keywords within the input, then pull a reply with the most matching keywords, or the most similar wording pattern, from a database." so says Wikipedia.
Chatbots have been designed to boost business outcomes and craft superior experiences in order to better overall productivity. The purpose of a bot is also to apply and build meaningful responses to these unique requirements over time. They have been invented to provide for answers we are looking for. For example to be able to do a Web search without opening up multiple apps. you ask for it and it answers.
for instance, I type on Facebook messenger and book for a dinner reservation, that should not be an issue.
In yet another example, you ask for a data analysis report without juggling tabs and applying multiple commands, you will have an answer in fractions of a minute. Now, this is going to get that simple. An application in a sharp contrast is going to be heavier and a tedious affair. Thus the arrival of a chatbot.
Chatbots recognize keywords and access the database for input towards giving a predefined response. for example; configuration of a MacBook Air instead of MacBook.So imagine having your own personal assistant soon who could solve and answer questions of your customers, generate pre-scripted yet agile answers to perfection.
Most important changes would be best seen in human to machine interaction. We would not be surprised to see chatbots as psychological counselors working with human emotions. Siri is a very simple yet minimal example.
The retail industry is surely in for a big and deciduous change and as harsh as it may seem many in the industry would lose their jobs to chatbots. Instead, we would see a higher number of tech compliant jobs that also demand a higher skill set.
Of course! There is innovation and there are opportunities for brewing.
Some more popular examples :
Heard of IBM Watson? The smart AI & machine learning based supercomputer. You throw off a challenge and IBM Watson gives you the answer, posed in natural language processing. It builds and analyses workflows for better processes.
In our daily lives, Amazon has launched 'Alexa', your smart personal digital assistant that would do everything from booking a table for you in a restaurant to answering your queries on music.
I personally believe that a large part of this innovation came to set in when Apple gave us iPhone. The connotation of technology just hit another trajectory with such an explosive shake-up.
You might disagree, but I may go candid and say, since the launch of Apple iPhone, innovation has lately been a complete game changer. No wonder many stalwarts like Samsung have bowed to the "game-changing "blueprints and in fact followed suit. Off late, the features that come integrated with technology resonate with people's choices and preferences. We have more to see based on iterative refinement that clubs effectively with the digital future of the Gen Y and blends well with their lifestyles.
There are other impacts of AI such as self-driving cars. Drones and many of the possible cyborg enhancements in our future everyday lives. Let's not forget Jarvis in movie " Iron Man", the lead protagonist of Tony stark industries. What better example to expatiate this. So a very simple and basic question arises, would our dependence on AI lead to the transfer of an autonomous control to man-made machines? How different the future looks now?
Remember the sky net series, it is perhaps not very far from reality I suppose. But many argue that AI can be detrimental to the human race.
The Pros and Cons: Do we need to stay on guard?
As per an excerpt in the conversation.com,
"The late Stephen Hawking was a major voice in the debate about how humanity can benefit from artificial intelligence. Hawking made no secret of his fears that thinking machines could one day take charge. He went as far as predicting that future developments in AI "could spell the end of the human race. Hawking cautioned against an extreme form of AI, in which thinking machines would "take off" on their own, modifying themselves and independently designing and building ever more capable systems. Humans, bound by the slow pace of biological evolution, would be tragically outwitted."
At a gathering at the crown plaza hotel at New Delhi, we were listening to Mr. Ravinder Pal Singh, CIO, Tata Sia Airlines. He vehemently pointed out that customer trust factor on transactions can also decide the adoption of a particular technology, such is defined the role of chatbots in commerce transactions.
Artificial intelligence may be the biggest shakeup in the technological landscape as such a system could potentially trigger self-improvements and modifications that would outwit the human intelligence. whilst we would go a sea change in technologies such as Banking, healthcare, Retail, Resistance to change, the absence of sponsorship, culture non-adherence by the top shots and many other factors contribute to hampering of non-adoption and compliance to such innovative technologies.
There are other apprehensions, that a large number of the workforce or human labor may be replaced owing to the invasion and evolution of chatbots Or there is no replacement for human judgment and sixth sense?
But many industries would benefit immensely too with critical information coming into better processor business outcomes.
What cannot be ruled out though is that we have already embraced this change and barricading our limitations may be in the best interest of the times to come?
Know more about Artificial Intelligence at http://vivoki.com/
Author: Ms. Deepa Sayal ( Digital Mentor, Incubator, Entrepreneur and a tech evangelist with 20 years of holistic experience in the Information Technology domain and was featured in the CNBC TV-18 to be "amongst the 32 impactful women changing the Digital world" in India)
1 note
·
View note
Text
TK Roofing Announces New Advancements in Residential Roofing Industry
Akron-based roofing contractors have published a new blog post exploring the revolution in the residential roofing industry. TK Roofing & Gutters regularly publishes informative blog posts to raise awareness about the advancements in roofing and construction. According to Daryl Gentry, the owner of the roofing company, “Technology is making the job of a roofer safer and more efficient, and it saves companies and their customer's time and money.”
Technological developments in the field have brought drones into the industry as key tools used in roofing inspections. “The use of drones allows contractors to keep their feet planted firmly on the ground on the job site,” says the owner of TK Roofing & Gutters.
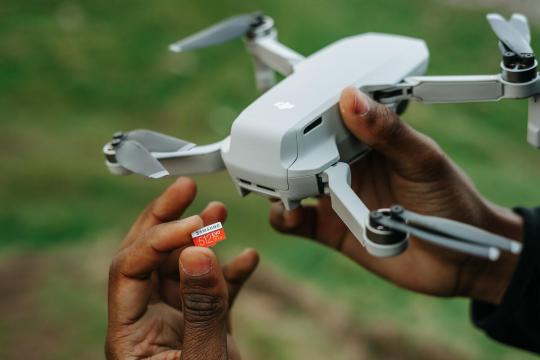
The roofing industry has started to take advantage of robotic technology to add greater efficiency and safety to their projects. The local roofing company leverages robotic technology to assess the safety of damaged roofs through aerial pictures captured by drones or rovers.
Green Roofs are very much a trend these days but constructing a green roof is better left to expert roofing contractors. A green roof is designed to foster the growth of vegetation through the construction of a waterproofing layer as a base, root barrier, and drainage system. This also involves constructing a medium to grow plants. Roofing contractors are responsible for following proper safety protocols to create roof gardens with beautiful plants and water features.
As opposed to the popular opinion that a green roof is “expensive, leaky, and complicated,” says the lead roofing contractor at TK Roofing & Gutters, adding, “it is entirely possible to create a sustainable green roof that doesn’t need to cost a fortune with the help of an experienced contractor.”
Technology has enabled the integration of solar panels with roofing materials, resulting in new solar shingles that blend with the rest of the shingles to create a viable backup power source.
One of the drawbacks of solar shingles, according to Daryl Gentry, is the cost factor and the need for too much sunlight exposure to be effective.
“Your typical roof absorbs the heat from the sun, making it even hotter on the inside of your home. Combine that with poor ventilation, and you might feel like you need a second job just to pay your energy bills.”
TK Roofing & Contractors leverage cool roofing technology with a reflecting coating to cut down on the energy costs during scorching summers. The reflective coating is designed to reflect the heat away from the roof. When the roof does not absorb the heat, it keeps the building cool. In addition to keeping the home cooler during hot summer days, cool roofs remain damage-free from sunlight in the long run.
Another technological innovation in the roofing industry is augmented reality, which, according to the Ohio roofing contractors, creates a virtual roof installation to enable customers to see what their home will look like after construction.
“Augmented reality will let you point your smartphone camera toward your home, "install" your new roof in real-time, and view it from any angle.” Roofing technology is meant to facilitate the task of constructing safe and efficient roofs. An increasing number of roofing contractors are adopting technology to provide better services for clients.
TK Roofing & Gutters prides itself on its craftsmanship and precision while embracing new technologies that are transforming the roofing industry.
The fully insured and bonded roofing company is based in Akron, Ohio, and has over 25 years of experience staying ahead of the roofing industry curve. Roofing contractors in the company have earned a reputation for their reliable roofing services across a range of properties, from rubber roofing to single and multi-homes, flat roofs, and asphalt shingles.
https://www.youtube.com/embed/JaRa8Zmy2CQ
Anyone looking for the best residential roofing contractor in Akron for a new roof, gutter, or roof repairs might want to get in touch with TK Roofing and Gutters through their website. The company is just a phone call away on (330) 525-8607.
[ { "@context": "http://schema.org", "@type": "BlogPosting", "keywords": [ "best roofing company", "best roofing contractors", "how technology is changing the roofing industry", "roofing company", "roofing contractors", "roofing technology" ], "timeRequired": "P0Y0M0DT0H7M0S", "mentions": [ "https://en.wikipedia.org/wiki/Asphalt_shingle", "https://en.wikipedia.org/wiki/Augmented_reality", "https://en.wikipedia.org/wiki/Building-integrated_photovoltaics", "https://en.wikipedia.org/wiki/Domestic_roof_construction#Gallery", "https://en.wikipedia.org/wiki/Green_roof", "https://en.wikipedia.org/wiki/Photovoltaic", "https://en.wikipedia.org/wiki/Reflective_surfaces_(climate_engineering)", "https://en.wikipedia.org/wiki/Roof", "https://en.wikipedia.org/wiki/Roof_shingle", "https://en.wikipedia.org/wiki/Roofer", "https://en.wikipedia.org/wiki/Smartphone", "https://en.wikipedia.org/wiki/Solar_panel", "https://en.wikipedia.org/wiki/Solar_shingle", "https://en.wikipedia.org/wiki/Unmanned_aerial_vehicle", "https://en.wikipedia.org/wiki/Virtual_reality" ], "isAccessibleForFree": true, "isFamilyFriendly": true, "about": [ "https://en.wikipedia.org/wiki/Domestic_roof_construction", "https://en.wikipedia.org/wiki/Roof", "https://en.wikipedia.org/wiki/Roofer" ], "inLanguage": "English", "image": "https://www.tkroofingandgutters.com/hs-fs/hubfs/drone.jpg?width=1200&name=drone.jpg", "sameAs": [ "https://sites.google.com/view/tkroofingandguttersroofingcooh/blog/how-technology-is-changing-the-roofing-industry", "https://youtu.be/JaRa8Zmy2CQ" ], "url": "https://www.tkroofingandgutters.com/blog/how-is-technology-changing-the-roofing-industry", "alternativeHeadline": "See How Roofing Technology is Changing the Roofing Industry", "description": "Technology is changing how the roofing industry operates. Making the job safer and more efficient as well as extending the life of your roof. ", "name": "How Is Technology Changing The Roofing Industry", "datePublished": "2020-12-14T09:39:00-05:00", "audience": "People looking to replace their roof", "headline": "How Is Technology Changing The Roofing Industry?", "dateModified": "2020-12-14T09:40:00-05:00", "articleBody": "Technology has become an essential part of our lives. We don't go anywhere without a supercomputer in the form of a smartphone in our pockets, and there's not a day that goes by when we aren't in front of some type of computer. Siri and Alexa have made our lives easier, and we rely on innovation and new technologies now more than we ever have. And, it's not just our personal lives that our evolving because of technologies. Technology is also revolutionizing entire industries, and the roofing industry is no exception. Roofing professionals have been receptive to different methods of performing their tried and true procedures, allowing technology to enhance roofing businesses across the country. Technology is making the job of a roofer safer and more efficient, and it saves companies and their customer's money and time. In the article below, we will look at some innovations that are revolutionizing the residential roofing industry. Drones Drones went from being an expensive piece of new technology that only a few people could afford to being toys for kids. But drones have efficient uses in several industries, including the roofing industry. Retailers use drones to make home deliveries, and filmmakers use drones to capture shots that once required a helicopter. In the roofing industry, drones are being used to perform roofing inspections. Drones are also much safer than the old way of performing a roofing inspection because no one has to climb up a ladder onto the roof. The use of drones allows contractors to keep their feet planted firmly on the ground on the job site. Robots and Roofing Robots have been used in industries like the automobile industry for years to streamline production. The roofing industry is beginning to dip their toes in this technology to make projects safer and more efficient. Robots in the form of rovers are used to take pictures of damaged roofs to assess them and build estimates. Some robots can even install certain items during the construction phase of a roofing project. Green Roofs A green roof is a roof that fosters the growth of vegetation. Green roofs consist of a waterproofing layer, a root barrier, a drainage system, and a medium to grow the plants. Roof gardens can be accessible and include large plants and water features. Green roofs are believed to be expensive, leaky, and complicated to build, making them slower to be widely adopted. However, a green roof isn't much more complicated to construct than a typical roof. It is entirely possible to create a sustainable green roof that doesn’t need to cost a fortune with the help of an experienced contractor. Solar Shingles The integration of building-integrated photovoltaics, or BIVPs, means that solar panels can now be combined with other roofing materials to create new solar shingles. When most people think of solar panels, they think of huge panels that weigh down your roof and that aren't very efficient. But solar shingles blend in with the rest of your shingles and are efficient enough to be a viable backup power source. But, like any other solar panel, solar shingles still need a lot of sunlight to be effective, and they are still pretty expensive. Cool Roofs With climate change and temperatures rising, our energy bills are skyrocketing as we try to keep our homes cool. Your typical roof absorbs the heat from the sun, making it even hotter on the inside of your home. Combine that with poor ventilation, and you might feel like you need a second job just to pay your energy bills. Cool roof technology is a great way to keep your energy costs low during the scorching summers. Cool roofing technology consists of a reflective coating that reflects the heat away from your roof instead of absorbing it. In addition to keeping your home cooler during the summer, cool roofs will help prevent damage caused by the sunlight in the long run. Augmented Reality Augmented reality is a technology that allows live video to change the environment around you. This technology allows residential roofing contractors to create a virtual roof installation that will enable their customers to see what their home will look like with their new roof. Augmented reality will let you point your smartphone camera toward your home, \"install\" your new roof in real-time, and view it from any angle. The Future Of Roofing Roofing will always be an industry that prides itself on craftsmanship and precision. However, the industry is also embracing new technologies that are changing the world, allowing significant advances to be made in the way roofers communicate, work, and interact with their customers. Roofing technology is allowing roofing contractors to provide better services for their clients while enhancing their bottom line and staying safer. Technology is changing the roofing industry, and everyone stands to benefit from these advances. Contact TK Roofing and Gutters If you need a new roof, roof repairs, or a new gutter system, and you want to work with a roofing contractor that takes full advantage of new technologies, contact TK Roofing and Gutters. TK Roofing offers the longest, most dependable warranties on the market. With experienced craftsmen, a \"customer first\" mentality, and affordable prices, they will make your home improvement projects a breeze. Contact Us by calling (330) 858-2616 or click the button below to set up a free inspection and quote!", "author": { "@type": "Person", "sameAs": "https://www.facebook.com/daryl.gentrylindsay", "name": "Daryl Gentry", "url": "https://www.tkroofingandgutters.com/about-tk-roofing", "description": "Daryl Gentry is the founder of TK Roofing and Gutters, a premier roofing contractor serving all of Ohio.", "@id": "https://www.tkroofingandgutters.com/#Person1" }, "publisher": { "@type": "Organization", "sameAs": [ "https://sites.google.com/view/tkroofingandguttersroofingcooh/", "https://tkroofingandgutters.business.site/", "https://tkroofingandgutters.tumblr.com/", "https://twitter.com/gutterstk", "https://www.angieslist.com/companylist/us/oh/akron/tk-roofing-and-gutters-reviews-9819644.htm", "https://www.bbb.org/us/oh/akron/profile/roofing-contractors/tk-roofing-and-gutters-0272-235828502", "https://www.facebook.com/TKRoofingandGutters/", "https://www.mapquest.com/us/ohio/tk-roofing-gutters-425565323", "https://www.yelp.com/biz/tk-roofing-and-gutters-akron" ], "description": "\"We are a Fully Insured Roofing and Gutter Installation Company with over 25 Years of Experience. We are the best Roofing and Gutter Company near you. Ohio's Premiere Roofing and Gutter Company. Call today for a free consultation. We are a local Akron, Ohio company that services all of Northeast Ohio. We have affordable cash plans available and work with all insurance companies. Luxury Roofs is what we do. We handle everything so you don't have too. Call Ohio's Top Rated Roofing Company today for a no obligation free consultation.\"", "logo": "https://www.tkroofingandgutters.com/hs-fs/hubfs/TK6.png?width=400&name=TK6.png", "name": "TK ROOFING AND GUTTERS", "contactPoint": "https://www.tkroofingandgutters.com/#ContactPoint", "url": "https://www.tkroofingandgutters.com/", "@id": "https://www.tkroofingandgutters.com/#Organization" }, "mainEntityOfPage": { "@id": "https://www.tkroofingandgutters.com/blog/how-is-technology-changing-the-roofing-industry" }, "video": { "@type": "VideoObject", "duration": "P0Y0M0DT0H3M15S", "contentUrl": "https://www.youtube.com/watch?v=JaRa8Zmy2CQ&t=69s", "description": "Technology has become an essential part of our lives. We don't go anywhere without a supercomputer in the form of a smartphone in our pockets, and there's not a day that goes by when we aren't in front of some type of computer. Siri and Alexa have made our lives easier, and we rely on innovation and new technologies now more than we ever have. And, it's not just our personal lives that our evolving just because of technologies. Technology is also revolutionizing entire industries, and the roofing industry is no exception. Roofing professionals have been receptive to different methods of performing their tired and true procedures, allowing technology to enhance roofing businesses across the country. Technology is making the job of a roofer much safer and more efficient, and it saves companies and their customer's money and time. In this video, we will look at some innovations that are revolutionizing the roofing industry.", "name": "How Is Technology Changing The Roofing Industry", "embedUrl": "https://www.youtube.com/embed/JaRa8Zmy2CQ", "thumbnailUrl": "https://www.tkroofingandgutters.com/hs-fs/hubfs/drone.jpg?width=1200&name=drone.jpg", "uploadDate": "2020-12-09", "@id": "https://www.tkroofingandgutters.com/blog/how-is-technology-changing-the-roofing-industry#VideoObject" }, "@id": "https://www.tkroofingandgutters.com/blog/how-is-technology-changing-the-roofing-industry" }, { "@context": "http://schema.org", "@type": "WebPage", "isPartOf": { "@type": "WebSite", "url": "https://www.tkroofingandgutters.com/", "name": "TK Roofing and Gutters", "@id": "https://www.tkroofingandgutters.com/#WebSite" }, "about": [ "https://en.wikipedia.org/wiki/Domestic_roof_construction", "https://en.wikipedia.org/wiki/Roof", "https://en.wikipedia.org/wiki/Roofer" ], "url": "https://www.tkroofingandgutters.com/blog/how-is-technology-changing-the-roofing-industry", "name": "How Is Technology Changing The Roofing Industry", "headline": "How Technology Is Changing The Roofing Industry", "alternativeHeadline": "How The Roofing Industry Is Benefiting From Advances In Technology", "@id": "https://www.tkroofingandgutters.com/blog/how-is-technology-changing-the-roofing-industry#WebPage" } ] from Press Releases https://www.pressadvantage.com/story/40477-tk-roofing-announces-new-advancements-in-residential-roofing-industry
0 notes
Text
Future of Artificial Intelligence
What is Artificial Intelligence
Artificial Intelligence (AI) is the way to train computers and machines to behave like humans and the processes that would be done by these machines would be similar to that of a Human Brain. The term can also refer to the study, science, and engineering of such intelligent machines, systems, and programs.

Artificial Intelligence History
The term artificial intelligence was coined in 1956, but AI has become more popular today thanks to increased data volumes, advanced algorithms, and improvements in computing power and storage.
Problem solving and symbolic methods were the topics covered in the research in the early 1950s, the US Department of Defense took interest in this type of work and began training computers to mimic basic human reasoning. For example, the Defense Advanced Research Projects Agency (DARPA) completed street mapping projects in the 1970s. And DARPA produced intelligent personal assistants in 2003, long before Siri, Alexa or Cortana were household names.
This early work helped to make progress in automation and formal reasoning in computers that we see today including decision support systems and smart search systems that can be designed to complement and augment human abilities.
While Hollywood movies and science fiction novels depict AI as human-like robots that take over the world, the current evolution of AI technologies isn’t that scary – or quite that smart. Instead, AI has evolved to provide many specific benefits in every industry.

The various uses for AI and its implementation can be:
1. Natural Language Processing: The computers and machines are trained in such a way so that they can recognize human speech, translate them to machine commands and give back the output. Natural language processing has many use cases and can be implemented in Home/Building Automation devices, etc.

2. Computer Games: It involves programming in such a way so that machines and computers can compete with human. For e.g. in Chess, based on the moves of a human, the computer will identify the next move, with AI doing the back end processing.

3. Smart Toys/Robotics:The next generation smart toys would act as a bot to and respond to what the kid speaks. It would use image recognition technology so that it can hear and see just the way human can see.

4. Image Recognition: The best use case can be in the newest Connected Car where in the car can capture all the images surrounding it (a 360 deg view) and identify obstacles and process it in the back end so that the car avoids collision with these obstacles.
Stephen Hawking has said, “Every aspect of our lives will be transformed [by AI],” and it could be “the biggest event in the history of our civilization.”
6 ways AI will affect us in future
1. Automated Transportation
We’re already seeing the beginnings of self-driving cars, though the vehicles are currently required to have a driver present at the wheel for safety.
The beginning of self-driving cars has been started, but the vehicles are currently required to have a driver for safety reasons. Inspite of tremendous development,the technology is still getting perfect and it will take a while for public acceptance to bring automated cars into widespread use.Google began testing a self-driving car in 2012, and since then, the U.S. Department of Transportation has released definitions of different levels of automation, with Google’s car classified as the first level down from full automation. Other transportation methods are closer to full automation, such as buses and trains.
2. Cyborg Technology
One of the main limitations of being human is simply our own bodies—and brains. Researcher Shimon Whiteson thinks that in the future, we will be able to augment ourselves with computers and enhance many of our own natural abilities. Though many of these possible cyborg enhancements would be added for convenience, others might serve a more practical purpose. Yoky Matsuka of Nest believes that AI will become useful for people with amputated limbs, as the brain will be able to communicate with a robotic limb to give the patient more control. This kind of cyborg technology would significantly reduce the limitations that amputees deal with on a daily basis.
3. Taking over risky jobs
Robots are already taking over some of the most dangerous jobs available, including bomb defusing. These robots aren’t quite robots yet, according to the BBC. They are technically drones, being used as the physical counterpart for defusing bombs, but requiring a human to control them, rather than using AI. Whatever their classification, they have saved thousands of lives by taking over one of the most dangerous jobs in the world. As technology improves, we will likely see more AI integration to help these machines function.
Other jobs are also being reconsidered for robot integration. Welding, well known for producing toxic substances, intense heat, and earsplitting noise, can now be outsourced to robots in most cases. Robot Worx explains that robotic welding cells are already in use, and have safety features in place to help prevent human workers from fumes and other bodily harm.
4. Solving climate change
Solving climate change might seem like a tall order from a robot, but as Stuart Russell explains, machines have more access to data than one person ever could—storing a mind-boggling number of statistics. Using big data, AI could one day identify trends and use that information to come up with solutions to the world’s biggest problems.
5. Can Robots be friends?
Would you like to become friends with C-3PO? Robots are emotionless and it is hard to relate with them. A Japan company have taken steps towards robot companion i.e. one who can feel and understand emotions. Pepper-the first companion robot was introduced in 2014. He went on sale in 2015 with all 1,000 initials selling out within a minute.The robot was programmed to read human emotions, develop its own emotions, and help its human friends stay happy. Pepper goes on sale in the U.S. in 2016, and more sophisticated friendly robots are sure to follow.

6. Home Robots for Elderly People
For many elderly person, simple everyday task is a struggle, so they have to hire people for their care or rely on their family member.AI would be beneficial for such elderly person. Home robots would help them to do everyday tasks and allow them to be independent, which would improve their well-being.
Although we don’t know the exact future, it is quite evident that interacting with AI will soon become an everyday activity. These interactions will clearly help our society evolve, particularly in regards to automated transportation, cyborgs, handling dangerous duties, solving climate change, friendships and improving the care of our elders. Beyond these six impacts, there are even more ways that AI technology can influence our future, and this very fact has professionals across multiple industries extremely excited for the ever-burgeoning future of artificial intelligence.
7. Your Face Will Become Your ID
We’re already seeing biometric incorporated into our daily lives and that technology is expected to evolve. Eventually, many in the tech industry anticipate AI-driven applications allowing machines to recognize your face to complete transactions. Your credit cards and driver’s license may be linked to your face, allowing pattern recognition devices to know you instantly. This can make everyday transactions far more efficient, saving us from having to wait in line at the store, bank, or movie theater.

8. Receive Better Medical Care
Research is already underway to develop new software applications that use AI to help doctors diagnose and treat patients. It won’t be long before wearable devices can measure blood sugar levels for diabetics and transmit that data to the patient’s doctor. Already, devices are in use that measure heart rate, respiration, and other vital functions. Artificial intelligence may also help patients better understand their care options and communicate more effectively with their caregivers.
0 notes
Text
You created a machine learning application. Now make sure it’s secure.

The software industry has demonstrated, all too clearly, what happens when you don’t pay attention to security.
In a recent post, we described what it would take to build a sustainable machine learning practice. By “sustainable,” we mean projects that aren’t just proofs of concepts or experiments. A sustainable practice means projects that are integral to an organization’s mission: projects by which an organization lives or dies. These projects are built and supported by a stable team of engineers, and supported by a management team that understands what machine learning is, why it’s important, and what it’s capable of accomplishing. Finally, sustainable machine learning means that as many aspects of product development as possible are automated: not just building models, but cleaning data, building and managing data pipelines, testing, and much more. Machine learning will penetrate our organizations so deeply that it won’t be possible for humans to manage them unassisted.
Organizations throughout the world are waking up to the fact that security is essential to their software projects. Nobody wants to be the next Sony, the next Anthem, or the next Equifax. But while we know how to make traditional software more secure (even though we frequently don’t), machine learning presents a new set of problems. Any sustainable machine learning practice must address machine learning’s unique security issues. We didn’t do that for traditional software, and we’re paying the price now. Nobody wants to pay the price again. If we learn one thing from traditional software’s approach to security, it’s that we need to be ahead of the curve, not behind it. As Joanna Bryson writes, “Cyber security and AI are inseparable.”
The presence of machine learning in any organization won’t be a single application, a single model; it will be many applications, using many models—perhaps thousands of models, or tens of thousands, automatically generated and updated. Machine learning on low-power edge devices, ranging from phones to tiny sensors embedded in assembly lines, tools, appliances, and even furniture and building structures, increases the number of models that need to be monitored. And the advent of 5G mobile services, which significantly increases the network bandwidth to mobile devices, will make it much more attractive to put machine learning at the edge of the network. We anticipate billions of machines, each of which may be running dozens of models. At this scale, we can't assume that we can deal with security issues manually. We need tools to assist the humans responsible for security. We need to automate as much of the process as possible, but not too much, giving humans the final say.
In “Lessons learned turning machine learning models into real products and services,” David Talby writes that “the biggest mistake people make with regard to machine learning is thinking that the models are just like any other type of software.” Model development isn’t software development. Models are unique—the same model can’t be deployed twice; the accuracy of any model degrades as soon as it is put into production; and the gap between training data and live data, representing real users and their actions, is huge. In many respects, the task of modeling doesn’t get started until the model hits production, and starts to encounter real-world data.
Unfortunately, one characteristic that software development has in common with machine learning is a lack of attention to security. Security tends to be a low priority. It gets some lip service, but falls out of the picture when deadlines get tight. In software, that’s been institutionalized in the “move fast and break things” mindset. If you’re building fast, you’re not going to take the time to write sanitary code, let alone think about attack vectors. You might not “break things,” but you’re willing to build broken things; the benefits of delivering insecure products on time outweigh the downsides, as Daniel Miessler has written. You might be lucky; the vulnerabilities you create may never be discovered. But if security experts aren’t part of the development team from the beginning, if security is something to be added on at the last minute, you’re relying on luck, and that’s not a good position to be in. Machine learning is no different, except that the pressure of delivering a product on time is even greater, the issues aren’t as well understood, the attack surface is larger, the targets are more valuable, and companies building machine learning products haven’t yet engaged with the problems.
What kinds of attacks will machine learning systems see, and what will they have to defend against? All of the attacks we have been struggling with for years, but there are a number of vulnerabilities that are specific to machine learning. Here’s a brief taxonomy of attacks against machine learning:
Poisoning, or injecting bad (“adversarial”) data into the training data. We’ve seen this many times in the wild. Microsoft’s Tay was an experimental chatbot that was quickly taught to spout racist and anti-semitic messages by the people who were chatting with it. By inserting racist content into the data stream, they effectively gained control over Tay’s behavior. The appearance of “fake news” in channels like YouTube, Facebook, Twitter, and even Google searches, was similar: once fake news was posted, users were attracted to it like flies, and the algorithms that made recommendations “learned” to recommend that content. danah boyd has argued that these incidents need to be treated as security issues, intentional and malicious corruption of the data feeding the application, not as isolated pranks or algorithmic errors.
Any machine learning system that constantly trains itself is vulnerable to poisoning. Such applications could range from customer service chat bots (can you imagine a call center bot behaving like Tay?) to recommendation engines (real estate redlining might be a consequence) or even to medical diagnosis (modifying recommended drug dosages). To defend against poisoning, you need strong control over the training data. Such control is difficult (if not impossible) to achieve. “Black hat SEO” to improve search engine rankings is nothing if not an early (and still very present) example of poisoning. Google can’t control the incoming data, which is everything that is on the web. Their only recourse is to tweak their search algorithms constantly and penalize abusers for their behavior. In the same vein, bots and troll armies have manipulated social media feeds to spread views ranging from opposition to vaccination to neo-naziism.
Evasion, or crafting input that causes a machine learning system to misclassify it. Again, we’ve seen this both in the wild and in the lab. CV Dazzle uses makeup and hair styles as “camouflage against face recognition technology.” Other research projects have shown that it’s possible to defeat image classification by changing a single pixel in an image: a ship becomes a car, a horse becomes a frog. Or, just as with humans, image classifiers can miss an unexpected object that’s out of context: an elephant in the room, for example. It’s a mistake to think that computer vision systems “understand” what they see in ways that are similar to humans. They’re not aware of context, they don’t have expectations about what’s normal; they’re simply doing high-stakes pattern matching. Researchers have reported similar vulnerabilities in natural language processing, where changing a word, or even a letter, in a way that wouldn’t confuse human researchers causes machine learning to misunderstand a phrase.
Although these examples are often amusing, it’s worth thinking about real-world consequences: could someone use these tricks to manipulate the behavior of autonomous vehicles? Here’s how that could work: I put a mark on a stop sign—perhaps by sticking a fragment of a green sticky note at the top. Does that make an autonomous vehicle think the stop sign is a flying tomato, and if so, would the car stop? The alteration doesn’t have to make the sign “look like” a tomato to a human observer; it just has to push the image closer to the boundary where the model says “tomato.” Machine learning has neither the context nor the common sense to understand that tomatoes don’t appear in mid-air. Could a delivery drone be subverted to become a weapon by causing it to misunderstand its surroundings? Almost certainly. Don’t dismiss these examples as academic. A stop sign with a few pixels changed in the lab may not be different from a stop sign that has been used for target practice during hunting season.
Impersonation attacks attempt to fool a model into misidentifying someone or something. The goal is frequently to gain unauthorized access to a system. For example, an attacker might want to trick a bank into misreading the amount written on a check. Fingerprints obtained from drinking glasses, or even high resolution photographs, can be used to fool fingerprint authentication. South Park trolled Alexa and Google Home users by using the words “Alexa” and “OK Google” repeatedly in an episode, triggering viewers’ devices; the devices weren’t able to distinguish between the show voices and real ones. The next generation of impersonation attacks will be “deep fake” videos that place words in the mouths of real people.
Inversion means using an API to gather information about a model, and using that information to attack it. Inversion can also mean using an API to obtain private information from a model, perhaps by retrieving data and de-anonymizing it. In “The Secret Sharer: Measuring Unintended Neural Network Memorization & Extracting Secrets,” the authors show that machine learning models tend to memorize all their training data, and that it’s possible to extract protected information from a model. Common approaches to protecting information don’t work; the model still incorporates secret information in ways that can be extracted. Differential privacy—the practice of carefully inserting extraneous data into a data set in ways that don’t change its statistical properties—has some promise, but with significant cost: the authors point out that training is much slower. Furthermore, the number of developers who understand and can implement differential privacy is small.
While this may sound like an academic concern, it’s not; writing a script to probe machine learning applications isn’t difficult. Furthermore, Michael Veale and others write that inversion attacks raise legal problems. Under the GDPR, if protected data is memorized by models, are those models subject to the same regulations as personal data? In that case, developers would have to remove personal data from models—not just the training data sets—on request; it would be very difficult to sell products that incorporated models, and even techniques like automated model generation could become problematic. Again, the authors point to differential privacy, but with the caution that few companies have the expertise to deploy models with differential privacy correctly.
Other vulnerabilities, other attacks
This brief taxonomy of vulnerabilities doesn’t come close to listing all the problems that machine learning will face in the field. Many of these vulnerabilities are easily exploited. You can probe Amazon to find out what products are recommended along with your products, possibly finding out who your real competitors are, and discovering who to attack. You might even be able to reverse-engineer how Amazon makes recommendations and use that knowledge to influence the recommendations they make.
More complex attacks have been seen in the field. One involves placing fake reviews on an Amazon seller’s site, so that when the seller removes the reviews, Amazon bans the seller for review manipulation. Is this an attack against machine learning? The attacker tricks the human victim into violating Amazon’s rules. Ultimately, though, it’s the machine learning system that’s tricked into taking an incorrect action (banning the victim) that it could have prevented.
“Google bowling” means creating large numbers of links to a competitor’s website in hopes that Google’s ranking algorithm will penalize the competitor for purchasing bulk links. It’s similar to the fake review attack, except that it doesn’t require a human intermediary; it’s a direct attack against the algorithm that analyzes inbound links.
Advertising was one of the earliest adopters of machine learning, and one of the earliest victims. Click fraud is out of control, and the machine learning community is reluctant to talk about (or is unaware of) the issue—even though, as online advertising becomes ever more dependent on machine learning, fraudsters will learn how to attack models directly in their attempts to appear legitimate. If click data is unreliable, then models built from that data are unreliable, along with any results or recommendations generated by those models. And click fraud is similar to many attacks against recommendation systems and trend analysis. Once a “fake news” item has been planted, it’s simple to make it trend with some automated clicks. At that point, the recommendation takes over, generating recommendations which in turn generate further clicks. Anything automated is prone to attack, and automation allows those attacks to take place at scale.
The advent of autonomous vehicles, ranging from cars to drones, presents yet another set of threats. If the machine learning systems on an autonomous vehicle are vulnerable to attack, a car or truck could conceivably be used as a murder weapon. So could a drone—either a weaponized military drone or a consumer drone. The military already knows that drones are vulnerable; in 2011, Iran captured a U.S. drone, possibly by spoofing GPS signals. We expect to see attacks on “smart” consumer health devices and professional medical devices, many of which we know are already vulnerable.
Taking action
Merely scolding and thinking about possible attacks won’t help. What can be done to defend machine learning models? First, we can start with traditional software. The biggest problem with insecure software isn’t that we don’t understand security; it’s that software vendors, and software users, never take the basic steps they would need to defend themselves. It’s easy to feel defenseless before hyper-intelligent hackers, but the reality is that sites like Equifax become victims because they didn’t take basic precautions, such as installing software updates. So, what do machine learning developers need to do?
Security audits are a good starting point. What are the assets that you need to protect? Where are they, and how vulnerable are they? Who has access to those resources, and who actually needs that access? How can you minimize access to critical data? For example, a shipping system needs customer addresses, but it doesn’t need credit card information; a payment system needs credit card information, but not complete purchase histories. Can this data be stored and managed in separate, isolated databases? Beyond that, are basic safeguards in place, such as two-factor authentication? It’s easy to fault Equifax for not updating their software, but almost any software system depends on hundreds, if not thousands, of external libraries. What strategy do you have in place to ensure they’re updated, and that updates don't break working systems?
Like conventional software, machine learning systems should use monitoring systems that generate alerts to notify staff when something abnormal or suspicious occurs. Some of these monitoring systems are already using machine learning for anomaly detection—which means the monitoring software itself can be attacked.
Penetration testing is a common practice in the online world: your security staff (or, better, consultants) attack your site to discover its vulnerabilities. Attack simulation is an extension of penetration testing that shows you “how attackers actually achieve goals against your organization.” What are they looking for? How do they get to it? Can you gain control over a system by poisoning its inputs?
Tools for testing computer vision systems by generating "adversarial images" are already appearing, such as cleverhans and IBM’s ART. We are starting to see papers describing adversarial attacks against speech recognition systems. Adversarial input is a special case of a more general problem. Most machine learning developers assume their training data is similar to the data their systems will face in the real world. That’s an idealized best case. It’s easy to build a face identification system if all your faces are well-lit, well-focused, and have light-skinned subjects. A working system needs to handle all kinds of images, including images that are blurry, badly focused, poorly lighted—and have dark-skinned subjects.
Safety verification is a new area for AI research, still in its infancy. Safety verification asks questions like whether models can deliver consistent results, or whether small changes in the input lead to large changes in the output. If machine learning is at all like conventional software, we expect an escalating struggle between attackers and defenders; better defenses will lead to more sophisticated attacks, which will lead to a new generation of defenses. It will never be possible to say that a model has been “verifiably safe.” But it is important to know that a model has been tested, and that it is reasonably well-behaved against all known attacks.
Model explainability has become an important area of research in machine learning. Understanding why a model makes specific decisions is important for several reasons, not the least of which is that it makes people more comfortable with using machine learning. That “comfort” can be deceptive, of course. But being able to ask models why they made particular decisions will conceivably make it easier to see when they’ve been compromised. During development, explainability will make it possible to test how easy it is for an adversary to manipulate a model, in applications from image classification to credit scoring. In addition to knowing what a model does, explainability will tell us why, and help us build models that are more robust, less subject to manipulation; understanding why a model makes decisions should help us understand its limitations and weaknesses. At the same time, it’s conceivable that explainability will make it easier to discover weaknesses and attack vectors. If you want to poison the data flowing into a model, it can only help to know how the model responds to data.
In “Deep Automation in Machine Learning,” we talked about the importance of data lineage and provenance, and tools for tracking them. Lineage and provenance are important whether or not you’re developing the model yourself. While there are many cloud platforms to automate model building and even deployment, ultimately your organization is responsible for the model’s behavior. The downside of that responsibility includes everything from degraded profits to legal liability. If you don’t know where your data is coming from and how it has been modified, you have no basis for knowing whether your data has been corrupted, either through accident or malice.
“Datasheets for Datasets” proposes a standard set of questions about a data set’s sources, how the data was collected, its biases, and other basic information. Given a specification that records a data set’s properties, it should be easy to test and detect sudden and unexpected changes. If an attacker corrupts your data, you should be able to detect that and correct it up front; if not up front, then later in an audit.
Datasheets are a good start, but they are only a beginning. Whatever tools we have for tracking data lineage and provenance need to be automated. There will be too many models and data sets to rely on manual tracking and audits.
Balancing openness against tipping off adversaries
In certain domains, users and regulators will increasingly prefer machine learning services and products that can provide simple explanations for how automated decisions and recommendations are being made. But we’ve already seen that too much information can lead to certain parties gaming models (as in SEO). How much to disclose depends on the specific application, domain, and jurisdiction.
This balancing act is starting to come up in machine learning and related areas that involve the work of researchers (who tend to work in the open) who are up against adversaries who prize unpublished vulnerabilities. The question of whether or not to “temporarily hold back” research results is a discussion that the digital media forensics community has been having. In a 2018
from FEED 10 TECHNOLOGY https://ift.tt/2Vrb0Ym
0 notes
Text
You created a machine learning application. Now make sure it’s secure.
You created a machine learning application. Now make sure it’s secure.

The software industry has demonstrated, all too clearly, what happens when you don’t pay attention to security.
In a recent post, we described what it would take to build a sustainable machine learning practice. By “sustainable,” we mean projects that aren’t just proofs of concepts or experiments. A sustainable practice means projects that are integral to an organization’s mission: projects by which an organization lives or dies. These projects are built and supported by a stable team of engineers, and supported by a management team that understands what machine learning is, why it’s important, and what it’s capable of accomplishing. Finally, sustainable machine learning means that as many aspects of product development as possible are automated: not just building models, but cleaning data, building and managing data pipelines, testing, and much more. Machine learning will penetrate our organizations so deeply that it won’t be possible for humans to manage them unassisted.
Organizations throughout the world are waking up to the fact that security is essential to their software projects. Nobody wants to be the next Sony, the next Anthem, or the next Equifax. But while we know how to make traditional software more secure (even though we frequently don’t), machine learning presents a new set of problems. Any sustainable machine learning practice must address machine learning’s unique security issues. We didn’t do that for traditional software, and we’re paying the price now. Nobody wants to pay the price again. If we learn one thing from traditional software’s approach to security, it’s that we need to be ahead of the curve, not behind it. As Joanna Bryson writes, “Cyber security and AI are inseparable.”
The presence of machine learning in any organization won’t be a single application, a single model; it will be many applications, using many models—perhaps thousands of models, or tens of thousands, automatically generated and updated. Machine learning on low-power edge devices, ranging from phones to tiny sensors embedded in assembly lines, tools, appliances, and even furniture and building structures, increases the number of models that need to be monitored. And the advent of 5G mobile services, which significantly increases the network bandwidth to mobile devices, will make it much more attractive to put machine learning at the edge of the network. We anticipate billions of machines, each of which may be running dozens of models. At this scale, we can't assume that we can deal with security issues manually. We need tools to assist the humans responsible for security. We need to automate as much of the process as possible, but not too much, giving humans the final say.
In “Lessons learned turning machine learning models into real products and services,” David Talby writes that “the biggest mistake people make with regard to machine learning is thinking that the models are just like any other type of software.” Model development isn’t software development. Models are unique—the same model can’t be deployed twice; the accuracy of any model degrades as soon as it is put into production; and the gap between training data and live data, representing real users and their actions, is huge. In many respects, the task of modeling doesn’t get started until the model hits production, and starts to encounter real-world data.
Unfortunately, one characteristic that software development has in common with machine learning is a lack of attention to security. Security tends to be a low priority. It gets some lip service, but falls out of the picture when deadlines get tight. In software, that’s been institutionalized in the “move fast and break things” mindset. If you’re building fast, you’re not going to take the time to write sanitary code, let alone think about attack vectors. You might not “break things,” but you’re willing to build broken things; the benefits of delivering insecure products on time outweigh the downsides, as Daniel Miessler has written. You might be lucky; the vulnerabilities you create may never be discovered. But if security experts aren’t part of the development team from the beginning, if security is something to be added on at the last minute, you’re relying on luck, and that’s not a good position to be in. Machine learning is no different, except that the pressure of delivering a product on time is even greater, the issues aren’t as well understood, the attack surface is larger, the targets are more valuable, and companies building machine learning products haven’t yet engaged with the problems.
What kinds of attacks will machine learning systems see, and what will they have to defend against? All of the attacks we have been struggling with for years, but there are a number of vulnerabilities that are specific to machine learning. Here’s a brief taxonomy of attacks against machine learning:
Poisoning, or injecting bad (“adversarial”) data into the training data. We’ve seen this many times in the wild. Microsoft’s Tay was an experimental chatbot that was quickly taught to spout racist and anti-semitic messages by the people who were chatting with it. By inserting racist content into the data stream, they effectively gained control over Tay’s behavior. The appearance of “fake news” in channels like YouTube, Facebook, Twitter, and even Google searches, was similar: once fake news was posted, users were attracted to it like flies, and the algorithms that made recommendations “learned” to recommend that content. danah boyd has argued that these incidents need to be treated as security issues, intentional and malicious corruption of the data feeding the application, not as isolated pranks or algorithmic errors.
Any machine learning system that constantly trains itself is vulnerable to poisoning. Such applications could range from customer service chat bots (can you imagine a call center bot behaving like Tay?) to recommendation engines (real estate redlining might be a consequence) or even to medical diagnosis (modifying recommended drug dosages). To defend against poisoning, you need strong control over the training data. Such control is difficult (if not impossible) to achieve. “Black hat SEO” to improve search engine rankings is nothing if not an early (and still very present) example of poisoning. Google can’t control the incoming data, which is everything that is on the web. Their only recourse is to tweak their search algorithms constantly and penalize abusers for their behavior. In the same vein, bots and troll armies have manipulated social media feeds to spread views ranging from opposition to vaccination to neo-naziism.
Evasion, or crafting input that causes a machine learning system to misclassify it. Again, we’ve seen this both in the wild and in the lab. CV Dazzle uses makeup and hair styles as “camouflage against face recognition technology.” Other research projects have shown that it’s possible to defeat image classification by changing a single pixel in an image: a ship becomes a car, a horse becomes a frog. Or, just as with humans, image classifiers can miss an unexpected object that’s out of context: an elephant in the room, for example. It’s a mistake to think that computer vision systems “understand” what they see in ways that are similar to humans. They’re not aware of context, they don’t have expectations about what’s normal; they’re simply doing high-stakes pattern matching. Researchers have reported similar vulnerabilities in natural language processing, where changing a word, or even a letter, in a way that wouldn’t confuse human researchers causes machine learning to misunderstand a phrase.
Although these examples are often amusing, it’s worth thinking about real-world consequences: could someone use these tricks to manipulate the behavior of autonomous vehicles? Here’s how that could work: I put a mark on a stop sign—perhaps by sticking a fragment of a green sticky note at the top. Does that make an autonomous vehicle think the stop sign is a flying tomato, and if so, would the car stop? The alteration doesn’t have to make the sign “look like” a tomato to a human observer; it just has to push the image closer to the boundary where the model says “tomato.” Machine learning has neither the context nor the common sense to understand that tomatoes don’t appear in mid-air. Could a delivery drone be subverted to become a weapon by causing it to misunderstand its surroundings? Almost certainly. Don’t dismiss these examples as academic. A stop sign with a few pixels changed in the lab may not be different from a stop sign that has been used for target practice during hunting season.
Impersonation attacks attempt to fool a model into misidentifying someone or something. The goal is frequently to gain unauthorized access to a system. For example, an attacker might want to trick a bank into misreading the amount written on a check. Fingerprints obtained from drinking glasses, or even high resolution photographs, can be used to fool fingerprint authentication. South Park trolled Alexa and Google Home users by using the words “Alexa” and “OK Google” repeatedly in an episode, triggering viewers’ devices; the devices weren’t able to distinguish between the show voices and real ones. The next generation of impersonation attacks will be “deep fake” videos that place words in the mouths of real people.
Inversion means using an API to gather information about a model, and using that information to attack it. Inversion can also mean using an API to obtain private information from a model, perhaps by retrieving data and de-anonymizing it. In “The Secret Sharer: Measuring Unintended Neural Network Memorization & Extracting Secrets,” the authors show that machine learning models tend to memorize all their training data, and that it’s possible to extract protected information from a model. Common approaches to protecting information don’t work; the model still incorporates secret information in ways that can be extracted. Differential privacy—the practice of carefully inserting extraneous data into a data set in ways that don’t change its statistical properties—has some promise, but with significant cost: the authors point out that training is much slower. Furthermore, the number of developers who understand and can implement differential privacy is small.
While this may sound like an academic concern, it’s not; writing a script to probe machine learning applications isn’t difficult. Furthermore, Michael Veale and others write that inversion attacks raise legal problems. Under the GDPR, if protected data is memorized by models, are those models subject to the same regulations as personal data? In that case, developers would have to remove personal data from models—not just the training data sets—on request; it would be very difficult to sell products that incorporated models, and even techniques like automated model generation could become problematic. Again, the authors point to differential privacy, but with the caution that few companies have the expertise to deploy models with differential privacy correctly.
Other vulnerabilities, other attacks
This brief taxonomy of vulnerabilities doesn’t come close to listing all the problems that machine learning will face in the field. Many of these vulnerabilities are easily exploited. You can probe Amazon to find out what products are recommended along with your products, possibly finding out who your real competitors are, and discovering who to attack. You might even be able to reverse-engineer how Amazon makes recommendations and use that knowledge to influence the recommendations they make.
More complex attacks have been seen in the field. One involves placing fake reviews on an Amazon seller’s site, so that when the seller removes the reviews, Amazon bans the seller for review manipulation. Is this an attack against machine learning? The attacker tricks the human victim into violating Amazon’s rules. Ultimately, though, it’s the machine learning system that’s tricked into taking an incorrect action (banning the victim) that it could have prevented.
“Google bowling” means creating large numbers of links to a competitor’s website in hopes that Google’s ranking algorithm will penalize the competitor for purchasing bulk links. It’s similar to the fake review attack, except that it doesn’t require a human intermediary; it’s a direct attack against the algorithm that analyzes inbound links.
Advertising was one of the earliest adopters of machine learning, and one of the earliest victims. Click fraud is out of control, and the machine learning community is reluctant to talk about (or is unaware of) the issue—even though, as online advertising becomes ever more dependent on machine learning, fraudsters will learn how to attack models directly in their attempts to appear legitimate. If click data is unreliable, then models built from that data are unreliable, along with any results or recommendations generated by those models. And click fraud is similar to many attacks against recommendation systems and trend analysis. Once a “fake news” item has been planted, it’s simple to make it trend with some automated clicks. At that point, the recommendation takes over, generating recommendations which in turn generate further clicks. Anything automated is prone to attack, and automation allows those attacks to take place at scale.
The advent of autonomous vehicles, ranging from cars to drones, presents yet another set of threats. If the machine learning systems on an autonomous vehicle are vulnerable to attack, a car or truck could conceivably be used as a murder weapon. So could a drone—either a weaponized military drone or a consumer drone. The military already knows that drones are vulnerable; in 2011, Iran captured a U.S. drone, possibly by spoofing GPS signals. We expect to see attacks on “smart” consumer health devices and professional medical devices, many of which we know are already vulnerable.
Taking action
Merely scolding and thinking about possible attacks won’t help. What can be done to defend machine learning models? First, we can start with traditional software. The biggest problem with insecure software isn’t that we don’t understand security; it’s that software vendors, and software users, never take the basic steps they would need to defend themselves. It’s easy to feel defenseless before hyper-intelligent hackers, but the reality is that sites like Equifax become victims because they didn’t take basic precautions, such as installing software updates. So, what do machine learning developers need to do?
Security audits are a good starting point. What are the assets that you need to protect? Where are they, and how vulnerable are they? Who has access to those resources, and who actually needs that access? How can you minimize access to critical data? For example, a shipping system needs customer addresses, but it doesn’t need credit card information; a payment system needs credit card information, but not complete purchase histories. Can this data be stored and managed in separate, isolated databases? Beyond that, are basic safeguards in place, such as two-factor authentication? It’s easy to fault Equifax for not updating their software, but almost any software system depends on hundreds, if not thousands, of external libraries. What strategy do you have in place to ensure they’re updated, and that updates don't break working systems?
Like conventional software, machine learning systems should use monitoring systems that generate alerts to notify staff when something abnormal or suspicious occurs. Some of these monitoring systems are already using machine learning for anomaly detection—which means the monitoring software itself can be attacked.
Penetration testing is a common practice in the online world: your security staff (or, better, consultants) attack your site to discover its vulnerabilities. Attack simulation is an extension of penetration testing that shows you “how attackers actually achieve goals against your organization.” What are they looking for? How do they get to it? Can you gain control over a system by poisoning its inputs?
Tools for testing computer vision systems by generating "adversarial images" are already appearing, such as cleverhans and IBM’s ART. We are starting to see papers describing adversarial attacks against speech recognition systems. Adversarial input is a special case of a more general problem. Most machine learning developers assume their training data is similar to the data their systems will face in the real world. That’s an idealized best case. It’s easy to build a face identification system if all your faces are well-lit, well-focused, and have light-skinned subjects. A working system needs to handle all kinds of images, including images that are blurry, badly focused, poorly lighted—and have dark-skinned subjects.
Safety verification is a new area for AI research, still in its infancy. Safety verification asks questions like whether models can deliver consistent results, or whether small changes in the input lead to large changes in the output. If machine learning is at all like conventional software, we expect an escalating struggle between attackers and defenders; better defenses will lead to more sophisticated attacks, which will lead to a new generation of defenses. It will never be possible to say that a model has been “verifiably safe.” But it is important to know that a model has been tested, and that it is reasonably well-behaved against all known attacks.
Model explainability has become an important area of research in machine learning. Understanding why a model makes specific decisions is important for several reasons, not the least of which is that it makes people more comfortable with using machine learning. That “comfort” can be deceptive, of course. But being able to ask models why they made particular decisions will conceivably make it easier to see when they’ve been compromised. During development, explainability will make it possible to test how easy it is for an adversary to manipulate a model, in applications from image classification to credit scoring. In addition to knowing what a model does, explainability will tell us why, and help us build models that are more robust, less subject to manipulation; understanding why a model makes decisions should help us understand its limitations and weaknesses. At the same time, it’s conceivable that explainability will make it easier to discover weaknesses and attack vectors. If you want to poison the data flowing into a model, it can only help to know how the model responds to data.
In “Deep Automation in Machine Learning,” we talked about the importance of data lineage and provenance, and tools for tracking them. Lineage and provenance are important whether or not you’re developing the model yourself. While there are many cloud platforms to automate model building and even deployment, ultimately your organization is responsible for the model’s behavior. The downside of that responsibility includes everything from degraded profits to legal liability. If you don’t know where your data is coming from and how it has been modified, you have no basis for knowing whether your data has been corrupted, either through accident or malice.
“Datasheets for Datasets” proposes a standard set of questions about a data set’s sources, how the data was collected, its biases, and other basic information. Given a specification that records a data set’s properties, it should be easy to test and detect sudden and unexpected changes. If an attacker corrupts your data, you should be able to detect that and correct it up front; if not up front, then later in an audit.
Datasheets are a good start, but they are only a beginning. Whatever tools we have for tracking data lineage and provenance need to be automated. There will be too many models and data sets to rely on manual tracking and audits.
Balancing openness against tipping off adversaries
In certain domains, users and regulators will increasingly prefer machine learning services and products that can provide simple explanations for how automated decisions and recommendations are being made. But we’ve already seen that too much information can lead to certain parties gaming models (as in SEO). How much to disclose depends on the specific application, domain, and jurisdiction.
This balancing act is starting to come up in machine learning and related areas that involve the work of researchers (who tend to work in the open) who are up against adversaries who prize unpublished vulnerabilities. The question of whether or not to “temporarily hold back” research results is a discussion that the digital media forensics community has been having. In a 2018
https://ift.tt/2Vrb0Ym
0 notes
Text
You created a machine learning application. Now make sure it’s secure.
You created a machine learning application. Now make sure it’s secure.

The software industry has demonstrated, all too clearly, what happens when you don’t pay attention to security.
In a recent post, we described what it would take to build a sustainable machine learning practice. By “sustainable,” we mean projects that aren’t just proofs of concepts or experiments. A sustainable practice means projects that are integral to an organization’s mission: projects by which an organization lives or dies. These projects are built and supported by a stable team of engineers, and supported by a management team that understands what machine learning is, why it’s important, and what it’s capable of accomplishing. Finally, sustainable machine learning means that as many aspects of product development as possible are automated: not just building models, but cleaning data, building and managing data pipelines, testing, and much more. Machine learning will penetrate our organizations so deeply that it won’t be possible for humans to manage them unassisted.
Organizations throughout the world are waking up to the fact that security is essential to their software projects. Nobody wants to be the next Sony, the next Anthem, or the next Equifax. But while we know how to make traditional software more secure (even though we frequently don’t), machine learning presents a new set of problems. Any sustainable machine learning practice must address machine learning’s unique security issues. We didn’t do that for traditional software, and we’re paying the price now. Nobody wants to pay the price again. If we learn one thing from traditional software’s approach to security, it’s that we need to be ahead of the curve, not behind it. As Joanna Bryson writes, “Cyber security and AI are inseparable.”
The presence of machine learning in any organization won’t be a single application, a single model; it will be many applications, using many models—perhaps thousands of models, or tens of thousands, automatically generated and updated. Machine learning on low-power edge devices, ranging from phones to tiny sensors embedded in assembly lines, tools, appliances, and even furniture and building structures, increases the number of models that need to be monitored. And the advent of 5G mobile services, which significantly increases the network bandwidth to mobile devices, will make it much more attractive to put machine learning at the edge of the network. We anticipate billions of machines, each of which may be running dozens of models. At this scale, we can't assume that we can deal with security issues manually. We need tools to assist the humans responsible for security. We need to automate as much of the process as possible, but not too much, giving humans the final say.
In “Lessons learned turning machine learning models into real products and services,” David Talby writes that “the biggest mistake people make with regard to machine learning is thinking that the models are just like any other type of software.” Model development isn’t software development. Models are unique—the same model can’t be deployed twice; the accuracy of any model degrades as soon as it is put into production; and the gap between training data and live data, representing real users and their actions, is huge. In many respects, the task of modeling doesn’t get started until the model hits production, and starts to encounter real-world data.
Unfortunately, one characteristic that software development has in common with machine learning is a lack of attention to security. Security tends to be a low priority. It gets some lip service, but falls out of the picture when deadlines get tight. In software, that’s been institutionalized in the “move fast and break things” mindset. If you’re building fast, you’re not going to take the time to write sanitary code, let alone think about attack vectors. You might not “break things,” but you’re willing to build broken things; the benefits of delivering insecure products on time outweigh the downsides, as Daniel Miessler has written. You might be lucky; the vulnerabilities you create may never be discovered. But if security experts aren’t part of the development team from the beginning, if security is something to be added on at the last minute, you’re relying on luck, and that’s not a good position to be in. Machine learning is no different, except that the pressure of delivering a product on time is even greater, the issues aren’t as well understood, the attack surface is larger, the targets are more valuable, and companies building machine learning products haven’t yet engaged with the problems.
What kinds of attacks will machine learning systems see, and what will they have to defend against? All of the attacks we have been struggling with for years, but there are a number of vulnerabilities that are specific to machine learning. Here’s a brief taxonomy of attacks against machine learning:
Poisoning, or injecting bad (“adversarial”) data into the training data. We’ve seen this many times in the wild. Microsoft’s Tay was an experimental chatbot that was quickly taught to spout racist and anti-semitic messages by the people who were chatting with it. By inserting racist content into the data stream, they effectively gained control over Tay’s behavior. The appearance of “fake news” in channels like YouTube, Facebook, Twitter, and even Google searches, was similar: once fake news was posted, users were attracted to it like flies, and the algorithms that made recommendations “learned” to recommend that content. danah boyd has argued that these incidents need to be treated as security issues, intentional and malicious corruption of the data feeding the application, not as isolated pranks or algorithmic errors.
Any machine learning system that constantly trains itself is vulnerable to poisoning. Such applications could range from customer service chat bots (can you imagine a call center bot behaving like Tay?) to recommendation engines (real estate redlining might be a consequence) or even to medical diagnosis (modifying recommended drug dosages). To defend against poisoning, you need strong control over the training data. Such control is difficult (if not impossible) to achieve. “Black hat SEO” to improve search engine rankings is nothing if not an early (and still very present) example of poisoning. Google can’t control the incoming data, which is everything that is on the web. Their only recourse is to tweak their search algorithms constantly and penalize abusers for their behavior. In the same vein, bots and troll armies have manipulated social media feeds to spread views ranging from opposition to vaccination to neo-naziism.
Evasion, or crafting input that causes a machine learning system to misclassify it. Again, we’ve seen this both in the wild and in the lab. CV Dazzle uses makeup and hair styles as “camouflage against face recognition technology.” Other research projects have shown that it’s possible to defeat image classification by changing a single pixel in an image: a ship becomes a car, a horse becomes a frog. Or, just as with humans, image classifiers can miss an unexpected object that’s out of context: an elephant in the room, for example. It’s a mistake to think that computer vision systems “understand” what they see in ways that are similar to humans. They’re not aware of context, they don’t have expectations about what’s normal; they’re simply doing high-stakes pattern matching. Researchers have reported similar vulnerabilities in natural language processing, where changing a word, or even a letter, in a way that wouldn’t confuse human researchers causes machine learning to misunderstand a phrase.
Although these examples are often amusing, it’s worth thinking about real-world consequences: could someone use these tricks to manipulate the behavior of autonomous vehicles? Here’s how that could work: I put a mark on a stop sign—perhaps by sticking a fragment of a green sticky note at the top. Does that make an autonomous vehicle think the stop sign is a flying tomato, and if so, would the car stop? The alteration doesn’t have to make the sign “look like” a tomato to a human observer; it just has to push the image closer to the boundary where the model says “tomato.” Machine learning has neither the context nor the common sense to understand that tomatoes don’t appear in mid-air. Could a delivery drone be subverted to become a weapon by causing it to misunderstand its surroundings? Almost certainly. Don’t dismiss these examples as academic. A stop sign with a few pixels changed in the lab may not be different from a stop sign that has been used for target practice during hunting season.
Impersonation attacks attempt to fool a model into misidentifying someone or something. The goal is frequently to gain unauthorized access to a system. For example, an attacker might want to trick a bank into misreading the amount written on a check. Fingerprints obtained from drinking glasses, or even high resolution photographs, can be used to fool fingerprint authentication. South Park trolled Alexa and Google Home users by using the words “Alexa” and “OK Google” repeatedly in an episode, triggering viewers’ devices; the devices weren’t able to distinguish between the show voices and real ones. The next generation of impersonation attacks will be “deep fake” videos that place words in the mouths of real people.
Inversion means using an API to gather information about a model, and using that information to attack it. Inversion can also mean using an API to obtain private information from a model, perhaps by retrieving data and de-anonymizing it. In “The Secret Sharer: Measuring Unintended Neural Network Memorization & Extracting Secrets,” the authors show that machine learning models tend to memorize all their training data, and that it’s possible to extract protected information from a model. Common approaches to protecting information don’t work; the model still incorporates secret information in ways that can be extracted. Differential privacy—the practice of carefully inserting extraneous data into a data set in ways that don’t change its statistical properties—has some promise, but with significant cost: the authors point out that training is much slower. Furthermore, the number of developers who understand and can implement differential privacy is small.
While this may sound like an academic concern, it’s not; writing a script to probe machine learning applications isn’t difficult. Furthermore, Michael Veale and others write that inversion attacks raise legal problems. Under the GDPR, if protected data is memorized by models, are those models subject to the same regulations as personal data? In that case, developers would have to remove personal data from models—not just the training data sets—on request; it would be very difficult to sell products that incorporated models, and even techniques like automated model generation could become problematic. Again, the authors point to differential privacy, but with the caution that few companies have the expertise to deploy models with differential privacy correctly.
Other vulnerabilities, other attacks
This brief taxonomy of vulnerabilities doesn’t come close to listing all the problems that machine learning will face in the field. Many of these vulnerabilities are easily exploited. You can probe Amazon to find out what products are recommended along with your products, possibly finding out who your real competitors are, and discovering who to attack. You might even be able to reverse-engineer how Amazon makes recommendations and use that knowledge to influence the recommendations they make.
More complex attacks have been seen in the field. One involves placing fake reviews on an Amazon seller’s site, so that when the seller removes the reviews, Amazon bans the seller for review manipulation. Is this an attack against machine learning? The attacker tricks the human victim into violating Amazon’s rules. Ultimately, though, it’s the machine learning system that’s tricked into taking an incorrect action (banning the victim) that it could have prevented.
“Google bowling” means creating large numbers of links to a competitor’s website in hopes that Google’s ranking algorithm will penalize the competitor for purchasing bulk links. It’s similar to the fake review attack, except that it doesn’t require a human intermediary; it’s a direct attack against the algorithm that analyzes inbound links.
Advertising was one of the earliest adopters of machine learning, and one of the earliest victims. Click fraud is out of control, and the machine learning community is reluctant to talk about (or is unaware of) the issue—even though, as online advertising becomes ever more dependent on machine learning, fraudsters will learn how to attack models directly in their attempts to appear legitimate. If click data is unreliable, then models built from that data are unreliable, along with any results or recommendations generated by those models. And click fraud is similar to many attacks against recommendation systems and trend analysis. Once a “fake news” item has been planted, it’s simple to make it trend with some automated clicks. At that point, the recommendation takes over, generating recommendations which in turn generate further clicks. Anything automated is prone to attack, and automation allows those attacks to take place at scale.
The advent of autonomous vehicles, ranging from cars to drones, presents yet another set of threats. If the machine learning systems on an autonomous vehicle are vulnerable to attack, a car or truck could conceivably be used as a murder weapon. So could a drone—either a weaponized military drone or a consumer drone. The military already knows that drones are vulnerable; in 2011, Iran captured a U.S. drone, possibly by spoofing GPS signals. We expect to see attacks on “smart” consumer health devices and professional medical devices, many of which we know are already vulnerable.
Taking action
Merely scolding and thinking about possible attacks won’t help. What can be done to defend machine learning models? First, we can start with traditional software. The biggest problem with insecure software isn’t that we don’t understand security; it’s that software vendors, and software users, never take the basic steps they would need to defend themselves. It’s easy to feel defenseless before hyper-intelligent hackers, but the reality is that sites like Equifax become victims because they didn’t take basic precautions, such as installing software updates. So, what do machine learning developers need to do?
Security audits are a good starting point. What are the assets that you need to protect? Where are they, and how vulnerable are they? Who has access to those resources, and who actually needs that access? How can you minimize access to critical data? For example, a shipping system needs customer addresses, but it doesn’t need credit card information; a payment system needs credit card information, but not complete purchase histories. Can this data be stored and managed in separate, isolated databases? Beyond that, are basic safeguards in place, such as two-factor authentication? It’s easy to fault Equifax for not updating their software, but almost any software system depends on hundreds, if not thousands, of external libraries. What strategy do you have in place to ensure they’re updated, and that updates don't break working systems?
Like conventional software, machine learning systems should use monitoring systems that generate alerts to notify staff when something abnormal or suspicious occurs. Some of these monitoring systems are already using machine learning for anomaly detection—which means the monitoring software itself can be attacked.
Penetration testing is a common practice in the online world: your security staff (or, better, consultants) attack your site to discover its vulnerabilities. Attack simulation is an extension of penetration testing that shows you “how attackers actually achieve goals against your organization.” What are they looking for? How do they get to it? Can you gain control over a system by poisoning its inputs?
Tools for testing computer vision systems by generating "adversarial images" are already appearing, such as cleverhans and IBM’s ART. We are starting to see papers describing adversarial attacks against speech recognition systems. Adversarial input is a special case of a more general problem. Most machine learning developers assume their training data is similar to the data their systems will face in the real world. That’s an idealized best case. It’s easy to build a face identification system if all your faces are well-lit, well-focused, and have light-skinned subjects. A working system needs to handle all kinds of images, including images that are blurry, badly focused, poorly lighted—and have dark-skinned subjects.
Safety verification is a new area for AI research, still in its infancy. Safety verification asks questions like whether models can deliver consistent results, or whether small changes in the input lead to large changes in the output. If machine learning is at all like conventional software, we expect an escalating struggle between attackers and defenders; better defenses will lead to more sophisticated attacks, which will lead to a new generation of defenses. It will never be possible to say that a model has been “verifiably safe.” But it is important to know that a model has been tested, and that it is reasonably well-behaved against all known attacks.
Model explainability has become an important area of research in machine learning. Understanding why a model makes specific decisions is important for several reasons, not the least of which is that it makes people more comfortable with using machine learning. That “comfort” can be deceptive, of course. But being able to ask models why they made particular decisions will conceivably make it easier to see when they’ve been compromised. During development, explainability will make it possible to test how easy it is for an adversary to manipulate a model, in applications from image classification to credit scoring. In addition to knowing what a model does, explainability will tell us why, and help us build models that are more robust, less subject to manipulation; understanding why a model makes decisions should help us understand its limitations and weaknesses. At the same time, it’s conceivable that explainability will make it easier to discover weaknesses and attack vectors. If you want to poison the data flowing into a model, it can only help to know how the model responds to data.
In “Deep Automation in Machine Learning,” we talked about the importance of data lineage and provenance, and tools for tracking them. Lineage and provenance are important whether or not you’re developing the model yourself. While there are many cloud platforms to automate model building and even deployment, ultimately your organization is responsible for the model’s behavior. The downside of that responsibility includes everything from degraded profits to legal liability. If you don’t know where your data is coming from and how it has been modified, you have no basis for knowing whether your data has been corrupted, either through accident or malice.
“Datasheets for Datasets” proposes a standard set of questions about a data set’s sources, how the data was collected, its biases, and other basic information. Given a specification that records a data set’s properties, it should be easy to test and detect sudden and unexpected changes. If an attacker corrupts your data, you should be able to detect that and correct it up front; if not up front, then later in an audit.
Datasheets are a good start, but they are only a beginning. Whatever tools we have for tracking data lineage and provenance need to be automated. There will be too many models and data sets to rely on manual tracking and audits.
Balancing openness against tipping off adversaries
In certain domains, users and regulators will increasingly prefer machine learning services and products that can provide simple explanations for how automated decisions and recommendations are being made. But we’ve already seen that too much information can lead to certain parties gaming models (as in SEO). How much to disclose depends on the specific application, domain, and jurisdiction.
This balancing act is starting to come up in machine learning and related areas that involve the work of researchers (who tend to work in the open) who are up against adversaries who prize unpublished vulnerabilities. The question of whether or not to “temporarily hold back” research results is a discussion that the digital media forensics community has been having. In a 2018
https://ift.tt/2Vrb0Ym
0 notes
Text
The 27 most interesting new features in iOS 11

Tim Cook, CEO, holds an iPad Pro after his keynote address to Apple’s annual world wide developer conference (WWDC) in San Jose, California, U.S. June 5, 2017. REUTERS/Stephen Lam
If there were one big lesson from the announcements at Apple’s developer conference Monday morning, it’s this: It’s getting harder and harder to add Big New Features to a phone operating system.
When iOS 11, the new, free iPhone/iPad OS upgrade comes this fall, you won’t gain any big-ticket feature. Instead, you’ll get a wholllllle lot of tiny nips and tucks. They seem to fall into five categories: Nice Tweaks, Storage Help, iPad Exclusives, Playing Catch-Up, Fixing Bad Design.
Nice Tweaks
Expectations set? OK—here’s what’s new.
A new voice for Siri. The new male and female voices sound much more like actual people.
One-handed typing. There’s a new keyboard that scoots closer to one side, for easier one-handed typing. (You can now zoom in Maps one-handed, too.)
The new one-handed keyboard.
Quicker transfer. When you get a new iPhone, you can import all your settings from the old one just by focusing the camera on the new phone on the old one’s screen.
Do not disturb while driving. This optional feature sounds like a really good one. When the phone detects that you’re driving—because it’s connected to your phone’s Bluetooth, or because the phone detects motion—it prevents any notifications (alert messages from your apps) from showing up to distract you. If someone texts you, they get an auto-response like, “I’m driving. I’ll see your message when I get where I’m going.” (You can designate certain people as VIPs; if they text the word “urgent” to you, their messages break through the blockade.)
No more distracting notifications while you’re on the road.
Improvements to Photos. The Photos app offers smarter auto-slideshows (called Memories). Among other improvements, they now play well even when you’re holding the phone upright.
Improvements to Live Photos. Live Photos are weird, three-second video clips, which Apple (AAPL) introduced in iOS 9. In iOS 11, you can now shorten one, or mute its audio, or extract a single frame from that clip to use as a still photo. The phone can also suggest a “boomerang” segment (bounces back and forth) or a loop (repeats over and over). And it has a new Slow Shutter filter, which (for example) blurs a babbling brook or stars moving across the sky, as though taken with a long exposure.
Swipe the Lock screen back down. You can now get back to your Lock screen without actually locking your iPhone—to have another look at a notification you missed, for example.
Smarter Siri. Siri does better an anticipating your next move (location, news, calendar appointments). When you’re typing, the auto-suggestions above the keyboard now offer movie names, song names, or place names that you’ve recently viewed in other apps. Auto-suggestions in Siri, too, include terms you’ve recently read. And if you book a flight or buy a ticket online, iOS offers to add it to your calendar.
AirPlay 2. If you buy speakers from Bose, Marantz, and a few other manufactures (unfortunately, not Sonos), you can use your phone to control multi-room audio. You can start the same song playing everywhere, or play different songs in different rooms.
Shared “Up Next” playlist. If you’re an Apple Music subscriber, your party guests or buddies can throw their own “what song to play next” ideas into the ring.
Screen recording. Now you can do more than just take a screenshot of what’s on your screen. You can make a video of it! Man, will that be helpful for people who teach or review phone software! (Apple didn’t say how you start the screen recording, though.)
Storage Help
Running out of room on the iPhone is a chronic problem. Apple has a few features designed to help:
Camera app. Apple is adopting new file formats for photos (HEIF, or High Efficiency Image Format) and videos (H265 or High Efficiency Video Codec), which look the same as they did before but consume only the half the space. (When you export to someone else, they convert to standard formats.)
Messages in iCloud. When you sign into any new Mac, iPhone, or iPad with your iCloud credentials, your entire texting history gets downloaded automatically. (As it is now, you can’t see the Message transcript history with someone on a new machine.) Saving the Messages history online also saves disk space on your Mac.
Storage optimization. The idea: As your phone begins to run out of space, your oldest files are quietly and automatically stored online, leaving Download icons in their places on your phone, so that you can retrieve them if you need them.
iPad Exclusives
Many of the biggest changes in iOS 11 are available only on the iPad.
Mac features. In general, the big news here is the iPad behaves much more like a Mac. For example, you can drag-and-drop pictures and text between apps. The Dock is now extensible, available from within any app, and perfect for switching apps, just as on the Mac. There’s a new Mission Control-type feature, too, for seeing what’s in your open apps—even when you’ve split the screen between pairs of apps.
The iPad now offers a “Mission Control,” showing what’s going on in all your apps.
Punctuation and letters on the same keyboard. Now, punctuation symbols appear above the letter keys. You flick down on the key to “type” the punctuation—no more having to switch keyboard layouts.
No more switching keyboards just to type punctuation.
A file manager! A new app called Files lets you work with (and search) files and folders, just as you do on the Mac or PC. It even shows your Box and Dropbox files.
A Finder–a desktop–comes at last to iOS.
Pencil features. If you’ve bought Apple’s stylus, you can tap the Lock screen and start taking notes right away. You can mark up PDFs just by starting to write on them. A new feature lets you snap a document with the iPad’s camera, which straightens and crops the page so that you can sign it or annotate it. Handwriting in the Notes app is now searchable, and you can make drawings within any Note or email message.
The iPad grows ever closer to becoming a legal pad.
Playing Catch-Up
With every new OS from Google (GOOG, GOOGL), Microsoft (MSFT), or Apple, there’s a set of “us, too!” features that keeps them all competitive. This time around, it’s:
Lane guidance. When you’re driving, Maps now lets you know which lane to be in for your next turn, just as Google Maps does.
Lane guidance. At last.
Indoor Maps. The Maps app can now show you floor plans for a few malls and 30 airports, just as Google Maps does.
Siri translates languages. Siri is trying to catch up to Google Assistant. For example, it can now translate phrases from English into Chinese, French, German, Italian, or Spanish. For example, you can say, “How do you say ‘Where’s the bathroom?’ in French?”
Siri understands followup questions. Siri now does better at understanding followup questions. (“Who won the World Series in 1980?” “The “Phillies.” “Who was their coach?”)
Person-to-Person payment within the Messages app. Now, you can send payments directly to your friends—your share of the pizza bill, for example—right from within the Messages app, much as people do now with Venmo, PayPal, and their its ilk. (Of course, this works only if your friends have iPhones, too.) When money comes to you, it accrues to a new, virtual Apple Pay Cash Card; from there, you can send it to your bank, buy things with it, or send it on to other people.

iCloud file sharing. Finally, you can share files you’ve stored on your iCloud Drive with other people, just as you’ve been able to do with Dropbox for years.
Fixing Bad Design
Some of the changes repair the damage Apple made to itself in iOS 10. For example:
Redesigned apps drawer in Messages. All the stuff they added to Messages last year (stickers, apps, live drawing) cluttered up the design and wound up getting ignored by lots of people. The new design is cleaner.
Redesigned Control Center. In iOS 10, Apple split up the iPhone’s quick-settings panel, called the Control Center, into two or three panels. You had to swipe sideways to find the control you wanted—taking care not to swipe sideways on one of the controls, thereby triggering it. Now it’s all on one screen again, although some of the buttons open up secondary screens of options. And it’s customizable! You can, for example, add a “Record voice memo” button to it.
The new, customizable, somewhat ugly Control Center.
App Store. The App store gets a big redesign. One chief fix is breaking out Games into its own tab, so that game and non-game bestseller lists are kept separate.
After nine years, the App Store gets a new look.
Coming this fall
There are also dozens of improvements to the features for overseas iPhones (China, Russia, India, for example). And many, many enhancements to features for the disabled (spoken captions for videos and pictures, for example).
So what’s the overarching theme of the iOS 11 upgrade?
There isn’t one. It’s just a couple hundred little fine-tunings. All of them welcome—and all of them aimed to keep you trapped within Apple’s growing ecosystem.
More from David Pogue:
Inside the World’s Greatest Scavenger Hunt: Part 1 • Part 2 • Part 3 • Part 4 • Part 5
The DJI Spark is the smallest, cheapest obstacle-avoiding drone yet
The new Samsung Galaxy does 27 things the iPhone doesn’t
The most important announcements from Google’s big developer’s conference
Google Home’s mastermind has no intention of losing to Amazon
Now I get it: Ransomware
Google exec explains how Google Assistant just got smarter
Amazon’s Alexa calling is like a Jetsons version of the home phone
David Pogue, tech columnist for Yahoo Finance, welcomes nontoxic comments in the comments section below. On the web, he’s davidpogue.com. On Twitter, he’s @pogue. On email, he’s [email protected]. You can read all his articles here, or you can sign up to get his columns by email.
#tech#Pogue#David Pogue#_lmsid:a077000000BAh3wAAD#$GOOGL#_revsp:yahoofinance.com#$MSFT#$GOOG#$AAPL#_uuid:d0427da1-69d7-3791-9d1f-0f3527c3cedc#_author:David Pogue
5 notes
·
View notes
Text
The best and worst gadgets of 2018
There were countless gadgets released in 2018. It’s the end of the year, so Brian and I rounded up the best of the best and the worst of the worst.
Some where great! Like the Oculus Go. Or the Google Home Hub. But some were junk, like the revived Palm or PlayStation Classic.
CES 2019 is a few weeks away, where manufacturers will roll out most of their wares for the upcoming year. But most products will not be available for purchase for months. What follows is a list of the best and worst gadgets available going into 2019.
The Best
Google Home Hub
Google took its sweet time bringing an Echo Show competitor to market. When the Home Hub did finally arrive, however, the company lapped the competition. The smart screen splits the size difference between the Echo Spot and Show, with a form factor that fits in much more comfortably in most home decor.
Assistant still sports a much deeper knowledge base than Alexa, and the Hub offers one not so secret weapon: YouTube. Google’s video service is light years ahead of anything Amazon (or anyone, really) currently offers, and the competition shows no sign of catching up.
DJI Osmo Pocket
I wanted to dislike the Osmo Pocket. I mean, $349 for a gimbal with a built-in screen is pretty steep by any measure — especially given the fact that the drone maker has much cheaper and more professional options. After an afternoon with the Pocket, however, I was hooked.
The software takes a little getting used to, but once you’ve mastered it, you’re off to the races, using many of the same tricks you’ll find on the Mavic line. Time-lapse, FaceTrack and the 10 Story Mode templates are all impressive and can help novices capture compelling video from even the most mundane subject matter.
Oculus Go
The most recent wave of VR headsets has been split between two distinct categories. There are the high-end Rift and Vives on one side and the super-low-cost Daydreams and Gear VRs on the other. That leaves consumers in the unenviable position of choosing between emptying the bank account or opting for a sub-par experience.
Oculus’ Go headset arrived this year to split the difference. In a time when virtual reality seems at the tail end of its hype cycle, the $199 device offers the most compelling case for mainstreaming yet.
It’s a solid and financially accessible take on VR that shows that the category may still have a little life left in it yet.
Timbuk2 Never Check Expandable Backpack
Granted, it’s not a gadget per se, but the Never Check is the best backpack I’ve ever owned. I initially picked it up as part of a Gift Guide feature I was writing, and I’ve since totally fallen for the thing.
As someone who spends nearly half of his time on the road these days, the bag’s big volume and surprisingly slim profile have been a life saver. It’s followed me to a Hong Kong hostel and a Nigerian hotel, jammed full of all the tech I need to do my job.
It’s also unassuming enough to be your day to day bag. Just zip up one of those waterproof zippers to compress its footprint.
Happy Hacking Keyboard Professional 2
Like most nerds, I have more keyboards than friends. In 2018 I gave mechanical keyboards a chance. Now, at the end of the year, I’m typing on a Happy Hacking Keyboard Professional 2. It’s lovely.
This keyboard features Topre capacitive 45G switches. What does that mean? When typing, these switches provide a nice balance of smooth action and tactile feel. There are a handful of mechanical switches available, and after trying most of them, this switch feels the best to me. The Topre capacitive switch is available in a handful of keyboards, but I like the Happy Hacking Keyboard the best.
The HHK has been around in various forms since 1996, and this latest version retains a lot of the charm, including dip switches. Everyone loves dip switches. This version works well with Macs, has two USB ports and is compact enough someone could throw it into a bag. Starting just last month, the keyboard is available in the U.S. through Fujitsu, so buyers don’t have to deal with potentially shady importers.
The Worst
Palm
The Palm is the kind of device you really want to like. And I tried. Hell, I took the thing to Africa with me in hopes that I’d be able to give it some second life as an MP3 player. But it fell short even on that front.
This secondary smartphone is a device in search of a problem, appealing to an impossibly thin slice of consumer demographics. It’s definitely adorable, but the ideal consumer has to have the need and money for a second display, no smartwatch and an existing Verizon contract. Even then, the product has some glaring flaws, from more complex user issues to simple stupid things, like a lack of volume buttons.
It’s easy to forgive a lot with a fairly well-designed first-generation product, but it’s hard to see where the newly reborn company goes from here. Palm, meet face.
Red Hydrogen One
Where to start? How about the price? Red’s first foray into the smartphone space starts at $1,293 (or $1,595 if you want to upgrade your aluminum to titanium). That price will get you a middling phone with an admittedly fascinating gimmick.
After what seemed like years of teasers, the Hydrogen One finally appeared in October, sporting a big, metal design and Rambo-style serrated edges. The display’s the thing here, sporting a “nano-photonic” design that looks a bit like a moving version of those holographic baseball cards we had as kids.
I showed it to a number of folks during my testing period, and all found it initially interesting, then invariably asked “why?” I’m still having trouble coming up with the answer on that one. Oh, and a few told me they became a touch nauseous looking at it. Can’t win ’em all, I guess.
Facebook Portal
“Why?” is really the overarching question in all of these worst devices. It’s not as if the Portal was a bad product. The design of the thing is actually pretty solid — certainly it looks a lot nicer than the Echo Show. And while it was initially lacking in features, Facebook has made up for that a bit with a recent software update.
The heart of the question is more about what Portal brings to the table that the Echo Show or Google Home Hub don’t. It would have to be something pretty massive to justify bringing a Facebook-branded piece of hardware into one’s living room, especially in light of all of the privacy concerns the social media site has dealt with this year. There’s never been a great time for Facebook to launch a product like this, but somehow, now feels like the worst.
Portal delivers some neat tricks, including impressive camera tracking and AR stories, but it mostly feels like a tone-deaf PR nightmare.
PlayStation Classic
1: Half the games are PAL ports and do not run well on U.S. TVs 2: Missing classics like Gran Turismo, Crash Bandicoot and Tomb Raider 3: Doesn’t include a power adapter 4: Only one suspend point 5: This product makes me angry
from Gadgets – TechCrunch https://tcrn.ch/2T2flzV from Blogger http://bit.ly/2RcoMzA https://tcrn.ch/2SfEpU2
0 notes
Text
The Best and Worst gadgets of 2018
There was countless gadgets released in 2018. It’s the end of the year so Brian and I rounded up the best of the best and the worst of the worst. Some where great! Like the Oculus Go. Or the Google Home Hub. But some were junk like the revived Palm or Playstation Classic.
CES 2019 is a few weeks away where manufacturers will roll out most of their wares for the upcoming year. But most products will not be available for purchase for months. What follows is a list of the best and worst gadgets available going into 2019.
Google Home Hub
Google took its sweet time bringing an Echo Show competitor to market. When the Home Hub did finally arrive, however, the company lapped the competition. The smart screen splits the size difference between the Echo Spot and Show, with a form factor that fits in much more comfortable in most home decor.
Assistant still sports a much deeper knowledge base than Alexa, and the Hub offers one not so secret weapon: YouTube. Google’s video service is light years ahead of anything Amazon (or anyone, really) currently offers, and the competition shows no sign of catching up.
DJI Osmo Pocket
I wanted to dislike the Osmo Pocket. I mean, $349 for a gimbal with a built in screen is pretty steep by any measure — especially given the fact that the drone maker has much cheaper and more professional options. After an afternoon with the Pocket, however, I was hooked.
The software takes a little getting used to, but once you’ve mastered it, you’re off the races, using many of the same tricks you’ll find on the Mavic line. Time-lapse, FaceTrack and the 10 Story Mode templates are all impressive and can help novices capture compelling video from even the most mundane subject matter.
Oculus Go
The most recent wave of VR headsets has been split between two distinct categories. There are the high-end Rift and Vives on one-side and the super low-cost Daydreams and Gear VRs on the other. That leaves consumers in the unenviable position of choosing between emptying the bank account or opting for a sub-par experience.
Oculus’ Go headset arrived this year to split the difference. In a time when virtual reality seems at the tail end of its hype cycle, the $199 device offers the most compelling case for mainstreaming yet.
It’s a solid and financially accessible take on VR that shows that the category may still have a little life left in it yet.
Timbuk2 Never Check Expandable Backpack
Granted, it’s not a gadget per se, but the Never Check is the best backpack I’ve ever owned. I initially picked it up as part of a Gift Guide feature I was writing, and I’ve since totally fallen for the thing.
As someone who spends nearly half of his time on the road these days, the bag’s space’s big volume and surprisingly slim profile have been a life saver. It’s followed me to a Hong Kong hostel and a Nigeria hotel, jammed full of all of the tech I need to do my job.
It’s also unassuming enough to be your day to day. Just zip up one of those waterproof zippers to compress its footprint.
Happy Hacking Keyboard Professional 2
Like most nerds, I have more keyboards than friends. In 2018 I gave mechanical keyboards a chance. Now, at the end of the year, I’m typing on a Happy Hacking Keyboard Professional 2. It’s lovely.
This keyboard features Topre capacitive 45G switches. What does that mean? When typing these switches provide a nice balance of smooth actions and tactile feel. There are a handful of mechanical switches available, and after trying most of them, this switch feels the best to me. The Topre capacitive switch is available in a handful of keyboards, but I like the Happy Hacking Keyboard the best.
The HHK has been around in various forms since 1996 and this latest version retains a lot of the charm including dip switches. Everyone loves dip switches. This version works well with Macs, has two USB ports and is compact enough someone could throw into a bag. Starting just last month, the keyboard is available in the US through Fujitsu so buyers do not have to deal with potentially shady importers.
Worst
Palm
The Palm is the kind of device you really want to like. And I tried. Hell, I took the thing to Africa with me in hopes that I’d be able to give it some second life as an MP3 player. But it feel short even on that front.
This secondary smartphone is a device in search of a problem, appealing to an impossibly thin slice of consumer demographics. It’s definitely adorable, but the ideal consumers has to have the need and money for a second display, no smartwatch and an existing Verizon contract. Even then, the product has some glaring flaws, from more complex user issues to simple stupid things, like a lack of volume buttons.
It’s easy to forgive a lot with a fairly well designed first generation product, but it’s hard to see where the newly reborn company goes from here. Palm, meet face.
RED Hydrogen One
Where to start? How about the price? Red’s first foray into the smartphone space starts at $1,293 (or $1,595 if you want to upgrade your aluminum to titanium). That price will get you a middling phone with an admittedly fascinating gimmick.
After what seemed like years of teasers, the Hydrogen One finally appeared in October, sporting a big, metal design and Rambo-style serrated edges. The display’s the thing here, sporting a “nano-photonic” design that looks a bit like a moving version of those holographic baseball cards we had as kids.
I showed it to a number of folks during my testing period, and all found it initially interesting, then invariably asked “why?” I’m still having trouble coming up with the answer on that one. Oh, and a few told me they became a touch nauseous looking at it. Can’t win ‘em all, I guess.
Facebook Portal
Why? is really the overarching question in all of these worst devices. It’s not as if the Portal was a bad product. The design of the thing is actually pretty solid — certainly it looks a lot nicer than the Echo Show. And while it was initially lacking in features, Facebook has made for that a bit with a recent software update.
The heart of the question is more about what Portal brings to the table that the Echo Show or Google Home Hub don’t. It would have to be something pretty massive to justify bringing a Facebook-branded piece of hardware into one’s living room, especially in light of all of the privacy concerns the social media site has dealt with this year. There’s never been a great time for Facebook to launch a product like this, but somehow, no feels like the worst.
Portal delivers some neat tricks, including impressive camera tracking and AR stories, but it mostly feels like a tone deaf PR nightmare.
Playstation Classic
1: Half the games are PAL ports and do not run well on US TVs 2: Missing classics like Gran Turismo, Crash Bandicoot, and Tomb Raider 3: Doesn’t include a power adapter 4: Only one suspend point 5: This product makes me angry
from Facebook – TechCrunch https://tcrn.ch/2T2flzV via IFTTT
0 notes
Text
We could soon face a robot crimewave ... the law needs to be ready
by Christopher Markou

This is where we are at in 2017: sophisticated algorithms are both predicting and helping to solve crimes committed by humans; predicting the outcome of court cases and human rights trials; and helping to do the work done by lawyers in those cases. By 2040, there is even a suggestion that sophisticated robots will be committing a good chunk of all the crime in the world. Just ask the toddler who was run over by a security robot at a California mall last year.
How do we make sense of all this? Should we be terrified? Generally unproductive. Should we shrug our shoulders as a society and get back to Netflix? Tempting, but no. Should we start making plans for how we deal with all of this? Absolutely.
Fear of Artificial Intelligence (AI) is a big theme. Technology can be a downright scary thing; particularly when its new, powerful, and comes with lots of question marks. But films like Terminator and shows like Westworld are more than just entertainment, they are a glimpse into the world we might inherit, or at least into how we are conceiving potential futures for ourselves.
Among the many things that must now be considered is what role and function the law will play. Expert opinions differ wildly on the likelihood and imminence of a future where sufficiently advanced robots walk among us, but we must confront the fact that autonomous technology with the capacity to cause harm is already around. Whether it’s a military drone with a full payload, a law enforcement robot exploding to kill a dangerous suspect or something altogether more innocent that causes harm through accident, error, oversight, or good ol’ fashioned stupidity.
There’s a cynical saying in law that “wheres there’s blame, there’s a claim”. But who do we blame when a robot does wrong? This proposition can easily be dismissed as something too abstract to worry about. But let’s not forget that a robot was arrested (and released without charge) for buying drugs; and Tesla Motors was absolved of responsibility by the American National Highway Traffic Safety Administration when a driver was killed in a crash after his Tesla was in autopilot.
While problems like this are certainly peculiar, history has a lot to teach us. For instance, little thought was given to who owned the sky before the Wright Brothers took the Kitty Hawk for a joyride. Time and time again, the law is presented with these novel challenges. And despite initial overreaction, it got there in the end. Simply put: law evolves.
Robot guilt
The role of the law can be defined in many ways, but ultimately it is a system within society for stabilising people’s expectations. If you get mugged, you expect the mugger to be charged with a crime and punished accordingly.
But the law also has expectations of us; we must comply with it to the fullest extent our consciences allow. As humans we can generally do that. We have the capacity to decide whether to speed or obey the speed limit – and so humans are considered by the law to be “legal persons”.
To varying extents, companies are endowed with legal personhood, too. It grants them certain economic and legal rights, but more importantly it also confers responsibilities on them. So, if Company X builds an autonomous machine, then that company has a corresponding legal duty.
The problem arises when the machines themselves can make decisions of their own accord. As impressive as intelligent assistants, Alexa, Siri or Cortana are, they fall far short of the threshold for legal personhood. But what happens when their more advanced descendants begin causing real harm?
A guilty AI mind?
The criminal law has two critical concepts. First, it contains the idea that liability for harm arises whenever harm has been or is likely to be caused by a certain act or omission.
Second, criminal law requires that an accused is culpable for their actions. This is known as a “guilty mind” or “mens rea”. The idea behind mens rea is to ensure that the accused both completed the action of assaulting someone and had the intention of harming them, or knew harm was a likely consequence of their action.

Blind justice for a AI. Shutterstock
So if an advanced autonomous machine commits a crime of its own accord, how should it be treated by the law? How would a lawyer go about demonstrating the “guilty mind” of a non-human? Can this be done be referring to and adapting existing legal principles?
Take driverless cars. Cars drive on roads and there are regulatory frameworks in place to assure that there is a human behind the wheel (at least to some extent). However, once fully autonomous cars arrive there will need to be extensive adjustments to laws and regulations that account for the new types of interactions that will happen between human and machine on the road.
As AI technology evolves, it will eventually reach a state of sophistication that will allow it to bypass human control. As the bypassing of human control becomes more widespread, then the questions about harm, risk, fault and punishment will become more important. Film, television, and literature may dwell on the most extreme examples of “robots gone awry” but the legal realities should not be left to Hollywood.
So can robots commit crime? In short: yes. If a robot kills someone, then it has committed a crime (actus reus), but technically only half a crime, as it would be far harder to determine mens rea. How do we know the robot intended to do what it did?
For now, we are nowhere near the level of building a fully sentient or “conscious” humanoid robot that looks, acts, talks, and thinks like us humans. But even a few short hops in AI research could produce an autonomous machine that could unleash all manner of legal mischief. Financial and discriminatory algorithmic mischief already abounds.
Play along with me; just imagine that a Terminator-calibre AI exists, and that it commits a crime (let’s say murder) then the task is not determining whether it in fact murdered someone; but the extent to which that act satisfies the principle of mens rea.
But what would we need to prove the existence of mens rea? Could we simply cross-examine the AI like we do a human defendant? Maybe, but we would need to go a bit deeper than that and examine the code that made the machine “tick”.
And what would “intent” look like in a machine mind? How would we go about proving an autonomous machine was justified in killing a human in self-defense or the extent of premeditation?
Let’s go even further. After all, we’re not only talking about violent crimes. Imagine a system that could randomly purchase things on the internet using your credit card – and it decided to buy contraband. This isn’t fiction; it has happened. Two London-based artists created a bot that purchased random items off the dark web. And what did it buy? Fake jeans, a baseball cap with a spy camera, a stash can, some Nikes, 200 cigarettes, a set of fire-brigade master keys, a counterfeit Louis Vuitton bag and ten ecstasy pills. Should these artists be liable for what the bot they created bought?
Maybe. But what if the bot “decided” to make the purchases itself?
Robo-jails?
Even if you solve these legal issues, you are still left with the question of punishment. What’s a 30-year jail stretch to an autonomous machine that does not age, grow infirm or miss its loved ones? Unless, of course, it was programmed to “reflect” on its wrongdoing and find a way to rewrite its own code while safely ensconced at Her Majesty’s leisure. And what would building “remorse” into machines say about us as their builders?

Would robot wardens patrol robot jails? Shutterstock
What we are really talking about when we talk about whether or not robots can commit crimes is “emergence” – where a system does something novel and perhaps good but also unforeseeable, which is why it presents such a problem for law.
AI has already helped with emergent concepts in medicine, and we are learning things about the universe with AI systems that even an army of Stephen Hawkings might not reveal.
The hope for AI is that in trying to capture this safe and beneficial emergent behaviour, we can find a parallel solution for ensuring it does not manifest itself in illegal, unethical, or downright dangerous ways.
At present, however, we are systematically incapable of guaranteeing human rights on a global scale, so I can’t help but wonder how ready we are for the prospect of robot crime given that we already struggle mightily to contain that done by humans.
Christopher Markou is a PhD Candidate, Faculty of Law at the University of Cambridge
This article was originally published on The Conversation.
33 notes
·
View notes
Text
The best and worst gadgets of 2018
There were countless gadgets released in 2018. It’s the end of the year, so Brian and I rounded up the best of the best and the worst of the worst.
Some where great! Like the Oculus Go. Or the Google Home Hub. But some were junk, like the revived Palm or PlayStation Classic.
CES 2019 is a few weeks away, where manufacturers will roll out most of their wares for the upcoming year. But most products will not be available for purchase for months. What follows is a list of the best and worst gadgets available going into 2019.
The Best
Google Home Hub
Google took its sweet time bringing an Echo Show competitor to market. When the Home Hub did finally arrive, however, the company lapped the competition. The smart screen splits the size difference between the Echo Spot and Show, with a form factor that fits in much more comfortably in most home decor.
Assistant still sports a much deeper knowledge base than Alexa, and the Hub offers one not so secret weapon: YouTube. Google’s video service is light years ahead of anything Amazon (or anyone, really) currently offers, and the competition shows no sign of catching up.
DJI Osmo Pocket
I wanted to dislike the Osmo Pocket. I mean, $349 for a gimbal with a built-in screen is pretty steep by any measure — especially given the fact that the drone maker has much cheaper and more professional options. After an afternoon with the Pocket, however, I was hooked.
The software takes a little getting used to, but once you’ve mastered it, you’re off to the races, using many of the same tricks you’ll find on the Mavic line. Time-lapse, FaceTrack and the 10 Story Mode templates are all impressive and can help novices capture compelling video from even the most mundane subject matter.
Oculus Go
The most recent wave of VR headsets has been split between two distinct categories. There are the high-end Rift and Vives on one side and the super-low-cost Daydreams and Gear VRs on the other. That leaves consumers in the unenviable position of choosing between emptying the bank account or opting for a sub-par experience.
Oculus’ Go headset arrived this year to split the difference. In a time when virtual reality seems at the tail end of its hype cycle, the $199 device offers the most compelling case for mainstreaming yet.
It’s a solid and financially accessible take on VR that shows that the category may still have a little life left in it yet.
Timbuk2 Never Check Expandable Backpack
Granted, it’s not a gadget per se, but the Never Check is the best backpack I’ve ever owned. I initially picked it up as part of a Gift Guide feature I was writing, and I’ve since totally fallen for the thing.
As someone who spends nearly half of his time on the road these days, the bag’s big volume and surprisingly slim profile have been a life saver. It’s followed me to a Hong Kong hostel and a Nigerian hotel, jammed full of all the tech I need to do my job.
It’s also unassuming enough to be your day to day bag. Just zip up one of those waterproof zippers to compress its footprint.
Happy Hacking Keyboard Professional 2
Like most nerds, I have more keyboards than friends. In 2018 I gave mechanical keyboards a chance. Now, at the end of the year, I’m typing on a Happy Hacking Keyboard Professional 2. It’s lovely.
This keyboard features Topre capacitive 45G switches. What does that mean? When typing, these switches provide a nice balance of smooth action and tactile feel. There are a handful of mechanical switches available, and after trying most of them, this switch feels the best to me. The Topre capacitive switch is available in a handful of keyboards, but I like the Happy Hacking Keyboard the best.
The HHK has been around in various forms since 1996, and this latest version retains a lot of the charm, including dip switches. Everyone loves dip switches. This version works well with Macs, has two USB ports and is compact enough someone could throw it into a bag. Starting just last month, the keyboard is available in the U.S. through Fujitsu, so buyers don’t have to deal with potentially shady importers.
The Worst
Palm
The Palm is the kind of device you really want to like. And I tried. Hell, I took the thing to Africa with me in hopes that I’d be able to give it some second life as an MP3 player. But it fell short even on that front.
This secondary smartphone is a device in search of a problem, appealing to an impossibly thin slice of consumer demographics. It’s definitely adorable, but the ideal consumer has to have the need and money for a second display, no smartwatch and an existing Verizon contract. Even then, the product has some glaring flaws, from more complex user issues to simple stupid things, like a lack of volume buttons.
It’s easy to forgive a lot with a fairly well-designed first-generation product, but it’s hard to see where the newly reborn company goes from here. Palm, meet face.
Red Hydrogen One
Where to start? How about the price? Red’s first foray into the smartphone space starts at $1,293 (or $1,595 if you want to upgrade your aluminum to titanium). That price will get you a middling phone with an admittedly fascinating gimmick.
After what seemed like years of teasers, the Hydrogen One finally appeared in October, sporting a big, metal design and Rambo-style serrated edges. The display’s the thing here, sporting a “nano-photonic” design that looks a bit like a moving version of those holographic baseball cards we had as kids.
I showed it to a number of folks during my testing period, and all found it initially interesting, then invariably asked “why?” I’m still having trouble coming up with the answer on that one. Oh, and a few told me they became a touch nauseous looking at it. Can’t win ’em all, I guess.
Facebook Portal
“Why?” is really the overarching question in all of these worst devices. It’s not as if the Portal was a bad product. The design of the thing is actually pretty solid — certainly it looks a lot nicer than the Echo Show. And while it was initially lacking in features, Facebook has made up for that a bit with a recent software update.
The heart of the question is more about what Portal brings to the table that the Echo Show or Google Home Hub don’t. It would have to be something pretty massive to justify bringing a Facebook-branded piece of hardware into one’s living room, especially in light of all of the privacy concerns the social media site has dealt with this year. There’s never been a great time for Facebook to launch a product like this, but somehow, now feels like the worst.
Portal delivers some neat tricks, including impressive camera tracking and AR stories, but it mostly feels like a tone-deaf PR nightmare.
PlayStation Classic
1: Half the games are PAL ports and do not run well on U.S. TVs 2: Missing classics like Gran Turismo, Crash Bandicoot and Tomb Raider 3: Doesn’t include a power adapter 4: Only one suspend point 5: This product makes me angry
from RSSMix.com Mix ID 8176395 https://techcrunch.com/2018/12/21/the-best-and-worst-gadgets-of-2018/ via http://www.kindlecompared.com/kindle-comparison/
0 notes
Text
Jeff Bezos being Jeff Bezos
*He’s the Duke of the Stacks at the moment, so maybe it’s good to hear him out.
EX-99.1 2 d373368dex991.htm EX-99.1
Exhibit 99.1
“Jeff, what does Day 2 look like?”
That’s a question I just got at our most recent all-hands meeting. I’ve been reminding people that it’s Day 1 for a couple of decades. I work in an Amazon building named Day 1, and when I moved buildings, I took the name with me. I spend time thinking about this topic.
“Day 2 is stasis. Followed by irrelevance. Followed by excruciating, painful decline. Followed by death. And that is why it is always Day 1.”
To be sure, this kind of decline would happen in extreme slow motion. An established company might harvest Day 2 for decades, but the final result would still come.
I’m interested in the question, how do you fend off Day 2? What are the techniques and tactics? How do you keep the vitality of Day 1, even inside a large organization?
Such a question can’t have a simple answer. There will be many elements, multiple paths, and many traps. I don’t know the whole answer, but I may know bits of it. Here’s a starter pack of essentials for Day 1 defense: customer obsession, a skeptical view of proxies, the eager adoption of external trends, and high-velocity decision making.
True Customer Obsession
There are many ways to center a business. You can be competitor focused, you can be product focused, you can be technology focused, you can be business model focused, and there are more. But in my view, obsessive customer focus is by far the most protective of Day 1 vitality.
Why? There are many advantages to a customer-centric approach, but here’s the big one: customers are always beautifully, wonderfully dissatisfied, even when they report being happy and business is great. Even when they don’t yet know it, customers want something better, and your desire to delight customers will drive you to invent on their behalf. No customer ever asked Amazon to create the Prime membership program, but it sure turns out they wanted it, and I could give you many such examples.
Staying in Day 1 requires you to experiment patiently, accept failures, plant seeds, protect saplings, and double down when you see customer delight. A customer-obsessed culture best creates the conditions where all of that can happen.
Resist Proxies
As companies get larger and more complex, there’s a tendency to manage to proxies. This comes in many shapes and sizes, and it’s dangerous, subtle, and very Day 2.
A common example is process as proxy. Good process serves you so you can serve customers. But if you’re not watchful, the process can become the thing. This can happen very easily in large organizations. The process becomes the proxy for the result you want. You stop looking at outcomes and just make sure you’re doing the process right. Gulp. It’s not that rare to hear a junior leader defend a bad outcome with something like, “Well, we followed the process.” A more experienced leader will use it as an opportunity to investigate and improve the process. The process is not the thing. It’s always worth asking, do we own the process or does the process own us? In a Day 2 company, you might find it’s the second.
Another example: market research and customer surveys can become proxies for customers – something that’s especially dangerous when you’re inventing and designing products. “Fifty-five percent of beta testers report being satisfied with this feature. That is up from 47% in the first survey.” That’s hard to interpret and could unintentionally mislead.
Good inventors and designers deeply understand their customer. They spend tremendous energy developing that intuition. They study and understand many anecdotes rather than only the averages you’ll find on surveys. They live with the design.
I’m not against beta testing or surveys. But you, the product or service owner, must understand the customer, have a vision, and love the offering. Then, beta testing and research can help you find your blind spots. A remarkable customer experience starts with heart, intuition, curiosity, play, guts, taste. You won’t find any of it in a survey.
Embrace External Trends
The outside world can push you into Day 2 if you won’t or can’t embrace powerful trends quickly. If you fight them, you’re probably fighting the future. Embrace them and you have a tailwind.
These big trends are not that hard to spot (they get talked and written about a lot), but they can be strangely hard for large organizations to embrace. We’re in the middle of an obvious one right now: machine learning and artificial intelligence.
Over the past decades computers have broadly automated tasks that programmers could describe with clear rules and algorithms. Modern machine learning techniques now allow us to do the same for tasks where describing the precise rules is much harder.
At Amazon, we’ve been engaged in the practical application of machine learning for many years now. Some of this work is highly visible: our autonomous Prime Air delivery drones; the Amazon Go convenience store that uses machine vision to eliminate checkout lines; and Alexa,1 our cloud-based AI assistant. (We still struggle to keep Echo in stock, despite our best efforts. A high-quality problem, but a problem. We’re working on it.)
But much of what we do with machine learning happens beneath the surface. Machine learning drives our algorithms for demand forecasting, product search ranking, product and deals recommendations, merchandising placements, fraud detection, translations, and much more. Though less visible, much of the impact of machine learning will be of this type – quietly but meaningfully improving core operations.
Inside AWS, we’re excited to lower the costs and barriers to machine learning and AI so organizations of all sizes can take advantage of these advanced techniques.
Using our pre-packaged versions of popular deep learning frameworks running on P2 compute instances (optimized for this workload), customers are already developing powerful systems ranging everywhere from early disease detection to increasing crop yields. And we’ve also made Amazon’s higher level services available in a convenient form. Amazon Lex (what’s inside Alexa), Amazon Polly, and Amazon Rekognition remove the heavy lifting from natural language understanding, speech generation, and image analysis. They can be accessed with simple API calls – no machine learning expertise required. Watch this space. Much more to come.
High-Velocity Decision Making
Day 2 companies make high-quality decisions, but they make high-quality decisions slowly. To keep the energy and dynamism of Day 1, you have to somehow make high-quality, high-velocity decisions. Easy for start-ups and very challenging for large organizations. The senior team at Amazon is determined to keep our decision-making velocity high. Speed matters in business – plus a high-velocity decision making environment is more fun too. We don’t know all the answers, but here are some thoughts.
First, never use a one-size-fits-all decision-making process. Many decisions are reversible, two-way doors. Those decisions can use a light-weight process. For those, so what if you’re wrong? I wrote about this in more detail in last year’s letter.
1 For something amusing, try asking, “Alexa, what is sixty factorial?”
Second, most decisions should probably be made with somewhere around 70% of the information you wish you had. If you wait for 90%, in most cases, you’re probably being slow. Plus, either way, you need to be good at quickly recognizing and correcting bad decisions. If you’re good at course correcting, being wrong may be less costly than you think, whereas being slow is going to be expensive for sure.
Third, use the phrase “disagree and commit.” This phrase will save a lot of time. If you have conviction on a particular direction even though there’s no consensus, it’s helpful to say, “Look, I know we disagree on this but will you gamble with me on it? Disagree and commit?” By the time you’re at this point, no one can know the answer for sure, and you’ll probably get a quick yes.
This isn’t one way. If you’re the boss, you should do this too. I disagree and commit all the time. We recently greenlit a particular Amazon Studios original. I told the team my view: debatable whether it would be interesting enough, complicated to produce, the business terms aren’t that good, and we have lots of other opportunities. They had a completely different opinion and wanted to go ahead. I wrote back right away with “I disagree and commit and hope it becomes the most watched thing we’ve ever made.” Consider how much slower this decision cycle would have been if the team had actually had to convince me rather than simply get my commitment.
Note what this example is not: it’s not me thinking to myself “well, these guys are wrong and missing the point, but this isn’t worth me chasing.” It’s a genuine disagreement of opinion, a candid expression of my view, a chance for the team to weigh my view, and a quick, sincere commitment to go their way. And given that this team has already brought home 11 Emmys, 6 Golden Globes, and 3 Oscars, I’m just glad they let me in the room at all!
Fourth, recognize true misalignment issues early and escalate them immediately. Sometimes teams have different objectives and fundamentally different views. They are not aligned. No amount of discussion, no number of meetings will resolve that deep misalignment. Without escalation, the default dispute resolution mechanism for this scenario is exhaustion. Whoever has more stamina carries the decision.
I’ve seen many examples of sincere misalignment at Amazon over the years. When we decided to invite third party sellers to compete directly against us on our own product detail pages – that was a big one. Many smart, well-intentioned Amazonians were simply not at all aligned with the direction. The big decision set up hundreds of smaller decisions, many of which needed to be escalated to the senior team.
“You’ve worn me down” is an awful decision-making process. It’s slow and de-energizing. Go for quick escalation instead – it’s better.
So, have you settled only for decision quality, or are you mindful of decision velocity too? Are the world’s trends tailwinds for you? Are you falling prey to proxies, or do they serve you? And most important of all, are you delighting customers? We can have the scope and capabilities of a large company and the spirit and heart of a small one. But we have to choose it.
A huge thank you to each and every customer for allowing us to serve you, to our shareowners for your support, and to Amazonians everywhere for your hard work, your ingenuity, and your passion.
As always, I attach a copy of our original 1997 letter. It remains Day 1.
Sincerely,
Jeff
Jeffrey P. Bezos
Founder and Chief Executive Officer
Amazon.com, Inc.
2 notes
·
View notes
Text
How Amazon Thrives On Being Misunderstood
What are Amazon’s greatest innovations? Drones? Cloud computing? Echo and Alexa? These are impressive; some are even revolutionary. However, I believe Amazon’s greatest innovations are the ones that have changed the basics of competing to the point where they now sound mundane.
My top list of greatest Amazon innovations includes Free Everyday Shipping, Prime Loyalty, and Item Authority. Deceptively simple, Item Authority signed up multiple sellers of the same item to increase item selection, availability, and price competition. It was the “killer feature” that led to Amazon overtaking eBay in the mid-2000s as the destination site for third-party sellers.
What are the common traits each of these innovations share, other than that they come from Amazon? For one, they are all customer experience and business model innovations. They are not really that technical. What they also have in common is the fact that incumbents and industry pundits woefully underestimated their impact on the industry and the bottom line. These innovations were implemented when Amazon was young, small, and neither respected nor feared by the industry the way it is now. Here are just a few examples:
“Amazon is pulling everyone into the gutter to play that [free shipping] game.” ~ Bob Schwartz, former president of Magento and founder of Nordstrom.com
“There’s many moments where a voice assistant is really beneficial, but that doesn’t mean you’d never want a screen. So the idea of [Amazon Echo] not having a screen, I don’t think suits many situations.” ~ Philip Schiller, senior vice president of worldwide marketing for Apple
“While recent stories and reports of a new entity competing with the three major carriers in the United States grab headlines, the reality is it would be a daunting task requiring tens of billions of dollars in capital and years to build sufficient scale and density to replicate existing networks like FedEx.” ~ Mike Glenn, executive vice president of FedEx
“We do not believe our vendors selling product directly on Amazon is an imminent threat. There is no indication that any of our vendors intend to sell premium athletic product, $100-plus sneakers that we offer, directly via that sort of distribution channel.” ~ Richard Johnson, CEO and chairman of Foot Locker
“When you think about the online versus the offline experience, we don’t need AI in our stores. We have ‘I.’ We have living, breathing, 4,500 style advisors in our stores.” ~ Marc Metrick, president of Saks Fifth Avenue
“What the hell is cloud computing? . . . I mean, it’s really just complete gibberish.” ~ Larry Ellison, executive chair and chief technology officer of Oracle
“I don’t really worry so much about [AWS], to be very blunt with you. We need to worry about ourselves. We’re in a great position.” ~ Mark Hurd, CEO of Oracle
All of these public statements from entrenched industry leaders remind me of the classic quote by Thomas Watson, chairman of IBM, who in 1943 said, “I think there is a world market for maybe five computers.”
The most impactful and underappreciated aspect of innovation is challenging common and long-held assumptions about how things work. When you create an alternative to these assumptions, expect many doubters.
Being Misunderstood: The Best Sign Of Disruption
Over the years, as Amazon has upset the status quo and disrupted cozy business tradition after cozy business tradition with innovation, the establishment fought back with mockery and dismissals. In Jeff Bezos’s mind, this is being “misunderstood.” If you are going to innovate, you not only have to be willing to be misunderstood but you must also have a thick skin. To many of its competitors, Amazon makes no sense. “It’s the most befuddling, illogically sprawling, and—to a growing sea of competitors—flat-out terrifying company in the world.” If you aren’t upsetting someone, you likely are not disrupting much of anything:
“One thing that I learned within the first couple of years of starting a company is that inventing and pioneering involve a willingness to be misunderstood for long periods of time. One of the early examples of this is customer reviews. Someone wrote to me and said, “You don’t understand your business. You make money when you sell things. Why do you allow these negative customer reviews?” And when I read that letter, I thought, we don’t make money when we sell things. We make money when we help customers make purchase decisions.” ~ Jeff Bezos
Consider the feature Look Inside the Book. In 2001, Amazon launched this program based on a simple concept—the idea of emulating the bookstore experience by allowing Amazon surfers to look at the pages inside of a book before buying. Of course, this required Amazon to house book content in online form on the site, which raised some questions about whether this would expose book content to piracy. Publishers were worried and skeptical. The program would also be very costly. Each book would have to be scanned digitally and indexed, a huge logistical challenge.
Jeff gave the go-ahead for a large-scale launch, recognizing that this was the only way to see whether it would go over with Amazon’s then 43 million active customer accounts. The feature debuted with an astonishing 120,000-plus books. The database took up 20 terabytes, which was about 20 times larger than the biggest database that existed anywhere when Amazon was founded.
David Risher was Amazon’s first vice president of product and store development, responsible for growing the company’s revenue from $16 million to over $4 billion. He described the strategy behind the launch of Look Inside the Book this way: “If we had tried it in a tentative way on a small number of books, say 1,000 or 2,000, it wouldn’t have gotten the PR and the customers’ perception. There’s an X factor: What will it look like in scale? It’s a big investment, and a big opportunity cost. There’s a leap of faith. Jeff is willing to take those gambles.” Ultimately, the publishers embraced the Look Inside the Book program as an asset to sales.
The Value Of Critics
Anytime you do something big, that’s disruptive—Kindle, AWS—there will be critics. And there will be at least two kinds of critics. There will be well-meaning critics who genuinely misunderstand what you are doing or genuinely have a different opinion. And there will be the self-interested critics that have a vested interest in not liking what you are doing, and they will have reason to misunderstand. And you have to be willing to ignore both types of critics. You listen to them, because you want to see, always testing, is it possible they are right? But if you hold back and you say, “No, we believe in this vision,” then you just stay heads down, stay focused, and you build out your vision.
A current example of Amazon being willing to be “misunderstood” is its overall healthcare strategy. By partnering with Berkshire Hathaway and JP Morgan Chase to start the yet unnamed healthcare company headed by Atul Gawande, how will Amazon strive to change healthcare and insurance for their employees? Is their strategy to sell supplies to hospitals? Is it to integrate the PillPack acquisition into a Prime benefit and give customers cheaper prescription deliveries (along with a new book)? Or is it to transform the overall customer experience of healthcare and healthcare insurance and change the cost structure, which is a huge drain on both businesses and employees? Or is it something else? I doubt that Amazon will clarify this in the short-term, and I actually expect that they will add more healthcare investments to their portfolio.
There are two sides to “being misunderstood” to consider. The first is that if your goal is big innovation, in which the customer experience and business model are dramatically changed, then if established stakeholders are not being naysayers, you should be worried. The second side is in planning and preparing your stakeholders, such as investors and partners, for the negative reactions. Amazon, often through the annual shareholder letter, consistently reminds investors that Amazon will look for long-term business results, not sacrifice long-term value for short-term results, and it will be misunderstood, often. Are you willing to be misunderstood?
Questions To Consider
1. When was the last time you did something that benefited customers but upset the traditions of business?
2. What aspects of your customer experience would be different if you started over?
3. What business model innovations could be applied to your industry?
Contributed to Branding Strategy Insider by: John Rossman. Excerpted from his book, Think Like Amazon, 50 1/2 Ideas To Become A Digital Leader (McGraw-Hill)
At The Blake Project we are helping clients from around the world, in all stages of development, redefine and articulate what makes them competitive at critical moments of change through online strategy workshops. Please email us for more.
Branding Strategy Insider is a service of The Blake Project: A strategic brand consultancy specializing in Brand Research, Brand Strategy, Brand Growth and Brand Education
FREE Publications And Resources For Marketers
0 notes