#Enzyme Products Database
Explore tagged Tumblr posts
Text
Unlock the Secrets of Pharmaceutical Marketing with Chemxpert Database
Dive into the world of pharmaceutical marketing with Chemxpert Database. Discover insights on the biggest pharmaceutical companies and the top 10 pharmaceutical companies globally. Stay updated on pharma marketing trends and explore detailed profiles of pharmaceutical companies in Germany. With Chemxpert Database, gain a competitive edge in the ever-evolving pharmaceutical industry.
#Industrial Chemicals Database#Food-Additives Database#Excipients Database#Enzyme Products Database#Dyes Chemical Database
1 note
·
View note
Link
1 note
·
View note
Text
Hydroquinone Prices | Pricing | Trend | News | Database | Chart | Forecast
Hydroquinone is a chemical compound widely used in skincare products for its skin-lightening properties. It is primarily used to treat conditions such as hyperpigmentation, melasma, freckles, and age spots. Hydroquinone works by inhibiting the enzyme tyrosinase, which is responsible for the production of melanin, the pigment that gives skin its color. As the demand for hydroquinone has increased in recent years, especially with rising awareness of skincare routines, the price of hydroquinone has become a subject of interest for many, particularly in the cosmetics and pharmaceutical industries. The prices of hydroquinone can fluctuate due to a variety of factors, including raw material costs, manufacturing processes, regulatory policies, supply and demand dynamics, and geographical influences.
Hydroquinone prices are largely influenced by the availability and cost of raw materials. Hydroquinone is synthesized through various chemical processes, and the availability of key chemicals, such as benzene or phenol, directly impacts production costs. When these raw materials experience price hikes due to supply shortages, geopolitical issues, or changes in environmental regulations, the price of hydroquinone rises correspondingly. This chain reaction from raw material costs to the final product pricing makes it important for manufacturers to secure stable sources of these inputs.
Get Real Time Prices for Hydroquinone : https://www.chemanalyst.com/Pricing-data/hydroquinone-1392
Another factor that influences hydroquinone pricing is the scale and efficiency of the manufacturing processes. Hydroquinone production involves complex chemical reactions, and companies with more advanced technologies can often produce the compound at a lower cost. This competitive advantage allows larger manufacturers to offer more competitive pricing while smaller or less technologically advanced producers may struggle to keep their prices low. Furthermore, fluctuations in energy costs also impact production expenses, as hydroquinone synthesis often requires high levels of energy for processing. If energy prices spike, manufacturers may increase product prices to compensate for the added expense.
Regulatory policies also play a critical role in determining hydroquinone prices. In many countries, hydroquinone is tightly regulated due to concerns about its safety when used in high concentrations or over extended periods. Some regions, such as the European Union, have banned the sale of over-the-counter products containing hydroquinone due to potential health risks, while others have imposed strict limits on allowable concentrations. In markets where hydroquinone is highly regulated, compliance costs for manufacturers, including quality control measures, safety testing, and certifications, can drive up production expenses. Consequently, companies operating in these regions may charge more for their products compared to those in less regulated markets.
On the demand side, the popularity of hydroquinone in skincare products is a major driver of price trends. As consumers become more conscious of skincare ingredients and seek solutions for skin conditions like dark spots and uneven skin tone, the demand for products containing hydroquinone has risen. This increase in demand has created a market where prices can rise, especially if supply struggles to keep pace. For cosmetic companies, this heightened demand can lead to higher wholesale prices for hydroquinone, which are then passed on to consumers through more expensive skincare products.
Geographical differences also contribute to variations in hydroquinone prices. Countries with robust chemical manufacturing sectors, such as China and India, often have lower production costs due to economies of scale and access to cheaper raw materials. As a result, hydroquinone sourced from these regions may be more affordable. Conversely, in countries with stricter environmental regulations, higher labor costs, or limited access to raw materials, the price of hydroquinone can be significantly higher. Additionally, shipping and logistics costs, as well as import taxes, can further contribute to the price disparities between different regions.
The impact of market competition also cannot be ignored when analyzing hydroquinone prices. As with many commodities, competition among manufacturers and suppliers can help to stabilize prices or, in some cases, drive them down. When several manufacturers compete for market share, they may offer lower prices to attract buyers. However, in markets where competition is limited or where a few key players dominate, prices can be kept artificially high. In such scenarios, consumers and businesses may face higher costs for hydroquinone-based products.
One more factor affecting hydroquinone prices is the increasing trend toward natural or alternative skin-lightening ingredients. With growing awareness of the potential side effects of hydroquinone, some consumers are shifting toward products that contain natural alternatives like kojic acid, arbutin, or licorice extract. This trend could reduce the demand for hydroquinone in certain markets, potentially leading to lower prices as manufacturers adjust to decreased demand. However, in markets where hydroquinone remains the most effective and popular skin-lightening ingredient, prices may continue to remain high.
In conclusion, the price of hydroquinone is influenced by a complex interplay of factors, including raw material costs, manufacturing efficiency, regulatory policies, supply and demand dynamics, and geographical considerations. The growing demand for hydroquinone in skincare, coupled with competition in the marketplace, will likely continue to shape its pricing trends in the foreseeable future. With the rise of alternative skin-lightening ingredients, it remains to be seen whether hydroquinone prices will stabilize, decrease, or experience further fluctuations. However, for now, hydroquinone remains a key ingredient in many skin treatments, and its price is a reflection of the global forces at play in the beauty and pharmaceutical industries.
Get Real Time Prices for Hydroquinone : https://www.chemanalyst.com/Pricing-data/hydroquinone-1392
Contact Us:
ChemAnalyst
GmbH - S-01, 2.floor, Subbelrather Straße,
15a Cologne, 50823, Germany
Call: +49-221-6505-8833
Email: [email protected]
Website: https://www.chemanalyst.com
#Hydroquinone#Hydroquinone Price#Hydroquinone Prices#Hydroquinone Price Monitor#Hydroquinone News#Hydroquinone Database#Hydroquinone Price Chart
0 notes
Text
Bazopril: Comprehensive Review on Uses, Benefits, Side Effects, and Patient Experience
Click here!
Bazopril is an ACE inhibitor widely prescribed for managing hypertension and heart failure. As a cornerstone in cardiovascular treatment, understanding its mechanism, efficacy, and patient impact is crucial for healthcare professionals.
Mechanism of Action:
Bazopril works by inhibiting the angiotensin-converting enzyme (ACE), which reduces the production of angiotensin II. This leads to vasodilation, reduced blood volume, and consequently lower blood pressure. The drug also decreases the workload on the heart, making it effective for heart failure patients.
Clinical Efficacy:
Clinical trials have shown Bazopril to be effective in reducing blood pressure and improving survival rates in heart failure patients. Comparative studies indicate that Bazopril is as effective as other ACE inhibitors, with some evidence suggesting superior tolerability in certain populations.

Visit this link!
Dosage and Administration:
Bazopril is typically administered once daily, with doses ranging from 5 to 40 mg depending on the condition being treated and patient-specific factors like age and renal function. Lower starting doses are recommended for elderly patients or those with renal impairment, with gradual titration based on response and tolerability.
Side Effects and Safety Profile:
Common side effects include cough, dizziness, and fatigue, which are generally mild and transient. However, serious adverse effects, such as angioedema and hyperkalemia, can occur, particularly in patients with a history of these conditions or those on concomitant medications like potassium-sparing diuretics. Bazopril is contraindicated in pregnant women due to the risk of fetal harm.
Patient Experience and Adherence:
Patient reviews suggest that Bazopril is well-tolerated, with many reporting significant improvements in blood pressure control. However, the persistent cough associated with ACE inhibitors remains a common reason for discontinuation. Adherence is generally high, especially when patients are well-informed about managing side effects and the importance of maintaining therapy.

Click here!
References
1. Smith, J., & Brown, L. (2022). Efficacy of Bazopril in the Management of Hypertension: A Systematic Review*. Journal of Cardiovascular Medicine, 15(3), 123-135. doi:10.1016/j.jcvmed.2022.03.005.
2. Johnson, R., et al. (2021). Comparative Analysis of Bazopril and Other ACE Inhibitors in Heart Failure Treatment*. New England Journal of Medicine, 384(7), 645-656. doi:10.1056/NEJMoa2020589.
3. American Heart Association. (2023). Guidelines for the Treatment of Hypertension*. Retrieved from https://www.heart.org/en/professional/clinical-practice-guidelines/hypertension
4. Food and Drug Administration (FDA). (2024). Bazopril Drug Information. Retrieved from https://www.fda.gov/drugs/drug-approvals-and-databases/bazopril
5. Williams, M., & Lee, H. (2023). Patient Experiences and Adherence to ACE Inhibitors: Focus on Bazopril. Journal of Patient Experience, 10(4), 256-263. doi:10.1177/23743735221130068.
6. Centers for Disease Control and Prevention (CDC). (2024). Chronic Disease Prevention and Management. Retrieved from https://www.cdc.gov/chronicdisease/index.htm
7. Kumar, S., & Patel, V. (2022). Pharmacokinetics and Pharmacodynamics of Bazopril: An Overview. Clinical Pharmacology & Therapeutics, 112(5), 785-795. doi:10.1002/cpt.2401.
8. National Institutes of Health (NIH). (2023). Bazopril Clinical Trials Summary. Retrieved from https://clinicaltrials.gov/ct2/results?term=Bazopril
Click here!
Adjust the references according to the actual sources you use for your content. for the Treatment of Hypertension*. Retrieved from https://www.heart.org/en/professional/clinical-practice-guidelines/hypertension
4. Food and Drug Administration (FDA). (2024). Bazopril Drug Information. Retrieved from https://www.fda.gov/drugs/drug-approvals-and-databases/bazopril
5. Williams, M., & Lee, H. (2023). Patient Experiences and Adherence to ACE Inhibitors: Focus on Bazopril. Journal of Patient Experience, 10(4), 256-263. doi:10.1177/23743735221130068.
6. Centers for Disease Control and Prevention (CDC). (2024). Chronic Disease Prevention and Management. Retrieved from https://www.cdc.gov/chronicdisease/index.htm
7. Kumar, S., & Patel, V. (2022). Pharmacokinetics and Pharmacodynamics of Bazopril: An Overview. Clinical Pharmacology & Therapeutics, 112(5), 785-795. doi:10.1002/cpt.2401.
8. National Institutes of Health (NIH). (2023). Bazopril Clinical Trials Summary. Retrieved from https://clinicaltrials.gov/ct2/results?term=Bazopril
Conclusion:
Bazopril is a reliable and effective option for managing hypertension and heart failure, with a safety profile that is well-established through extensive clinical use. While side effects like cough can affect adherence, the overall benefits in terms of cardiovascular outcomes make Bazopril a valuable part of treatment protocols.
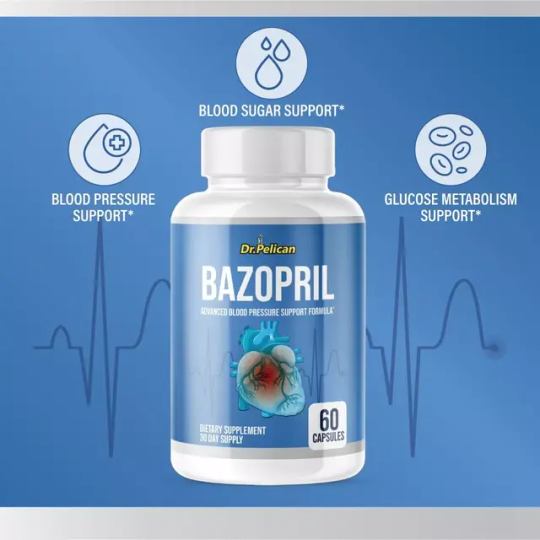




Get Started today Now!
#Bazopril#HypertensionTreatment#HeartFailureMedication#ACEInhibitors#BloodPressureControl#CardiovascularHealth#MedicationReview#Pharmacology#PatientCare#HealthAndWellness#DrugSafety#HealthcareProfessionals#writers on tumblr#clickbank#digital marketing#my post#text post#tumblr milestone#weightloss#artists on tumblr#weight loss#weightlossjourney#viralpost#viral#vintage#virtual photography#healthandwellness#healthylifestyle#fitnesssupplements#health and fitness
1 note
·
View note
Text
Characterization of a marine bacteria through a novel metabologenomics approach
Exploiting microbial natural products is a key pursuit of the bioactive compound discovery field. Recent advances in modern analytical techniques have increased the volume of microbial genomes and their encoded biosynthetic products measured by mass spectrometry-based metabolomics. However, connecting multi-omics data to uncover metabolic processes of interest is still challenging. This results in a large portion of genes and metabolites remaining unannotated. Further exacerbating the annotation challenge, databases and tools for annotation and omics integration are scattered, requiring complex computations to annotate and integrate omics datasets. Here we performed a two-way integrative analysis combining genomics and metabolomics data to describe a new approach to characterize the marine bacterial isolate BRA006 and to explore its biosynthetic gene cluster (BGC) content as well as the bioactive compounds detected by metabolomics. We described BRA006 genomic content and structure by comparing Illumina and Oxford Nanopore MinION sequencing approaches. Digital DNA:DNA hybridization (dDDH) taxonomically assigned BRA006 as a potential new species of the Micromonospora genus. Starting from LC-ESI(+)-HRMS/MS data, and mapping the annotated enzymes and metabolites belonging to the same pathways, our integrative analysis allowed us to correlate the compound Brevianamide F to a new BGC, previously assigned to other function. http://dlvr.it/TBpG2S
0 notes
Text
Medicated Feed Additives Market Size, Share, Comprehensive Analysis, Opportunity Assessment By 2030
The market research study titled “Medicated Feed Additives Market Share, Trends, and Outlook | 2031,” guides organizations on market economics by identifying current Medicated Feed Additives market size, total market share, and revenue potential. This further includes projections on future market size and share in the estimated period. The company needs to comprehend its clientele and the demand it creates to focus on a smaller selection of items. Through this chapter, market size assists businesses in estimating demand in specific marketplaces and comprehending projected patterns for the future.
The Medicated Feed Additives market report also provides in-depth insights into major industry players and their strategies because we understand how important it is to remain ahead of the curve. Companies may utilize the objective insights provided by this market research to identify their strengths and limitations. Companies that can capitalize on the fresh perspective gained from competition analysis are more likely to have an edge in moving forward.
With this comprehensive research roadmap, entrepreneurs and stakeholders can make informed decisions and venture into a successful business. This research further reveals strategies to help companies grow in the Medicated Feed Additives market.
Market Analysis and Forecast
This chapter evaluates several factors that impact on business. The economics of scale described based on market size, growth rate, and CAGR are coupled with future projections of the Medicated Feed Additives market. This chapter is further essential to analyze drivers of demand and restraints ahead of market participants. Understanding Medicated Feed Additives market trends helps companies to manage their products and position themselves in the market gap.
This section offers business environment analysis based on different models. Streamlining revenues and success is crucial for businesses to remain competitive in the Medicated Feed Additives market. Companies can revise their unique selling points and map the economic, environmental, and regulatory aspects.
Report Attributes
Details
Segmental Coverage
Mixture Type
Supplements
Concentrates
Premix Feeds
Base Mixes
Type
Antioxidants
Antibiotics
Probiotics and Prebiotics
Enzymes
Amino Acids
Others
Category
Category I
Category II
Livestock
Ruminants
Poultry
Swine
Aquaculture
Others
Geography
North America
Europe
Asia Pacific
and South and Central America
Regional and Country Coverage
North America (US, Canada, Mexico)
Europe (UK, Germany, France, Russia, Italy, Rest of Europe)
Asia Pacific (China, India, Japan, Australia, Rest of APAC)
South / South & Central America (Brazil, Argentina, Rest of South/South & Central America)
Middle East & Africa (South Africa, Saudi Arabia, UAE, Rest of MEA)
Market Leaders and Key Company Profiles
Adisseo France Sas
Alltech Inc. (Ridley)
Archer Daniels Midland Company
Biostadt India Limited
Cargill, Incorporated
CHS Inc.
Hipro Animal Nutrition
Purina Animal Nutrition (Land O' Lakes)
Zagro
Zoetis Inc.
Other key companies
Our Unique Research Methods at The Insight Partners
We offer syndicated market research solutions and consultation services that provide complete coverage of global markets. This report includes a snapshot of global and regional insights. We pay attention to business growth and partner preferences, that why we offer customization on all our reports to meet individual scope and regional requirements.
Our team of researchers utilizes exhaustive primary research and secondary methods to gather precise and reliable information. Our analysts cross-verify facts to ensure validity. We are committed to offering actionable insights based on our vast research databases.
Strategic Recommendations
Strategic planning is crucial for business success. This section offers strategic recommendations needed for businesses and investors. Forward forward-focused vision of a business is what makes it through thick and thin. Knowing business environment factors helps companies in making strategic moves at the right time in the right direction.
Summary:
Medicated Feed Additives Market Forecast and Growth by Revenue | 2031
Market Dynamics – Leading trends, growth drivers, restraints, and investment opportunities
Market Segmentation – A detailed analysis by product, types, end-user, applications, segments, and geography
Competitive Landscape – Top key players and other prominent vendors
About Us:
The Insight Partners is a one-stop industry research provider of actionable intelligence. We help our clients in getting solutions to their research requirements through our syndicated and consulting research services. We specialize in industries such as Semiconductor and Electronics, Aerospace and Defense, Automotive and Transportation, Biotechnology, Healthcare IT, Manufacturing and Construction, Medical Devices, Technology, Media and Telecommunications, Chemicals and Materials.
Contact Us: www.theinsightpartners.com
0 notes
Text
“Rosetta Stone” of cell signaling could expedite precision cancer medicine
New Post has been published on https://sunalei.org/news/rosetta-stone-of-cell-signaling-could-expedite-precision-cancer-medicine/
“Rosetta Stone” of cell signaling could expedite precision cancer medicine
A newly complete database of human protein kinases and their preferred binding sites provides a powerful new platform to investigate cell signaling pathways.
Culminating 25 years of research, MIT, Harvard University, and Yale University scientists and collaborators have unveiled a comprehensive atlas of human tyrosine kinases — enzymes that regulate a wide variety of cellular activities — and their binding sites.
The addition of tyrosine kinases to a previously published dataset from the same group now completes a free, publicly available atlas of all human kinases and their specific binding sites on proteins, which together orchestrate fundamental cell processes such as growth, cell division, and metabolism.
Now, researchers can use data from mass spectrometry, a common laboratory technique, to identify the kinases involved in normal and dysregulated cell signaling in human tissue, such as during inflammation or cancer progression.
“I am most excited about being able to apply this to individual patients’ tumors and learn about the signaling states of cancer and heterogeneity of that signaling,” says Michael Yaffe, who is the David H. Koch Professor of Science at MIT, the director of the MIT Center for Precision Cancer Medicine, a member of MIT’s Koch Institute for Integrative Cancer Research, and a senior author of the new study. “This could reveal new druggable targets or novel combination therapies.”
The study, published in Nature, is the product of a long-standing collaboration with senior authors Lewis Cantley at Harvard Medical School and Dana-Farber Cancer Institute, Benjamin Turk at Yale School of Medicine, and Jared Johnson at Weill Cornell Medical College.
The paper’s lead authors are Tomer Yaron-Barir at Columbia University Irving Medical Center, and MIT’s Brian Joughin, with contributions from Kontstantin Krismer, Mina Takegami, and Pau Creixell.
Kinase kingdom
Human cells are governed by a network of diverse protein kinases that alter the properties of other proteins by adding or removing chemical compounds called phosphate groups. Phosphate groups are small but powerful: When attached to proteins, they can turn proteins on or off, or even dramatically change their function. Identifying which of the almost 400 human kinases phosphorylate a specific protein at a particular site on the protein was traditionally a lengthy, laborious process.
Beginning in the mid 1990s, the Cantley laboratory developed a method using a library of small peptides to identify the optimal amino acid sequence — called a motif, similar to a scannable barcode — that a kinase targets on its substrate proteins for the addition of a phosphate group. Over the ensuing years, Yaffe, Turk, and Johnson, all of whom spent time as postdocs in the Cantley lab, made seminal advancements in the technique, increasing its throughput, accuracy, and utility.
Johnson led a massive experimental effort exposing batches of kinases to these peptide libraries and observed which kinases phosphorylated which subsets of peptides. In a corresponding Nature paper published in January 2023, the team mapped more than 300 serine/threonine kinases, the other main type of protein kinase, to their motifs. In the current paper, they complete the human “kinome” by successfully mapping 93 tyrosine kinases to their corresponding motifs.
Next, by creating and using advanced computational tools, Yaron-Barir, Krismer, Joughin, Takegami, and Yaffe tested whether the results were predictive of real proteins, and whether the results might reveal unknown signaling events in normal and cancer cells. By analyzing phosphoproteomic data from mass spectrometry to reveal phosphorylation patterns in cells, their atlas accurately predicted tyrosine kinase activity in previously studied cell signaling pathways.
For example, using recently published phosphoproteomic data of human lung cancer cells treated with two targeted drugs, the atlas identified that treatment with erlotinib, a known inhibitor of the protein EGFR, downregulated sites matching a motif for EGFR. Treatment with afatinib, a known HER2 inhibitor, downregulated sites matching the HER2 motif. Unexpectedly, afatinib treatment also upregulated the motif for the tyrosine kinase MET, a finding that helps explain patient data linking MET activity to afatinib drug resistance.
Actionable results
There are two key ways researchers can use the new atlas. First, for a protein of interest that is being phosphorylated, the atlas can be used to narrow down hundreds of kinases to a short list of candidates likely to be involved. “The predictions that come from using this will still need to be validated experimentally, but it’s a huge step forward in making clear predictions that can be tested,” says Yaffe.
Second, the atlas makes phosphoproteomic data more useful and actionable. In the past, researchers might gather phosphoproteomic data from a tissue sample, but it was difficult to know what that data was saying or how to best use it to guide next steps in research. Now, that data can be used to predict which kinases are upregulated or downregulated and therefore which cellular signaling pathways are active or not.
“We now have a new tool now to interpret those large datasets, a Rosetta Stone for phosphoproteomics,” says Yaffe. “It is going to be particularly helpful for turning this type of disease data into actionable items.”
In the context of cancer, phosophoproteomic data from a patient’s tumor biopsy could be used to help doctors quickly identify which kinases and cell signaling pathways are involved in cancer expansion or drug resistance, then use that knowledge to target those pathways with appropriate drug therapy or combination therapy.
Yaffe’s lab and their colleagues at the National Institutes of Health are now using the atlas to seek out new insights into difficult cancers, including appendiceal cancer and neuroendocrine tumors. While many cancers have been shown to have a strong genetic component, such as the genes BRCA1 and BRCA2 in breast cancer, other cancers are not associated with any known genetic cause. “We’re using this atlas to interrogate these tumors that don’t seem to have a clear genetic driver to see if we can identify kinases that are driving cancer progression,” he says.
Biological insights
In addition to completing the human kinase atlas, the team made two biological discoveries in their recent study. First, they identified three main classes of phosphorylation motifs, or barcodes, for tyrosine kinases. The first class is motifs that map to multiple kinases, suggesting that numerous signaling pathways converge to phosphorylate a protein boasting that motif. The second class is motifs with a one-to-one match between motif and kinase, in which only a specific kinase will activate a protein with that motif. This came as a partial surprise, as tyrosine kinases have been thought to have minimal specificity by some in the field.
The final class includes motifs for which there is no clear match to one of the 78 classical tyrosine kinases. This class includes motifs that match to 15 atypical tyrosine kinases known to also phosphorylate serine or threonine residues. “This means that there’s a subset of kinases that we didn’t recognize that are actually playing an important role,” says Yaffe. It also indicates there may be other mechanisms besides motifs alone that affect how a kinase interacts with a protein.
The team also discovered that tyrosine kinase motifs are tightly conserved between humans and the worm species C. elegans, despite the species being separated by more than 600 million years of evolution. In other words, a worm kinase and its human homologue are phosphorylating essentially the same motif. That sequence preservation suggests that tyrosine kinases are highly critical to signaling pathways in all multicellular organisms, and any small change would be harmful to an organism.
The research was funded by the Charles and Marjorie Holloway Foundation, the MIT Center for Precision Cancer Medicine, the Koch Institute Frontier Research Program via L. Scott Ritterbush, the Leukemia and Lymphoma Society, the National Institutes of Health, Cancer Research UK, the Brain Tumour Charity, and the Koch Institute Support (core) grant from the National Cancer Institute.
0 notes
Text
The Role of Machine Learning in Catalysis: Advancing Discovery and Optimization
Harnessing the Power of Artificial Intelligence to Transform Catalysis
Advancements in catalysis research have the potential to revolutionize various industries, from energy production to pharmaceuticals. Catalysis plays a crucial role in accelerating chemical reactions, improving efficiency, and reducing environmental impact. Traditionally, catalysis research has relied on empirical knowledge and trial-and-error approaches.
However, with the advent of machine learning and artificial intelligence (AI), there has been a paradigm shift in how catalysis is studied and optimized. Machine learning algorithms have the ability to analyze vast amounts of data, identify patterns, and make predictions, leading to the discovery of new catalysts and the optimization of existing ones. In this article, we will explore the applications of machine learning in catalysis and how it is transforming the field.
youtube
Predictive Modeling and Catalyst Design
One of the key areas where machine learning has made significant contributions is in predictive modeling and catalyst design. By training algorithms on large datasets of experimental and computational data, researchers can develop models that can accurately predict the performance of catalysts under different conditions. These models take into account various factors such as catalyst composition, structure, and reaction conditions to provide insights into catalytic activity and selectivity.
This approach allows researchers to screen a vast chemical space and identify promising catalyst candidates for specific reactions.
For example, a study by Ahneman et al. used machine learning to predict reaction performance in C-N cross-coupling reactions. By training the algorithm on a dataset of experimental results, the researchers were able to identify key features that contribute to high reaction yields.
This approach not only accelerated the discovery of high-performing catalysts but also provided insights into the underlying reaction mechanisms.
Another study by Kim et al. employed active learning combined with experiments to search for an optimal multi-metallic alloy catalyst. The algorithm iteratively selected new catalyst compositions to synthesize and tested their performance, using the feedback to improve its predictions.
This approach allowed the researchers to rapidly explore a large chemical space and identify a highly efficient catalyst for a specific reaction.
Accelerating Materials Discovery
Machine learning has also played a crucial role in accelerating materials discovery in catalysis. By analyzing large databases of materials properties and performance, algorithms can identify trends and correlations that can guide the design of new materials with desired catalytic properties. This approach has been particularly valuable in the field of heterogeneous catalysis, where the catalyst is in a different phase from the reactants.
For example, the Open Catalyst 2020 (OC20) dataset, developed by Chanussot et al., consists of nearly 1.3 million density functional theory (DFT) relaxations of materials surfaces and adsorbates. This extensive database allows researchers to explore a wide range of materials and reactions, providing valuable insights into the factors that influence catalytic activity and selectivity.
Furthermore, machine learning has been used to analyze X-ray absorption spectra and transmission electron microscopy images of catalysts. These techniques enable researchers to gain a deeper understanding of catalyst structures and their evolution during reactions. Mitchell et al.
developed an automated image analysis method for single-atom detection in catalytic materials using transmission electron microscopy. This approach allows for the identification and characterization of catalytic active sites at the atomic scale, providing valuable insights for catalyst design and optimization.
Enzyme Engineering and Biocatalysis
Machine learning has also been applied to enzyme engineering and biocatalysis, offering new possibilities for the design of highly efficient biocatalysts. By training algorithms on large datasets of enzyme sequences and structures, researchers can develop models that can predict enzyme properties and guide the engineering of novel enzymes with desired functionalities.
For example, Wu et al. used machine learning-assisted directed protein evolution with combinatorial libraries to engineer enzymes for improved properties. The algorithm guided the design of protein libraries and selected variants with desired characteristics for further experimental testing.
This approach allowed for the rapid optimization of enzymes for specific reactions, opening up new possibilities for the synthesis of valuable compounds.
Similarly, Lu et al. employed machine learning-aided engineering to design hydrolases for the depolymerization of polyethylene terephthalate (PET). By training the algorithm on a large dataset of enzyme sequences and PET degradation activities, the researchers were able to predict enzyme variants with enhanced PET-degrading capabilities.
This approach offers a promising solution for the recycling of PET, a major environmental challenge.
Challenges and Opportunities
While machine learning has shown great promise in catalysis research, there are still challenges that need to be addressed. One of the main challenges is the availability and quality of data. High-quality datasets are essential for training accurate machine learning models, but obtaining such datasets can be time-consuming and expensive.
Furthermore, there is a need for standardized data formats and protocols to enable data sharing and collaboration among researchers.
Another challenge is the interpretability of machine learning models. While these models can make accurate predictions, understanding the underlying factors that contribute to the predictions can be challenging. Interpretable machine learning methods, such as decision trees and rule-based models, can help address this issue by providing explanations for the model's predictions.
Despite these challenges, the opportunities offered by machine learning in catalysis research are immense. By combining experimental data, computational modeling, and machine learning algorithms, researchers can accelerate the discovery of new catalysts, optimize existing ones, and gain a deeper understanding of catalytic mechanisms. Machine learning has the potential to revolutionize the field of catalysis and pave the way for more sustainable and efficient chemical processes.
Machine learning has emerged as a powerful tool in catalysis research, enabling the discovery and optimization of catalysts with unprecedented efficiency. By leveraging large datasets, advanced algorithms, and computational modeling, researchers can accelerate the development of new catalysts, gain insights into catalytic mechanisms, and design more sustainable chemical processes. As the field continues to evolve, it is essential to address challenges related to data availability, model interpretability, and standardization.
With continued advancements in machine learning and catalysis research, we can expect to see further breakthroughs and innovations in the field, leading to a more sustainable and efficient future.
0 notes
Text
Deep learning for single-cell sequencing: a microscope to see the diversity of cells
New Post has been published on https://thedigitalinsider.com/deep-learning-for-single-cell-sequencing-a-microscope-to-see-the-diversity-of-cells/
Deep learning for single-cell sequencing: a microscope to see the diversity of cells
The history of each living being is written in its genome, which is stored as DNA and present in nearly every cell of the body. No two cells are the same, even if they share the same DNA and cell type, as they still differ in the regulators that control how DNA is expressed by the cell. The human genome consists of 3 billion base pairs spread over 23 chromosomes. Within this vast genetic code, there are approximately 20,000 to 25,000 genes, constituting the protein-coding DNA and accounting for about 1% of the total genome [1]. To explore the functioning of complex systems in our bodies, especially this small coding portion of DNA, a precise sequencing method is necessary, and single-cell sequencing (sc-seq) technology fits this purpose.
In 2013, Nature selected single-cell RNA sequencing as the Method of the Year [2] (Figure 3), highlighting the importance of this method for exploring cellular heterogeneity through the sequencing of DNA and RNA at the individual cell level. Subsequently, numerous tools have emerged for the analysis of single-cell RNA sequencing data. For example, the scRNA-tools database has been compiling software for the analysis of single-cell RNA data since 2016, and by 2021, the database includes over 1000 tools [3]. Among these tools, many involve methods that leverage Deep Learning techniques, which will be the focus of this article – we will explore the pivotal role that Deep Learning, in particular, has played as a key enabler for advancing single-cell sequencing technologies.
Background
Flow of genetic information from DNA to protein in cells
Let’s first go over what exactly cells and sequences are. The cell is the fundamental unit of our bodies and the key to understanding how our bodies function in good health and how molecular dysfunction leads to disease. Our bodies are made of trillions of cells, and nearly every cell contains three genetic information layers: DNA, RNA, and protein. DNA is a long molecule containing the genetic code that makes each person unique. Like a source code, it includes several instructions showing how to make each protein in our bodies. These proteins are the workhorses of the cell that carry out nearly every task necessary for cellular life. For example, the enzymes that catalyze chemical reactions within the cell and DNA polymerases that contribute to DNA replication during cell division, are all proteins. The cell synthesizes proteins in two steps: Transcription and Translation (Figure 1), which are known as gene expression. DNA is first transcribed into RNA, then RNA is translated into protein. We can consider RNA as a messenger between DNA and protein.
Figure 1. The central dogma of biology
While the cells of our body share the same DNA, they vary in their biological activity. For instance, the distinctions between immune cells and heart cells are determined by the genes that are either activated or deactivated in these cells. Generally, when a gene is activated, it leads to the creation of more RNA copies, resulting in increased protein production. Therefore, as cell types differ based on the quantity and type of RNA/protein molecules synthesized, it becomes intriguing to assess the abundance of these molecules at the single-cell level. This will enable us to investigate the behavior of our DNA within each cell and attain a high-resolution perspective of the various parts of our bodies.
In general, all single-cell sequencing technologies can be divided into three main steps:
Isolation of single cells from the tissue of interest and extraction of genetic material from each isolated cell
Amplification of genetic material from each isolated cell and library preparation
Sequencing of the library using a next-generation sequencer and data analysis
Navigating through the intricate steps of cellular biology and single-cell sequencing technologies, a pivotal question emerges: How is single-cell sequencing data represented numerically?
Structure of single-cell sequencing data
The structure of single-cell sequencing data takes the form of a matrix (Figure 2), where each row corresponds to a cell that has been sequenced and annotated with a unique barcode. The number of rows equals the total number of cells analyzed in the experiment. On the other hand, each column corresponds to a specific gene. Genes are the functional units of the genome that encode instructions for the synthesis of proteins or other functional molecules. In the case of scRNA seq data, the numerical entries in the matrix represent the expression levels of genes in individual cells. These values indicate the amount of RNA produced from each gene in a particular cell, providing insights into the activity of genes within different cells.
Figure 2. Schema of single-cell sequencing data
Single Cell Sequencing Overview
For more than 150 years, biologists have wanted to identify all the cell types in the human body and classify them into distinct types based on accurate descriptions of their properties. The Human Cell Atlas Project (HCAP), the genetic equivalent of the Human Genome Project [4], is an international collaborative effort to map all the cells in the human body.” We can conceptualize the Human Cell Atlas as a map endeavoring to portray the human body coherently and systematically. Much like Google Maps, which allows us to zoom in for a closer examination of intricate details, the Human Cell Atlas provides insights into spatial information, internal attributes, and even the relationships among elements”, explains Aviv Regev, a computational and systems biologist at the Broad Institute of MIT and Harvard and Executive Vice President and Head of Genentech Research.
This analogy seamlessly aligns with the broader impact of single-cell sequencing, since it allows the analysis of individual cells instead of bulk populations. This technology proves invaluable in addressing intricate biological inquiries related to developmental processes and comprehending heterogeneous cellular or genetic changes under various treatment conditions or disease states. Additionally, it facilitates the identification of novel cell types within a given cellular population. The initiation of the first single-cell RNA sequencing (scRNA-seq) paper in 2009 [5], subsequently designated as the “method of the year” in 2013 [2], marked the genesis of an extensive endeavor to advance both experimental and computational techniques dedicated to unraveling the intricacies of single-cell transcriptomes.
As the technological landscape evolves, the narrative transitions to the advancements in single-cell research, particularly the early focus on single-cell RNA sequencing (scRNA-seq) due to its cost-effectiveness in studying complex cell populations.” In some ways, RNA has always been one of the easiest things to measure,” says Satija [6], a researcher at the New York Genome Center (NYGC). Yet, the rapid development of single-cell technology has ushered in a new era of possibilities—multimodal single-cell data integration. Recognized as the “Method of the Year 2019” by Nature [7] (Figure 3), this approach allows the measurement of different cellular modalities, including the genome, epigenome, and proteome, within the same cell. The layering of multiple pieces of information provides powerful insights into cellular identity, posing the challenge of effectively modeling and combining datasets generated from multimodal measurements. This integration challenge is met with the introduction of Multi-view learning [8] methods, exploring common variations across modalities. This sophisticated approach, incorporating deep learning techniques, showcases relevant results across various fields, particularly in biology and biomedicine.
Amidst these advancements, a distinct challenge surfaces in the persistent limitation of single-cell RNA sequencing—the loss of spatial information during transcriptome profiling by isolating cells from their original position. Spatially resolved transcriptomics (SRT) emerges as a pivotal solution [9], addressing the challenge by preserving spatial details during the study of complex biological systems. This recognition of spatially resolved transcriptomics as the method of the year 2020 solidifies its place as a critical solution to the challenges inherent in advancing our understanding of complex biological systems.
Figure 3. Evolution of single-cell sequencing over time
Having explored the panorama of single-cell sequencing, let us now delve into the role of deep learning in the context of single-cell sequencing.
Deep Learning on single-cell sequencing
Deep learning is increasingly employed in single-cell analysis due to its capacity to handle the complexity of single-cell sequencing data. In contrast, conventional machine-learning approaches require significant effort to develop a feature engineering strategy, typically designed by domain experts. The deep learning approach, however, autonomously captures relevant characteristics from single-cell sequencing data, addressing the heterogeneity between single-cell sequencing experiments, as well as the associated noise and sparsity in such data. Below are three key reasons for the application of deep learning in single-cell sequencing:
High-Dimensional Data: Single-cell sequencing generates high-dimensional data, with thousands of genes and their expression levels measured for each cell. Deep learning models are adept at capturing complex relationships and patterns within this data, which can be challenging for traditional statistical methods.
Non-Linearity: Single-cell gene expression data is characterized by its inherent nonlinearity between gene expressions and cell-to-cell heterogeneity. Traditional statistical methods encounter difficulties in capturing the non-linear relationships present in single-cell gene expression data. In contrast, deep learning models are flexible and able to learn complex non-linear mappings.
Heterogeneity: Single-cell data is often characterized by diverse cell populations with varying gene expression profiles, presenting a complex landscape. Deep learning models can play a crucial role in identifying, clustering, and characterizing these distinct cell types or subpopulations, thereby facilitating a deeper understanding of cellular heterogeneity within a sample.
As we explore the reasons behind using deep learning in single-cell sequencing data, it leads us to the question: What deep learning architectures are often used in sc-seq data analysis?
Background on Autoencoders
Autoencoders (AEs) stand out among various deep-learning architectures (such as GANs and RNNs) as an especially relied upon method for decoding the complexities of single-cell sequencing data. Widely employed for dimensionality reduction while preserving the inherent heterogeneity in the single-cell sequencing data. By clustering cells in the reduced-dimensional space generated by autoencoders, researchers can effectively identify and characterize different cell types or subpopulations. This approach enhances our ability to discern and analyze the diverse cellular components within single-cell datasets. In contrast to non-deep learning models, such as principal component analysis (PCA), which are integral components of established scRNA-seq data analysis software like Seurat [10], autoencoders distinguish themselves by uncovering non-linear manifolds. While PCA is constrained to linear transformations, the flexibility of autoencoders to capture complex non-linear mappings makes it an advanced method to find nuanced relationships embedded in single-cell genomics.
To mitigate the overfitting challenge associated with autoencoders, several enhancements to the autoencoder structure have been implemented, specifically tailored to offer advantages in the context of sc-seq data. One notable adaptation often used in the context of sc-seq data is the denoising autoencoder (DAEs), which amplifies the autoencoder’s reconstruction capability by introducing noise to the initial network layer. This involves randomly transforming some of its units to zero. The Denoising Autoencoder then reconstructs the input from this intentionally corrupted version, empowering the network to capture more relevant features and preventing it from merely memorizing the input (overfitting). This refinement significantly bolsters the model’s resilience against data noise, thereby elevating the quality of the low-dimensional representation of samples (i.e., bottleneck) derived from the sc-seq data.
A third variation of autoencoders frequently employed in sc-seq data analysis is variational autoencoders (VAEs), exemplified by models like scGen [19], scVI [14], scANVI [28], etc. VAEs, as a type of generative model, learn a latent representation distribution of the data. Instead of encoding the data into a vector of p-dimensional latent variables, the data is encoded into two vectors of size p: a vector of means η and a vector of standard deviations σ. VAEs introduce a probabilistic element to the encoding process, facilitating the generation of synthetic single-cell data and offering insights into the diversity within a cell population. This nuanced approach adds another layer of complexity and richness to the exploration of single-cell genomics.
Applications of deep learning in sc-seq data analysis
This section outlines the main applications of deep learning in improving various stages of sc-seq data analysis, highlighting its effectiveness in advancing crucial aspects of the process.
scRNA-seq data imputation and denoising
Single-cell RNA sequencing (scRNA-seq) data encounter inherent challenges, with dropout events being a prominent concern that leads to significant issues—resulting in sparsity within the gene expression matrix, often characterized by a substantial number of zero values. This sparsity significantly shapes downstream bioinformatics analyses. Many of these zero values arise artificially due to deficiencies in sequencing techniques, including problems like inadequate gene expression, low capture rates, sequencing depth, or other technical factors. As a consequence, the observed zero values do not accurately reflect the true underlying expression levels. Hence, not all zeros in scRNA-seq data can be considered mere missing values, deviating from the conventional statistical approach of imputing missing data values. Given the intricate distinction between true and false zero counts, traditional imputation methods with predefined missing values may prove inadequate for scRNA-seq data. For instance, a classical imputation method, like Mean Imputation, might entail substituting these zero values with the average expression level of that gene across all cells. However, this approach runs the risk of oversimplifying the complexities introduced by dropout events in scRNA-seq data, potentially leading to biased interpretations.
ScRNA-seq data imputation methods can be divided into two categories: deep learning–based imputation method and non–deep learning imputation method. The non–deep learning imputation algorithms involve fitting statistical probability models or utilizing the expression matrix for smoothing and diffusion. This simplicity renders it effective for certain types of samples. For example, Wagner et al. [11] utilized the k-nearest neighbors (KNN) method, identifying nearest neighbors between cells and aggregating gene-specific Unique Molecular Identifiers (UMI) counts to impute the gene expression matrix. In contrast, Huang et al. [12] proposed the SVAER algorithm, leveraging gene-to-gene relationships for imputing the gene expression matrix. For larger datasets (comprising tens of thousands or more), high-dimensional, sparse, and complex scRNA-seq data, traditional computational methods face difficulties, often rendering analysis using these methods difficult and infeasible. Consequently, many researchers have turned to designing methods based on deep learning to address these challenges.
Most deep learning algorithms for imputing dropout events are based on autoencoders (AEs). For instance, in 2018, Eraslan et al. [13] introduced the deep count autoencoder (DCA). DCA utilizes a deep autoencoder architecture to address dropout events in single-cell RNA sequencing (scRNA-seq) data. It incorporates a probabilistic layer in the decoder to model the dropout process. This probabilistic layer accommodates the uncertainty associated with dropout events, enabling the model to generate a distribution of possible imputed values. To capture the characteristics of count data in scRNA-seq, DCA models the observed counts as originating from a negative binomial distribution.
Single-cell variational inference (scVI) is another deep learning algorithm introduced by Lopez et al. [14]. ScVI is a probabilistic variational autoencoder (VAE) that combines deep learning and probabilistic modeling to capture the underlying structure of the scRNA-seq data. ScVI can be used for imputation, denoising, and various other tasks related to the analysis of scRNA-seq data. In contrast to the DCA model, scVI employs Zero-Inflated Negative Binomial (ZINB) distribution in the decoder part to generate a distribution of possible counts for each gene in each cell. The Zero-Inflated Negative Binomial (ZINB) distribution allows modeling the probability of a gene expression being zero (to model dropout events) as well as the distribution of positive values (to model non-zero counts).
Additionally, another study addressed the scRNA-seq data imputation challenge by introducing a recurrent network layer in their model, known as scScope [15]. This novel architecture iteratively performs imputations on zero-valued entries of input scRNA-seq data. The flexibility of scScope’s design allows for the iterative improvement of imputed outputs through a chosen number of recurrent steps (T). Noteworthy is the fact that reducing the time recurrence of scScope to one (i.e., T = 1) transforms the model into a traditional autoencoder (AE). As scScope is essentially a modification of traditional AEs, its runtime is comparable to other AE-based models.
It’s important to note that the application of deep learning in scRNA-seq data imputation and denoising is particularly advantageous due to its ability to capture non-linear relationships among genes. This contrasts with standard linear approaches, making deep learning more adept at providing informed and accurate imputation strategies in the context of single-cell genomics.
Batch effect removal
Single-cell data is commonly aggregated from diverse experiments that vary in terms of experimental laboratories, protocols, sample compositions, and even technology platforms. These differences result in significant variations or batch effects within the data, posing a challenge in the analysis of biological variations of interest during the process of data integration. To address this issue, it becomes necessary to correct batch effects by removing technical variance when integrating cells from different batches or studies. The first method that appears for batch correction is a linear method based on linear regression such as Limma package [16] that provides the removeBatchEffect function which fits a linear model that considers the batches and their impact on gene expression. After fitting the model, it sets the coefficients associated with each batch to zero, effectively removing their impact. Another method called ComBat [17] does something similar but adds an extra step to refine the process, making the correction even more accurate by using a technique called empirical Bayes shrinkage.
However, batch effects can be highly nonlinear, making it difficult to correctly align different datasets while preserving key biological variations. In 2018, Haghverdi et al. introduced the Mutual Nearest Neighbors (MNN) algorithm to identify pairs of cells from different batches in single-cell data [18]. These identified mutual nearest neighbors aid in estimating batch effects between batches. By applying this correction, the gene expression values are adjusted to account for the estimated batch effects, aligning them more closely and reducing discrepancies introduced by the different batches. For extensive single-cell datasets with highly nonlinear batch effects, traditional methods may prove less effective, prompting researchers to explore the application of neural networks for improved batch correction.
One of the pioneering models that employ deep learning for batch correction is the scGen model. Developed by Lotfollahi et al., ScGen [19] utilizes a variational autoencoder (VAE) architecture. This involves pre-training a VAE model on a reference dataset to adjust real single-cell data and alleviate batch effects. Initially, the VAE is trained to capture latent features within the reference dataset’s cells. Subsequently, this trained VAE is applied to the actual data, producing latent representations for each cell. The adjustment of gene expression profiles is then based on aligning these latent representations, to reduce batch effects and harmonize profiles across different experimental conditions.
Figure 4. scGen removes batch effects [19]. a, UMAP visualization of 4 technically diverse pancreatic datasets with their corresponding batch and cell types. b, Data corrected by scGen mixes shared cell types from different studies while preserving the biological variance of cells.
On the other hand, Zou et al. introduced DeepMNN [20], which employs a residual neural network and the mutual nearest neighbor (MNN) algorithm for scRNA-seq data batch correction. Initially, MNN pairs are identified across batches in a principal component analysis (PCA) subspace. Subsequently, a batch correction network is constructed using two stacked residual blocks to remove batch effects. The loss function of DeepMNN comprises a batch loss, computed based on the distance between cells in MNN pairs in the PCA subspace, and a weighted regularization loss, ensuring the network’s output similarity to the input.
The majority of existing scRNA-seq methods are designed to remove batch effects first and then cluster cells, which potentially overlooks certain rare cell types. Recently, Xiaokang et al. developed scDML [21], a deep metric learning model to remove batch effect in scRNA-seq data, guided by the initial clusters and the nearest neighbor information intra and inter-batches. First, the graph-based clustering algorithm is used to group cells based on gene expression similarities, then the KNN algorithm is applied to identify k-nearest neighbors for each cell in the dataset, and the MNN algorithm to identify mutual nearest neighbors, focusing on reciprocal relationships between cells. To remove batch effects, deep triplet learning is employed, considering hard triplets. This helps in learning a low-dimensional embedding that accounts for the original high-dimensional gene expression and removes batch effects simultaneously.
Cell type annotation
Cell type annotation in single-cell sequencing involves the process of identifying and labeling individual cells based on their gene expression profiles, which allows researchers to capture the diversity within a heterogeneous population of cells, and understand the cellular composition of tissues, and the functional roles of different cell types in biological processes or diseases. Traditionally, researchers have used manual methods [22] to annotate cell sub-populations. This involves identifying gene markers or gene signatures that are differentially expressed in a specific cell cluster. Once gene markers are identified, researchers manually interpret the biological relevance of these markers to assign cell-type labels to the clusters. This traditional manual annotation approach is time-consuming and requires considerable human effort, especially when dealing with large-scale single-cell datasets. Due to the challenges associated with manual annotation, researchers are turning to automate and streamline the cell annotation process.
Two primary strategies are employed for cell type annotation: unsupervised-based and supervised-based. In the unsupervised realm, clustering methods such as Scanpy [23] and Seurat [10] are utilized, demanding prior knowledge of established cellular markers. The identification of clusters hinges on the unsupervised grouping of cells without external reference information. However, a drawback to this approach is a potential decrease in replicability with an increased number of clusters and multiple selections of cluster marker genes.
Conversely, supervised-based strategies rely on deep-learning models trained on labeled data. These models discern intricate patterns and relationships within gene expression data during training, enabling them to predict cell types for unlabeled data based on acquired patterns. For example, Joint Integration and Discrimination (JIND) [24] deploys a GAN-style deep architecture, where an encoder is pre-trained on classification tasks, circumventing the need for an autoencoder framework. This model also accounts for batch effects. AutoClass [25] integrates an autoencoder and a classifier, combining output reconstruction loss with a classification loss for cell annotation alongside data imputation. Additionally, TransCluster, [26] rooted in the Transformer framework and convolutional neural network (CNN), employs feature extraction from the gene expression matrix for single-cell annotation.
Despite the power of deep neural networks, obtaining a large number of accurately and unbiasedly annotated cells for training is challenging, given the labor-intensive manual inspection of marker genes in scRNAseq data. In response, semi-supervised learning has been leveraged in computational cell annotation. For instance, the SemiRNet [27] model uses both unlabeled and a limited amount of labeled scRNAseq cells to implement cell identification. SemiRNet, based on recurrent convolutional neural networks (RCNN), incorporates a shared network, a supervised network, and an unsupervised network. Furthermore, single‐cell ANnotation using Variational Inference (scANVI) [28], a semi‐supervised variant of scVI [14], maximizes the utility of existing cell state annotations. Cell BLAST, an autoencoder-based generative model, harnesses large-scale reference databases to learn nonlinear low-dimensional representations of cells, employing a sophisticated cell similarity metric—normalized projection distance—to map query cells to specific cell types and identify novel cell types.
Multi-omics Data Integration
Recent studies have demonstrated the potential of deep learning models in addressing complex and multimodal biological challenges [29]. Among the algorithms proposed thus far, it is primarily deep learning-based models that provide the essential computational adaptability necessary for effectively modeling and incorporating nearly any form of omic data including genomics (studying DNA sequences and genetic variations), epigenomics (examining changes in gene activity unrelated to DNA sequence, such as DNA modifications and chromatin structure), transcriptomics (investigating RNA molecules and gene expression through RNA sequencing), and proteomics (analyzing all proteins produced by an organism, including structures, abundances, and modifications). Deep Learning architectures, including autoencoders (AE) and generative adversarial networks (GAN), have been often used in multi-omics integration problems in single cells. The key question in multi-omics integration revolves around how to effectively represent the diverse multi-omics data within a unified latent space.
One of the early methods developed using Variational Autoencoders (VAE) for the integration of multi-omics single-cell data is known as totalVI [30]. The totalVI model, which is VAE-based, offers a solution for effectively merging scRNA-seq and protein data. In this model, totalVI takes input matrices containing scRNA-seq and protein count data. Specifically, it treats gene expression data as sampled from a negative binomial distribution, while protein data are treated as sampled from a mixture model consisting of two negative binomial distributions. The model first learns shared latent space representations through its encoder, which are then utilized to reconstruct the original data, taking into account the differences between the two original data modalities. Lastly, the decoder component estimates the parameters of the underlying distributions for both data modalities using the shared latent representation.
On the other hand, Zuo et al. [31] introduced scMVAE as a multimodal variational autoencoder designed to integrate transcriptomic and chromatin accessibility data in the same individual cells. scMVAE employs two separate single-modal encoders and two single-modal decoders to effectively model both transcriptomic and chromatin data. It achieves this by combining three distinct joint-learning strategies with a probabilistic Gaussian Mixture Model.
Figure 5 . UMAP embedding for the latent space of the MULTIGRATE for CITE-seq dataset combines gene expression and cell surface protein data [32].
Recently, Lotfollahi et al. [32] introduced an unsupervised deep generative model known as MULTIGRATE for the integration of multi-omic datasets. MULTIGRATE employs a multi-modal variational autoencoder structure that shares some similarities with the scMVAE model. However, it offers added generality and the capability to integrate both paired and unpaired single-cell data. To enhance cell alignment, the loss function incorporates Maximum Mean Discrepancy (MMD), penalizing any misalignment between the point clouds associated with different assays. Incorporating transfer learning, MULTIGRATE can map new multi-omic query datasets into a reference atlas and also perform imputations for missing modalities.
Conclusion
The application of deep learning in single-cell sequencing functions as an advanced microscope, revealing intricate insights within individual cells and providing a profound understanding of cellular heterogeneity and complexity in biological systems. This cutting-edge technology empowers scientists to explore previously undiscovered aspects of cellular behavior. However, the challenge lies in choosing between traditional tools and the plethora of available deep-learning options. The landscape of tools is vast, and researchers must carefully consider factors such as data type, complexity, and the specific biological questions at hand. Navigating this decision-making process requires a thoughtful evaluation of the strengths and limitations of each tool in relation to research goals.
On the other hand, a critical need in the development of deep learning approaches for single-cell RNA sequencing (scRNA-seq) analysis is robust benchmarking. While many studies compare deep learning performance to standard methods, there is a lack of comprehensive comparisons across various deep learning models. Moreover, methods often claim superiority based on specific datasets and tissues (e.g., pancreas cells, immune cells), making it challenging to evaluate the necessity of specific terms or preprocessing steps. Addressing these challenges requires an understanding of when deep learning models fail and their limitations. Recognizing which types of deep learning approaches and model structures are beneficial in specific cases is crucial for developing new approaches and guiding the field.
In the realm of multi-omics single-cell integration, most deep learning methods aim to find a shared latent representation for all modalities. However, shared representation learning faces challenges such as heightened noise, sparsity, and the intricate task of balancing modalities. Inherent biases across institutions complicate generalization. Despite being less prevalent than single-modality approaches, integrating diverse modalities with unique cell populations is crucial. Objectives include predicting expression across modalities and identifying cells in similar states. Despite advancements, further efforts are essential for enhanced performance, particularly concerning unique or rare cell populations present in one technology but not the other.
Author Bio
Fatima Zahra El Hajji holds a master’s degree in bioinformatics from the National School of Computer Science and Systems Analysis (ENSIAS), she subsequently worked as an AI intern at Piercing Star Technologies. Currently, she is a Ph.D. student at the University Mohammed VI Polytechnic (UM6P), working under the supervision of Dr. Rachid El Fatimy and Dr. Tariq Daouda. Her research focuses on the application of deep learning techniques in single-cell sequencing data.
Citation
For attribution in academic contexts or books, please cite this work as
Fatima Zahra El Hajji, "Deep learning for single-cell sequencing: a microscope to see the diversity of cells", The Gradient, 2024.
BibTeX citation:
@article{elhajji2023nar, author = El Hajji, Fatima Zahra, title = Deep learning for single-cell sequencing: a microscope to see the diversity of cells, journal = The Gradient, year = 2024, howpublished = urldeep-learning-for-single-cell-sequencing-a-microscope-to-uncover-the-rich-diversity-of-individual-cells,
References
National Human Genome Research Institute (NHGRI) : A Brief Guide to Genomics , https://www.genome.gov/about-genomics/fact-sheets/A-Brief-Guide-to-Genomics
Method of the Year 2013. Nat Methods 11, 1 (2014). https://doi.org/10.1038/nmeth.2801
Zappia, L., Theis, F.J. Over 1000 tools reveal trends in the single-cell RNA-seq analysis landscape. Genome Biol 22, 301 (2021). https://doi.org/10.1186/s13059-021-02519-4
Collins FS, Fink L. The Human Genome Project. Alcohol Health Res World. 1995;19(3):190-195. PMID: 31798046; PMCID: PMC6875757.
Tang F, Barbacioru C, Wang Y, et al. mRNA-Seq whole-transcriptome analysis of a single cell. Nat Methods. 2009; 6: 377-382.
Eisenstein, M. The secret life of cells. Nat Methods 17, 7–10 (2020). https://doi.org/10.1038/s41592-019-0698-y
Method of the Year 2019: Single-cell multimodal omics. Nat Methods 17, 1 (2020). https://doi.org/10.1038/s41592-019-0703-5
Zhao, Jing et al. “Multi-view learning overview: Recent progress and new challenges.” Inf. Fusion 38 (2017): 43-54.
Zhu, J., Shang, L. & Zhou, X. SRTsim: spatial pattern preserving simulations for spatially resolved transcriptomics. Genome Biol 24, 39 (2023).
Butler, A., Hoffman, P., Smibert, P., Papalexi, E., & Satija, R. (2018). Integrating single-cell transcriptomic data across different conditions, technologies, and species. Nature biotechnology, 36(5), 411-420
Wagner, F., Yan, Y., & Yanai, I. (2018). K-nearest neighbor smoothing for high-throughput single-cell RNA-Seq data. bioRxiv, 217737. Cold Spring Harbor Laboratory. https://doi.org/10.1101/217737
Huang, M., Wang, J., Torre, E. et al. SAVER: gene expression recovery for single-cell RNA sequencing. Nat Methods 15, 539–542 (2018). https://doi.org/10.1038/s41592-018-0033-z
Eraslan G, Simon LM, Mircea M, Mueller NS, Theis FJ. Single-cell RNA-seq denoising using a deep count autoencoder. Nat Commun. 2019 Jan 23;10(1):390. doi: 10.1038/s41467-018-07931-2. PMID: 30674886; PMCID: PMC6344535.
Lopez, R., Regier, J., Cole, M. B., Jordan, M. I.,& Yosef, N. (2018). Deep generative modeling for single-cell transcriptomics. Nature methods, 15(12), 1053-1058.
Y. Deng, F. Bao, Q. Dai, L.F. Wu, S.J. Altschuler Scalable analysis of cell-type composition from single-cell transcriptomics using deep recurrent learning
Ritchie ME, Phipson B, Wu D, Hu Y, Law CW, Shi W, Smyth GK. limma powers differential expression analyses for RNA-sequencing and microarray studies. Nucleic Acids Res. 2015 Apr 20;43(7):e47. doi: 10.1093/nar/gkv007. Epub 2015 Jan 20. PMID: 25605792; PMCID: PMC4402510.
Johnson W.E. , Li C., Rabinovic A. Adjusting batch effects in microarray expression data using empirical bayes methods. Biostatistics. 2007; 8:118–127.
Haghverdi, L., Lun, A., Morgan, M. et al. Batch effects in single-cell RNA-sequencing data are corrected by matching mutual nearest neighbors. Nat Biotechnol 36, 421–427 (2018). https://doi.org/10.1038/nbt.4091
Lotfollahi, M., Wolf, F. A., & Theis, F. J. (2019). scGen predicts single-cell perturbation responses. Nature methods, 16(8), 715-721.
Zou, B., Zhang, T., Zhou, R., Jiang, X., Yang, H., Jin, X., & Bai, Y. (2021). deepMNN: deep learning-based single-cell RNA sequencing data batch correction using mutual nearest neighbors. Frontiers in Genetics, 1441.
Yu, X., Xu, X., Zhang, J. et al. Batch alignment of single-cell transcriptomics data using deep metric learning. Nat Commun 14, 960 (2023). https://doi.org/10.1038/s41467-023-36635-5
Z.A. Clarke, T.S. Andrews, J. Atif, D. Pouyabahar, B.T. Innes, S.A. MacParland, et al. Tutorial: guidelines for annotating single-cell transcriptomic maps using automated and manual methods Nat Protoc, 16 (2021), pp. 2749-2764
Wolf, F., Angerer, P. & Theis, F. SCANPY: large-scale single-cell gene expression data analysis. Genome Biol 19, 15 (2018). https://doi.org/10.1186/s13059-017-1382-0
Mohit Goyal, Guillermo Serrano, Josepmaria Argemi, Ilan Shomorony, Mikel Hernaez, Idoia Ochoa, JIND: joint integration and discrimination for automated single-cell annotation, Bioinformatics, Volume 38, Issue 9, March 2022, Pages 2488–2495, https://doi.org/10.1093/bioinformatics/btac140
H. Li, C.R. Brouwer, W. Luo A universal deep neural network for in-depth cleaning of single-cell RNA-seq data Nat Commun, 13 (2022), p. 1901
Song T, Dai H, Wang S, Wang G, Zhang X, Zhang Y and Jiao L (2022) TransCluster: A Cell-Type Identification Method for single-cell RNA-Seq data using deep learning based on transformer. Front. Genet. 13:1038919. doi: 10.3389/fgene.2022.1038919
Dong X, Chowdhury S, Victor U, Li X, Qian L. Semi-Supervised Deep Learning for Cell Type Identification From Single-Cell Transcriptomic Data. IEEE/ACM Trans Comput Biol Bioinform. 2023 Mar-Apr;20(2):1492-1505. doi: 10.1109/TCBB.2022.3173587. Epub 2023 Apr 3. PMID: 35536811.
Xu, C., Lopez, R., Mehlman, E., Regier, J., Jordan, M. I., & Yosef, N. (2021). Probabilistic harmonization and annotation of single‐cell transcriptomics data with deep generative models. Molecular Systems Biology, 17(1), e9620. https://doi.org/10.15252/msb.20209620
Tasbiraha Athaya, Rony Chowdhury Ripan, Xiaoman Li, Haiyan Hu, Multimodal deep learning approaches for single-cell multi-omics data integration, Briefings in Bioinformatics, Volume 24, Issue 5, September 2023, bbad313, https://doi.org/10.1093/bib/bbad313
Gayoso, A., Lopez, R., Steier, Z., Regier, J., Streets, A., & Yosef, N. (2019). A Joint Model of RNA Expression and Surface Protein Abundance in Single Cells. bioRxiv, 791947. https://www.biorxiv.org/content/early/2019/10/07/791947.abstract
Chunman Zuo, Luonan Chen. Deep-joint-learning analysis model of single cell transcriptome and open chromatin accessibility data. Briefings in Bioinformatics. 2020.
Lotfollahi, M., Litinetskaya, A., & Theis, F. J. (2022). Multigrate: single-cell multi-omic data integration.bioRxiv.https://www.biorxiv.org/content/early/2022/03/17/2022.03.16.484643
#000#2022#2023#2024#Accessibility#accounting#Accounts#acids#ai#alcohol#algorithm#Algorithms#amp#Analysis#applications#approach#architecture#Article#background#Behavior#billion#Biology#biomedicine#biotechnology#Books#Broad Institute#Capture#cell#cell types#Cells
1 note
·
View note
Text
Silage Additives Market Share Growing High CAGR During 2023-29
The Silage Additives Market Report provides an exhaustive analysis of the growth drivers, current trends, restraining forces, and opportunities present in the market.
The global Silage additives market size was valued at USD 2,523.2 million in 2022 and is poised to grow at a significant CAGR of 4.6% during the forecast period 2023-29. It also includes market size and projection estimations for each of the five major regions from 2023 to 2029. The research report includes historical data, trending features, and market growth estimates for the future. Furthermore, the study includes a global and regional estimation and further split by nations and categories within each region. The research also includes factors and barriers to the Silage additives market growth, as well as their impact on the market's future growth. The report gives a comprehensive overview of both primary and secondary data.
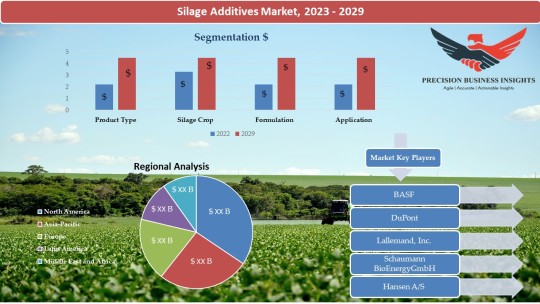
View the detailed report description here - https://www.precisionbusinessinsights.com/market-reports/silage-additives-market ��
The global Silage additives market segmentation:
1) By Product Type : Organic acids, Enzymes, Sugars, Inoculants, Others.
2) By Silage Crop : Alfalfa, Oats, Rye, Barley, Corn, Sorghum, Others.
3) By Formulation : Dry, Liquid.
4) By Application : Inhibition, Stimulation, Moisture Absorption, Others.
The primary factors of the Silage additives market drivers are the increasing focus of governments on feed cost reduction, and boosting animal productivity. The Silage additives market report helps to provide the best results for business enhancement and business growth. It further helps to obtain the reactions of consumers to a novel product or service. It becomes possible for business players to take action for changing perceptions. It uncovers and identifies potential issues of the customers. It becomes easy to obtain the reactions of the customers to a novel product or service. It also enlightens further advancement, so it suits its intended market.
The Silage additives marketresearchreport gives a comprehensive outlook across the region with special emphasis on key regions such as North America, Europe, Asia Pacific, Latin America, and the Middle East and Africa. North America was the largest region in the Silage additives market report, accounting for the highest share in 2022. It was followed by Asia Pacific, and then the other regions. Request sample report at - https://www.precisionbusinessinsights.com/request-sample/?product_id=21129
The important profiles and strategies adopted by Silage additives market key players are BASF SE (Germany) DuPont, Pioneer (DuPont) (U.S.) Lallemand, Inc. (U.S.) Schaumann BioEnergyGmbH (Germany) Hansen A/S (Denmark) Biomin (Austria) Volac International Limited (UK) Micron Bio-Systems (U.S.) American Farm Products (U.S.) Nutreco N.V. (Netherlands) BioZyme, Inc. (U.S.). covered here to help them in strengthening their place in the market.
About Precision Business Insights: We are a market research company that strives to provide the highest quality market research insights. Our diverse market research experts are enthusiastic about market research and therefore produce high-quality research reports. We have over 500 clients with whom we have a good business partnership and capacity to provide in-depth research analysis for more than 30 countries. In addition to deliver more than 150 custom solutions, we already have accounts with the top five medical device manufacturers.
Precision Business Insights offers a variety of cost-effective and customized research services to meet research requirements. We are a leading research service provider because of our extensive database built by our experts and the services we provide.
Contact:
Mr. Satya
Precision Business Insights | Toll Free: +1 866 598 1553
Email: [email protected] Kemp House, 152 – 160 City Road, London EC1V 2NX Web: https://precisionbusinessinsights.com/ | D U N S® Number: 852781747
#silage additives market size#silage additives market share#silage additives market growth#silage additives market analysis#silage additives market trends
0 notes
Text
Innovative Insights in Pharmacy Equipment & Pharmaceutical Solutions
Chemxpert Database provides comprehensive pharmaceutical industry analysis, offering insights into the latest pharmacy equipment and pharmaceutical solutions. Our platform is a valuable resource for method development and supports nutraceutical companies in staying ahead of market trends. Discover unparalleled data and expertise to elevate your business with Chemxpert Database.
#Cosmetics Database#Dyes Chemical Database#Enzyme Products Database#Excipients Database#Fertilizers Database
1 note
·
View note
Text
Significance and improvement in Feed Formulation Technologies
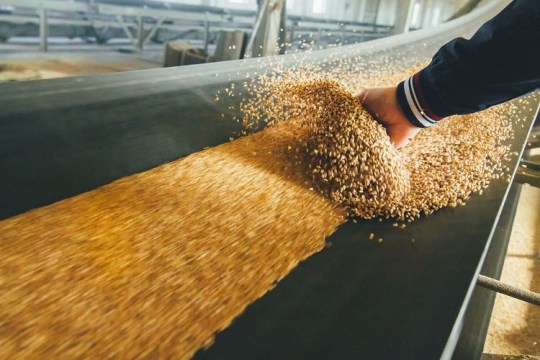
Feed formulation technologies play a crucial role in animal nutrition by providing balanced and cost-effective diets for livestock, poultry, and aquaculture species. These technologies aim to optimize nutrient composition, enhance animal health and performance, reduce environmental impact, and improve overall profitability for farmers.
Here are some significant aspects and improvements in feed formulation technologies in animal nutrition:
Precision Nutrition: Feed formulation technologies have evolved to consider individual animal requirements, allowing for precise nutrient delivery. This approach considers factors such as species, breed, age, weight, production goals, and environmental conditions. By tailoring diets to meet specific nutritional needs, animals can achieve optimal growth, reproduction, and overall health.
Nutrient Balancing: Advanced feed formulation tools enable precise balancing of essential nutrients, including proteins, carbohydrates, fats, vitamins, and minerals. These technologies optimize nutrient ratios to achieve proper growth, muscle development, immune function, and reproductive performance. Balancing diets also helps prevent nutrient deficiencies or excesses that can lead to health issues or inefficient feed utilization.
Ingredient Evaluation and Substitution: Feed formulation technologies allow for thorough analysis and evaluation of various feed ingredients. This includes assessing nutritional content, digestibility, and potential anti-nutritional factors. With access to a wide range of ingredient options, these tools help in finding suitable substitutes to optimize diets while reducing costs and maintaining nutritional value.
Feed Efficiency and Environmental Sustainability: Improved feed formulation technologies focus on enhancing feed efficiency, minimizing waste, and reducing the environmental impact of livestock production. By formulating diets that maximize nutrient utilization and minimize nutrient excretion, these technologies help reduce the environmental footprint associated with animal farming, including greenhouse gas emissions, water pollution, and land use.
Software and Data Integration: Modern feed formulation software and tools leverage computational algorithms and data integration to optimize formulations. They incorporate extensive databases of ingredient composition, nutritional values, and feeding trial results. By utilizing historical data, these technologies can make accurate predictions, adjust formulations, and track performance to continually improve animal diets.
Nutrigenomics and Functional Feed Additives: Emerging fields such as nutrigenomics explore the interaction between diet and gene expression in animals. Feed formulation technologies can incorporate this knowledge to develop diets that promote desirable gene expression patterns, improving traits such as disease resistance, growth, and feed efficiency. Functional feed additives, such as prebiotics, probiotics, enzymes, and phytogenics, are also incorporated into formulations to enhance gut health, nutrient absorption, and immune function.
Optimization of Feed Costs and Availability: Feed formulation technologies consider cost-effective ingredient selection and availability, considering market prices and local availability. They optimize diets to reduce feed costs without compromising animal health or performance, helping farmers maintain profitability even during fluctuations in ingredient prices.
Overall, the significance of feed formulation technologies lies in their ability to provide balanced, customized, and sustainable diets for animals. These advancements contribute to improved animal health, production efficiency, environmental sustainability, and economic viability for the livestock, poultry, and aquaculture industries.
To Know more: https://www.trouwnutritionasiapacific.com/en-in/our-approach/our-products-and-programmes/
Reach out to us on [email protected]
#animal feed#animal nutrition india#animal health#precision#broiler feed#poultry feed#cattle feed#Trouw Nutrition India
0 notes
Text
Dolutegravir Prices | Pricing | Trend | News | Database | Chart | Forecast
Dolutegravir is an antiretroviral medication primarily used in the treatment of HIV/AIDS. As part of the class of drugs known as integrase inhibitors, Dolutegravir works by blocking the action of an enzyme called integrase, which the HIV virus uses to replicate. Since its approval by the U.S. Food and Drug Administration (FDA) in 2013, Dolutegravir has become a crucial component in many HIV treatment regimens. However, one of the most significant concerns around the world, especially in low-income and middle-income countries, has been the pricing of Dolutegravir. Pricing influences access to this essential medication, and efforts are ongoing to make it more affordable for those who need it most.
The cost of Dolutegravir varies widely depending on geographic location, patent laws, and whether the drug is branded or generic. In high-income countries such as the United States, Dolutegravir is often sold under the brand name Tivicay, manufactured by ViiV Healthcare. The brand-name version of Dolutegravir tends to be expensive, with prices in the U.S. being notably higher than in other regions. For example, a month's supply of Tivicay can cost thousands of dollars. This high cost is driven by research and development expenses, regulatory costs, and the need to recoup the investment in bringing a new drug to market. However, the high cost in wealthier nations often makes it difficult for those without adequate health insurance or government assistance to access the medication.
Get Real Time Prices for Dolutegravir : https://www.chemanalyst.com/Pricing-data/dolutegravir-1534
In contrast, many low- and middle-income countries rely on generic versions of Dolutegravir, which are significantly less expensive. Generic Dolutegravir became available after licensing agreements and patent waivers were negotiated between pharmaceutical companies, non-governmental organizations, and international agencies. These agreements allowed for the production of more affordable versions of the drug, making it accessible to larger populations. In countries such as India, which has a robust generic pharmaceutical industry, the price of Dolutegravir can be as low as a few dollars per month. This drastic reduction in price has been crucial in improving access to HIV treatment, particularly in regions that are heavily burdened by the epidemic, such as sub-Saharan Africa.
Global health organizations like the World Health Organization (WHO) and the Joint United Nations Programme on HIV/AIDS (UNAIDS) have played a pivotal role in advocating for lower prices for HIV medications, including Dolutegravir. The Medicines Patent Pool (MPP) is another initiative that has helped to lower the cost of Dolutegravir. By facilitating voluntary licensing agreements, the MPP enables generic drug manufacturers to produce and sell Dolutegravir at a fraction of the price of the branded version. These efforts have resulted in broader availability of the drug, but challenges remain in ensuring that everyone who needs Dolutegravir can afford it.
The introduction of Dolutegravir as part of first-line HIV treatment regimens in many countries has been a game-changer. Not only is Dolutegravir highly effective in suppressing the HIV virus, but it also has a high barrier to resistance, meaning that patients are less likely to develop resistance to the drug over time. Moreover, Dolutegravir has fewer side effects compared to older antiretroviral medications, making it a preferred option for many patients and healthcare providers. Given its effectiveness, there has been a global push to make Dolutegravir the standard of care in HIV treatment. However, pricing remains a barrier in some parts of the world, where even the generic versions may be out of reach for the most vulnerable populations.
Efforts to reduce the price of Dolutegravir are ongoing, with various international partnerships and coalitions working to negotiate lower costs. For example, the Global Fund to Fight AIDS, Tuberculosis and Malaria has been instrumental in securing lower prices for Dolutegravir through bulk purchasing agreements. These agreements allow for large quantities of the drug to be purchased at a reduced cost, which can then be distributed to countries in need. Additionally, some countries have implemented pricing controls or subsidies to make Dolutegravir more affordable for their populations. These strategies have been successful to varying degrees, depending on the political and economic landscape of each country.
Another factor influencing the price of Dolutegravir is the expiration of patents. As patents on the drug begin to expire in more countries, the opportunity for increased competition among generic manufacturers grows. This competition is expected to drive prices down even further, making Dolutegravir more accessible to a wider range of people. However, patent expirations are staggered across different regions, meaning that some countries may experience price reductions sooner than others. In countries where the patent is still in effect, advocacy efforts are focused on encouraging pharmaceutical companies to voluntarily lower their prices or enter into more licensing agreements that would allow for the production of generics.
In recent years, there has been a growing emphasis on the importance of affordable HIV treatment as part of global health initiatives. The United Nations has set ambitious goals for reducing the number of new HIV infections and ensuring that those living with HIV have access to treatment. Dolutegravir is central to these efforts, but its price remains a critical issue. Without continued pressure on pharmaceutical companies and governments, there is a risk that some populations will continue to be left behind in the fight against HIV/AIDS.
In conclusion, the pricing of Dolutegravir reflects a complex interplay of factors, including patent laws, production costs, and global health policies. While progress has been made in making the drug more affordable, particularly in low- and middle-income countries, there is still work to be done to ensure that everyone who needs Dolutegravir can access it. The future of HIV treatment depends not only on the development of new and effective medications but also on the ability to make these treatments affordable and accessible to all. Through continued international cooperation and advocacy, it is possible to further reduce the price of Dolutegravir and ensure that it reaches those who need it most.
Get Real Time Prices for Dolutegravir : https://www.chemanalyst.com/Pricing-data/dolutegravir-1534
Contact Us:
ChemAnalyst
GmbH - S-01, 2.floor, Subbelrather Straße,
15a Cologne, 50823, Germany
Call: +49-221-6505-8833
Email: [email protected]
Website: https://www.chemanalyst.com
#Dolutegravir#Dolutegravir Price#Dolutegravir Prices#Dolutegravir News#Dolutegravir Market#Dolutegravir Pricing
0 notes
Text
High Protein Flour Market Highlights On Evolution by 2021-2031 | North American Millers’ Association, Sresta Natural Bioproducts Pvt. Ltd, E H L Ltd
Global High Protein Flour Market report from Global Insight Services is the single authoritative source of intelligence on High Protein Flour Market. The report will provide you with analysis of impact of latest market disruptions such as Russia-Ukraine war and Covid-19 on the market. Report provides qualitative analysis of the market using various frameworks such as Porters’ and PESTLE analysis. Report includes in-depth segmentation and market size data by categories, product types, applications, and geographies. Report also includes comprehensive analysis of key issues, trends and drivers, restraints and challenges, competitive landscape, as well as recent events such as M&A activities in the market.
Get Access to A Free Sample Copy of Our Latest Report – https://www.globalinsightservices.com/request-sample/GIS23682
High protein flour is a type of flour that has a higher protein content than regular flour. Protein is an essential nutrient that helps the body build and repair tissues, and it is also necessary for the production of enzymes and hormones. High protein flour can be made from a variety of grains, but wheat is the most common type of grain used. The protein content of wheat flour varies depending on the type of wheat used, but it is typically around 11-12%.
Key Trends
There are several key trends in high protein flour technology.
One is the development of new and improved strains of wheat. This has led to the development of wheat varieties with higher protein content and improved baking qualities.
Another trend is the use of new processing techniques, such as the use of enzymes, to improve the quality of high protein flour.
Finally, there has been a trend toward the use of natural ingredients, such as soy, to improve the nutritional quality of high-protein flour.
Key Drivers
The key drivers of the high protein flour market are the growing demand for healthy and nutritious food, the rising trend of veganism, and the increasing popularity of low-carbohydrate diets.
High protein flour is a healthy and nutritious alternative to regular flour, and it is also suitable for people who are following a vegan or low-carbohydrate diet.
Get Customized Report as Per Your Requirement – https://www.globalinsightservices.com/request-customization/GIS23682
Market Segments
The high protein flour market is segmented by type, source, end-user, and region. By type, the market is classified into unbleached, and bleached. Based on the source, it is bifurcated into wheat, and almond. On the basis of the end-user, it is divided into food, beverages, bakery, and others. Region-wise, the market is segmented into North America, Europe, Asia Pacific, and the Rest of the World.
Key Players
The global high protein flour market includes players such as Archer Daniels Midland Company, Unilever Inc., ITC Ltd., Conagra Brands Inc., The Pillsbury Company LLC, General Mills, King Arthur Flour Company Inc, North American Millers’ Association, Sresta Natural Bioproducts Pvt. Ltd, E H L Ltd., and others.
Buy Now - https://www.globalinsightservices.com/checkout/single_user/GIS23682
With Global Insight Services, you receive:
· 10-year forecast to help you make strategic decisions
· In-depth segmentation which can be customized as per your requirements
· Free consultation with lead analyst of the report
· Excel data pack included with all report purchases
· Robust and transparent research methodology
Ground breaking research and market player-centric solutions for the upcoming decade according to the present market scenario
New Report Published by Global Insight Services: https://www.globalinsightservices.com/reports/hydrogen-projects-database/
About Global Insight Services:
Global Insight Services (GIS) is a leading multi-industry market research firm headquartered in Delaware, US. We are committed to providing our clients with highest quality data, analysis, and tools to meet all their market research needs. With GIS, you can be assured of the quality of the deliverables, robust & transparent research methodology, and superior service.
Contact Us:
Global Insight Services LLC 16192, Coastal Highway, Lewes DE 19958 E-mail: [email protected] Phone: +1–833–761–1700 Website: https://www.globalinsightservices.com/
0 notes
Text
Molecular Enzymology and Drug Targets
Molecular Enzymology and Drug Targets journal aims is to publish the work of designing and synthesis of enzymes and high unmet medical need are based on innovative drug targets. This is an international journal for rapid dissemination of significant data related to enzymes and their use in biotechnology and drug discovery.
The journal is an interdisciplinary medium serving several branches of life sciences. This Journals publishes original articles, short communications, notes, and mini reviews. Full length reviews are only published after invitation from the editorial board. Preliminary studies are inappropriate for publishing in Journal of Molecular enzymology and Drug Targets, unless the authors report the significance of their findings, in that case, the manuscript can be accepted. Average first decision of submitted manuscript is expected to be 14 days.
Submit manuscript as an e-mail attachment to the Editorial Office at [email protected]
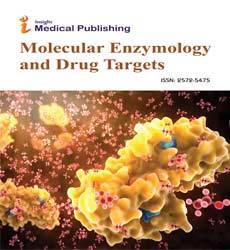
0 notes
Text
I have my first assessment on Tuesday. A piece of coursework I have two weeks to complete.
I have to find a gene on a genetic database, identify and remove the non-coding regions, write a set of primers for cloning with restriction enzyme sequences and a fluorescent tag, design another set of primers for qualitative PCR to detect the difference between gDNA and cDNA, and write a method (lab instructions) of inserting the gene sequence into a plasmid vector, propagating it in transformed E.coli cells, screening the cells for the transformed plasmid, detecting the presence of the gene insert in e.coli colonies (hence the qualitative PCR primers) and purifying the gene product from the bacteria so it can be used, and naming the various applications it can be used in.
My lecturer made an insane PowerPoint with a practice gene and went through every step with various annotations. So I do feel that I know what I'm doing (more or less). For example, in my textbook it had quite a convoluted method to resolve ORFs, involving a database I don't know how to use, while we're just looking at the gene annotations and cutting out the exons manually nucleotide by nucleotide like 12th century monks, bypassing the ORF issue. So it's not that bad. Maybe
8 notes
·
View notes