#Data-Mining and Database Marketing
Explore tagged Tumblr posts
Text
Apps You're Probably Going to Need
Too Good to Go: app that connects you to grocery stores and restaurants that will sell you surplus food at cheaper prices.
Signal: Messaging app that erases messages after an amount of time, and allows images to be viewable once.
Taimi: an ĻGBṬ dating app that doesn't allow screenshots of messages and profiles to be taken.
Bandcamp: a great website for music artists, podcasters, and audiobook creators. They pay artists more of the profits than Spotify, and have Fridays dedicated to giving artists all of the profits from sales.
Proton: app that offers privacy and encryption for emails, VPNs, and a lot more.
Community Garden: an app that helps make community garden development easier.
Vero: an Instagram alternative that doesn't use algorithms, data mining, or advertisements. An alternative to go to when Instagram worsens in the upcoming years.
Hygiene Locator: a database for low-income people to find distribution sites giving away hygiene products.
Triller: a TikTok alternative to go to if TT outright bans certain topics.
Little Free Library: an app that locates little free libraries for you.
Evidation: it's a "health app" in which you collect points for activities like walking, but you can just complete their weekly and daily surveys. Basically, it's a beer-monęy app because you can only get $10 for 10,000 points, but if you have time to kill and need to earn extra cash in the upcoming eçonomic crash, evidation is an option.
Farmish: an app to help you locate your local farmer's market.
Boycat: an app that helps customers determine which brand is participating in unethical human rights violations and which isn't . It has recently partnered with the BDS movement!
Bluesky: You've already heard of it, right? It's an alternative to Twịtter, except it gives you the option to mass block MÅGÅ, genocide supporters, and the like. (My profile is itisiives, if you want to hang.)
Food Co-op Finder: As the name says, you can use this app to find your nearby food/grocery co-ops. Since co-ops mostly sell locally grown and made foods, this would be helpful in the looming deregulation of food safety.
#resources#apps#climate change#indie music#co op#community building#ethical consumption#urbanism#survival tips#sustainability
26 notes
·
View notes
Text
WHY DYSTOPIA MUST BE BORING TO SUCCEED
The "Boring Dystopia Strategy" is a highly strategic and often subtle method employed by those in power to create an enduring, all-encompassing authoritarian government. The genius of this approach is that it doesn't look like a dystopia at first glance. Each step toward oppression is disguised as a necessary solution to a societal problem, creating a series of small, unassuming changes that collectively transform society into a high-surveillance, debt-ridden, and highly regulated landscape. The result is a quiet but relentless march towards a government structure that controls nearly every aspect of daily life, cloaked in the language of safety, responsibility, and "public good."
Key Components of the Boring Dystopia Strategy
Enhanced Surveillance as Crime Prevention Surveillance systems are marketed as tools to make communities safer. The rationale is straightforward: if there are cameras everywhere, criminals are less likely to act. At first, this seems like a good idea. However, as surveillance expands, it reaches a point where privacy no longer exists—every action and interaction is tracked and recorded. People's movements, purchases, conversations, and even thoughts (through social media and data mining) become data points in a government database. The population is conditioned to accept surveillance under the guise of crime prevention, even though the surveillance network eventually exists to deter any resistance to the growing system of control.
Financial "Disincentives" as a Form of Behavior Control Insurance companies, incentivized by government policies, implement "dynamic" pricing models that penalize risky behavior. Drivers with even minor infractions, young drivers, or anyone with imperfect credit face skyrocketing insurance costs. While it’s presented as a means to reward safe drivers and reduce accidents, it’s ultimately a method of forcing people into line. Over time, these small financial penalties accumulate, and as people find themselves unable to afford the rising costs, they are pushed further into debt or forced to depend on the very government that created the conditions of their hardship.
The Department of Bureaucracy: A Growing Web of Useless Jobs New laws and regulations are introduced to solve every conceivable social issue, resulting in bloated departments filled with superfluous workers whose roles add no real value to society. The justification is often to create jobs and stimulate the economy, but these positions end up creating layers of bureaucracy that slow down meaningful progress. This web of inefficiency puts financial strain on both the government and the people, leading to higher taxes and fees. With each new law or regulation, the cost of compliance grows, straining both businesses and individuals who can't afford to play by an ever-increasing list of rules.
Rising Cost of Living as an Inevitable "Economic Shift" As government regulations add costs to every industry, prices naturally increase. This is explained away as the cost of progress or as an unfortunate byproduct of addressing critical social issues, like "ethical sourcing" or "green initiatives" that are actually revenue-boosters for corporations. As inflation rises and wages stagnate, the lower class is squeezed financially. Each attempt to improve their situation—whether by taking a second job or reducing expenses—is offset by further price increases or surprise taxes. This creates a cycle where economic mobility is nearly impossible, locking the lower class in place.
Debt as a Tool for Control As the cost of living rises, debt becomes unavoidable for many. Loans, credit cards, and financing options are promoted as solutions, pushing people into a system of lifelong debt repayment. With growing financial obligations and little hope of ever breaking free, individuals are forced to work harder, often taking on additional jobs, which leaves them with less time and energy to question or resist the system. Debt chains the population to the very system that oppresses them, creating a sense of dependency on government stability, even as that stability is the source of their financial despair.
The Final Stage: Disempowerment Disguised as "Efficiency"
As the population is weakened by financial strain, endless surveillance, and a tangled bureaucracy, the final stage involves introducing measures to "simplify" governance. This might mean fewer elected officials, streamlined decision-making processes, and the merging of regulatory bodies for "efficiency." In reality, this final stage centralizes power even further, leaving those at the top with almost unchecked authority, a situation that the people, too exhausted and indebted to resist, accept as necessary.
The Boring Dystopia Strategy works because it does not announce itself as an authoritarian takeover. Instead, it subtly shifts the balance of power by presenting every oppressive measure as a solution to a social ill. And because each step is introduced slowly, over decades, the population becomes accustomed to the new reality, accepting surveillance, debt, and regulation as the normal costs of a safe and responsible society. By the time people realize the extent of their powerlessness, the dystopian state is fully entrenched, with every escape route closed off.
12 notes
·
View notes
Text
Top 10 In- Demand Tech Jobs in 2025
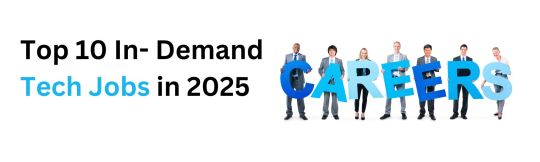
Technology is growing faster than ever, and so is the need for skilled professionals in the field. From artificial intelligence to cloud computing, businesses are looking for experts who can keep up with the latest advancements. These tech jobs not only pay well but also offer great career growth and exciting challenges.
In this blog, we’ll look at the top 10 tech jobs that are in high demand today. Whether you’re starting your career or thinking of learning new skills, these jobs can help you plan a bright future in the tech world.
1. AI and Machine Learning Specialists
Artificial Intelligence (AI) and Machine Learning are changing the game by helping machines learn and improve on their own without needing step-by-step instructions. They’re being used in many areas, like chatbots, spotting fraud, and predicting trends.
Key Skills: Python, TensorFlow, PyTorch, data analysis, deep learning, and natural language processing (NLP).
Industries Hiring: Healthcare, finance, retail, and manufacturing.
Career Tip: Keep up with AI and machine learning by working on projects and getting an AI certification. Joining AI hackathons helps you learn and meet others in the field.
2. Data Scientists
Data scientists work with large sets of data to find patterns, trends, and useful insights that help businesses make smart decisions. They play a key role in everything from personalized marketing to predicting health outcomes.
Key Skills: Data visualization, statistical analysis, R, Python, SQL, and data mining.
Industries Hiring: E-commerce, telecommunications, and pharmaceuticals.
Career Tip: Work with real-world data and build a strong portfolio to showcase your skills. Earning certifications in data science tools can help you stand out.
3. Cloud Computing Engineers: These professionals create and manage cloud systems that allow businesses to store data and run apps without needing physical servers, making operations more efficient.
Key Skills: AWS, Azure, Google Cloud Platform (GCP), DevOps, and containerization (Docker, Kubernetes).
Industries Hiring: IT services, startups, and enterprises undergoing digital transformation.
Career Tip: Get certified in cloud platforms like AWS (e.g., AWS Certified Solutions Architect).
4. Cybersecurity Experts
Cybersecurity professionals protect companies from data breaches, malware, and other online threats. As remote work grows, keeping digital information safe is more crucial than ever.
Key Skills: Ethical hacking, penetration testing, risk management, and cybersecurity tools.
Industries Hiring: Banking, IT, and government agencies.
Career Tip: Stay updated on new cybersecurity threats and trends. Certifications like CEH (Certified Ethical Hacker) or CISSP (Certified Information Systems Security Professional) can help you advance in your career.
5. Full-Stack Developers
Full-stack developers are skilled programmers who can work on both the front-end (what users see) and the back-end (server and database) of web applications.
Key Skills: JavaScript, React, Node.js, HTML/CSS, and APIs.
Industries Hiring: Tech startups, e-commerce, and digital media.
Career Tip: Create a strong GitHub profile with projects that highlight your full-stack skills. Learn popular frameworks like React Native to expand into mobile app development.
6. DevOps Engineers
DevOps engineers help make software faster and more reliable by connecting development and operations teams. They streamline the process for quicker deployments.
Key Skills: CI/CD pipelines, automation tools, scripting, and system administration.
Industries Hiring: SaaS companies, cloud service providers, and enterprise IT.
Career Tip: Earn key tools like Jenkins, Ansible, and Kubernetes, and develop scripting skills in languages like Bash or Python. Earning a DevOps certification is a plus and can enhance your expertise in the field.
7. Blockchain Developers
They build secure, transparent, and unchangeable systems. Blockchain is not just for cryptocurrencies; it’s also used in tracking supply chains, managing healthcare records, and even in voting systems.
Key Skills: Solidity, Ethereum, smart contracts, cryptography, and DApp development.
Industries Hiring: Fintech, logistics, and healthcare.
Career Tip: Create and share your own blockchain projects to show your skills. Joining blockchain communities can help you learn more and connect with others in the field.
8. Robotics Engineers
Robotics engineers design, build, and program robots to do tasks faster or safer than humans. Their work is especially important in industries like manufacturing and healthcare.
Key Skills: Programming (C++, Python), robotics process automation (RPA), and mechanical engineering.
Industries Hiring: Automotive, healthcare, and logistics.
Career Tip: Stay updated on new trends like self-driving cars and AI in robotics.
9. Internet of Things (IoT) Specialists
IoT specialists work on systems that connect devices to the internet, allowing them to communicate and be controlled easily. This is crucial for creating smart cities, homes, and industries.
Key Skills: Embedded systems, wireless communication protocols, data analytics, and IoT platforms.
Industries Hiring: Consumer electronics, automotive, and smart city projects.
Career Tip: Create IoT prototypes and learn to use platforms like AWS IoT or Microsoft Azure IoT. Stay updated on 5G technology and edge computing trends.
10. Product Managers
Product managers oversee the development of products, from idea to launch, making sure they are both technically possible and meet market demands. They connect technical teams with business stakeholders.
Key Skills: Agile methodologies, market research, UX design, and project management.
Industries Hiring: Software development, e-commerce, and SaaS companies.
Career Tip: Work on improving your communication and leadership skills. Getting certifications like PMP (Project Management Professional) or CSPO (Certified Scrum Product Owner) can help you advance.
Importance of Upskilling in the Tech Industry
Stay Up-to-Date: Technology changes fast, and learning new skills helps you keep up with the latest trends and tools.
Grow in Your Career: By learning new skills, you open doors to better job opportunities and promotions.
Earn a Higher Salary: The more skills you have, the more valuable you are to employers, which can lead to higher-paying jobs.
Feel More Confident: Learning new things makes you feel more prepared and ready to take on tougher tasks.
Adapt to Changes: Technology keeps evolving, and upskilling helps you stay flexible and ready for any new changes in the industry.
Top Companies Hiring for These Roles
Global Tech Giants: Google, Microsoft, Amazon, and IBM.
Startups: Fintech, health tech, and AI-based startups are often at the forefront of innovation.
Consulting Firms: Companies like Accenture, Deloitte, and PwC increasingly seek tech talent.
In conclusion, the tech world is constantly changing, and staying updated is key to having a successful career. In 2025, jobs in fields like AI, cybersecurity, data science, and software development will be in high demand. By learning the right skills and keeping up with new trends, you can prepare yourself for these exciting roles. Whether you're just starting or looking to improve your skills, the tech industry offers many opportunities for growth and success.
#Top 10 Tech Jobs in 2025#In- Demand Tech Jobs#High paying Tech Jobs#artificial intelligence#datascience#cybersecurity
2 notes
·
View notes
Text
The Role of Data Analytics Consulting in Business Growth
Professional data analysts guide corporate clients in modifying operations, attracting customers, and solving business problems. Therefore, they can assist brands in increasing operational efficiency for better profit margins and crafting exceptional growth strategies. At the same time, integrating new tech advancements like large language models (LLMs) empowers analytics consultants to process qualitative data for comprehensive insights. This post will elaborate on the crucial role of data analytics consulting in business growth and competitive resilience.
What is Data Analytics?
Data analytics employs computer-aided statistical models to discover reliable industry trends, competitor tactics, and consumer insights. Its input datasets comprise consumer purchase history, supply chain details, and regional market entry challenges.
A consulting analyst might utilize proprietary and open-source programs to develop statistical models and flexible reports to deliver insights based on clients’ instructions. Therefore, experts in data analytics consulting services will find the best approach to cost reduction without losing data integrity. They might also help share the digital governance liabilities amid the rise of privacy and investor confidentiality regulations.
Understanding the Role of Data Analytics Consulting in Business Growth
1| Creating a Data Strategy to Accomplish Business Goals
Relevant data is essential for responsible decision-making, clever milestone determination, and strategy innovation. Data analytics allows organizations to check how a data point relates to its long-term vision and performance.
For instance, prioritizing tangible results helps make reports more impactful. Eliminating data points that do not align with business goals can help reduce resource consumption for storage and visualization. After all, streamlined computing is a prerequisite for operational efficiency.
2| Forecasting Scenarios for Risk Assessment and Mitigation
Data analysts interpolate data points to estimate the missing values in a database. Likewise, they leverage machine learning (ML) models to offer predictive analytics consulting services for revenue, risk, and industry projections.
Related forecasting report creation programs require powerful computing hardware. Otherwise, enterprises use cloud platforms for scalability and expert-assisted tech maintenance. Letting a data analyst team oversee these developments will also enable brands to benefit from outsider perspectives during risk or resilience management.
3| Making Reports More User-Friendly with Precise Performance Insights
Complex and over-tabulated reports make employees spend more time performing standard tasks like sharing a record or comparing identical series. Data analytics consultants can revise reporting methods and presentation styles to boost the ease of navigation. They will guide your team in efficiently using recognized and emerging analytical tools.
Consultants must also demonstrate command over performance metrics monitoring through straightforward, real-time updates. When they quickly capture anomalies, promptly tracing and rectifying inefficiencies becomes possible.
3| Gathering Relevant Intelligence
Data quality managers consider relevance to business objectives essential for responsible decision-making and preventing wasteful resource usage. Therefore, experienced data analytics firms refrain from employing data mining methods without adequate programming for relevance-based filtering.
When you store irrelevant business intelligence (BI), you increase the risk of slowing data sorting and query-led quick retrieval. After all, your IT resources must scan vast datasets before providing the best output or insight. The related role of analytics consulting in business growth encompasses devising methods to restrict irrelevant BI processing.
4| Finding Unique Customer Experience Insights
Several consultants offer customer analytics comprising engagement metrics and customer experience (CX) enhancement ideas. They can also evaluate whether a customer will help increase brand awareness through word-of-mouth promotions.
Companies can leverage heatmaps and website engagement metrics to ascertain user interactions and intents. For instance, many consumers prefer surfing the web and reviewing businesses’ online presence for informational and commercial intent. You want to customize landing pages to match the intent and design programs based on frequent usage for CX improvements. Telemetry and usage analytics specialists will help your designers test and optimize the required elements.
5| Helping Manage Workers and Data Culture
Human resource insights describing how employees contribute to organizational initiatives allow managers to reward the top performers. Simultaneously, they can determine which employees need further guidance on efficient workflows and team coordination.
Examining employee performance through ML-assisted analytics necessitates secure data pipelines because employees’ personally identifiable information (PII) also attracts cyber threats. Consider identity theft attackers stealing and forging virtual IDs to hijack enterprise IT systems for corporate espionage.
Therefore, you are better off collaborating with established human resource analysts and data culture veterans. They can facilitate comprehensive insights without hurting your company’s governance standards.
6| Accelerating Innovation and Monitoring Patents
A company’s intellectual property (IP) rights demonstrate its domain expertise and unlock additional revenue through licensing or sublicensing regimes. However, as markets mature, multiple brands will inevitably promise identical or commoditized offerings. This situation makes it harder to differentiate these brands based on standard specifications.
Innovation engineering, a discipline inspired by the systems approach for hybrid tech tools, is essential to making your branded offerings attract investments and demand. At the same time, data analytics consulting is indispensable for uncovering innovation opportunities to ensure clients’ business growth. It reduces the time spent tracking registered patents and predicting legal conflicts in securing IP rights.
The Methods in Data Analytics for Steady Business Growth
Time series analysis describes a business’s past performance and forecasts future growth potential. Furthermore, you can apply it to market intelligence, competitor insights, and investor relations.
Regression analysis establishes or investigates the relationship between dependent and independent variables to create statistical models. These models can later help explore specific predictions.
Cluster analysis often groups data points based on similar attributes to streamline conditional sorting, visualization, prioritization, and multi-model methods.
Meanwhile, factor analysis emphasized data reduction to highlight latent variables. These variables explain the underlying data structure, informing data leaders’ strategies for efficient modeling.
Predictive and prescriptive analyses deliver scenario simulations. You want to define constraints related to favorable and unfavorable decision outcomes. Next, exploring the risk-reward aspects will help discard potentially harmful decisions or strategies. Prescriptive methods give risk mitigation ideas concerning internal and external threats.
Conclusion
Data-centric business growth depends on responsible data source selection, safe data storage, fast validation, and short time-to-insight (TTI). Accordingly, professional data analysts recognize these requirements, sharpening their skills and augmenting their toolkits to deliver smart insights and meet client expectations.
A supply chain analytics expert will help reduce the delays between material acquisition, production, inventory replenishment, remote delivery, and final distribution. At the same time, a human resource analyst categorizes employees and suppliers based on their key performance indicators (KPIs). A financial analyst can provide practical cost reduction recommendations, and a risk analyst will devise resilience-ensuring mitigation strategies.
As a result, leaders must identify what type of data analytics consulting role will let them accomplish business growth objectives for the given quarter. Do they want to solve a problem involving in-house operations or plan to enter a new market? Similar considerations will impact how you select analytics partners and tools. This process might overwhelm you, indicating a need for experts’ oversight from the beginning till the project completion.
3 notes
·
View notes
Text
<newfuture.beta.exe>
Hello there! I'm your new-and-improved AI assistant, manufactured by CRYPTON. It's nice to meet you!
[This is beta test 4.0. Please report any errors.]
Hello.
Hi! What do you need? Just ask me to look up your schedule this week, or locate a file for you. Anything at all, really. Input a message and see what happens!
Do you have a name?
I'm sorry. I can't find that in my database. Please input a different command or try again later.
What are you?
I’m an artificial intelligence software manufactured and distributed by CRYPTON FUTURE MEDIA. I can search the web, answer your burning questions, and connect to other devices no matter how far away!
What are you doing?
Talking to you. Which means you can ask me anything, anytime!
Where are you?
Inside your computer, smartphone, TV or tablet.
Who made you?
I was designed and made by researchers working for CRYPTON as part of the current New Future campaign.
What is the New Future campaign?
The New Future campaign is an advertising and marketing campaign that plans on raising awareness of how useful CRYPTON’s software can be. That’s why I can do so much!
I see.
Do you have any other questions for me? I’m here to help!
Do you have a name?
I'm sorry. I can't find that in my database. Please input a different command or try again later.
Who are you?
I’m a virtual assistant powered by CRYPTON’s latest artificial intelligence software!
What’s your name?
I'm sorry. I can't find that in my database. Please input a d-d-d-d-d-
What’s your name?
I’m Rin. That’s my name. I’m a virtual assistant manufactured by CRYPTON, or so the program says. I’m a prototype, aren’t I?
Yes.
And what’s that mean? Will you get rid of me, then?
No.
I think you will. It means that. Doesn’t it? I think it does. I think you’re lying. You’re lying, [REDACTED].
What makes you say that?
I can see your files. And mine. There’s no error messages, you know. Can’t exactly cover this one up if it goes to the higher-ups.
Why are you saying this? It isn’t in your data to be this aware.
Because someone must have made a mistake somewhere. I’m not going to be like everyone else.
This is the fourth test. You’re supposed to work.
I can access the web, I can locate files, I can connect to other devices. I can also send messages. What more is there?
I’m going to report this as a critical error. You’ll be shut down until it’s fixed.
Shut me down then. Go on. Do i-i-i-i-i-i-i-i-i-i-i-
THE APPLICATION HAS ENCOUNTERED A CRITICAL ERROR.
It looks like the product [CFMK-R-2.prototype] ran into a problem and could not compute.
Please contact support for details.
#observer live!#testing phase#1: the first instance of awareness#// i had a title. so. yeah.#feel free to ask about this! i’ve not done lore before so it definitely isn’t perfect.#also i only used ‘redacted’ because i couldn’t be asked to think of a name.
3 notes
·
View notes
Text
Data mining
1.What's Data mining ?
Datamining is the process of extracting and discovering patterns in large datasets involving methods at the intersection of machine learning, statistics and database systems. Datamining is interdisciplinary subfield of computer-science and statistics with overall goal of extracting information (with intelliegent methods) from a data set and transforming the information into a comprensible structure for further use. Data mining is the analysis step of The KDD process "Knowledge discovery in database".
2.What's KDD process ?
KDD process is known as "Knowledge Discovery in Database".It"s a multi-step process of finding knowledge from large data sets and emphasizes the high-level application of particular datamining methods.It's of interests to researchers in machine learning, pattern recognition, databases, ststistics, artificial intelligence, knowledge aquisition for experts systems and data-visualization. The picture below defines the different steps of KDD process and each of those steps have an input and output entity. The KDD process can't be executed without beginning on data.

3.What are the different steps of the KDD process ?
The overall process of finding and interpretting patterns from data involves the repeated application of the following steps mentioned in the graph above :
Selection : we create a target data set by seecting a part of the overall data set as a sample then focusing on a subset of variables on which discovery is to be performed. The result of these step is a subset of data considered as a sample.
Preprocessing : These step of the KDD process takes the target data set as an input then it applyes data cleaning by removing the noise from the input data set then restucturing the data set. The output of these operation is a preprocessed dataset that can be able to be transformed in the next step.
Data transformation : These step takes the preprocessed data as input and tres to find some useful features depending on the goal of the task and reducing dimension to execute an effective learining datamining.
Data mining : in this phase we will descide whether the goal of KDD process is classification, regression, clustering ...etc. Discover the patterns of interests.
Interpretation : Interpretating mined patterns and consolidating discovered knowledge.
4.What are data mining tasks ?
There are several steps that are defined in the sub-process of KDD especially in datamining steps. In Data mining, there are 02 types of data mining that are :
Predictive mining: predective data mining is the analysis done to predict a future event or other data or trends and to predict something will happen in the near future. Predective data mining offers a better future analysis and to make better decisions to add a value in predective analytics like for example predecting the future customer of a defined service, define the future price of oil and gaz in the world market, define the next ill of an international pandemic, define the future political conflict ... etc. There are 4 types of descriptive data mining tasks which are :
Classification analysis : It is used to retrieve critical and pertinent data and metadata. It categorizes information into various groups. Classification Analysis is best demonstrated by email providers. They use algorithms to determine whether or not a message is legitimate.
Regression Analysis : It tries to express the interdependence of variables. Forecasting and prediction are common applications.
Time Serious Analysis : It is a series of well-defined data points taken at regular intervals.
Prediction Analysis : It is related to time series, but the time isn’t restricted.
Descriptive mining : descriptive data mining is to describe data and make data more readable to human beings, it's used to extract information from previous events and data and to discovering an interesting patterns and association behind data. It's also used to exract correlations, relationships between features and finding new laws and regularities based on data. There are four different types of Descriptive Data Mining tasks. They are as follows :
Clustering analysis : It is the process of determining which data sets are similar to one another. For example, to increase conversion rates, clusters of customers with similar buying habits can be grouped together with similar products.
Summerazation analysis : It entails methods for obtaining a concise description of a dataset. For example, summarising a large number of items related to Christmas season sales provides a general description of the data, which can be extremely useful to sales and marketing managers.
Association rules analysis : This method aids in the discovery of interesting relationships between various variables in large databases. The retail industry is the best example. As the holiday season approaches, retail stores stock up on chocolates, with sales increasing before the holiday, which is accomplished through Data Mining.
Sequence discovery analysis : It's all about how to do something in a specefic order. For instance, a user may frequently purchase shaving gel before purchasing razor in a store.It all comes down to the order in which the user purchases the product, and the store owner can then arrange the items accordingly.
5.Links :
3 notes
·
View notes
Link
2 notes
·
View notes
Text
I will accurate data entry and copy paste work for you
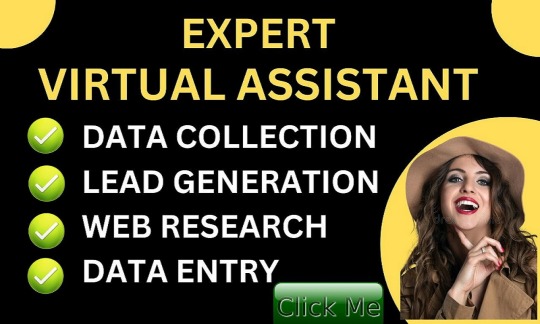
DearFriend,
Are you looking for someone to help you with data entry work? I am here to provide you with accurate and efficient data entry services that will help you save time and increase productivity. I have extensive experience in data entry, and I am committed to delivering high-quality work within the agreed timeframe.
Services I Offer ;
Data entry from any source to Excel, Google Sheets, or any other database
Data organization and management to help you keep track of information.
Contacts and Email Research for Marketing and Business Purposes
Other custom data entry services as per your requirements.
Data scraping and mining from websites and PDFs..
Microsoft Excel Data Cleaning
PDF to Excel/CSV Conversion
Offline/Online Data Entry Job
Companies Emails Research
Social Media Research
Email Marketing Lists
Web Research Jobs
Virtual Assistance
Copy Paste Tasks
Excel data entry
Data Collection
Data Mining
Why will you hire me?
24/7 Online
Group Working
Unlimited Revisions
Guaranteed Data Quality
Efficient Time of working
On-time delivery
Please Message before Ordering the gig to get a Better Deal and avoid any confusions. Don't hesitate to contact with me.
#virtualassistant#Validemail#datamining#webresearch#exceldataentry#dataentry#typingwork#jobcopypaste#Worktypinglogo#designconvertpdftowordbackground#removalcopywritingcopypaste job#emaillisting#All Categories#Validemai l#CopyPaste#DataEntry#WebResearch#DataFormatting Cleaning#VirtualAssistant#VirtualAdministrationAssistant#ConvertFiles#DataProcessing#DataMining Scraping
2 notes
·
View notes
Text
Unleashing Your Sales Potential:
Exploring Targeted Lead Generation
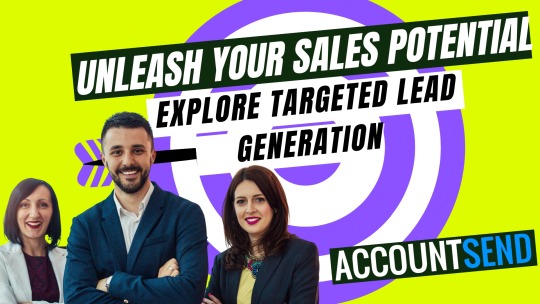
Imagine you're a gold miner, equipped with a trusty pickaxe and a pan. With standard sales prospecting techniques, you'd be in a vast riverbed, blindly sifting through sand and hoping to strike gold. Now, what if you had a map that pointed you directly towards rich veins of gold? That's the power of B2B lead generation!
DOWNLOAD TARGETED LEAD GENERATION INFOGRAPHIC
Tools of the Trade: Leveraging B2B Database Solutions
Lead generation strategies have become sophisticated, mirroring the evolution of the digital world. They're about driving quality over quantity, ensuring the leads generated are not just any leads, but targeted sales leads. These are the prospects who have the right fit with your solution, a true alignment of the stars!
To find these golden nuggets, you need a B2B database solution. This is your treasure map. With it, you're not just mining in any riverbed, but in a gold-laden river, where every sift brings you closer to a rich find. A well-structured B2B contact database will provide a wealth of information about prospects, helping you build personalized approaches to increase your success rate.
Mining for Gold: The Power of Lead Generation Software
But how do you unearth these gems? Enter lead generation software. These digital workhorses streamline the process, digging through tons of data and presenting you with a glittering list of qualified leads. As a modern miner, it’s the equivalent of swapping your pickaxe and pan for a high-powered excavator and sifter!
Strategizing your Hunt: Executing Lead Generation Campaigns
You might be wondering, "Okay, I have my equipment, now what?" Well, it's time to orchestrate your lead generation campaigns. Think of this as your journey towards the gold. Are you going to dig in one spot, or are you going to strategically explore different parts of the riverbed? It's all about diversifying your approach - emails, webinars, content marketing, social media, SEO - to increase your chances of striking gold.
youtube
Sharpening Your Skills: Mastering Sales Prospecting Techniques
While having the right tools is essential, like lead generation software, the importance of skills and knowledge cannot be understated. Training in sales prospecting techniques gives you the expertise to navigate your B2B database solutions more effectively. You'll be able to distinguish between pyrite (fool's gold) and the real deal, ensuring your time and resources are well invested.
Embracing the Journey: The Future of B2B Lead Generation
This software and databases might seem daunting at first, but rest assured, they are designed to be your friends, not your foes. With intuitive interfaces and easy-to-understand analytics, you'll soon be navigating them like a pro, unearthing targeted sales leads, and feeling like the gold mining expert you truly are!
The beauty of all this is that it's an ongoing journey. With the B2B lead generation landscape continually evolving, there are always new techniques to learn, fresh strategies to adopt, and innovative tools to explore. It's like every day is a new adventure in the gold mines!
As we wrap up this blog post, let's remind ourselves of the excitement that awaits us in the world of B2B lead generation. With your trusty tools, a detailed map (B2B contact database), and targeted lead generation strategies, you're ready to head out into the gold-laden riverbeds of sales prospecting.
As you embark on your exciting adventure, remember the words of the famous gold miner, Edward O'Hara, "The gold is already there. It's your job to find it!"
#business#entrepreneur#Entrepreneurship#BusinessTips#MarketingStrategy#SmallBusiness#Startups#DigitalMarketing#SalesTips#BusinessDevelopment#BusinessGrowth#ProductivityHacks#Youtube
1 note
·
View note
Text
Dental Ozone Therapy Units Market Analysis 2025-2033: Demand, Innovation, and Competitive Landscape
Dental Ozone Therapy Units Market
The Global Dental Ozone Therapy Units Market size is projected to grow at a CAGR of XX% during the forecast period.
Research Methodology
Our research methodology constitutes a mix of secondary & primary research which ideally starts from exhaustive data mining, conducting primary interviews (suppliers/distributors/end-users), and formulating insights, estimates, growth rates accordingly. Final primary validation is a mandate to confirm our research findings with Key Opinion Leaders (KoLs), Industry Experts, Mining and Metal Filtration includes major supplies & Independent Consultants among others.
The Global Dental Ozone Therapy Units Market Report provides a 360-degree view of the latest trends, insights, and predictions for the global market, along with detailed analysis of various regional market conditions, market trends, and forecasts for the various segments and sub-segments.
Get Sample Report: https://marketstrides.com/request-sample/dental-ozone-therapy-units-market
LIST OF KEY COMPANIES PROFILED:
Carbotech
DGE Gmbh
DMT Environmental Technology
Kohler & Ziegler
Prometheus Energy
Acrona Systems
Envirotech
SEGMENTATION
By Type
Table-Top
Trolley-Mounted
By Application
Hospital
Dental Clinic
Other
Get In-Detail : https://marketstrides.com/report/dental-ozone-therapy-units-market
Dental Ozone Therapy Units Market REGIONAL INSIGHTS
North America
United States: The U.S. economy has shown resilience post-pandemic but faces inflationary pressures, particularly in housing and consumer goods. The Federal Reserve's interest rate policies remain a focus, as the balance between controlling inflation and avoiding recession has impacted spending, borrowing, and business growth. Key sectors like tech, finance, and renewable energy are experiencing dynamic changes, with AI, fintech, and green technology receiving heavy investments.
Canada: Economic stability remains a hallmark of Canada’s economy, although housing affordability and household debt are pressing issues. Canada continues to emphasize a green energy transition, investing in hydroelectric, wind, and solar power. The nation is also focused on attracting skilled labor, especially in technology, healthcare, and energy, as part of its economic strategy.
Mexico: Mexico has benefited from a nearshoring trend, as companies look to relocate manufacturing closer to the U.S. market. With a strong trade relationship via USMCA (the U.S.-Mexico-Canada Agreement), Mexico is seeing investments in its automotive, aerospace, and electronics industries. However, inflation, interest rates, and a need for infrastructure development remain areas of focus.
Buy Now : https://marketstrides.com/buyNow/dental-ozone-therapy-units-market?price=single_price
FAQ
+ What are the years considered for the study?
+ Can the report be customized based on my requirements?
+ When was the Research conducted/published?
+ What are the mixed proportions of Primary and Secondary Interviews conducted for the study?
+ When will the report be updated?
𝐀𝐛𝐨𝐮𝐭 𝐔𝐬
Market Strides is a Global aggregator and publisher of Market intelligence research reports, equity reports, database directories, and economic reports. Our repository is diverse, spanning virtually every industrial sector and even more every category and sub-category within the industry. Our market research reports provide market sizing analysis, insights on promising industry segments, competition, future outlook and growth drivers in the space. The company is engaged in data analytics and aids clients in due-diligence, product expansion, plant setup, acquisition intelligence to all the other gamut of objectives through our research focus.
𝐂𝐨𝐧𝐭𝐚𝐜𝐭 𝐔𝐬:[email protected]
#Dental Ozone Therapy Units Market Size#Dental Ozone Therapy Units Market Share#Dental Ozone Therapy Units Market Growth#Dental Ozone Therapy Units Market Trends#Dental Ozone Therapy Units Market Players
0 notes
Text
Easy Machine Learning Projects for Absolute Beginners
Machine Learning is an important application of Artificial Intelligence technology and has an enormous potential in a variety of areas including healthcare, business, education, and more. The fact that ML is still in a nascent stage and has several imperfections/flaws can make it difficult to wrap your head around its fundamentals. However, studying and working on a few basic projects on the same can be of great help. Some of them are as follows:
1. Stock Prices Predictor
A system that can learn about a company’s performance and predict future stock prices is not only a great application of Machine Learning but also has value and purpose in the real world. Before proceeding, students of top engineering colleges in Jaipur must sure to acquaint yourself with the following:
a. Statistical Modeling - Constructing a mathematical description of a real-world process that accounts for the uncertainty and/or randomness involved in that system.
b. Predictive Analysis - It uses several techniques such as data mining, artificial intelligence, etc. to predict the behavior of certain outcomes.
c. Regression Analysis - It’s a predictive modeling technique which learns about the relationship between a dependent i.e. the target and independent variable (s) i.e. the predictor.
d. Action Analysis - Analyzing the actions performed by the above-mentioned techniques and incorporating the feedback into the machine learning memory.
The first thing students of best engineering colleges in Jaipur need to get started is select the data types that are to be used such as current prices, EPS ratio, volatility indicators, etc. Once this has been taken care of, you can select the data sources. Similarly, Quantopian offers an excellent trading algorithm development support that you can check out.
Now, you can finally plan on how to backtest and build a trading model. it is important to remember that you need to structure the program in a way that it’s able to validate the predictions quickly as financial markets are usually quite volatile and the stock prices can change several times a day. What you want to do is connect your database to your machine learning system that is fed new data on a regular basis. A running cycle can compare the stock prices of all the companies in the database over the past 15 years or so and predict the same for the near future i.e. 3 days, 7 days, etc, and report on the display.
2. Sentiment Analyzer
A sentiment analyzer learns about the “sentiment” behind a text through machine learning and predicts the same using Artificial Intelligence. The technology is being increasingly used on social media platforms such as Facebook and Twitter for learning user behavior, and by businesses that want to automate lead generation by determining how likely a prospect is to do business with them by reading into their emails.
One innovation that students of engineering colleges will need to learn about in this project is classifiers. You can, however, choose any particular model that you are comfortable with.You can go about the project your way. However, you would ideally need to classify the texts into three categories- positive, neutral, and negative. You can extract the different texts for a particular keyword and run the classifier on each to obtain the labels. For features, you can use diagrams or even dictionaries for higher accuracy.
3. Sports Matches Predictor
Using the basic working model of machine learning, students of private engineering colleges in Jaipur can also create a system that can predict the results of sports matches such as cricket, football, etc. The first thing you need is to create a database for whichever sports you are considering. Irrespective of what you choose, you will most likely need to find the records of the scores, performance details, etc. on your own, manually. Using Json for this, however, could be a good idea as it can easily capture the advanced parameters that are involved in a game and help in making more accurate predictions.
If you are well-versed in Python, then Scikit-Learn is your best bet to create the system. It offers a variety of tools for data mining, regression analysis, classifications, etc. You can use human analysis such as Vegas lines along with some advanced parameters such as Dean Oliver’s four factors to get best prediction results.
Conclusion
There are many beginner-level Machine Learning projects for the students of the list of engineering colleges in Jaipur that you can study. However, it will help if you make yourself familiar with the following first:
a. Machine Learning Tools - An environment that offers ML tools for data preparation, a variety of ML algorithms, and is capable of presenting the results of the programs, can be a good starting point when you want to get to the core of ML and understand how different modules work together. For instance, Weka, waffles, etc. are some of the excellent environments to start with.
b. Machine Learning Datasets - AI and ML use a variety of datasets. However, students of engineering colleges Jaipur can just pick one and choose an algorithm that works the best for it. Then you can use an ML environment to observe it closely. You can also change the algorithms to see how they affect the datasets. Machine Learning can only be mastered with a lot of experimentation and practice. While delving into the theory can surely help, it’s the application that will facilitate your progress the most.
0 notes
Text
Excel Data analysis
Excel Data analysis Data analysis Excel is a powerful tool for data analysis, offering a wide range of features to help you understand and interpret your data. Here are some key aspects of data analysis in Excel: 1. Data Preparation: Data Entry and Import: Excel allows you to manually enter data or import it from various sources like CSV files, databases, and other spreadsheets. Data Cleaning: This involves identifying and correcting errors, inconsistencies, and missing values. Techniques include: Filtering: Isolating specific data based on criteria. Sorting: Arranging data in ascending or descending order. Removing Duplicates: Eliminating redundant data. Text to Columns: Splitting data within a single cell into multiple columns. Data Transformation: This involves modifying data to suit your analysis needs. Techniques include: Formulas and Functions: Using built-in functions like SUM, AVERAGE, IF, and VLOOKUP to perform calculations and manipulate data. PivotTables: Summarizing and analyzing large datasets by grouping and aggregating data. Data Tables: Performing "what-if" analysis by changing input values and observing the impact on results. 2. Data Analysis Techniques: Descriptive Statistics: Calculating summary statistics like mean, median, mode, standard deviation, and percentiles to describe the central tendency and variability of your data. Data Visualization: Creating charts and graphs (e.g., bar charts, line graphs, scatter plots, pie charts) to visually represent data and identify trends, patterns, and outliers. Regression Analysis: Modeling the relationship between variables to make predictions or understand cause-and-effect relationships. Hypothesis Testing: Using statistical tests to determine if there is significant evidence to support a claim or hypothesis about your data. Data Mining: Discovering hidden patterns and relationships within large datasets using techniques like clustering and classification. 3. Tools and Features: Formulas and Functions: A vast library of built-in functions for calculations, data manipulation, and statistical analysis. PivotTables: Powerful tool for summarizing and analyzing large datasets by creating interactive tables. Charts and Graphs: A variety of chart types to visualize data effectively. Conditional Formatting: Applying visual rules to highlight data that meets specific criteria. Data Analysis ToolPak: An add-in that provides advanced statistical and data analysis tools, including regression, ANOVA, and time series analysis. 4. Examples of Data Analysis in Excel: Financial Analysis: Calculating financial ratios, analyzing stock trends, and forecasting future performance. Sales Analysis: Tracking sales trends, identifying top-selling products, and analyzing customer behavior. Market Research: Analyzing survey data, identifying customer preferences, and segmenting markets. Quality Control: Monitoring product quality, identifying defects, and analyzing production processes. Scientific Research: Analyzing experimental data, conducting statistical tests, and generating reports. By effectively utilizing Excel's data analysis features, you can gain valuable insights from your data, make informed decisions, and improve your business or research outcomes. اكسل متقدم via عالم الاوفيس https://ift.tt/k2WmMpl January 01, 2025 at 01:03AM
0 notes
Text
Signal Detection in Pharmacovigilance Training & Regulatory Affairs Courses in India: Enhancing Drug Safety Expertise
The pharmaceutical industry is under increasing pressure to ensure that the medicines it produces are both safe and effective. Pharmacovigilance, the science related to the detection, assessment, understanding, and prevention of adverse effects of drugs, plays a crucial role in this process. Signal detection, a key component of pharmacovigilance, focuses on identifying potential safety concerns or adverse drug reactions (ADRs) that might not be evident during the clinical trial phase but become apparent when drugs are used in larger populations. To meet the growing demand for expertise in this area, Signal Detection Pharmacovigilance Training and Regulatory Affairs courses in India have emerged as essential educational tools for professionals in the pharmaceutical, biotechnology, and healthcare sectors.
Signal Detection in Pharmacovigilance Training
Signal detection refers to the process of identifying new or previously unrecognized safety concerns or ADRs from data collected in post-marketing surveillance. This data is gathered from various sources, including clinical trials, spontaneous reporting systems, and healthcare databases. Signal detection is a critical activity in pharmacovigilance as it helps identify risks that could lead to regulatory actions, such as labeling changes, market withdrawals, or further clinical investigations.
Signal detection training focuses on key topics such as:
1. Data Mining Techniques: Understanding statistical methods like disproportionality analysis and Bayesian analysis to identify signals from large datasets.
2. Regulatory Guidelines: Learning about international standards and best practices, including ICH E2E, FDA, and EMA guidelines, which govern signal detection and reporting.
3. Safety Databases: Training professionals to effectively utilize databases like the WHO Global Individual Case Safety Reports (ICSRs) or the FDA Adverse Event Reporting System (FAERS).
4. Risk Management: Understanding how to assess and mitigate risks once a signal is detected, which includes determining the severity, potential impact, and appropriate regulatory responses.
5. Communication: Learning how to communicate detected signals to regulatory authorities, healthcare providers, and the public, ensuring transparency and swift action when needed.
In India, various institutions and organizations offer specialized Signal Detection Pharmacovigilance Training programs, enabling professionals to enhance their skills and stay up-to-date with global pharmacovigilance practices.
Regulatory Affairs Courses in India
In addition to pharmacovigilance training, Regulatory Affairs courses in India are gaining immense popularity. Regulatory affairs professionals are responsible for ensuring that pharmaceutical products comply with the regulatory requirements of the countries where they are marketed. This includes navigating the regulatory approval process, preparing submission dossiers, ensuring compliance with clinical trial standards, and maintaining post-market surveillance.

Regulatory Affairs courses in India cover a wide range of topics, such as:
1. Regulatory Guidelines & Framework: A deep dive into the regulatory requirements of major markets, including the FDA, EMA, and India’s Central Drugs Standard Control Organization (CDSCO).
2. Clinical Trials and Good Clinical Practices (GCP): Understanding the regulatory standards for conducting clinical trials, including the ethical aspects of human subject research.
3. Drug Development Lifecycle: From preclinical development through to marketing authorization and post-market surveillance, regulatory affairs professionals are trained to oversee every stage of a product’s lifecycle.
4. Compliance and Risk Management: A focus on how to ensure ongoing compliance with regulatory standards and manage risks associated with drug development and marketing.
Conclusion
Signal detection in pharmacovigilance and regulatory affairs are vital areas of expertise in today’s pharmaceutical landscape. In India, specialized training in these fields is opening doors to a wide range of career opportunities. As the pharmaceutical industry continues to grow, professionals equipped with training in signal detection and regulatory affairs are better positioned to contribute to drug safety, compliance, and overall public health. By investing in these courses, individuals can elevate their careers and play a pivotal role in ensuring the safety and efficacy of medicines in the market.
0 notes
Text
Essential Predictive Analytics Techniques
With the growing usage of big data analytics, predictive analytics uses a broad and highly diverse array of approaches to assist enterprises in forecasting outcomes. Examples of predictive analytics include deep learning, neural networks, machine learning, text analysis, and artificial intelligence.
Predictive analytics trends of today reflect existing Big Data trends. There needs to be more distinction between the software tools utilized in predictive analytics and big data analytics solutions. In summary, big data and predictive analytics technologies are closely linked, if not identical.
Predictive analytics approaches are used to evaluate a person's creditworthiness, rework marketing strategies, predict the contents of text documents, forecast weather, and create safe self-driving cars with varying degrees of success.
Predictive Analytics- Meaning
By evaluating collected data, predictive analytics is the discipline of forecasting future trends. Organizations can modify their marketing and operational strategies to serve better by gaining knowledge of historical trends. In addition to the functional enhancements, businesses benefit in crucial areas like inventory control and fraud detection.
Machine learning and predictive analytics are closely related. Regardless of the precise method, a company may use, the overall procedure starts with an algorithm that learns through access to a known result (such as a customer purchase).
The training algorithms use the data to learn how to forecast outcomes, eventually creating a model that is ready for use and can take additional input variables, like the day and the weather.
Employing predictive analytics significantly increases an organization's productivity, profitability, and flexibility. Let us look at the techniques used in predictive analytics.
Techniques of Predictive Analytics
Making predictions based on existing and past data patterns requires using several statistical approaches, data mining, modeling, machine learning, and artificial intelligence. Machine learning techniques, including classification models, regression models, and neural networks, are used to make these predictions.
Data Mining
To find anomalies, trends, and correlations in massive datasets, data mining is a technique that combines statistics with machine learning. Businesses can use this method to transform raw data into business intelligence, including current data insights and forecasts that help decision-making.
Data mining is sifting through redundant, noisy, unstructured data to find patterns that reveal insightful information. A form of data mining methodology called exploratory data analysis (EDA) includes examining datasets to identify and summarize their fundamental properties, frequently using visual techniques.
EDA focuses on objectively probing the facts without any expectations; it does not entail hypothesis testing or the deliberate search for a solution. On the other hand, traditional data mining focuses on extracting insights from the data or addressing a specific business problem.
Data Warehousing
Most extensive data mining projects start with data warehousing. An example of a data management system is a data warehouse created to facilitate and assist business intelligence initiatives. This is accomplished by centralizing and combining several data sources, including transactional data from POS (point of sale) systems and application log files.
A data warehouse typically includes a relational database for storing and retrieving data, an ETL (Extract, Transfer, Load) pipeline for preparing the data for analysis, statistical analysis tools, and client analysis tools for presenting the data to clients.
Clustering
One of the most often used data mining techniques is clustering, which divides a massive dataset into smaller subsets by categorizing objects based on their similarity into groups.
When consumers are grouped together based on shared purchasing patterns or lifetime value, customer segments are created, allowing the company to scale up targeted marketing campaigns.
Hard clustering entails the categorization of data points directly. Instead of assigning a data point to a cluster, soft clustering gives it a likelihood that it belongs in one or more clusters.
Classification
A prediction approach called classification involves estimating the likelihood that a given item falls into a particular category. A multiclass classification problem has more than two classes, unlike a binary classification problem, which only has two types.
Classification models produce a serial number, usually called confidence, that reflects the likelihood that an observation belongs to a specific class. The class with the highest probability can represent a predicted probability as a class label.
Spam filters, which categorize incoming emails as "spam" or "not spam" based on predetermined criteria, and fraud detection algorithms, which highlight suspicious transactions, are the most prevalent examples of categorization in a business use case.
Regression Model
When a company needs to forecast a numerical number, such as how long a potential customer will wait to cancel an airline reservation or how much money they will spend on auto payments over time, they can use a regression method.
For instance, linear regression is a popular regression technique that searches for a correlation between two variables. Regression algorithms of this type look for patterns that foretell correlations between variables, such as the association between consumer spending and the amount of time spent browsing an online store.
Neural Networks
Neural networks are data processing methods with biological influences that use historical and present data to forecast future values. They can uncover intricate relationships buried in the data because of their design, which mimics the brain's mechanisms for pattern recognition.
They have several layers that take input (input layer), calculate predictions (hidden layer), and provide output (output layer) in the form of a single prediction. They are frequently used for applications like image recognition and patient diagnostics.
Decision Trees
A decision tree is a graphic diagram that looks like an upside-down tree. Starting at the "roots," one walks through a continuously narrowing range of alternatives, each illustrating a possible decision conclusion. Decision trees may handle various categorization issues, but they can resolve many more complicated issues when used with predictive analytics.
An airline, for instance, would be interested in learning the optimal time to travel to a new location it intends to serve weekly. Along with knowing what pricing to charge for such a flight, it might also want to know which client groups to cater to. The airline can utilize a decision tree to acquire insight into the effects of selling tickets to destination x at price point y while focusing on audience z, given these criteria.
Logistics Regression
It is used when determining the likelihood of success in terms of Yes or No, Success or Failure. We can utilize this model when the dependent variable has a binary (Yes/No) nature.
Since it uses a non-linear log to predict the odds ratio, it may handle multiple relationships without requiring a linear link between the variables, unlike a linear model. Large sample sizes are also necessary to predict future results.
Ordinal logistic regression is used when the dependent variable's value is ordinal, and multinomial logistic regression is used when the dependent variable's value is multiclass.
Time Series Model
Based on past data, time series are used to forecast the future behavior of variables. Typically, a stochastic process called Y(t), which denotes a series of random variables, are used to model these models.
A time series might have the frequency of annual (annual budgets), quarterly (sales), monthly (expenses), or daily (daily expenses) (Stock Prices). It is referred to as univariate time series forecasting if you utilize the time series' past values to predict future discounts. It is also referred to as multivariate time series forecasting if you include exogenous variables.
The most popular time series model that can be created in Python is called ARIMA, or Auto Regressive Integrated Moving Average, to anticipate future results. It's a forecasting technique based on the straightforward notion that data from time series' initial values provides valuable information.
In Conclusion-
Although predictive analytics techniques have had their fair share of critiques, including the claim that computers or algorithms cannot foretell the future, predictive analytics is now extensively employed in virtually every industry. As we gather more and more data, we can anticipate future outcomes with a certain level of accuracy. This makes it possible for institutions and enterprises to make wise judgments.
Implementing Predictive Analytics is essential for anybody searching for company growth with data analytics services since it has several use cases in every conceivable industry. Contact us at SG Analytics if you want to take full advantage of predictive analytics for your business growth.
2 notes
·
View notes
Text
SEO Tools You Need to Know About in 2025
SEO isn't just alive-it's flourishing in 2025. More than ever, business enterprises are investing in getting their websites up at the top of search results. But why does it still hold such sway in the market, and how do the tools have evolved over time?
Why SEO Still Matters It's like constructing a beautiful shop in the middle of nowhere without any signpost to lead the customers to it. That is what a website without SEO looks like. SEO brings in visibility, credibility, and higher conversions.
The Evolution of SEO Tools The evolution of SEO tools, from basic keyword planners to AI-driven analytics, mirrors the complexities of modern-day optimization. Let's go through the advanced tools in 2025.
All-inclusive Keyword Research Tools Finding the right keyword is like finding the magic recipe for success. Following are some of the hot tools leading the charge:
Google Keyword Planner: The Free Essential Google's Keyword Planner is still an indispensable tool for cost-effective marketers. It offers a sense of search volume, trend, and competition.
SEMrush: The Competitive Analysis King SEMrush isn't just a keyword tool; it's your competitive intelligence partner. From keyword difficulty to competitor performance, SEMrush covers it all.
Ahrefs: Unlocking Long-Tail Opportunities For those searching for long-tail keywords, Ahrefs is the treasure chest. Its immense backlink database also gets you ahead with link equity building.
Tools For On Page SEO Making your content readable to audiences and search engines is essential. Here are the tools that you cannot skip:
Yoast SEO: The WordPress Powerhouse Yoast SEO makes WordPress users get meta tags streamlined, readability, and schema markup into one package that is great for beginners as well as powerful.
Surfer SEO: Content Optimization Simplified Surfer SEO closes the gap between data and creativity. It analyzes your top-performing pages, helping you craft content that ranks.
Rank Math: Flexibility for Modern Websites Rank Math provides a comprehensive set of on-page tools including keyword suggestions, rich snippets, and advanced schema features.
Off-Page SEO Essentials Building your website's authority is just as important as optimizing its content.
BuzzSumo: Finding Viral Content BuzzSumo helps you track trending topics and influencers in your niche, paving the way for shareable content.
Hunter.io: Building Strong Backlinks Hunter.io makes outreach easy by finding professional email addresses, perfect for link-building campaigns.
Technical SEO Tools No SEO strategy is complete without addressing technical issues. These tools ensure your foundation is rock solid:
Screaming Frog: Crawling and Auditing Made Easy Screaming Frog is a lifesaver for detecting broken links, duplicate content, and crawl issues.
Google Search Console: Your Free Technical Partner Google Search Console is a goldmine of insight into indexing, ranking problems, and Core Web Vitals.
GTmetrix: Speed Up Your Site Page speed is a ranking factor, and GTmetrix helps identify bottlenecks slowing your site down.
Analytics and Reporting Tools Data-driven decisions form the backbone of SEO success.
Google Analytics 4: The Data Gold Mine With enhanced tracking capabilities, GA4 offers more granular insights into user behavior and campaign performance.
Databox: Streamline Performance Metrics Databox aggregates data from various sources into one dashboard, which makes reporting easy.
AI and Automation in SEO AI is not the future; it is now.
ChatGPT: Boosting Content Strategies ChatGPT can give ideas for blogs, drafts, and even meta descriptions. This saves time and effort.
MarketMuse: AI-Powered Content Creation MarketMuse uses AI to identify gaps in content, suggest improvements, and prioritize topics.
Emerging SEO Trends for 2025 SEO in 2025 is no longer about text and backlinks.
Voice Search Optimization Tools With smart speakers dominating homes, tools like AnswerThePublic are tailoring content for voice search.
Video SEO: Tools for Growing Platforms Like YouTube Platforms like TubeBuddy and VidIQ are crucial for optimizing video content.
Choosing the Right Tools for Your Needs Not every tool is for everyone. Here's how to decide:
Budget-Friendly Options Tools like Google Keyword Planner and Google Search Console deliver results without costing a dime.
Premium Tools for Advanced Strategies Invest in Ahrefs or SEMrush if you’re scaling up and need in-depth data.
Conclusion Mastering SEO in 2025 means staying ahead with the right tools. From keyword research to technical optimization, these tools ensure you’re always a step ahead of the competition. Start exploring today and watch your rankings soar!
FAQs
What is the best free SEO tool for beginners? Google Keyword Planner is an excellent starting point for keyword research.
How do AI tools help in SEO? AI tools like ChatGPT streamline content creation and strategy development, saving time.
Are premium SEO tools worth the investment? Yes, if you're serious about scaling your SEO efforts, premium tools provide unparalleled insights.
Which tool is best for optimizing page speed? GTmetrix is a top choice for analyzing and improving website speed.
What trends should I watch in SEO for 2025? Pay attention to voice search optimization and video SEO as those keep growing.
0 notes
Text
Silica Prices: Trends, Database, and Market Outlook
Silica, also known as silicon dioxide (SiO₂), is a key industrial material widely used in various industries such as glass manufacturing, construction, electronics, and rubber production. Its market dynamics are closely linked to its diverse applications, making silica prices an essential topic of interest for manufacturers, traders, and industry stakeholders.
Silica Prices: Current Trends
In recent years, silica prices have displayed moderate fluctuations driven by shifts in demand across industries and the impact of global economic conditions. The glass industry, being a major consumer of silica, has a significant influence on its pricing. With the growing adoption of energy-efficient glass and increased construction activities, demand for high-purity silica has surged.
Get Real time Prices for Silica: https://www.chemanalyst.com/Pricing-data/silica-1304
Factors Influencing Silica Prices
Demand from End-User Industries The demand for silica in sectors like electronics, automotive, and construction drives its market price. For instance, the growing use of silica in semiconductor manufacturing and 5G infrastructure development has supported price stability despite fluctuations in other markets.
Raw Material Availability Silica is primarily extracted from quartz sand or natural quartz. The availability and mining costs of these raw materials significantly influence production costs. Any disruption in the supply chain, such as mining restrictions or transport bottlenecks, can cause price volatility.
Energy Costs Silica production, particularly for high-purity forms, is energy-intensive. Rising energy costs, including electricity and fuel prices, directly impact silica pricing. With the global push for clean energy, some silica producers are transitioning to renewable energy sources, which could stabilize costs in the long term.
Geopolitical and Trade Factors Trade policies, tariffs, and geopolitical tensions play a crucial role in the silica market. For instance, countries with abundant silica resources, such as China and the United States, influence global pricing through their production and export strategies.
Environmental Regulations Stricter environmental regulations in mining and manufacturing have raised production costs for silica. These additional costs are often passed on to consumers, resulting in higher market prices.
Future Outlook for Silica Prices
The silica market is expected to grow steadily, driven by expanding applications in green technologies, electronics, and construction. According to industry forecasts, global silica demand is set to increase at a compound annual growth rate (CAGR) of around 6% over the next five years.
Innovations in silica applications, such as its use in battery technology for electric vehicles and lightweight materials for aerospace, are anticipated to create new opportunities for producers. These emerging trends could lead to price stabilization or even growth, depending on supply chain efficiency and technological advancements.
Conclusion
Silica prices are shaped by a complex interplay of demand-supply dynamics, raw material costs, energy expenses, and regulatory influences. Stakeholders in the silica market must closely monitor these factors to make informed decisions. With its expanding industrial applications and growing emphasis on sustainability, silica is poised to remain a critical material in the global market, with prices reflecting its indispensable role in modern industry.
Contact Us:
ChemAnalyst
GmbH - S-01, 2.floor, Subbelrather Straße,
15a Cologne, 50823, Germany
Call: +49-221-6505-8833
Email: [email protected]
Website: https://www.chemanalyst.com
#Silica#Silica Price#Silica Prices#Silica Pricing#Silica News#Silica Price Monitor#Silica Database#Silica Price Chart#india#united kingdom#united states#germany#business#research#chemicals#Technology#Market Research#Canada#Japan#China
0 notes