#GBM Industries
Explore tagged Tumblr posts
Text
Everything You Need to Know About Liquid Painting Plant!!
A Liquid Painting Plant is an essential system in industrial applications where high-quality surface finishing is required. These plants are specifically designed to apply liquid paint onto various products, ensuring a smooth, durable, and aesthetically pleasing finish.
Read more: Everything You Need to Know About Liquid Painting Plant
0 notes
Text
India's Leading Manufacturer of Belt Conveyor Ovens – GBM Industries
Discover high-quality, efficient belt conveyor ovens from GBM Industries, India’s top manufacturer, supplier, and exporter. As an ISO-certified company, GBM Industries ensures unmatched quality and reliability in every product, crafted to meet the diverse needs of various industries. With state-of-the-art manufacturing techniques and a commitment to customer satisfaction, our belt conveyor ovens are designed for optimal performance and durability.
To explore more, visit our comprehensive PPT presentation and see The Best Manufacturer of Belt Conveyor Ovens in India!
0 notes
Text
Industrial Ovens: Unmatched Quality from GBM Industries
GBM Industries is a trusted manufacturer of industrial ovens designed for diverse applications. With cutting-edge technology, our ovens ensure uniform heat distribution, energy efficiency, and long-lasting durability. Perfect for curing, drying, or baking with precision and consistency!
Discover unmatched performance and reliability. 👇
Read more! ✅Industrial Ovens: Unmatched Quality from GBM Industries
0 notes
Text
Incorporating Memory and Stochastic Volatility into Geometric Brownian Motion Model
Incorporating Memory and Stochastic Volatility into Geometric Brownian Motion Model Geometric Brownian Motion (GBM) is a widely used mathematical model for simulating the random behavior of asset prices in financial markets. It assumes that the price of an asset follows a continuous-time stochastic process, where the logarithmic returns are normally distributed. GBM is foundational in option pricing models like Black-Scholes-Merton. Despite its widespread use, the GBM model has limitations. Reference [1] addresses these limitations by incorporating long memory (long-range dependence) and stochastic volatility into the GBM framework. Three models were studied: Model 1, the classic GBM, which excludes both memory and stochastic volatility, Model 2, the fractional geometric Brownian motion (FGBM), which includes memory but ignores stochastic volatility, and Model 3 incorporates both memory and stochastic volatility. The study empirically analyzes these models by forecasting the Euro exchange rate against three currencies: Saudi Riyal (SAR), US Dollar (USD), and Australian Dollar (AUD). The authors pointed out, Exchange rates play a crucial role in the financial trade of any country, especially in international trade. Therefore, understanding the future direction of exchange rates is a priority for stakeholders. To achieve this goal, many researchers in the literature have proposed several models. In this study, the researchers utilized three GBM-based models to predict the exchange rates of three currency pairs: EUR/USD, EUR/SAR, and EUR/AUD. The first model followed the traditional GBM approach without considering memory or assuming stochastic volatility. The second model, known as GFBM, incorporated memory but ignored the assumption of stochastic volatility. Finally, the third model, also a type of GFBM, took both memory and stochastic volatility into account. After performing predictions with all three models, it was observed that the third model demonstrated superior performance, as evidenced by its lowest Mean Squared Error (MSE). This result indicates that incorporating memory and assuming stochastic volatility in GBM positively impacts its effectiveness as a tool for predicting exchange rate prices. Therefore, given the high accuracy shown by model 3, it can confidently be used for forecasting future exchange rates. In short, the findings suggest that incorporating long-range memory and stochastic volatility significantly enhances the model's predictive power. Let us know what you think in the comments below or in the discussion forum. References [1] Mahan Farzina, Mehdi Sadeghi Moghaddamb, Amir Mohammad Shahbalaei Kashan, The Effects of Adding Memory and stochastic volatility in the GBM Method for Predicting the Euro Exchange Rate, Applied Innovations in Industrial Management 4-1 (2024) 30–41 Post Source Here: Incorporating Memory and Stochastic Volatility into Geometric Brownian Motion Model via Harbourfront Technologies - Feed https://ift.tt/0u4gUzo December 02, 2024 at 07:20PM
0 notes
Text
Drum Heating Ovens by GBM Industries: Precision & Efficiency Redefined 🛢️⚙️
Explore how GBM Industries delivers top-notch drum heating solutions with their advanced ovens. Ensuring even heat distribution, durability, and efficiency, these ovens are built for industrial excellence. 💡✨
If you want to know more visit our latest article !! Drum Heating Ovens by GBM Industries: Precision & Efficiency Redefined

0 notes
Text
The Fully Automatic Liquid Painting Plant by GBM Industries 🎨🏭
Experience unmatched precision and efficiency with GBM Industries' Fully Automatic Liquid Painting Plant. Designed for streamlined production, this advanced painting system ensures consistent, high-quality finishes on various materials, from plastic to metal. Whether you're in automotive, electronics, or general manufacturing, this plant delivers superior coating results every time.
Key Benefits:
🤖 Full Automation: Ensures fast, precise, and consistent paint application.
🌬️ Dust-Free Enclosures: Maintains a clean environment for flawless finishes.
🔄 Energy Efficiency: Reduces operational costs while maximizing productivity.
⚙️ Customizable Settings: Tailored options to meet specific industry requirements.
Boost your production efficiency and product quality today!
👉 Visit Now GBM Industries 🌟
0 notes
Text
Introduction to Machine Learning
Introduction to Machine Learning
Machine learning, a subset of artificial intelligence (AI), focuses on developing algorithms that enable computers to learn from and make decisions based on data. This technology has rapidly evolved, transforming industries by enabling systems to automatically improve their performance through experience. In this blog, we'll delve into the fundamentals of machine learning, its types, key algorithms, applications, challenges, and future trends.
Fundamentals of Machine Learning
Machine learning is about creating models that can predict outcomes or identify patterns without being explicitly programmed to perform the task. These models learn from data by identifying correlations and patterns. The process typically involves the following steps:
Data Collection: Gathering relevant data from various sources.
Data Preparation: Cleaning and organizing the data to make it suitable for analysis.
Model Selection: Choosing the appropriate machine learning algorithm for the task.
Training: Feeding the prepared data into the algorithm to build the model.
Evaluation: Assessing the model's performance using various metrics.
Optimization: Tuning the model to improve its accuracy and efficiency.
Deployment: Integrating the model into a production environment where it can make predictions on new data.
Types of Machine Learning
Machine learning can be categorized into three main types:
Supervised Learning: Involves training a model on a labeled dataset, which means that each training example is paired with an output label. Common algorithms include linear regression, logistic regression, support vector machines, and neural networks.
Unsupervised Learning: Deals with unlabeled data and the goal is to infer the natural structure present within the set of data points. Common algorithms include k-means clustering, hierarchical clustering, and principal component analysis.
Reinforcement Learning: Involves training models to make a sequence of decisions by rewarding them for good decisions and penalizing them for bad ones. It’s commonly used in robotics, gaming, and autonomous systems. Algorithms include Q-learning and deep Q-networks (DQNs).
Key Algorithms in Machine Learning
Machine learning encompasses a variety of algorithms suited for different types of tasks. Some of the key algorithms include:
Linear Regression: Used for predicting a continuous value. It establishes a relationship between the input and output variables by fitting a linear equation to observed data.
Logistic Regression: Used for binary classification problems. It models the probability of a binary outcome based on one or more predictor variables.
Decision Trees: A tree-like model used for classification and regression. It splits the data into subsets based on the value of input features.
Support Vector Machines (SVMs): Used for classification tasks. It finds the hyperplane that best separates the data into different classes.
k-Nearest Neighbors (k-NN): A simple, instance-based learning algorithm used for classification and regression. It assigns the class of a data point based on the majority class of its k nearest neighbors.
Naive Bayes: A probabilistic classifier based on Bayes’ theorem. It assumes independence between predictors, making it fast and effective for large datasets.
Neural Networks: Modeled after the human brain, these algorithms are used for various tasks, including image and speech recognition. They consist of layers of interconnected nodes (neurons) that process input data.
Random Forests: An ensemble method that combines multiple decision trees to improve classification accuracy.
Gradient Boosting Machines (GBMs): Another ensemble technique that builds models sequentially, with each new model correcting errors made by the previous ones.
Applications of Machine Learning
Machine learning has a wide range of applications across various industries:
Healthcare: Predicting disease outbreaks, diagnosing illnesses, and personalizing treatment plans.
Finance: Fraud detection, algorithmic trading, credit scoring, and risk management.
Retail: Personalized recommendations, inventory management, and sales forecasting.
Manufacturing: Predictive maintenance, quality control, and supply chain optimization.
Transportation: Autonomous vehicles, route optimization, and traffic prediction.
Marketing: Customer segmentation, sentiment analysis, and targeted advertising.
Entertainment: Content recommendation, game AI, and music composition.
Agriculture: Crop monitoring, yield prediction, and automated irrigation systems.
Challenges in Machine Learning
Despite its numerous advantages, machine learning faces several challenges:
Data Quality and Quantity: High-quality, relevant data is essential for building effective models. Inadequate or poor-quality data can lead to inaccurate models.
Interpretability: Many machine learning models, especially complex ones like deep neural networks, are often seen as "black boxes," making it difficult to understand how they make decisions.
Scalability: As data volumes grow, scaling machine learning models to handle large datasets efficiently can be challenging.
Bias and Fairness: Ensuring that models are fair and unbiased is critical, as biased models can perpetuate existing inequalities.
Security and Privacy: Protecting sensitive data and ensuring models are secure from adversarial attacks is crucial.
Integration and Deployment: Integrating machine learning models into existing systems and deploying them at scale can be complex and resource-intensive.
Future Trends in Machine Learning
The future of machine learning looks promising, with several emerging trends set to shape the field:
Automated Machine Learning (AutoML): Tools and techniques that automate the end-to-end process of applying machine learning, making it accessible to non-experts.
Explainable AI (XAI): Developing methods to make machine learning models more interpretable and transparent.
Federated Learning: A distributed approach to training models across multiple devices while keeping data localized, enhancing privacy.
TinyML: Implementing machine learning on small, resource-constrained devices, enabling edge computing.
Quantum Machine Learning: Leveraging quantum computing to solve complex machine learning problems more efficiently.
Synthetic Data: Using AI to generate synthetic datasets to train models, addressing issues of data scarcity and privacy.
Conclusion
Machine learning is revolutionizing how we approach problem-solving and decision-making across various domains. By enabling systems to learn from data, machine learning models are driving innovation and efficiency. As the field continues to evolve, the integration of advanced techniques and the focus on ethical considerations will further enhance the impact of machine learning on our lives.
If you are looking to harness the power of machine learning for your business, consider partnering with UpCore Technologies. With our extensive expertise in AI and machine learning solutions, we can help you streamline processes, enhance decision-making, and unlock new opportunities. Contact us today to learn more about how we can assist you in achieving your business goals.
0 notes
Text
Understanding the Dynamics of the Glioblastoma Market: Drivers, Barriers, and Future Outlook
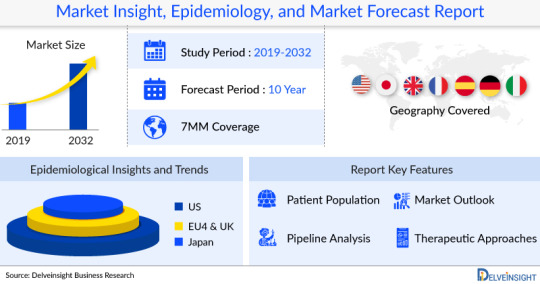
Glioblastoma, also known as glioblastoma multiforme (GBM), is the most aggressive and malignant type of primary brain tumor in adults. It belongs to a group of tumors called gliomas, which arise from glial cells in the brain.
Glioblastoma Market Drivers
The glioblastoma market, which encompasses diagnostics, treatments, and supportive care for patients with glioblastoma, is influenced by several key drivers. These drivers shape the development of new therapies, diagnostic tools, and supportive care approaches aimed at improving patient outcomes. Some of the significant drivers in the glioblastoma market include:
High Unmet Medical Need: Glioblastoma represents a significant unmet medical need due to its aggressive nature, limited treatment options, and poor prognosis. The urgent need for effective therapies drives research and development efforts to discover novel treatment modalities and improve existing treatment strategies.
Advances in Molecular Biology: Increasing understanding of the molecular pathways and genetic alterations involved in glioblastoma pathogenesis has led to the identification of potential therapeutic targets. Advances in molecular biology, genomics, and tumor profiling techniques enable the development of targeted therapies tailored to the specific molecular characteristics of individual tumors.
Innovations in Immunotherapy: Immunotherapy has emerged as a promising approach for the treatment of glioblastoma. Research into immune checkpoint inhibitors, chimeric antigen receptor (CAR) T-cell therapy, cancer vaccines, and adoptive cell therapy aims to harness the immune system's ability to recognize and eliminate tumor cells. Immunotherapy holds potential for improving treatment outcomes and extending survival in glioblastoma patients.
Technological Advancements in Imaging: Technological advancements in neuroimaging, such as advanced magnetic resonance imaging (MRI) techniques, positron emission tomography (PET), and molecular imaging, enable more accurate diagnosis, tumor characterization, and treatment monitoring in glioblastoma patients. Improved imaging modalities facilitate early detection of tumor recurrence, guiding treatment decisions and improving patient management.
Precision Medicine Approaches: The shift towards precision medicine in glioblastoma treatment involves tailoring therapies to the individual molecular profile of each patient's tumor. Biomarker-driven treatment strategies, including molecular profiling, genetic testing, and tumor sequencing, help identify predictive biomarkers and select the most effective treatment options for glioblastoma patients.
Clinical Trial Innovation: Clinical trials play a critical role in evaluating the safety and efficacy of investigational therapies in glioblastoma patients. Innovative trial designs, including adaptive trials, basket trials, and umbrella trials, allow for more efficient evaluation of multiple treatment strategies and combinations. Collaborative efforts between academia, industry, and regulatory agencies drive the development of novel therapies through clinical research.
Regulatory Incentives: Regulatory agencies provide incentives and expedited pathways for the development and approval of therapies targeting rare and life-threatening diseases like glioblastoma. Orphan drug designation, fast-track designation, breakthrough therapy designation, and accelerated approval pathways facilitate the development and regulatory review of promising therapies, expediting their availability to patients.
Increasing Healthcare Investment: Rising healthcare expenditure and investment in neuro-oncology research contribute to the advancement of glioblastoma treatment options. Pharmaceutical companies, biotechnology firms, academic institutions, and government agencies allocate resources towards glioblastoma research, drug development, and clinical trials, driving innovation and progress in the field.
Glioblastoma Market Barriers
Despite ongoing efforts to develop effective treatments for glioblastoma, several barriers hinder progress in the glioblastoma market. These barriers pose challenges to research, drug development, patient care, and overall improvements in treatment outcomes. Some of the significant barriers in the glioblastoma market include:
Limited Treatment Options: Glioblastoma is notoriously difficult to treat, with few effective therapeutic options available. The aggressive nature of the disease, combined with its resistance to conventional treatments, poses significant challenges for clinicians and researchers. The lack of diverse treatment modalities contributes to the limited survival benefit and poor prognosis associated with glioblastoma.
Tumor Heterogeneity: Glioblastoma tumors are characterized by extensive molecular and cellular heterogeneity, which complicates treatment decision-making and response assessment. Intra-tumoral heterogeneity, inter-tumoral heterogeneity, and tumor evolution over time contribute to treatment resistance, disease recurrence, and therapeutic failure. Developing therapies that target the diverse subpopulations of tumor cells within glioblastoma tumors remains a significant challenge.
Blood-Brain Barrier (BBB): The blood-brain barrier presents a formidable obstacle to drug delivery in glioblastoma treatment. The BBB restricts the passage of many therapeutic agents, including chemotherapy drugs and targeted therapies, into the brain parenchyma, limiting their effectiveness. Strategies to bypass or overcome the BBB, such as nanoparticle-based drug delivery systems and focused ultrasound techniques, face technical and safety challenges in clinical translation.
Tumor Recurrence and Resistance: Glioblastoma tumors frequently recur after initial treatment, often with increased aggressiveness and resistance to therapy. Tumor recurrence is associated with the persistence of cancer stem cells, tumor microenvironmental changes, and molecular alterations that promote treatment resistance. Overcoming mechanisms of tumor recurrence and developing therapies that target resistant tumor cells are critical unmet needs in glioblastoma treatment.
Lack of Biomarkers: The absence of reliable biomarkers for glioblastoma diagnosis, prognosis, and treatment response prediction hampers personalized treatment approaches. Biomarker discovery efforts face challenges such as tumor heterogeneity, dynamic changes in biomarker expression over time, and limited validation in clinical settings. Biomarker-driven treatment strategies require robust biomarkers that accurately predict treatment outcomes and guide therapeutic decisions.
Clinical Trial Design Challenges: Clinical trials in glioblastoma face challenges related to patient recruitment, trial design, and endpoint selection. Glioblastoma patients often have complex medical histories, comorbidities, and limited eligibility criteria for clinical trials, leading to slow accrual and challenges in achieving statistical power. Additionally, determining clinically meaningful endpoints, such as overall survival and progression-free survival, in the context of glioblastoma treatment poses methodological challenges.
Regulatory Hurdles: Regulatory approval processes for new glioblastoma therapies are stringent and time-consuming, requiring substantial preclinical and clinical evidence of safety and efficacy. Challenges in demonstrating meaningful clinical benefit, establishing appropriate surrogate endpoints, and navigating regulatory pathways delay the development and approval of novel treatments. Regulatory agencies seek to balance patient safety with the need for expedited access to innovative therapies for life-threatening diseases like glioblastoma.
Limited Funding and Investment: Glioblastoma research and drug development rely heavily on funding from government agencies, philanthropic organizations, and private investors. Limited funding availability, competing research priorities, and perceived risks associated with glioblastoma drug development may deter investment in the field. Glioblastoma research often requires long-term commitments and substantial resources to overcome the scientific, clinical, and regulatory challenges inherent to the disease.
Future Glioblastoma Market Analysis
Analyzing the future of the glioblastoma market involves considering emerging trends, technological advancements, research breakthroughs, regulatory developments, and evolving patient needs. Here's a prospective analysis of the future glioblastoma market:
Advancements in Precision Medicine: The future of glioblastoma treatment lies in precision medicine approaches that tailor therapies to the molecular profile of individual tumors. Advances in molecular profiling, genomics, and biomarker discovery will enable the identification of specific therapeutic targets and predictive markers for patient stratification. Precision medicine strategies will improve treatment outcomes and reduce toxicity by matching patients with the most effective and well-tolerated therapies.
Immunotherapy Revolution: Immunotherapy holds tremendous promise for transforming the treatment landscape of glioblastoma. Ongoing research into immune checkpoint inhibitors, chimeric antigen receptor (CAR) T-cell therapy, cancer vaccines, and adoptive cell therapy aims to harness the immune system's ability to recognize and eliminate tumor cells. Combination immunotherapy approaches and innovative treatment modalities, such as oncolytic viruses and immune cell engineering, will enhance anti-tumor immune responses and overcome immune evasion mechanisms.
Targeted Therapies and Novel Drug Development: The future glioblastoma market will witness the development of targeted therapies that selectively inhibit critical signaling pathways and genetic alterations driving tumor growth and progression. Small molecule inhibitors, antibody-drug conjugates, bi-specific antibodies, and gene therapy approaches will target specific oncogenic drivers and vulnerabilities in glioblastoma tumors. Combination therapies that exploit synergistic interactions between targeted agents, immunotherapies, and standard treatments will improve therapeutic efficacy and overcome treatment resistance.
Innovative Drug Delivery Systems: Overcoming the blood-brain barrier (BBB) remains a major challenge in glioblastoma treatment. Future advancements in drug delivery systems, such as nanoparticle-based carriers, liposomes, and focused ultrasound techniques, will enhance drug penetration into the brain parenchyma and improve therapeutic efficacy. Targeted drug delivery platforms that selectively deliver therapeutic agents to tumor cells while sparing healthy brain tissue will minimize off-target effects and maximize treatment benefit.
Biomarker-Driven Clinical Trials: Biomarker-driven clinical trials will become increasingly important for evaluating targeted therapies and immunotherapies in glioblastoma patients. Molecular profiling, genetic testing, and tumor sequencing will guide patient selection, treatment allocation, and response assessment in clinical trial settings. Adaptive trial designs, basket trials, and umbrella trials will facilitate the rapid evaluation of multiple treatment strategies and combinations, accelerating the development of novel therapies.
Digital Health Integration: Digital health technologies will play a significant role in improving glioblastoma patient care and treatment outcomes. Telemedicine platforms, mobile apps, wearable devices, and remote monitoring tools will enable remote patient management, real-time symptom tracking, and virtual consultations with healthcare providers. Digital health solutions will enhance patient engagement, adherence to treatment regimens, and access to supportive care services, thereby improving quality of life and survival outcomes.
Regulatory Incentives and Expedited Pathways: Regulatory agencies will continue to provide incentives and expedited pathways for the development and approval of innovative therapies for glioblastoma. Orphan drug designation, fast-track designation, breakthrough therapy designation, and accelerated approval pathways will facilitate the regulatory review and expedited access to promising therapies for glioblastoma patients. Collaboration between regulators, industry stakeholders, and patient advocacy groups will streamline the drug development process and accelerate the availability of new treatments.
Patient-Centric Care Models: The future glioblastoma market will prioritize patient-centered care models that address the holistic needs and preferences of individuals living with glioblastoma. Multidisciplinary care teams, shared decision-making, and personalized treatment plans will optimize patient outcomes and improve quality of life. Psychosocial support, palliative care services, and survivorship programs will address the emotional, social, and supportive care needs of glioblastoma patients and their caregivers.
Evolving Glioblastoma Treatment Outlook
The evolving treatment outlook for glioblastoma involves a multifaceted approach aimed at improving patient outcomes through advances in precision medicine, immunotherapy, targeted therapies, innovative drug delivery systems, and supportive care. Here's an overview of the evolving glioblastoma treatment outlook:
Precision Medicine: The future of glioblastoma treatment lies in precision medicine approaches that tailor therapies to the individual molecular characteristics of each patient's tumor. Molecular profiling, genetic testing, and biomarker analysis guide treatment decisions, allowing for the selection of targeted therapies that address specific genetic alterations and signaling pathways driving tumor growth. Precision medicine strategies improve treatment efficacy, minimize toxicity, and overcome resistance mechanisms, ultimately leading to better outcomes for glioblastoma patients.
Immunotherapy Revolution: Immunotherapy represents a transformative approach to glioblastoma treatment by harnessing the immune system's natural ability to recognize and eliminate cancer cells. Immune checkpoint inhibitors, chimeric antigen receptor (CAR) T-cell therapy, cancer vaccines, and adoptive cell therapy are being investigated in clinical trials for glioblastoma. These immunotherapeutic approaches enhance anti-tumor immune responses, overcome immunosuppressive mechanisms within the tumor microenvironment, and improve long-term survival outcomes in a subset of patients.
Targeted Therapies: Advances in molecular biology and drug development have led to the identification of specific molecular targets and signaling pathways implicated in glioblastoma pathogenesis. Targeted therapies, such as small molecule inhibitors, antibody-drug conjugates, and bi-specific antibodies, selectively inhibit oncogenic drivers and vulnerabilities in glioblastoma tumors. Combination therapies that target multiple signaling pathways or exploit synergistic interactions between targeted agents and immunotherapies show promise in overcoming treatment resistance and improving therapeutic outcomes.
Innovative Drug Delivery Systems: Overcoming the blood-brain barrier (BBB) remains a significant challenge in glioblastoma treatment. Innovative drug delivery systems, including nanoparticle-based carriers, liposomes, and focused ultrasound techniques, enhance drug penetration into the brain parenchyma and improve therapeutic efficacy. Targeted drug delivery platforms enable the selective delivery of therapeutic agents to tumor cells while sparing healthy brain tissue, minimizing off-target effects and maximizing treatment benefit.
Combination Therapies: Combination therapy approaches that combine standard treatments (surgery, radiation therapy, chemotherapy) with targeted therapies, immunotherapies, and innovative drug delivery systems are being explored to improve treatment outcomes in glioblastoma. Rational combinations that target complementary pathways or overcome treatment resistance mechanisms offer synergistic effects and enhanced therapeutic efficacy. Clinical trials evaluating combination regimens are ongoing to identify optimal treatment strategies for glioblastoma patients.
Supportive Care and Symptom Management: In addition to anti-tumor therapies, supportive care and symptom management play a crucial role in optimizing quality of life for glioblastoma patients. Palliative care services, psychosocial support, pain management, and symptom relief interventions address the physical, emotional, and social needs of patients and their caregivers throughout the disease trajectory. Integrating supportive care into comprehensive treatment plans improves patient well-being, enhances treatment tolerance, and ensures holistic care delivery.
Clinical Trial Innovation: Clinical trials remain essential for evaluating novel treatments and advancing the standard of care for glioblastoma. Innovative trial designs, biomarker-driven approaches, and adaptive strategies facilitate the rapid evaluation of promising therapies in glioblastoma patients. Collaboration between academia, industry, regulatory agencies, and patient advocacy groups accelerates the translation of scientific discoveries into clinical practice and drives progress in glioblastoma treatment.
Patient-Centered Care Models: Patient-centered care models prioritize the individual needs, preferences, and values of glioblastoma patients throughout their treatment journey. Multidisciplinary care teams, shared decision-making, and personalized treatment plans empower patients to actively participate in their care and make informed treatment choices. Patient support programs, educational resources, and survivorship initiatives promote patient engagement, resilience, and empowerment in coping with the challenges of glioblastoma.
Role of Companies in the Glioblastoma Market
In the Glioblastoma market, companies such as Bayer, Chimerix, Aivita Biomedical, Denovo Biopharma, Northwest Therapeutics, VBL Therapeutics, Laminar Pharmaceuticals, MedImmune, DNAtrix, Immunomic Therapeutics, Imvax, MimiVax, CNS Pharmaceuticals, Epitopoietic Research Corporation (ERC), Istari Oncology, SonALAsense, Kintara Therapeutics, Bristol Myers Squibb, Medicenna Therapeutics, BioMimetix, Eisai, Merck Sharp & Dohme, Kazia Therapeutics, Oblato, Genenta Science, Enterome, Inovio Pharmaceuticals, Karyopharm Therapeutics, Forma Therapeutics, VBI Vaccines, and TME Pharma, and others play a pivotal role in driving innovation, research, development, and the provision of treatments and therapies for individuals suffering from this chronic inflammatory skin condition. These companies encompass pharmaceutical giants, biotechnology firms, medical device manufacturers, and healthcare service providers, each contributing uniquely to the advancement of Glioblastoma management. Pharmaceutical companies lead the charge in developing novel drugs, ranging from topical corticosteroids to biologics targeting specific immune pathways implicated in Glioblastoma pathogenesis.
Glioblastoma Market Outlook - Key Conclusion and Analysis
The Glioblastoma market is undergoing a transformative period, driven by advances in research, innovation in therapeutic approaches, and shifting treatment paradigms. While significant progress has been made in improving outcomes for patients with Glioblastoma, several barriers continue to challenge the market's expansion, including high treatment costs, safety concerns, and regulatory hurdles. Looking ahead, personalized medicine, novel therapeutic targets, and digital health solutions are poised to shape the future of Glioblastoma management, offering new hope for patients and caregivers alike. Efforts to address these challenges and capitalize on emerging opportunities will be critical in advancing the field and ultimately improving the lives of individuals living with Glioblastoma.
Get a more detailed overview, at: Glioblastoma Market Outlook and Forecast
#Glioblastoma market#Glioblastoma treatment market#Glioblastoma therapies#Glioblastoma market share#Glioblastoma market size#Glioblastoma
0 notes
Text
Guide to Water Wash Painting Booths: Benefits & Applications
The Water Wash Painting Booth by GBM Industries is a state-of-the-art solution designed to deliver precision, efficiency, and environmental compliance for industrial painting applications. GBM's Water Wash Painting Booths. Engineered for precision, efficiency, and eco-friendliness, our booths ensure superior air quality and minimal environmental impact. Perfect for industrial and automotive applications.
✨ Read more: Your Guide to Water Wash Painting Booths: Components, Applications, and Environmental Benefits
0 notes
Text
Dry Back Painting Oven Manufacturer and Exporter in India – GBM Industries
Explore the innovative dry back painting ovens from GBM, a leading manufacturer and exporter in India. Learn about their advanced features, efficiency, and role in enhancing industrial painting processes. Ideal for companies looking to optimize their production lines with cutting-edge equipment.
🔗For more information read our article now !! Dry Back Painting Oven Manufacturer and Exporter in India – GBM Industries
0 notes
Text
Great British Menu (GBM) returned to our screens on January 23rd for season 19. The BBC2 show puts the nation’s most talented chefs to the test. At Women in the Food Industry we are continuing our series of interviews with the strong female line-up of chefs from across Britain competing to serve their dish at the final banquet. Our co-founder, Mecca Ibrahim, interviewed Melissa McCabe who runs her own food truck Street FEAST based out of Camlough.
0 notes
Text
GBM Industries - Leading Manufacturer of Batch Ovens in India
GBM Industries is a renowned ISO-certified manufacturer, supplier, and exporter of high-quality batch ovens in India. Our range includes diesel, gas, oil-fired, and specialized ovens for various industrial applications such as baking, coating, drying, and curing. Designed for efficiency, durability, and consistent performance, our batch ovens are engineered to meet diverse industrial needs while optimizing energy usage.
🚀 Take the Next Step – Discover Our latest PPT ! GBM Industries - Leading Manufacturer of Batch Ovens in India
1 note
·
View note
Text
https://www.polarismarketresearch.com/industry-analysis/glioblastoma-multiforme-gbm-treatment-market
0 notes
Text
Enhance Your Production Efficiency with GBM Industries’ IR Conveyor Oven:
🚀 Enhance your production game with GBM Industries' IR Conveyor Oven! 🔥 This cutting-edge solution boosts efficiency, ensures consistent quality, and saves energy. Perfect for streamlining industrial processes and achieving top-notch results. 🌟 🛠️
Take the next step and visit our latest article now !! Enhance Your Production Efficiency with GBM Industries’ IR Conveyor Oven
0 notes
Text
Everything You Need to Know About Industrial Ovens 🔥
GBM Industries offers a premium range of industrial ovens, including advanced batch, curing, and drying ovens. As a leading manufacturer from India 🇮🇳, we provide efficient, custom-designed solutions tailored to your production needs. Our cutting-edge technology ensures high-performance equipment built to meet your specific requirements.
🔗 Visit Now and discover the article : Everything You Need to Know About Industrial Ovens | by Vikas Singh Nayal | Oct, 2024 | Medium
0 notes