#RPA applications in banking
Explore tagged Tumblr posts
Text
Harnessing Automation: RPA in Banking Industry
Robotic Process Automation (RPA) assists banks and accounting units in automating repetitive manual operations, allowing staff to concentrate on more important activities and giving the company a competitive edge. Let’s discuss more about prominent use cases and benefits of RPA in Banking and Finance.

Harness the power of automation in the banking industry. Explore the multifaceted benefits and applications of RPA for a competitive edge in finance.
#RPA in banking and finance#RPA in banking industry#RPA in banking#RPA in banking sector#RPA automation in banking#benefits of RPA in banking#RPA applications in banking#RPA use cases in banking#RPA in banking and financial sector#RPA in bfsi sector#use of RPA in banking#RPA in bfsi
0 notes
Text
Fintech As A Service Market Size, Share And Trends Analysis Report
The global fintech as a service market size is expected to reach USD 949.49 billion by 2030, growing at a CAGR of 17.5% from 2023 to 2030, according to a new report by Grand View Research, Inc. The increasing adoption of financial technology-based solutions and platforms globally is anticipated to drive the growth of the market. The increasing adoption of cloud-based software, artificial intelligence, and big data integrated with financial services is expected to drive the growth of the market for Fintech-as-a-Service.
In May 2023, Finastra, a global provider of financial software applications and platforms, joined forces with BKN301 Group, a fintech company committed to removing financial obstacles. This collaboration aims to address financial barriers in rapidly expanding markets. The goal is to introduce inventive solutions that foster economic development, facilitating mutual opportunities between these markets and those in the Western regions.
The investments made in financial technology companies are estimated to generate opportunities for market growth over the forecast period. For instance, in July 2021, an API developer, Railsbank Technology Ltd, raised USD 70 million in a fundraising event headed by Anthos Capital, a U.S.-based investment company. The allocated funds will be utilized to advance the company's Fintech-as-a-service platform and offer customers a range of solutions for product development.
The outbreak of the COVID-19 pandemic is expected to play a crucial role in driving the growth of the Fintech-as-a-Service platform market over the forecast period. The increasing demand for digital financial services increased drastically during the pandemic. Banks and financial institutions were looking at innovative ways to provide their services online during the pandemic which bolstered the growth of the market.
Gather more insights about the market drivers, restrains and growth of the Fintech As A Service Market
Fintech As A Service Market Report Highlights
• The fund transfer segment is expected to witness the fastest growth over the forecast period. The fund transfer applications and software being introduced such as Meta Pay by some of the major companies worldwide are expected to drive the growth of the segment
• The artificial intelligence segment is anticipated to witness significant growth over the projected period. Artificial intelligence is experiencing rapid growth due to its ability to automate and optimize financial processes, enhance customer experiences, and provide data-driven insights for better decision-making.
• The KYC verification segment is expected to witness the fastest growth over the forecast period The rising fraudulent activities such as ransomware, and identity theft among others across the globe is one of the major factors that propelled the market growth in 2021.
• The insurance segment is expected to witness the fastest growth over the forecast period. Keeping consumers' changing preferences in mind, insurers are offering flexible-term products and are customizing their offerings according to customer needs. With this changing trend, insurers are adopting Fintech-as-a-Service platforms which is driving the market’s growth
• The Asia Pacific regional market is expected to witness the fastest growth over the forecast period. The growing demand for technology-based payment platforms across the region is expected to play a decisive role in driving the growth of the regional market
Fintech As A Service Market Segmentation
Grand View Research has segmented the global fintech as a service market based on type, technology, application, end-use, and region:
Fintech As A Service Type Outlook (Revenue, USD Billion, 2017 - 2030)
• Payment
• Fund Transfer
• Loan
• Others
Fintech As A Service Technology Outlook (Revenue, USD Billion, 2017 - 2030)
• API
• Artificial Intelligence
• RPA
• Blockchain
• Others
Fintech As A Service Application Outlook (Revenue, USD Billion, 2017 - 2030)
• KYC Verification
• Fraud Monitoring
• Compliance & Regulatory Support
• Others
Fintech As A Service End-use Outlook (Revenue, USD Billion, 2017 - 2030)
• Banks
• Financial Lending Companies
• Insurance
• Others
Fintech As A Service Regional Outlook (Revenue, USD Billion, 2017 - 2030)
• North America
o U.S.
o Canada
• Europe
o Germany
o UK
o France
• Asia Pacific
o China
o India
o Japan
o South Korea
o Australia
• Latin America
o Brazil
o Mexico
• Middle East & Africa (MEA)
o Kingdom of Saudi Arabia (KSA)
o UAE
o South Africa
Order a free sample PDF of the Fintech As A Service Market Intelligence Study, published by Grand View Research.
#Fintech As A Service Market#Fintech As A Service Market Size#Fintech As A Service Market Share#Fintech As A Service Market Analysis#Fintech As A Service Market Growth
0 notes
Text
Hyper automation: Revolutionizing Business Operations in 2025
In today’s fast-paced digital era, businesses are constantly searching for ways to enhance efficiency, reduce costs, and stay competitive. Enter hyper automation—a transformative approach that combines multiple technologies like Artificial Intelligence (AI), Robotic Process Automation (RPA), and Machine Learning (ML) to automate complex processes end-to-end. Hyper automation doesn’t just streamline operations; it redefines the way organizations think about productivity and growth.
As we step into 2025, hyper automation is no longer a buzzword but a strategic priority for enterprises worldwide. Let’s dive into what hyper automation is, why it matters, and how it’s shaping the future of business operations.
What is Hyper automation?
Hyper automation is the advanced application of automation technologies to augment and automate as many business processes as possible. Unlike traditional automation, which focuses on specific repetitive tasks, hyper automation looks at the broader picture, integrating tools and systems to create a fully connected and intelligent workflow.
Key technologies driving hyper automation include:
AI & ML: Enabling systems to learn, adapt, and make intelligent decisions.
RPA: Automating repetitive tasks like data entry and report generation.
Business Process Management (BPM): Streamlining workflows across departments.
Data Analytics: Providing actionable insights through real-time data processing.
IoT Integration: Automating processes across connected devices.
The Benefits of Hyper automation
Enhanced Productivity By automating time-consuming tasks, employees can focus on high-value work like strategy and innovation. For example, automating customer onboarding can reduce manual errors and improve response times.
Cost Efficiency Hyper automation eliminates inefficiencies, reduces operational costs, and enhances ROI. A study by Gartner suggests that organizations leveraging hyper automation can see cost reductions of up to 30%.
Improved Decision-Making AI-driven analytics empower businesses to make informed decisions based on real-time data, enhancing agility and responsiveness to market changes.
Scalability and Flexibility As businesses grow, hyper automation allows them to scale operations without significantly increasing overhead costs.
Better Customer Experiences Automating customer service processes, like chatbots and personalized recommendations, ensures faster and more accurate responses, boosting customer satisfaction.
Key Industries Adopting Hyper automation
Banking and Financial Services From loan approvals to fraud detection, hyper automation is transforming the financial sector by improving accuracy and speed.
Healthcare Automated patient scheduling, claims processing, and even AI-driven diagnostics are revolutionizing healthcare services.
Retail Supply chain optimization, inventory management, and personalized shopping experiences are being enhanced through hyper automation.
Manufacturing Hyper automation in manufacturing leverages IoT and AI for predictive maintenance, production planning, and quality control.
Challenges in Hyper automation Implementation
While hyper automation offers numerous advantages, its implementation is not without challenges:
Integration Complexity: Combining various technologies into a cohesive system requires expertise and investment.
Data Security Concerns: Increased automation can expose vulnerabilities if proper security measures aren’t in place.
Change Management: Employees may resist transitioning to automated processes, requiring proper training and communication.
The Future of Hyper automation
As we move into 2025, hyper automation is set to become even more sophisticated and accessible. Here are some trends to watch:
AI-First Automation: More businesses will leverage AI for decision-making, making workflows smarter and more autonomous.
Citizen Development: Low-code platforms will enable non-technical employees to create and manage automated workflows, democratizing hyper automation.
Sustainability Integration: Automation will play a key role in helping organizations meet their sustainability goals by optimizing resource usage and reducing waste.
Increased Adoption Across SMEs: Small and medium-sized enterprises will embrace hyper automation as tools become more affordable and scalable.
Conclusion
Hyper automation is more than just a technological advancement; it’s a paradigm shift in how businesses operate. By integrating intelligent technologies, organizations can achieve unprecedented efficiency, adaptability, and innovation.
As 2025 unfolds, the question is no longer if businesses should adopt hyper automation but how quickly they can do so to remain competitive. Whether you’re a startup or a multinational corporation, hyper automation holds the key to unlocking the future of work.
Are you ready to embrace hyper automation? Share your thoughts in the comments!
0 notes
Text
Retail Banking System Market Trends: Navigating the Future of Financial Services
The retail banking system Market has undergone dramatic shifts in recent years, driven by rapid technological advancements, changing consumer preferences, and the evolving regulatory landscape. These shifts have paved the way for a new era in banking, one that emphasizes digital innovation, personalization, and financial inclusion. As we move into 2025 and beyond, the retail banking system market is set to experience further transformation. Understanding the key trends that are shaping the future of financial services is essential for both banking institutions and consumers to navigate this evolving landscape.

1. Digital and Mobile Banking Innovation
The most significant trend in the retail banking system market is the rise of digital and mobile banking. Technology has completely reshaped the way consumers interact with their financial institutions. Digital transformation in the banking sector was already gaining momentum before the COVID-19 pandemic, but the crisis accelerated the adoption of digital banking services as consumers and businesses sought safer, more convenient ways to manage their finances without physically visiting bank branches.
Mobile banking applications have become the cornerstone of this transformation. These apps provide customers with 24/7 access to their accounts, enabling them to check balances, transfer funds, pay bills, apply for loans, and even invest in stocks with the touch of a button. Furthermore, the increasing penetration of smartphones and improved internet connectivity globally has expanded access to these services, especially in emerging markets.
Alongside mobile banking apps, digital-only banks (also known as neobanks) have gained traction. These banks offer a fully online experience, with no physical branches, providing consumers with lower fees, higher interest rates, and user-friendly interfaces. Neobanks are often more agile than traditional banks, enabling them to offer innovative financial products and better customer experiences. As these digital-only banks grow, traditional banks are investing heavily in their own digital banking solutions to stay competitive.
2. The Integration of Artificial Intelligence and Automation
Another prominent trend is the increasing integration of artificial intelligence (AI) and automation within retail banking systems. AI is transforming the banking sector by enabling banks to offer more personalized services, improve operational efficiency, and enhance customer experience.
AI-driven solutions, such as chatbots and virtual assistants, are becoming commonplace in retail banking. These tools can provide customers with real-time support, assist with transaction queries, and offer financial advice at any time of day. For example, AI-powered chatbots are helping to reduce the need for human intervention in customer service, which not only enhances efficiency but also lowers operational costs.
Moreover, banks are using AI to analyze vast amounts of customer data to gain insights into consumer behavior and preferences. This data can then be used to personalize product offerings, recommend services, and improve overall customer satisfaction. Machine learning algorithms are also being employed to detect fraudulent activity in real-time, improving security and minimizing risk.
Automation, powered by AI and robotic process automation (RPA), is also streamlining many of the repetitive, time-consuming tasks that were previously handled manually by bank employees. These processes include data entry, transaction processing, and compliance checks. By automating these tasks, banks can improve accuracy, speed, and efficiency, while freeing up human resources for more value-added activities.
3. Open Banking and API Integrations
Open banking is one of the most disruptive trends in the retail banking market. Open banking refers to the practice of sharing financial data and services across different banks and third-party providers via secure application programming interfaces (APIs). This trend allows customers to access a broader range of financial products and services that can be integrated into their banking experience.
The implementation of open banking is being driven by regulatory changes, such as the European Union’s Payment Services Directive 2 (PSD2), which mandates that banks must allow third-party providers to access customer data (with the customer’s consent) to foster innovation and competition in the financial sector. In many cases, open banking enables consumers to access services such as budgeting tools, personalized investment advice, and alternative lending options from non-traditional financial institutions.
Open banking has also paved the way for financial technology (fintech) companies to partner with banks and offer new services that were previously unavailable. For example, many fintech firms now provide loans, payments, and insurance products directly through banking platforms. This collaboration between banks and fintech companies is driving innovation and creating more diverse offerings for consumers.
With the rise of open banking, banks are increasingly focused on API integration and developing platforms that enable seamless, secure data sharing between institutions and third-party providers. This trend is transforming how financial services are delivered and is expected to continue to gain momentum in the years ahead.
4. Emphasis on Financial Inclusion
As the global financial landscape evolves, there is a growing emphasis on improving financial inclusion, especially in developing regions. Traditionally, a large portion of the global population has been excluded from formal banking services, particularly in emerging markets. However, the increasing adoption of digital banking solutions, mobile phones, and internet connectivity is helping to bridge this gap.
Digital financial services, such as mobile wallets and mobile money platforms, have emerged as powerful tools for promoting financial inclusion. These services provide individuals in underserved or unbanked areas with the ability to access basic banking functions, such as money transfers, bill payments, and savings, using just their mobile phones. Companies like M-Pesa in Africa have revolutionized the way people access and manage money, bringing financial services to millions who previously had no access to banking.
Retail banks are also recognizing the importance of extending their services to underserved populations. Many are investing in mobile-first solutions, microloans, and digital lending platforms to provide financial access to small businesses and individuals who may not have the credit history required to access traditional banking products. Additionally, fintech companies are playing a key role in promoting financial inclusion by offering low-cost financial services and innovative lending solutions tailored to the needs of underserved groups.
Conclusion
The retail banking system is undergoing a profound transformation, driven by key trends such as digital banking, AI and automation, open banking, and financial inclusion. These trends are reshaping how banks interact with customers, streamline operations, and offer new, innovative financial products. As consumers increasingly demand more personalized, accessible, and convenient banking experiences, retail banks must continue to adapt and innovate to stay relevant.
As we look toward the future of financial services, the importance of technology in shaping the industry will only grow. Retail banks that embrace digital transformation, collaborate with fintechs, and focus on customer-centric solutions will be best positioned to thrive in an increasingly competitive and dynamic market. By navigating these emerging trends effectively, financial institutions can continue to meet the evolving needs of customers and remain at the forefront of the retail banking sector.
0 notes
Text
Operational Efficiency
Operational efficiency is a critical goal for any organization aiming to optimize performance, reduce costs, and improve productivity. Data Science plays a pivotal role in achieving this by leveraging data to streamline operations, automate processes, and enhance decision-making. This section explores how Data Science contributes to operational efficiency through various applications and methodologies.
Automation
Automation is a significant driver of operational efficiency, and Data Science is at the forefront of this revolution. By automating repetitive and mundane tasks, organizations can free up human resources for more strategic activities.
Robotic Process Automation (RPA)
Robotic Process Automation involves the use of software robots to automate routine tasks such as data entry, invoicing, and customer onboarding. RPA not only reduces the time required to perform these tasks but also minimizes human error, ensuring higher accuracy and efficiency.
Data Entry: Automating data entry processes reduces manual errors and speeds up data processing. For example, banks use RPA to process loan applications, extracting and inputting data from forms automatically.
Invoice Processing: RPA can handle invoice processing by extracting information from invoices, matching it with purchase orders, and entering it into accounting systems. This streamlines the accounts payable process and reduces processing time.
Customer Onboarding: In industries like finance and telecom, RPA helps in automating the customer onboarding process by verifying documents, conducting background checks, and setting up accounts, thereby enhancing the customer experience.
Intelligent Automation
Intelligent Automation combines RPA with Artificial Intelligence (AI) and Machine Learning (ML) to handle more complex tasks that require cognitive capabilities. This advanced form of automation is capable of making decisions, learning from data, and adapting to new situations.
Customer Service: AI-driven chatbots and virtual assistants provide 24/7 customer support, handling inquiries and resolving issues without human intervention. This not only improves customer satisfaction but also reduces the workload on human agents.
Fraud Detection: Intelligent automation systems analyze transaction patterns to detect fraudulent activities in real-time. For example, credit card companies use AI models to identify and block suspicious transactions automatically.
Supply Chain Optimization: Intelligent automation optimizes supply chain operations by predicting demand, managing inventory, and coordinating logistics. AI models can suggest optimal routes for delivery trucks, reducing fuel costs and delivery times.
Workflow Optimization
Data Science helps organizations optimize their workflows by identifying inefficiencies and bottlenecks in business processes. By analyzing process data, companies can streamline operations and improve productivity.
Employee Performance: Analyzing employee performance data helps in identifying areas for improvement and optimizing workforce allocation. For example, call centers use performance analytics to schedule shifts based on call volume predictions.
Production Processes: In manufacturing, data science analyzes production line data to identify inefficiencies and optimize workflows. For instance, predictive maintenance models can schedule maintenance activities during non-peak hours, reducing downtime.
Document Management: Automating document management processes, such as filing, retrieving, and sharing documents, improves efficiency and reduces the risk of errors. This is particularly important in sectors like healthcare and legal services where document handling is critical.
Resource Management
Efficient resource management is essential for maximizing productivity and minimizing waste. Data Science provides the tools needed to achieve optimal resource allocation and utilization.
Human Resources Management
Data Science plays a crucial role in managing human resources by analyzing employee data to optimize recruitment, performance, and retention strategies.
Recruitment: Predictive analytics models analyze historical hiring data to identify the characteristics of successful employees. This helps in screening and selecting candidates who are more likely to perform well and stay with the company.
Performance Management: Data-driven performance management systems track employee performance metrics and provide insights into areas for improvement. This helps in setting realistic goals, providing targeted training, and recognizing top performers.
Retention Strategies: Predictive models identify employees at risk of leaving the organization by analyzing factors such as job satisfaction, engagement levels, and career progression. This enables HR teams to implement proactive retention strategies, such as personalized career development plans.
Asset Management
Effective management of physical assets is crucial for reducing operational costs and maximizing their lifespan. Data Science helps in monitoring and maintaining assets more efficiently.
Predictive Maintenance: Predictive maintenance models analyze sensor data from equipment to predict potential failures before they occur. This allows for scheduled maintenance activities, reducing downtime and maintenance costs.
Inventory Optimization: Data Science optimizes inventory management by predicting demand and adjusting stock levels accordingly. For example, retailers use demand forecasting models to ensure they have the right products in stock without overstocking.
Asset Utilization: By analyzing usage patterns, organizations can optimize the utilization of their assets. For instance, transportation companies use telematics data to optimize fleet usage and reduce fuel consumption.
Energy Management
Data Science is instrumental in optimizing energy consumption, leading to cost savings and environmental benefits.
Smart Grids: Smart grids use data analytics to monitor and manage electricity distribution in real-time. This ensures efficient energy use, reduces losses, and integrates renewable energy sources more effectively.
Building Management: Data science optimizes energy use in buildings by analyzing data from HVAC systems, lighting, and other utilities. Smart building systems adjust energy consumption based on occupancy patterns and external weather conditions.
Industrial Processes: In industrial settings, data analytics optimize energy use in production processes. For example, predictive models can adjust machinery settings to minimize energy consumption while maintaining production efficiency.
Risk Management Managing risk is crucial for the stability and growth of any organization. Data Science enhances risk management through predictive analytics and real-time monitoring.
Financial Risk Management
Financial institutions rely on Data Science to assess and mitigate various types of financial risk.
Credit Risk: Predictive models analyze credit history, transaction data, and other factors to assess the creditworthiness of loan applicants. This helps banks make informed lending decisions and reduce default rates.
Market Risk: Data Science models analyze market trends and economic indicators to predict potential market risks. This allows financial institutions to adjust their investment strategies and minimize losses.
Operational Risk: Analyzing operational data helps in identifying and mitigating risks associated with internal processes, systems, and human errors. For example, banks use data analytics to monitor compliance with regulatory requirements and prevent operational failures.
Operational Risk Management
Data Science helps organizations identify and mitigate operational risks across various domains.
Supply Chain Risks: Predictive analytics models forecast potential supply chain disruptions due to factors like natural disasters, geopolitical events, or supplier issues. This enables companies to develop contingency plans and ensure business continuity.
Equipment Failure: Predictive maintenance models analyze sensor data from machinery to predict equipment failures. This allows for proactive maintenance, reducing downtime and preventing costly breakdowns.
Compliance and Safety: Data Science helps organizations monitor compliance with safety regulations and standards. For example, in the construction industry, analytics models analyze safety data to identify potential hazards and implement preventive measures.
Cybersecurity
In the digital age, cybersecurity is a critical concern for organizations. Data Science plays a key role in enhancing cybersecurity through anomaly detection and threat prediction.
Anomaly Detection: Machine learning models analyze network traffic to detect unusual patterns that may indicate a cyber attack. For example, sudden spikes in data transfer or unusual login activities can trigger alerts for further investigation.
Threat Prediction: Predictive analytics models identify potential threats by analyzing historical attack data and emerging threat patterns. This helps organizations implement preventive measures and strengthen their security posture.
Incident Response: Data Science aids in the incident response process by analyzing attack patterns and determining the source and impact of a breach. This enables quicker resolution and minimizes damage.
Cost Reduction
Reducing operational costs is a primary objective for businesses aiming to improve their bottom line. Data Science helps organizations identify cost-saving opportunities and implement efficient practices.
Supply Chain Optimization
Optimizing supply chain operations through data analytics leads to significant cost savings.
Demand Forecasting: Predictive models forecast demand for products, helping businesses maintain optimal inventory levels. This prevents overstocking and stockouts, reducing inventory carrying costs and lost sales.
Logistics Efficiency: Data Science optimizes logistics by analyzing transportation data to identify the most efficient routes and modes of delivery. This reduces fuel consumption, delivery times, and transportation costs.
Procurement Strategies: Analyzing procurement data helps organizations identify cost-saving opportunities in supplier selection, contract negotiation, and purchasing practices. For example, companies can use spend analysis to consolidate purchases and negotiate better terms with suppliers.
Operational Efficiency
Streamlining operations through data-driven insights leads to cost savings and improved productivity.
Process Automation: Automating routine tasks reduces labor costs and increases efficiency. For example, automating invoice processing reduces the need for manual data entry, speeding up the accounts payable process.
Workflow Optimization: Analyzing workflow data helps identify inefficiencies and optimize business processes. For instance, lean manufacturing principles combined with data analytics can reduce waste and improve production efficiency.
Resource Allocation: Data Science optimizes resource allocation by predicting demand and adjusting staffing levels accordingly. For example, call centers use predictive models to schedule shifts based on call volume forecasts, reducing labor costs.
Energy Savings
Optimizing energy consumption leads to significant cost savings and environmental benefits.
Smart Energy Management: Data Science enables smart energy management systems that monitor and control energy use in real-time. For example, smart thermostats adjust heating and cooling settings based on occupancy and weather conditions, reducing energy costs.
Industrial Energy Efficiency: Analyzing energy consumption data in industrial processes helps identify inefficiencies and implement energy-saving measures. For instance, optimizing machine settings can reduce energy use without compromising production output.
Renewable Energy Integration: Data Science helps integrate renewable energy sources into the grid more effectively. Predictive models forecast energy production from solar and wind sources, allowing for better planning and utilization.
Quality Control
Maintaining high-quality standards is essential for customer satisfaction and business success. Data Science enhances quality control through advanced analytics and real-time monitoring.
Manufacturing Quality
In manufacturing, maintaining product quality is crucial for customer satisfaction and regulatory compliance. Data Science helps in monitoring and controlling quality throughout the production process.
Defect Detection: Machine learning models analyze production data to detect defects and anomalies in real-time. This allows for immediate corrective actions, reducing the number of defective products and ensuring high-quality standards.
Process Control: Statistical process control (SPC) techniques combined with data analytics monitor production processes to ensure they stay within predefined quality limits. For example, analyzing temperature and pressure data in real-time helps maintain optimal conditions for product manufacturing.
Supplier Quality Management: Data Science helps in monitoring the quality of raw materials supplied by vendors. By analyzing supplier performance data, companies can identify reliable suppliers and negotiate better terms.
Service Quality
In service industries, maintaining high service quality is critical for customer retention and competitive advantage. Data Science provides insights into service performance and customer satisfaction.
Customer Feedback Analysis: Analyzing customer feedback and reviews helps in identifying areas for improvement. For example, sentiment analysis of social media comments provides insights into customer perceptions and experiences.
Service Performance Metrics: Monitoring key performance indicators (KPIs) such as response time, resolution time, and customer satisfaction scores helps in maintaining high service standards. Data-driven insights enable service managers to address issues promptly and improve service quality.
Personalized Service: Data Science enables personalized service experiences by analyzing customer data and preferences. For instance, recommendation systems in online platforms suggest products and services based on individual customer profiles.
Compliance
Ensuring compliance with regulations and standards is critical for avoiding penalties and maintaining reputation. Data Science helps organizations monitor compliance and identify potential issues.
Regulatory Compliance: Analyzing compliance data helps organizations ensure they meet regulatory requirements. For example, financial institutions use data analytics to monitor transactions and detect potential money laundering activities.
Safety Standards: In industries such as healthcare and manufacturing, maintaining safety standards is crucial. Data Science helps in monitoring safety data and identifying potential hazards, ensuring compliance with safety regulations.
Audit and Reporting: Data-driven audit and reporting systems provide accurate and timely information for regulatory audits. This reduces the risk of non-compliance and ensures transparency in business operations.
Inventory Management
Effective inventory management is essential for minimizing costs and meeting customer demand. Data Science optimizes inventory levels through predictive analytics and real-time monitoring.
Demand Forecasting
Accurate demand forecasting is crucial for maintaining optimal inventory levels. Data Science models analyze historical sales data, market trends, and other factors to predict future demand.
Seasonal Trends: Predictive models account for seasonal variations in demand, helping businesses stock up on popular items during peak seasons and reduce inventory during off-peak periods.
Market Conditions: Analyzing market conditions and consumer behavior provides insights into potential demand shifts. For example, economic indicators and competitor activities can influence demand for certain products.
Promotional Impact: Data Science helps in predicting the impact of promotional activities on demand. This enables businesses to adjust inventory levels and avoid stockouts or overstock situations.
Inventory Optimization
Optimizing inventory levels involves balancing the cost of holding inventory with the need to meet customer demand. Data Science provides the tools needed to achieve this balance.
Safety Stock: Predictive models calculate optimal safety stock levels to buffer against uncertainties in demand and supply. This ensures that businesses can meet customer demand without incurring excessive holding costs.
Reorder Points: Data Science determines optimal reorder points based on demand forecasts and lead times. This helps in maintaining inventory levels and preventing stockouts.
Inventory Turnover: Analyzing inventory turnover ratios helps in identifying slow-moving and fast-moving items. This enables businesses to make informed decisions about purchasing, stocking, and pricing strategies.
Supply Chain Coordination
Effective supply chain coordination is essential for optimizing inventory management. Data Science enhances visibility and collaboration across the supply chain.
Real-Time Monitoring: Real-time monitoring systems provide visibility into inventory levels and movement across the supply chain. This enables businesses to respond quickly to changes in demand and supply conditions.
Supplier Collaboration: Data-driven insights enhance collaboration with suppliers, ensuring timely deliveries and reducing lead times. For example, sharing demand forecasts with suppliers helps in synchronizing production and delivery schedules.
Logistics Optimization: Data Science optimizes logistics operations by analyzing transportation data to identify the most efficient routes and modes of delivery. This reduces transportation costs and improves delivery times.
Warehouse Management
Efficient warehouse management is crucial for optimizing inventory storage and retrieval processes. Data Science provides insights into warehouse operations and helps in improving efficiency.
Layout Optimization: Analyzing warehouse layout data helps in optimizing storage space and reducing retrieval times. For example, frequently picked items can be stored closer to the shipping area to speed up order fulfillment.
Inventory Tracking: Real-time inventory tracking systems provide accurate information about inventory levels and locations within the warehouse. This reduces the risk of stockouts and overstock situations.
Order Fulfillment: Data Science optimizes order fulfillment processes by analyzing order data and warehouse operations. For example, batch picking strategies can reduce the time required to pick and pack orders, improving overall efficiency.
In conclusion, Data Science is a powerful tool for enhancing operational efficiency across various domains. By leveraging data-driven insights, organizations can automate processes, optimize resource management, manage risks effectively, reduce costs, maintain high-quality standards, and manage inventory efficiently. As data continues to grow in volume and complexity, the role of Data Science in driving operational efficiency will only become more critical, shaping the future of business and industry.
Read More on Strategic Leap
0 notes
Text
Digital Transformation for Bank: Embracing Automation in Banking and Financial Services
The monetary business is going through a seismic shift, with Digital Transformation for banks turning into the foundation of progress. With developing client requests and savage rivalry, automation in banking and financial services isn't simply a decision — it's a need. Be that as it may, what's the significance here for banks and monetary foundations, and how might they use innovation to remain ahead? We should jump into the upheaval that is reclassifying the monetary area.

What is Digital Transformation for a Bank?
Digital Transformation for banks alludes to the reception of trend setting innovations to smooth out activities, upgrade client encounters, and lift functional proficiency. By utilizing instruments, for example, simulated intelligence, AI, and information investigation, banks are moving towards a future where manual cycles become outdated.
For banks, this change isn't just about offering on the web administrations. It includes rethinking cycles to make them quicker, more astute, and more customized. From portable banking applications to man-made intelligence driven client assistance chatbots, advanced change is making ready for more intelligent tasks and expanded consumer loyalty.
The Role of Automation in Banking and Financial Services
Mechanization in banking and monetary administrations has turned into a unique advantage. By decreasing manual mediation, monetary foundations are accomplishing accuracy, effectiveness, and speed more than ever. This is the way robotization is having an effect:
1. Smoothed out Cycles
Manual cycles like credit endorsements, KYC checks, and record taking care of are currently robotized, diminishing time required to circle back and human mistakes. For example, Mechanical Cycle Computerization (RPA) empowers banks to handle large number of exchanges with unparalleled exactness.
2. Upgraded Client care
Computerization empowers all day, every day client care through man-made intelligence controlled chatbots and remote helpers. Clients can ask about balances, resolve inquiries, or even apply for credits without visiting a branch.
3. Risk The executives and Misrepresentation Recognition
High level calculations fueled via Digital Transformation For Banks are assisting manages an account with recognizing misrepresentation continuously, guaranteeing the security of exchanges and safeguarding client information.
4. Cost Decrease
Robotizing administrative center tasks saves critical functional expenses while further developing exactness. This permits banks to divert assets to advancement and better assistance conveyance.
Why is Digital Transformation Crucial for Banks Today?
Banks face various difficulties in the present powerful market, including:
Rising client assumptions for quicker and consistent administrations.
Expanded rivalry from fintech new companies.
Administrative tensions and consistence necessities.
Information driven dynamic requirements.
By focusing on Digital Transformation for banks, monetary establishments can address these difficulties head-on. Automation in Banking and Financial Services empowers banks to measure up to assumptions as well as surpass them.
Key Advantages of Automation and Digital Transformation for Banks
Further developed Client Experience: Customized administrations, fast reactions, and simple admittance to banking administrations.
Expanded Effectiveness: Computerization lessens delays and guarantees consistent work processes.
Adaptability: As advanced stages develop, banks can extend their administrations without compromising execution.
Information Experiences: simulated intelligence controlled investigation assist manages an account with understanding client conduct, settling on information driven choices.
Future Patterns in Banking Automation and Digital Transformation
As innovation keeps on developing, here are a few patterns that will additionally change the business:
Man-made intelligence and AI: Prescient investigation for risk evaluation and client commitment.
Blockchain Innovation: Guaranteeing secure, straightforward exchanges.
Cloud Banking: Versatile framework for more prominent readiness and cost reserve funds.
Open Banking: Cooperative models to make imaginative monetary items.
Conclusion
The excursion toward Digital Transformation For Banks and Digital Transformation For Banks is one loaded up with open doors. Monetary establishments that embrace these progressions will flourish in an undeniably advanced world, offering outstanding client encounters and functional productivity.
Banks that defer this change risk being abandoned. This is the ideal opportunity to enhance, robotize, and make a vigorous computerized biological system that takes care of developing business sector requests. The eventual fate of banking is advanced — and it's as of now here!
#digital transformation for bank#banking financial document automation#automation in banking and financial services#ckyc automation for banks india
0 notes
Text
The Transformative Impact of Generative AI on Process Automation

The Transformative Impact of Generative AI in Process Automation In today's fast-paced digital age, organizations across the industries are constantly looking for innovative solutions to enhance efficiency, reduce costs, and optimize processes. Generative AI, with its ability to create, predict, and adapt, is emerging as a game-changing technology in the realm of process automation. This transformative combination is driving major advancements in productivity, decision-making, and operational agility. In this report, we will dive further into the deep impact of AI-generated processes on automation and reveal to you how businesses can utilize it right. What is Generative AI? Generative AI refers to the capability of artificial intelligence systems to create new data or content in ways that are similar to humans generating such outputs. Trained on huge datasets, these systems can produce text, images, music, and even predictive models. Generative AI has found application in popular applications such as NLP, image synthesis, and conversational AI. Generative AI extends beyond the rule-based traditional system when combined with automation frameworks. Organizations are able to rethink workflows and redefine efficiency through this integration. Process Automation Process automation refers to the use of technology to perform repetitive work tasks and streamline workflows. RPA has been considered one of the key points in digital transformation. This will allow businesses to minimize workloads and enhance accuracy in general work.
However, traditional RPA systems are often limited by their dependence on structured data and predefined rules. This is where Generative AI and Robotic Process Automation come into play, offering a synergistic solution that combines the strengths of both technologies.
How Generative AI Improves Process Automation Generative AI improves traditional automation systems in several revolutionary ways: 1. Sophisticated Decision-Making Generative AI models can analyze huge amounts of unstructured data and generate insights to support decision-making processes. This allows businesses to perform complex workflows, such as financial forecasting or customer behavior analysis, with greater accuracy. 2. Dynamic Adaptability Unlike traditional automation systems where the rules are static, generative AI learns and evolves, hence ideal for industries which are dynamic in nature such as healthcare, finance, and e-commerce. 3. Intelligent Document Processing The process of document analysis, summarization, and content generation with the help of generative AI can be automated by organizations. As an example, relevant details can be extracted from the contract or customer feedback with AI in place, thereby significantly bringing down manual efforts. 4. Natural Language Interaction Generative AI NLP capabilities facilitate more intuitive interactions with automation systems. Chatbots with generative AI can be used to address customer inquiries, provide customized responses, and refer complex issues with no effort. 5. Predictive Analytics and Risk Management Through the analysis of historical data, generative AI can determine patterns and predict outcomes. This will enable businesses to proactively reduce risks and optimize operations. Real-World Applications of Generative AI in Process Automation 1. Financial Services Banks and financial institutions are using generative AI to detect fraud, analyze credit risk, and generate reports automatically. These applications increase accuracy and reduce operational costs. 2. Healthcare In the healthcare industry, generative AI is used in medical imaging, automated patient scheduling, and even drug discovery by analyzing molecular structures. 3. Manufacturing Manufacturers use generative AI for predictive maintenance, supply chain optimization, and automated quality control. By integrating AI with robotics, factories are achieving higher efficiency and precision. 4. Customer Service Generative AI-powered chatbots and virtual assistants are transforming customer service by delivering instant, context-aware responses. This integration enhances user satisfaction while reducing the burden on human agents.
5. Marketing and Content Creation
Marketing teams utilize generative AI for personalized campaign creation, automated ad copy generation, and sentiment analysis. This allows brands to resonate better with their audience.
For businesses seeking the Best Generative AI for Process Automation, the right tools can unlock unparalleled efficiency and innovation.
Advantages of Generative AI in Automating Processes The incorporation of generative AI into process automation has several practical advantages listed below: Improved Efficiency: Automatic time-consuming processes free up personnel to focus on strategic activities of high value. Reduce Costs Automation through AI tends to reduce the amount of manual intervention, thereby saving tremendous amounts of money. Increase Scalability Generative AI allows businesses to scale operation without a proportional increase in resources. Precise Results AI reduces errors associated with hand operations, thereby providing consistent and quality results. Customer Satisfaction: Intelligent automation provides more personalized and timely customer engagement, leading to improved satisfaction and loyalty. Obstacles of Adoption Although it has innumerable benefits, there are obstacles to the adoption of generative AI in automation: Privacy of Data: Processing sensitive information requires high security to prevent breaches Skill Gap: AI-based systems necessitate a high level of expertise and, therefore, require investing in training and recruitment. Integrating with Legacy Systems: Most organizations find it challenging to integrate AI into their old infrastructure. Such challenges will require strategic planning, being around trusted providers, and encouraging innovative cultures. Generative AI and Robotic Process Automation platforms can provide business-specific solutions for such complexity. Future of Generative AI: Process Automation Generative AI combined with automation is the future of how business operates. Focus areas: Hyperautomation: AI meets RPA, along with machine learning, to create end-to-end automated processes. AI-Inspired Innovation: The generative AI would evoke innovation in business models and services across industries. Human-AI Symbiosis: Automation shall enhance human ability, marrying the worlds of creativity with efficiency. Conclusion Generative AI revolutionizes process automation by making workflow intelligent, adaptable, and creative. Applications lie in all industrial sectors. Thus, it helps to achieve hitherto unforeseen efficiencies and innovation levels within organizations. It is with such solutions as Generative AI and Robotic Process Automation and the Best Generative AI for Process Automation that businesses will remain competitive in the landscape. The embracing of this technology is no longer an option but a necessity for any organization to thrive in the digital world. The future of process automation has already arrived, and it runs on the limitless power of generative AI.
0 notes
Text
How RPA is Driving Transformation in the Banking Sector
The banking industry is undergoing a significant transformation, fueled by technological advancements and the need to enhance operational efficiency. Among the most powerful tools driving this change is Robotic Process Automation (RPA). With its ability to streamline complex tasks and improve accuracy, RPA is reshaping the future of banking, offering immense benefits for both financial institutions and their customers.
What Exactly is RPA?
Robotic Process Automation (RPA) is a technology that uses software robots or "bots" to automate repetitive, rule-based tasks across various applications and systems. These bots perform the same tasks that humans would do, but they do so much faster, with greater precision, and without the risk of human error. RPA is particularly valuable in industries like banking, where there is a high volume of transactions, data management, and compliance requirements.
RPA’s Impact on Banking Operations
Accelerated Transaction Processing: With RPA in place, tasks like processing payments, verifying transactions, and updating customer accounts can be completed much faster than manual methods. This reduces delays and increases the overall speed of operations, leading to quicker service for customers.
Cost Savings: Financial institutions can save significant amounts of money by automating repetitive tasks. This reduces the need for human labor in routine processes and allows banks to reallocate resources toward more strategic initiatives like innovation and customer engagement.
Enhanced Accuracy and Reduced Risk: RPA bots are designed to follow strict rules, ensuring tasks like data entry and document processing are completed with high accuracy. This level of precision is crucial in areas like regulatory compliance, where even minor errors can lead to hefty penalties.
Improved Customer Service: As RPA handles back-office processes, banking staff can focus on higher-value customer-facing tasks. This translates to better customer service, with faster response times, personalized experiences, and smoother interactions overall.
Real-World Applications of RPA in Banking
Customer Onboarding: Opening a bank account involves multiple steps, including verifying personal details, validating documents, and credit checks. RPA can automate many of these steps, making the onboarding process faster and more efficient for both banks and customers.
Account Management: Routine account management tasks, such as updating customer information, verifying account details, and processing transactions, can be automated with RPA. This not only increases efficiency but also ensures that data is kept up to date and accurate.
Fraud Detection: RPA can be utilized to monitor transactions in real time, flagging suspicious activities for further review. Bots can analyze patterns and detect anomalies, reducing the risk of fraud and improving security.
Loan Processing: Banks are using RPA to automate the loan approval process, from collecting application data to verifying financial documents. This accelerates the approval timeline, providing customers with faster responses while reducing administrative costs for the bank.
Compliance and Reporting: Staying compliant with ever-changing regulations is a constant challenge for banks. RPA can automate regulatory reporting, ensuring that all necessary documents are prepared accurately and submitted on time, reducing the burden on compliance teams.
The Future of RPA in Banking
As RPA continues to evolve, its integration with other cutting-edge technologies, such as Artificial Intelligence (AI) and Machine Learning (ML), will unlock even greater potential. AI-powered bots will be able to handle more complex tasks, from customer sentiment analysis to predictive analytics, providing banks with deeper insights and enhanced decision-making capabilities.
Furthermore, with the increasing adoption of RPA, banks will be able to offer more tailored and innovative services, pushing the boundaries of what is possible in the financial sector.
Conclusion
RPA is no longer a buzzword in banking; it’s a critical tool that is enabling financial institutions to stay competitive, enhance customer experiences, and improve operational efficiency. By automating routine processes, banks can focus on strategic initiatives that drive growth and innovation. The future of banking is digital, and RPA is at the heart of this transformation.
At Code Stew, we specialize in helping businesses harness the power of RPA to streamline operations and achieve digital transformation. Stay tuned for more insights and best practices on how RPA is changing the face of banking.
#RPAinBanking#AutomationForBanks#DigitalBanking#RoboticAutomation#AIinFinance#BankingTransformation#FintechInnovation#BankingSolutions#CustomerService
1 note
·
View note
Text
Exploring the Role of AI in the Future of Banking and Finance
Artificial Intelligence (AI) has emerged as a transformative force across industries, and the financial services sector is no exception. From streamlining operations to improving customer experiences, AI is reshaping the way financial institutions operate and innovate. As we look to the future, it becomes evident that AI development company will play a pivotal role in driving efficiency, enabling better decision-making, and opening new avenues for growth in financial services.

1. Enhanced Customer Experience
AI is revolutionizing customer interactions in financial services by making them more personalized and efficient. Chatbots and virtual assistants powered by natural language processing (NLP) are becoming the norm, offering 24/7 support to customers.
Personalized Financial Advice: AI can analyze customer data to provide tailored advice on savings, investments, and expense management. For example, robo-advisors use AI algorithms to offer investment recommendations based on an individual’s financial goals and risk tolerance.
Improved Accessibility: AI-powered tools help bridge language barriers and provide financial services to underserved populations, enhancing inclusivity.
In the future, we can expect AI to offer even more sophisticated and intuitive interfaces, making financial services more accessible to a broader audience.
2. Fraud Detection and Risk Management
Fraud detection and risk management are among the most critical applications of AI in financial services. AI systems can analyze vast amounts of data in real-time, identifying unusual patterns or transactions that may indicate fraudulent activity.
Advanced Fraud Detection: AI uses machine learning to identify anomalies in transaction data, flagging suspicious activities with greater accuracy than traditional rule-based systems.
Risk Assessment: AI models can predict credit risk by analyzing diverse data points, from financial history to social behavior, allowing lenders to make more informed decisions.
In the future, AI will continue to evolve, leveraging advancements in predictive analytics and behavioral biometrics to combat increasingly sophisticated fraud schemes.
3. Process Automation
AI-driven automation is streamlining back-office operations, reducing costs, and improving efficiency. Tasks such as document processing, compliance checks, and reconciliation are being automated using AI.
Robotic Process Automation (RPA): Combining AI with RPA enables financial institutions to automate repetitive tasks, such as data entry and account updates, freeing up human employees for more strategic roles.
Compliance Automation: AI can help financial institutions stay compliant with ever-changing regulations by automatically monitoring and reporting irregularities.
As automation technology advances, we can anticipate an even greater reduction in operational overhead and faster processing times across financial institutions.
4. Predictive Analytics for Business Growth
AI’s ability to analyze historical data and identify trends is helping financial institutions make data-driven decisions.
Market Predictions: AI models can analyze financial markets to predict trends, providing valuable insights for traders and investors.
Customer Retention: Predictive analytics can identify customers who are likely to churn, allowing banks to take proactive measures to retain them.
Product Development: Financial institutions can use AI to identify unmet customer needs and develop products or services tailored to those gaps.
Future advancements in AI will make these predictions more precise, enabling financial institutions to stay ahead in an increasingly competitive market.
5. Personalized Wealth Management
Wealth management is another area where AI is making significant inroads. Robo-advisors are already transforming investment strategies for individual and institutional clients.
Dynamic Portfolio Management: AI can continuously monitor and adjust investment portfolios based on market conditions and individual preferences.
Enhanced Client Insights: AI tools provide wealth managers with deep insights into client behavior, enabling more personalized advice.
As AI systems grow more sophisticated, they will likely offer hyper-personalized strategies that align with real-time market dynamics and individual client goals.
6. AI in Financial Inclusion
AI is playing a crucial role in expanding access to financial services for underserved populations, particularly in developing economies.
Micro-lending Platforms: AI assesses creditworthiness based on alternative data sources, such as mobile payment histories and social media activity, enabling loans to individuals without traditional credit histories.
Blockchain and AI Integration: The integration of AI with blockchain can facilitate secure and transparent transactions for the unbanked population.
In the future, AI-driven solutions will continue to bridge gaps in financial inclusion, fostering economic growth and reducing inequality.
7. Ethical Considerations and Challenges
Despite its immense potential, the adoption of AI in financial services comes with challenges and ethical concerns.
Bias in AI Models: AI systems can inherit biases present in training data, leading to unfair outcomes.
Data Privacy: The extensive use of customer data for AI-driven insights raises concerns about privacy and security.
Regulatory Compliance: Financial institutions must navigate complex regulatory landscapes to ensure ethical AI deployment.
The future will demand robust frameworks for ethical AI, emphasizing transparency, fairness, and accountability.
8. Future Trends and Innovations
The future of AI in financial services is poised to be shaped by emerging trends and technologies:
Explainable AI (XAI): Financial institutions will adopt XAI to ensure transparency in AI-driven decision-making processes, building trust with customers and regulators.
Quantum Computing: The integration of AI with quantum computing will unlock unparalleled processing power, enabling complex financial modeling and risk assessment.
AI-Powered Ecosystems: Open banking and API-driven ecosystems will leverage AI to offer seamless integration between various financial services.
Decentralized Finance (DeFi): AI will play a key role in automating and optimizing decentralized financial platforms, enhancing accessibility and efficiency.
Conclusion
The future of AI in financial services is bright and transformative. From improving customer experiences to driving financial inclusion and combating fraud, AI is redefining the boundaries of what’s possible. However, to fully realize its potential, financial institutions must address challenges related to ethics, bias, and regulatory compliance. By embracing innovation and ensuring responsible AI deployment, the financial industry can pave the way for a smarter, more inclusive, and efficient future.
1 note
·
View note
Text
Transforming Transactions: RPA's Role in Revolutionizing the Banking Industry
Revolutionize your transactions with RPA. Learn about the transformative role of robotic process automation in the banking industry and how it's revolutionizing financial operations.

Robotic Process Automation (RPA) in Banking helps banks and accounting departments automate repetitive, time-consuming manual processes, empowering the employees to focus on more critical tasks and gain a competitive edge.
#rpa in banking industry#RPA in banking#rpa in banking sector#RPA automation in banking#benefits of rpa in banking#RPA applications in banking
0 notes
Text
Factoring Services Market Growth: Key Trends Driving the Future of the Industry
The global factoring services market size is expected to reach USD 8,188.67 billion by 2030, exhibiting a CAGR of 10.5% from 2024 to 2030, according to a new study by Grand View Research, Inc. The increasing demand for quick and hassle-free financing sources among Micro, Small, and Medium Enterprises (MSMEs) is driving market growth. Factoring services help businesses secure working capital loans and mitigate credit risks. Furthermore, the integration of Artificial Intelligence (AI) and machine learning is anticipated to enhance fraud risk identification, streamline underwriting processes, provide operational savings, and simplify payment applications. Fintech companies and banks have embraced these technologies to develop innovative products and maintain competitiveness in the market.
Since many factoring companies and other lenders have adopted disintegrated data architecture, the use of Application Programming Interface (API), File Transfer Protocol (FTP), or other types of protocols may not be suitable for all kinds of factoring operations. RPA helps businesses automate many processes and become more efficient because it does not necessitate backend coding and helps reduce difficulties associated with code-based data integrations. Furthermore, low-code applications are in high demand because they enable organizations to deploy robust apps that can be easily customized to the organization's needs while saving time, money, and errors in the operation.
Many Business-to-Consumer (B2C) transactions and payment methods, including cheques, are being replaced with electronic alternatives. Digitizing accounts receivable processes helps reduce printing costs and boosts employee profits. Numerous enterprises prefer on-premises deployment of accounts receivable solutions for complete control over infrastructure, assets, and business records. This preference is especially strong in the healthcare and BFSI sectors due to the critical nature of data across these industries. These factors are expected to drive the demand for automation in the factoring services market.
Gather more insights about the market drivers, restrains and growth of the Factoring Services Market
Factoring Services Market Report Highlights
• Based on category, the international segment is expected to register a CAGR of 11.0% from 2024 to 2030. International factoring service providers bridge helps in bridging the gap by extending credit against foreign receivables, offering clients extra capital to foster business growth and handle expenses. Consequently, international factoring emerges as an essential tool for businesses seeking global expansion while upholding financial stability
• Based on type, the non-recourse segment is expected to register a CAGR of 10.8% from 2024 to 2030. Non-recourse factoring enables business expansion by permitting companies to onboard new clients and scale their activities without raising credit risk exposure. This flexibility in client acquisition and the capacity to capitalize on growth prospects are key drivers behind the growing preference for non-recourse factoring
• The non-banking financial institution segment is expected to register a CAGR of 11.9% from 2024 to 2030. Non-banking financial institutions provide adaptable and personalized factoring solutions, unlike conventional banking systems. NBFIs can customize terms, pricing structures, advance rates, and service levels to align with the specific preferences and circumstances of factoring businesses. This flexibility empowers customers to select arrangements that precisely meet their business needs
• North America is expected to register a CAGR of 10.0% from 2024 to 2030. In North America, factoring companies are honing their focus on delivering specialized services tailored to niche markets like transportation, staffing, and advertising. This strategic specialization enables these companies to gain deeper insights into the distinct requirements and obstacles faced by their clients, thereby offering customized financing solutions and additional value-added services
Factoring Services Market Segmentation
Grand View Research has segmented the global factoring services market based on category, type, financial institution, end-use, and region:
Factoring Services Category Outlook (Revenue, USD Billion, 2018 - 2030)
• Domestic
• International
Factoring Services Type Outlook (Revenue, USD Billion, 2018 - 2030)
• Recourse
• Non-recourse
Factoring Services Financial Institution Outlook (Revenue, USD Billion, 2018 - 2030)
• Banks
• Non-banking Financial Institutions
Factoring Services End-use Outlook (Revenue, USD Billion, 2018 - 2030)
• Manufacturing
• Transport & Logistics
• Information Technology
• Healthcare
• Construction
• Staffing
• Others
Factoring Services Regional Outlook (Revenue, USD Billion, 2018 - 2030)
• North America
o U.S.
o Canada
o Mexico
• Europe
o UK
o Germany
o France
o Italy
o Spain
o Poland
o Belgium
o Netherlands
• Asia Pacific
o China
o India
o Japan
o South Korea
o Australia
• Latin America
o Brazil
• Middle East & Africa (MEA)
o UAE
o Saudi Arabia
o South Africa
Order a free sample PDF of the Factoring Services Market Intelligence Study, published by Grand View Research.
#Factoring Services Market#Factoring Services Market Size#Factoring Services Market Share#Factoring Services Market Analysis#Factoring Services Market Growth
0 notes
Text
Top Industries for Software Testers and Emerging Job Roles
Introduction
As technology becomes the backbone of modern businesses, the role of software testing has gained unprecedented importance across industries. Ensuring reliable, efficient, and secure software is essential, whether it's for financial transactions, patient records, online shopping experiences, or connected devices in smart cars. The demand for software testers is both vast and specialized, with each industry requiring unique testing expertise to maintain system integrity and user satisfaction. This exploration highlights the top industries hiring software testers, delving into specific job roles, and the emerging, specialized positions that are shaping the future of software testing.
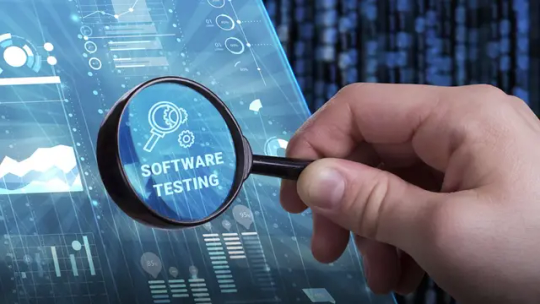
Top Industries for Software Testers
Information Technology (IT) Services and Consulting
IT services are the traditional hub for software testing jobs, where testers work on various software solutions, including web applications, mobile applications, and enterprise solutions.
Job Roles: QA Analyst, Test Automation Engineer, Software Development Engineer in Test (SDET)
Banking, Financial Services, and Insurance (BFSI)
With the rise of digital banking, financial services need robust, secure, and reliable software, requiring extensive testing for compliance and security.
Job Roles: Performance, Security, and Compliance Testers
Healthcare and Pharmaceuticals
Software testing is crucial here to ensure accuracy and reliability, especially for applications managing patient data or supporting diagnostics.
Job Roles: Health IT Tester, Validation Analyst, Clinical Software Tester
E-commerce and Retail
Online shopping platforms depend on seamless user experiences, making functionality, load, and performance testing essential.
Job Roles: User Experience Tester, Functional Tester, Load and Performance Tester
Telecommunications
Telecommunication systems require extensive testing to maintain service reliability and address network performance and security issues.
Job Roles: Network Tester, Mobile Application Tester, Automation Tester
Gaming and Entertainment
With a focus on real-time performance and user engagement, testing is crucial in the gaming industry to avoid crashes and maintain interactivity.
Job Roles: Game Tester, Mobile Game Tester, and Usability Tester
Automotive and Aerospace
As industries shift towards autonomous systems and IoT, software testing becomes critical in ensuring system safety and functionality.
Job Roles: Embedded Software Tester, IoT Tester, Safety-Critical Tester
Education and E-Learning
The growth of online learning platforms requires testing for accessibility, scalability, and content accuracy.
Job Roles: Accessibility Tester, Functional Tester, Content QA Specialist
Emerging Job Roles in Software Testing
Automation Architect
Design and implement automation frameworks that can be scaled across multiple projects or teams.
AI/ML Test Engineer
It focuses on testing AI and machine learning models, ensuring accuracy and bias-free results.
Security Test Engineer
Specializes in penetration testing and vulnerability assessment to safeguard applications from cyber threats.
Cloud Test Engineer
Tests cloud-based applications, focusing on scalability, data security, and performance on cloud infrastructure.
DevOps Test Engineer
Works within DevOps teams, automating CI/CD pipeline testing processes to streamline development and deployment.
Mobile Automation Tester
Specializes in testing mobile applications with automation tools tailored for mobile operating systems.
Big Data Tester
Tests the accuracy and integrity of large datasets, focusing on data processing and validation.
Robotic Process Automation (RPA) Tester
Tests RPA solutions to ensure they work as intended and provide efficiency gains as planned.
Conclusion
In today’s technology-driven world, software testers play a critical role in ensuring that digital systems meet high standards of quality, security, and performance. The diverse industries embracing software testing underline its importance across various domains, from banking and healthcare to gaming and telecommunications. Additionally, the evolution of new job roles like AI/ML Test Engineer, Security Test Engineer, and Cloud Test Engineer showcases how the field is adapting to meet the demands of emerging technologies. As software testing continues to grow, testers equipped with specialized skills will find abundant opportunities, making it an exciting and versatile career path with long-term potential for advancement.
0 notes
Text
Sify's Digital Services: Empowering Businesses for the Future
In today's hyper-connected world, businesses need to be agile, innovative, and deeply integrated with digital technologies to stay competitive. Sify’s Digital Services portfolio offers end-to-end solutions that empower organizations to navigate the complexities of digital transformation seamlessly. With a focus on optimizing business processes, enhancing customer experiences, and leveraging emerging technologies, Sify’s Digital Services help companies not only adapt to change but thrive in the digital era.
Why Sify's Digital Services?
Comprehensive Digital Transformation Sify’s Digital Services cover the entire digital transformation journey, from strategy and consulting to implementation and ongoing support. With expertise across various industries, Sify tailors its services to the unique needs of each business, ensuring that transformation aligns with specific business objectives and maximizes ROI.
Cutting-Edge Cloud Solutions Sify is a trusted partner in cloud transformation, providing a range of services across private, public, and hybrid cloud environments. From cloud migration and management to cloud-native application development, Sify’s cloud services enable organizations to scale efficiently, optimize costs, and improve data accessibility—all while maintaining security and compliance.
Enhanced Customer Experiences through Digital Experience Management In a customer-centric marketplace, delivering personalized and seamless experiences is essential. Sify’s Digital Experience Management solutions leverage data analytics, AI, and machine learning to create tailored customer journeys. By integrating digital touchpoints and enhancing engagement, Sify helps businesses build brand loyalty and drive customer satisfaction.
Cybersecurity and Compliance As businesses go digital, safeguarding data and protecting against cyber threats become critical. Sify’s cybersecurity services include advanced threat detection, incident response, and regulatory compliance management. With multi-layered security solutions, Sify ensures that businesses can operate securely in an increasingly complex cyber landscape.
Data Analytics and Business Intelligence Data is at the heart of every digital strategy. Sify’s Data Analytics and Business Intelligence services turn raw data into actionable insights, helping organizations make data-informed decisions that drive growth. From predictive analytics to real-time dashboards, Sify’s solutions empower businesses to identify trends, optimize processes, and make strategic adjustments faster.
Smart Automation with AI and RPA Sify leverages artificial intelligence (AI) and robotic process automation (RPA) to automate repetitive and time-consuming tasks, boosting efficiency and productivity. With customized automation solutions, Sify enables businesses to focus on high-value activities, improve accuracy, and accelerate time-to-market.
Agile Development and Application Modernization Legacy systems can limit an organization’s agility and scalability. Sify’s application modernization services revitalize outdated software, transforming it into modern, cloud-native applications that can support new business demands. With Agile development methodologies, Sify helps businesses accelerate digital innovation and stay ahead in a rapidly changing market.
Key Industry Applications of Sify’s Digital Services
Sify’s Digital Services are trusted by industries including:
Banking and Financial Services: Secure, compliant digital platforms for efficient transaction processing, personalized customer experiences, and data-driven insights.
Healthcare: Digital solutions that streamline patient engagement, support telemedicine, and enhance data management for improved healthcare delivery.
Retail and E-commerce: AI-driven analytics, customer engagement tools, and e-commerce solutions that enable seamless shopping experiences and drive customer loyalty.
Manufacturing: Smart automation, predictive maintenance, and supply chain optimization for improved operational efficiency and reduced costs.
Education: Digital learning solutions and virtual classrooms that deliver interactive, accessible, and effective education experiences.
The Sify Advantage
With decades of experience in technology services and a deep commitment to innovation, Sify is uniquely positioned to help businesses succeed in the digital age. Sify’s team of experts is dedicated to delivering tailored solutions, continuous support, and measurable results that drive transformation. By prioritizing security, scalability, and flexibility, Sify’s Digital Services help clients address current needs while preparing for future growth.
Partner with Sify for a Digital-First Future
Sify’s Digital Services empower businesses to reimagine what’s possible with technology. By transforming operations, enhancing customer interactions, and adopting smart automation, Sify helps organizations stay relevant, resilient, and ready for the future.
0 notes
Text
Applications of AI in Banking Sector
AI revolution is transforming the banking industry: Different applications that churn efficiency and experience through AI transform the banking sector. From establishing 24/7 customer support through chatbots to AI-driven fraud detection systems, banks are faster in building services on more technology innovations. Personalized advice about financial matters through machine learning algorithms helps people take the right choices. At the same time, AI smoothes processes like loan approvals and risk assessment, becoming more efficient and accurate than them. These integrations put banks on a sure trajectory of success.
USM Business Systems
Services:
Mobile app development
Artificial Intelligence
Machine Learning
Android app development
RPA
Big data
HR Management
Workforce Management
IoT
IOS App Development
Cloud Migration
#AI in banking#AI in banking sector#banking AI applications#financial technology AI#digital banking AI#AI loan processing#AI fraud detection
0 notes
Text
Robotic Process Automation: Boosting Efficiency Across Industries

What if you could delegate the most tedious tasks in your workday to a digital assistant who never tires, gets bored, or makes mistakes?
Robotic Process Automation (RPA) technology is revolutionizing business operations by automating repetitive tasks. With the precision of a Swiss watch and the agility of a ninja, RPA enables businesses to concentrate on innovation while minimizing errors and enhancing efficiency. In 2022, 85% of large enterprises adopted RPA, establishing it as a key solution for increasing productivity. Moreover, a survey by Automation Anywhere found that 78% of respondents plan to significantly boost their budgets for automation.
Understanding Robotic Process Automation (RPA): Benefits and Challenges
Robotic Process Automation (RPA) is revolutionizing business operations by automating repetitive, rule-based tasks. RPA leverages software bots that replicate human actions to execute processes such as data entry, invoice processing, and customer service inquiries. These bots adhere to predefined workflows and are activated by specific events or conditions, allowing for seamless automation of routine tasks without human intervention.
The benefits of RPA for businesses are significant. By automating mundane tasks, RPA enhances operational efficiency and accuracy, thereby reducing the likelihood of errors associated with manual processes. This results in considerable cost savings, as tasks that previously required hours of human effort can now be completed in minutes. Additionally, RPA enables employees to concentrate on more strategic, value-added activities, thereby improving job satisfaction and productivity.
However, adopting RPA presents challenges, including resistance to change, integration issues with existing systems, and the need for ongoing maintenance. To overcome these challenges, organizations should emphasize clear communication about the benefits of RPA, offer comprehensive training, and choose scalable, flexible RPA solutions that integrate seamlessly with their current technology infrastructure.
Having explored what Robotic Process Automation (RPA) is and its benefits, let’s now examine how this technology is being utilized across various industries to drive efficiency and accuracy.
Real-World Applications of Robotic Process Automation (RPA)
RPA in Finance: Enhancing Accuracy and Fraud Prevention
RPA is transforming the finance industry by automating complex, data-intensive tasks. It plays a critical role in reconciling bank statements, automating loan processing, and significantly reducing the risk of human error. Additionally, RPA supports fraud detection and prevention by continuously monitoring transactions for suspicious activities. In customer onboarding and Know Your Customer (KYC) verification, RPA ensures regulatory compliance while expediting the process.
For example, KeyBank, a regional American bank, adopted RPA early to boost efficiency and accuracy in its operations. RPA in banking automates processes like invoice management, purchase orders, and accounts receivable, allowing KeyBank to streamline payments and deliver smoother, error-free transactions at every stage.
RPA in Healthcare: Improving Patient Care and Operational Efficiency
RPA in healthcare is making significant strides by improving both patient care and administrative efficiency. Automating patient data entry and management ensures accurate records with minimal manual intervention. RPA also optimizes appointment scheduling and reminders, reducing no-shows and enhancing clinic management. Additionally, it streamlines medical records management, ensuring that patient information is always up-to-date and easily accessible.
A UK-based startup, T-Systems, is utilizing RPA to develop healthcare solutions, including a system for tracking the time and location of organs during transplant operations. Similarly, India-based Feat Systems offers an RPA-enabled web app that automates appointment creation, reminders, and cancellations, making the process more accessible and efficient for users.
RPA in Customer Service: Revolutionizing Response Times and Experience
RPA is transforming customer service by automating repetitive tasks, improving response times, and ensuring that customer inquiries are handled efficiently. Automated customer service processes such as ticket routing, order processing, and fulfillment are accelerated, resulting in faster delivery times and an enhanced customer experience. RPA-powered chatbots offer 24/7 support, addressing routine inquiries and escalating more complex issues to human agents.
For instance, Vaasan Sähkö Group leverages automation to enhance customer communication, leading to improved satisfaction. Sisua Digital implemented the Kyösti robot for Y-Foundation to boost customer service operations and deliver swift, reliable support.
RPA in Human Resources: Streamlining Employee Management
In human resources, RPA is transforming traditionally manual and time-consuming tasks such as employee onboarding and offboarding. Automation ensures consistency and compliance with company policies while assisting with time and attendance management by providing accurate tracking and reporting.
Romanian startup Aggranda specializes in creating intelligent automation solutions for HR companies. It automates routine tasks like data entry, payroll management, and time tracking, significantly reducing manual effort and streamlining HR processes.
What’s Next for Robotic Process Automation?
Robotic Process Automation (RPA) is evolving rapidly, especially when integrated with artificial intelligence (AI). By automating repetitive tasks, RPA allows employees to shift their focus to more strategic, creative, and value-driven activities, fostering a more engaged and productive workforce. Industry projections suggest that by 2030, AI-powered automation technologies could replace up to 30% of work hours in the United States.
The future of RPA looks bright, with advancements such as cognitive automation, machine learning integration, and cloud-based RPA solutions on the horizon. These innovations will further transform business operations, offering greater scalability, flexibility, and efficiency, setting the stage for a new era of automation.
#robotic process automation#rpa#automation#automation technology#artificial intelligence#market analysis#triton market research
0 notes
Text
The Evolution of Investment Banking – How Digital Transformation is Redefining Deal-Making
Investment banking professionals serve institutional investors and high-net-worth individuals in conducting higher-value transactions. For instance, an investment bank (IB) might be a negotiator, market-maker, or pitch deck facilitator for business merger deals.
At the same time, simplifying the intricate nuances of risk models and detailed documentation with the help of the latest financial tech upgrades for IBs is popular. The new programs are already helping the investment banking teams refine their workflows through automation, AI, and blockchain technologies, which are excelling at deal sourcing, offer optimization, and initial public offering assistance.
Given the need to promote greater efficiency and universal data democratization for IB deal-making, staying knowledgeable about those technologies is vital. This post will explain the evolution of investment banking.
What is Deal-Making in Investment Banking?
Investment banks’ deal-making is essentially the process of completing mergers and acquisitions, raising capital, or financial transactions between firms or investors. Therefore, modern investment banking services provide deal-making support to unify practices like structuring deals, advising clients, and executing complex financial deals to achieve strategic business objectives.
The Evolution of Investment Banking – Digital Transformation Redefining Deal-Making
1| Automation of the Manual Activities
Investment banking heavily relied on manual work. For example, data entry, industry research, and due diligence, similar to several other aspects of financial analysis, would require human efforts. These highly redundant tasks now take less time and more accurately depict trends because of tools like RPA or robotic process automation. This advantage means investment bankers can engage in other valuable activities related to client relationships and strategic planning.
2| Artificial Intelligence and Machine Learning
Fintech tools have leveraged AI and predictive data analytics to improve risk management and deal-sourcing practices. For example, machine learning algorithms by a data insights company can check datasets describing multibillion-dollar business deals to identify investment opportunities. They can predict market trends and flag real-time risks. Furthermore, AI enhances the due diligence process crucial to corporate mergers and acquisitions (M&A) by not only automating financial document review but also boosting valuation modeling’s effectiveness.
3| Big Data and Advanced Analytics
Investment banks are now employing big data for the proper understanding of the market situation, the behavior of consumers, and the competitive landscape. Advanced analytics help bankers better inform decisions by providing insights into the potential of the deal. Such innovations also help to make targeted client outreach, providing insight into the financial history, investment preferences, and risks tolerated by such clients.
4| Blockchain and Distributed Ledger Technology (DLT)
Blockchain technology promotes much higher visibility and efficiencies in settlement procedures and syndications. For example, smart contracts facilitated through a blockchain platform automatically process escrow, compliance, and disbursement as part of the settlement. It essentially eliminates many middlemen involved in the transaction. Therefore, stakeholders acquire easier, inexpensive ways to interact.
5| Cloud Computing
The cloud delivers real-time shared ecosystems, letting investment bankers work together much better to make deal negotiations succeed worldwide. It also provides on-demand computing resources. As a result, large-scale models for simulations and risk analytics can be run much faster and at significantly lower cost than an investment bank’s on-premises infrastructure. Undoubtedly, cloud applications are vital among digital platforms for online capital-raising activities.
Conclusion
Digital deal-making ensured the stakeholder-empowering evolution of the investment banking and financial services industry. It may make deal categorization, sourcing, negotiation, marketing, pitch deck creation, and feasibility analyses much more accessible, efficient, and transparent.
Actually, automation via AI, blockchain, and cloud computing promotes new norms for the betterment of the client experience. All of this helps facilitate greater accessibility to financial markets.
However, a greater number of skilled professionals who have solved cybersecurity problems are necessary for embracing modern financial technologies. Teaming up with experienced investment banking tech professionals is advisable to benefit from the above-mentioned trends in this industry.
0 notes