#HypercomputeCluster
Explore tagged Tumblr posts
Text
A3 Ultra VMs With NVIDIA H200 GPUs Pre-launch This Month
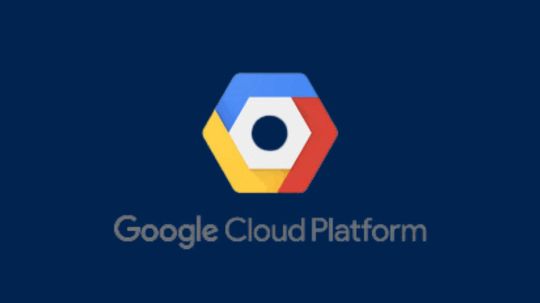
Strong infrastructure advancements for your future that prioritizes AI
To increase customer performance, usability, and cost-effectiveness, Google Cloud implemented improvements throughout the AI Hypercomputer stack this year. Google Cloud at the App Dev & Infrastructure Summit:
Trillium, Google’s sixth-generation TPU, is currently available for preview.
Next month, A3 Ultra VMs with NVIDIA H200 Tensor Core GPUs will be available for preview.
Google’s new, highly scalable clustering system, Hypercompute Cluster, will be accessible beginning with A3 Ultra VMs.
Based on Axion, Google’s proprietary Arm processors, C4A virtual machines (VMs) are now widely accessible
AI workload-focused additions to Titanium, Google Cloud’s host offload capability, and Jupiter, its data center network.
Google Cloud’s AI/ML-focused block storage service, Hyperdisk ML, is widely accessible.
Trillium A new era of TPU performance
Trillium A new era of TPU performance is being ushered in by TPUs, which power Google’s most sophisticated models like Gemini, well-known Google services like Maps, Photos, and Search, as well as scientific innovations like AlphaFold 2, which was just awarded a Nobel Prize! We are happy to inform that Google Cloud users can now preview Trillium, our sixth-generation TPU.
Taking advantage of NVIDIA Accelerated Computing to broaden perspectives
By fusing the best of Google Cloud’s data center, infrastructure, and software skills with the NVIDIA AI platform which is exemplified by A3 and A3 Mega VMs powered by NVIDIA H100 Tensor Core GPUs it also keeps investing in its partnership and capabilities with NVIDIA.
Google Cloud announced that the new A3 Ultra VMs featuring NVIDIA H200 Tensor Core GPUs will be available on Google Cloud starting next month.
Compared to earlier versions, A3 Ultra VMs offer a notable performance improvement. Their foundation is NVIDIA ConnectX-7 network interface cards (NICs) and servers equipped with new Titanium ML network adapter, which is tailored to provide a safe, high-performance cloud experience for AI workloads. A3 Ultra VMs provide non-blocking 3.2 Tbps of GPU-to-GPU traffic using RDMA over Converged Ethernet (RoCE) when paired with our datacenter-wide 4-way rail-aligned network.
In contrast to A3 Mega, A3 Ultra provides:
With the support of Google’s Jupiter data center network and Google Cloud’s Titanium ML network adapter, double the GPU-to-GPU networking bandwidth
With almost twice the memory capacity and 1.4 times the memory bandwidth, LLM inferencing performance can increase by up to 2 times.
Capacity to expand to tens of thousands of GPUs in a dense cluster with performance optimization for heavy workloads in HPC and AI.
Google Kubernetes Engine (GKE), which offers an open, portable, extensible, and highly scalable platform for large-scale training and AI workloads, will also offer A3 Ultra VMs.
Hypercompute Cluster: Simplify and expand clusters of AI accelerators
It’s not just about individual accelerators or virtual machines, though; when dealing with AI and HPC workloads, you have to deploy, maintain, and optimize a huge number of AI accelerators along with the networking and storage that go along with them. This may be difficult and time-consuming. For this reason, Google Cloud is introducing Hypercompute Cluster, which simplifies the provisioning of workloads and infrastructure as well as the continuous operations of AI supercomputers with tens of thousands of accelerators.
Fundamentally, Hypercompute Cluster integrates the most advanced AI infrastructure technologies from Google Cloud, enabling you to install and operate several accelerators as a single, seamless unit. You can run your most demanding AI and HPC workloads with confidence thanks to Hypercompute Cluster’s exceptional performance and resilience, which includes features like targeted workload placement, dense resource co-location with ultra-low latency networking, and sophisticated maintenance controls to reduce workload disruptions.
For dependable and repeatable deployments, you can use pre-configured and validated templates to build up a Hypercompute Cluster with just one API call. This include containerized software with orchestration (e.g., GKE, Slurm), framework and reference implementations (e.g., JAX, PyTorch, MaxText), and well-known open models like Gemma2 and Llama3. As part of the AI Hypercomputer architecture, each pre-configured template is available and has been verified for effectiveness and performance, allowing you to concentrate on business innovation.
A3 Ultra VMs will be the first Hypercompute Cluster to be made available next month.
An early look at the NVIDIA GB200 NVL72
Google Cloud is also awaiting the developments made possible by NVIDIA GB200 NVL72 GPUs, and we’ll be providing more information about this fascinating improvement soon. Here is a preview of the racks Google constructing in the meantime to deliver the NVIDIA Blackwell platform’s performance advantages to Google Cloud’s cutting-edge, environmentally friendly data centers in the early months of next year.
Redefining CPU efficiency and performance with Google Axion Processors
CPUs are a cost-effective solution for a variety of general-purpose workloads, and they are frequently utilized in combination with AI workloads to produce complicated applications, even if TPUs and GPUs are superior at specialized jobs. Google Axion Processors, its first specially made Arm-based CPUs for the data center, at Google Cloud Next ’24. Customers using Google Cloud may now benefit from C4A virtual machines, the first Axion-based VM series, which offer up to 10% better price-performance compared to the newest Arm-based instances offered by other top cloud providers.
Additionally, compared to comparable current-generation x86-based instances, C4A offers up to 60% more energy efficiency and up to 65% better price performance for general-purpose workloads such as media processing, AI inferencing applications, web and app servers, containerized microservices, open-source databases, in-memory caches, and data analytics engines.
Titanium and Jupiter Network: Making AI possible at the speed of light
Titanium, the offload technology system that supports Google’s infrastructure, has been improved to accommodate workloads related to artificial intelligence. Titanium provides greater compute and memory resources for your applications by lowering the host’s processing overhead through a combination of on-host and off-host offloads. Furthermore, although Titanium’s fundamental features can be applied to AI infrastructure, the accelerator-to-accelerator performance needs of AI workloads are distinct.
Google has released a new Titanium ML network adapter to address these demands, which incorporates and expands upon NVIDIA ConnectX-7 NICs to provide further support for virtualization, traffic encryption, and VPCs. The system offers best-in-class security and infrastructure management along with non-blocking 3.2 Tbps of GPU-to-GPU traffic across RoCE when combined with its data center’s 4-way rail-aligned network.
Google’s Jupiter optical circuit switching network fabric and its updated data center network significantly expand Titanium’s capabilities. With native 400 Gb/s link rates and a total bisection bandwidth of 13.1 Pb/s (a practical bandwidth metric that reflects how one half of the network can connect to the other), Jupiter could handle a video conversation for every person on Earth at the same time. In order to meet the increasing demands of AI computation, this enormous scale is essential.
Hyperdisk ML is widely accessible
For computing resources to continue to be effectively utilized, system-level performance maximized, and economical, high-performance storage is essential. Google launched its AI-powered block storage solution, Hyperdisk ML, in April 2024. Now widely accessible, it adds dedicated storage for AI and HPC workloads to the networking and computing advancements.
Hyperdisk ML efficiently speeds up data load times. It drives up to 11.9x faster model load time for inference workloads and up to 4.3x quicker training time for training workloads.
With 1.2 TB/s of aggregate throughput per volume, you may attach 2500 instances to the same volume. This is more than 100 times more than what big block storage competitors are giving.
Reduced accelerator idle time and increased cost efficiency are the results of shorter data load times.
Multi-zone volumes are now automatically created for your data by GKE. In addition to quicker model loading with Hyperdisk ML, this enables you to run across zones for more computing flexibility (such as lowering Spot preemption).
Developing AI’s future
Google Cloud enables companies and researchers to push the limits of AI innovation with these developments in AI infrastructure. It anticipates that this strong foundation will give rise to revolutionary new AI applications.
Read more on Govindhtech.com
#A3UltraVMs#NVIDIAH200#AI#Trillium#HypercomputeCluster#GoogleAxionProcessors#Titanium#News#Technews#Technology#Technologynews#Technologytrends#Govindhtech
2 notes
·
View notes