#BigQuery use cases
Explore tagged Tumblr posts
Text
Aible And Google Cloud: Gen AI Models Sets Business Security
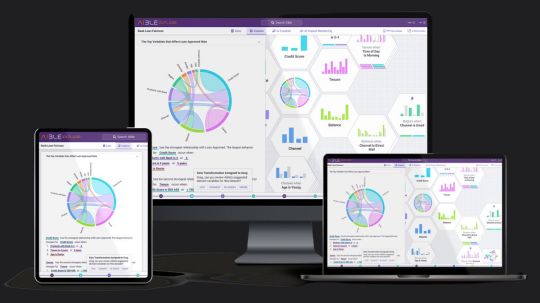
Enterprise controls and generative AI for business users in real time.
Aible
With solutions for customer acquisition, churn avoidance, demand prediction, preventive maintenance, and more, Aible is a pioneer in producing business impact from AI in less than 30 days. Teams can use AI to extract company value from raw enterprise data. Previously using BigQuery’s serverless architecture to save analytics costs, Aible is now working with Google Cloud to provide users the confidence and security to create, train, and implement generative AI models on their own data.
The following important factors have surfaced as market awareness of generative AI’s potential grows:
Enabling enterprise-grade control
Businesses want to utilize their corporate data to allow new AI experiences, but they also want to make sure they have control over their data to prevent unintentional usage of it to train AI models.
Reducing and preventing hallucinations
The possibility that models may produce illogical or non-factual information is another particular danger associated with general artificial intelligence.
Empowering business users
Enabling and empowering business people to utilize gen AI models with the least amount of hassle is one of the most beneficial use cases, even if gen AI supports many enterprise use cases.
Scaling use cases for gen AI
Businesses need a method for gathering and implementing their most promising use cases at scale, as well as for establishing standardized best practices and controls.
Regarding data privacy, policy, and regulatory compliance, the majority of enterprises have a low risk tolerance. However, given its potential to drive change, they do not see postponing the deployment of Gen AI as a feasible solution to market and competitive challenges. As a consequence, Aible sought an AI strategy that would protect client data while enabling a broad range of corporate users to swiftly adapt to a fast changing environment.
In order to provide clients complete control over how their data is used and accessed while creating, training, or optimizing AI models, Aible chose to utilize Vertex AI, Google Cloud’s AI platform.
Enabling enterprise-grade controls
Because of Google Cloud’s design methodology, users don’t need to take any more steps to ensure that their data is safe from day one. Google Cloud tenant projects immediately benefit from security and privacy thanks to Google AI products and services. For example, protected customer data in Cloud Storage may be accessed and used by Vertex AI Agent Builder, Enterprise Search, and Conversation AI. Customer-managed encryption keys (CMEK) can be used to further safeguard this data.
With Aible‘s Infrastructure as Code methodology, you can quickly incorporate all of Google Cloud’s advantages into your own applications. Whether you choose open models like LLama or Gemma, third-party models like Anthropic and Cohere, or Google gen AI models like Gemini, the whole experience is fully protected in the Vertex AI Model Garden.
In order to create a system that may activate third-party gen AI models without disclosing private data outside of Google Cloud, Aible additionally collaborated with its client advisory council, which consists of Fortune 100 organizations. Aible merely transmits high-level statistics on clusters which may be hidden if necessary instead of raw data to an external model. For instance, rather of transmitting raw sales data, it may communicate counts and averages depending on product or area.
This makes use of k-anonymity, a privacy approach that protects data privacy by never disclosing information about groups of people smaller than k. You may alter the default value of k; the more private the information transmission, the higher the k value. Aible makes the data transmission even more secure by changing the names of variables like “Country” to “Variable A” and values like “Italy” to “Value X” when masking is used.
Mitigating hallucination risk
It’s crucial to use grounding, retrieval augmented generation (RAG), and other strategies to lessen and lower the likelihood of hallucinations while employing gen AI. Aible, a partner of Built with Google Cloud AI, offers automated analysis to support human-in-the-loop review procedures, giving human specialists the right tools that can outperform manual labor.
Using its auto-generated Information Model (IM), an explainable AI that verifies facts based on the context contained in your structured corporate data at scale and double checks gen AI replies to avoid making incorrect conclusions, is one of the main ways Aible helps eliminate hallucinations.
Hallucinations are addressed in two ways by Aible’s Information Model:
It has been shown that the IM helps lessen hallucinations by grounding gen AI models on a relevant subset of data.
To verify each fact, Aible parses through the outputs of Gen AI and compares them to millions of responses that the Information Model already knows.
This is comparable to Google Cloud’s Vertex AI grounding features, which let you link models to dependable information sources, like as your company’s papers or the Internet, to base replies in certain data sources. A fact that has been automatically verified is shown in blue with the words “If it’s blue, it’s true.” Additionally, you may examine a matching chart created only by the Information Model and verify a certain pattern or variable.
The graphic below illustrates how Aible and Google Cloud collaborate to provide an end-to-end serverless environment that prioritizes artificial intelligence. Aible can analyze datasets of any size since it leverages BigQuery to efficiently analyze and conduct serverless queries across millions of variable combinations. One Fortune 500 client of Aible and Google Cloud, for instance, was able to automatically analyze over 75 datasets, which included 150 million questions and answers with 100 million rows of data. That assessment only cost $80 in total.
Aible may also access Model Garden, which contains Gemini and other top open-source and third-party models, by using Vertex AI. This implies that Aible may use AI models that are not Google-generated while yet enjoying the advantages of extra security measures like masking and k-anonymity.
All of your feedback, reinforcement learning, and Low-Rank Adaptation (LoRA) data are safely stored in your Google Cloud project and are never accessed by Aible.
Read more on Govindhtech.com
#Aible#GenAI#GenAIModels#BusinessSecurity#AI#BigQuery#AImodels#VertexAI#News#Technews#Technology#Technologynews#Technologytrends#govindhtech
2 notes
·
View notes
Text
A Comprehensive Analysis of AWS, Azure, and Google Cloud for Linux Environments
In the dynamic landscape of cloud computing, selecting the right platform is a critical decision, especially for a Linux-based, data-driven business. Amazon Web Services (AWS), Microsoft Azure, and Google Cloud Platform (GCP) stand as the giants in the cloud industry, each offering unique strengths. With AWS Training in Hyderabad, professionals can gain the skills and knowledge needed to harness the capabilities of AWS for diverse applications and industries. Let’s delve into a simplified comparison to help you make an informed choice tailored to your business needs.
Amazon Web Services (AWS):
Strengths:
AWS boasts an extensive array of services and a global infrastructure, making it a go-to choice for businesses seeking maturity and reliability. Its suite of tools caters to diverse needs, including robust options for data analytics, storage, and processing.
Considerations:
Pricing in AWS can be intricate, but the platform provides a free tier for newcomers to explore and experiment. The complexity of pricing is offset by the vast resources and services available, offering flexibility for businesses of all sizes.
Microsoft Azure:
Strengths:
Azure stands out for its seamless integration with Microsoft products. If your business relies heavily on tools like Windows Server, Active Directory, or Microsoft SQL Server, Azure is a natural fit. It also provides robust data analytics services and is expanding its global presence with an increasing number of data centers.
Considerations:
Azure’s user-friendly interface, especially for those familiar with Microsoft technologies, sets it apart. Competitive pricing, along with a free tier, makes it accessible for businesses looking to leverage Microsoft’s extensive ecosystem.
Google Cloud Platform (GCP):
Strengths:
Renowned for innovation and a developer-friendly approach, GCP excels in data analytics and machine learning. If your business is data-driven, Google’s BigQuery and other analytics tools offer a compelling proposition. Google Cloud is known for its reliability and cutting-edge technologies.
Considerations:
While GCP may have a slightly smaller market share, it compensates with a focus on innovation. Its competitive pricing and a free tier make it an attractive option, especially for businesses looking to leverage advanced analytics and machine learning capabilities. To master the intricacies of AWS and unlock its full potential, individuals can benefit from enrolling in the Top AWS Training Institute.
Considerations for Your Linux-based, Data-Driven Business:
1. Data Processing and Analytics:
All three cloud providers offer robust solutions for data processing and analytics. If your business revolves around extensive data analytics, Google Cloud’s specialization in this area might be a deciding factor.
2. Integration with Linux:
All three providers support Linux, with AWS and Azure having extensive documentation and community support. Google Cloud is also Linux-friendly, ensuring compatibility with your Linux-based infrastructure.
3. Global Reach:
Consider the geographic distribution of data centers. AWS has a broad global presence, followed by Azure. Google Cloud, while growing, may have fewer data centers in certain regions. Choose a provider with data centers strategically located for your business needs.
4. Cost Considerations:
Evaluate the pricing models for your specific use cases. AWS and Azure offer diverse pricing options, and GCP’s transparent and competitive pricing can be advantageous. Understand the cost implications based on your anticipated data processing volumes.
5. Support and Ecosystem:
Assess the support and ecosystem offered by each provider. AWS has a mature and vast ecosystem, Azure integrates seamlessly with Microsoft tools, and Google Cloud is known for its developer-centric approach. Consider the level of support, documentation, and community engagement each platform provides.
In conclusion, the choice between AWS, Azure, and GCP depends on your unique business requirements, preferences, and the expertise of your team. Many businesses adopt a multi-cloud strategy, leveraging the strengths of each provider for different aspects of their operations. Starting with the free tiers and conducting a small-scale pilot can help you gauge which platform aligns best with your specific needs. Remember, the cloud is not a one-size-fits-all solution, and the right choice depends on your business’s distinctive characteristics and goals.
2 notes
·
View notes
Text
A comparison of Snowflake with legacy systems and other cloud platforms.
Here’s a structured comparison of Snowflake vs. Legacy Systems vs. Other Cloud Platforms to highlight their differences in terms of architecture, performance, scalability, cost, and use cases.
1. Architecture
2. Performance & Scalability
3. Cost & Pricing
4. Security & Compliance
5. Use Cases
Key Takeaways
Snowflake offers seamless scalability, pay-as-you-go pricing, and cross-cloud flexibility, making it ideal for modern analytics workloads.
Legacy Systems struggle with scalability, maintenance, and cost efficiency.
Other Cloud Platforms like AWS Redshift, BigQuery, and Azure Synapse provide similar capabilities but with vendor lock-in and varying scalability options.
WEBSITE: https://www.ficusoft.in/snowflake-training-in-chennai/
0 notes
Text
Best Informatica Cloud Training in India | Informatica IICS
Cloud Data Integration (CDI) in Informatica IICS
Introduction
Cloud Data Integration (CDI) in Informatica Intelligent Cloud Services (IICS) is a powerful solution that helps organizations efficiently manage, process, and transform data across hybrid and multi-cloud environments. CDI plays a crucial role in modern ETL (Extract, Transform, Load) and ELT (Extract, Load, Transform) operations, enabling businesses to achieve high-performance data processing with minimal complexity. In today’s data-driven world, businesses need seamless integration between various data sources, applications, and cloud platforms. Informatica Training Online
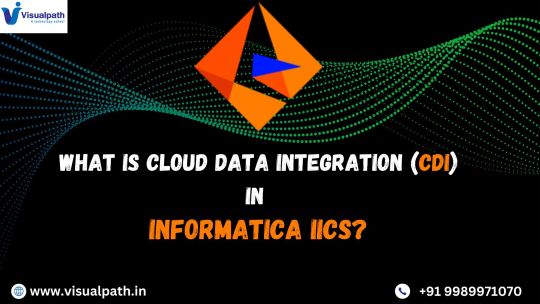
What is Cloud Data Integration (CDI)?
Cloud Data Integration (CDI) is a Software-as-a-Service (SaaS) solution within Informatica IICS that allows users to integrate, transform, and move data across cloud and on-premises systems. CDI provides a low-code/no-code interface, making it accessible for both technical and non-technical users to build complex data pipelines without extensive programming knowledge.
Key Features of CDI in Informatica IICS
Cloud-Native Architecture
CDI is designed to run natively on the cloud, offering scalability, flexibility, and reliability across various cloud platforms like AWS, Azure, and Google Cloud.
Prebuilt Connectors
It provides out-of-the-box connectors for SaaS applications, databases, data warehouses, and enterprise applications such as Salesforce, SAP, Snowflake, and Microsoft Azure.
ETL and ELT Capabilities
Supports ETL for structured data transformation before loading and ELT for transforming data after loading into cloud storage or data warehouses.
Data Quality and Governance
Ensures high data accuracy and compliance with built-in data cleansing, validation, and profiling features. Informatica IICS Training
High Performance and Scalability
CDI optimizes data processing with parallel execution, pushdown optimization, and serverless computing to enhance performance.
AI-Powered Automation
Integrated Informatica CLAIRE, an AI-driven metadata intelligence engine, automates data mapping, lineage tracking, and error detection.
Benefits of Using CDI in Informatica IICS
1. Faster Time to Insights
CDI enables businesses to integrate and analyze data quickly, helping data analysts and business teams make informed decisions in real-time.
2. Cost-Effective Data Integration
With its serverless architecture, businesses can eliminate on-premise infrastructure costs, reducing Total Cost of Ownership (TCO) while ensuring high availability and security.
3. Seamless Hybrid and Multi-Cloud Integration
CDI supports hybrid and multi-cloud environments, ensuring smooth data flow between on-premises systems and various cloud providers without performance issues. Informatica Cloud Training
4. No-Code/Low-Code Development
Organizations can build and deploy data pipelines using a drag-and-drop interface, reducing dependency on specialized developers and improving productivity.
5. Enhanced Security and Compliance
Informatica ensures data encryption, role-based access control (RBAC), and compliance with GDPR, CCPA, and HIPAA standards, ensuring data integrity and security.
Use Cases of CDI in Informatica IICS
1. Cloud Data Warehousing
Companies migrating to cloud-based data warehouses like Snowflake, Amazon Redshift, or Google BigQuery can use CDI for seamless data movement and transformation.
2. Real-Time Data Integration
CDI supports real-time data streaming, enabling enterprises to process data from IoT devices, social media, and APIs in real-time.
3. SaaS Application Integration
Businesses using applications like Salesforce, Workday, and SAP can integrate and synchronize data across platforms to maintain data consistency. IICS Online Training
4. Big Data and AI/ML Workloads
CDI helps enterprises prepare clean and structured datasets for AI/ML model training by automating data ingestion and transformation.
Conclusion
Cloud Data Integration (CDI) in Informatica IICS is a game-changer for enterprises looking to modernize their data integration strategies. CDI empowers businesses to achieve seamless data connectivity across multiple platforms with its cloud-native architecture, advanced automation, AI-powered data transformation, and high scalability. Whether you’re migrating data to the cloud, integrating SaaS applications, or building real-time analytics pipelines, Informatica CDI offers a robust and efficient solution to streamline your data workflows.
For organizations seeking to accelerate digital transformation, adopting Informatics’ Cloud Data Integration (CDI) solution is a strategic step toward achieving agility, cost efficiency, and data-driven innovation.
For More Information about Informatica Cloud Online Training
Contact Call/WhatsApp: +91 7032290546
Visit: https://www.visualpath.in/informatica-cloud-training-in-hyderabad.html
#Informatica Training in Hyderabad#IICS Training in Hyderabad#IICS Online Training#Informatica Cloud Training#Informatica Cloud Online Training#Informatica IICS Training#Informatica Training Online#Informatica Cloud Training in Chennai#Informatica Cloud Training In Bangalore#Best Informatica Cloud Training in India#Informatica Cloud Training Institute#Informatica Cloud Training in Ameerpet
0 notes
Text
Exploring Cloud Computing Careers: Roles, Skills, and How to Get Started
Cloud computing has transformed how businesses operate by providing scalable, on-demand access to computing resources via the internet. As organizations increasingly adopt cloud technologies, the need for skilled professionals in this field has skyrocketed. But what exactly does a cloud computing job involve? In this article, we’ll explore the roles, responsibilities, and skills needed for a career in cloud computing. We’ll also highlight how the Boston Institute of Analytics' Cloud Computing Course can help you thrive in this exciting domain.
What Do Cloud Computing Jobs Entail?
A career in cloud computing revolves around using cloud-based technologies to design, implement, and manage applications and services hosted on platforms like Amazon Web Services (AWS), Microsoft Azure, and Google Cloud Platform (GCP). These roles play a crucial part in helping businesses harness the cloud's power to improve efficiency, scalability, and cost-effectiveness.
Cloud computing jobs span various areas, such as infrastructure management, application development, data analytics, and cybersecurity. Let’s dive into some of the most common roles within this field:
1. Cloud Engineer
Cloud engineers focus on building, deploying, and maintaining cloud infrastructure. Their work ensures that applications and services run seamlessly in the cloud. Key responsibilities include:
Configuring cloud environments.
Automating deployments with tools like Terraform or Ansible.
Monitoring and optimizing cloud system performance.
2. Cloud Architect
Cloud architects design overarching cloud strategies and infrastructure frameworks. They collaborate with stakeholders to align cloud solutions with organizational objectives. Their responsibilities include:
Creating cloud architecture designs.
Choosing the best cloud services for specific use cases.
Ensuring security and compliance in cloud environments.
3. DevOps Engineer
DevOps engineers work to streamline collaboration between development and operations teams, emphasizing automation and continuous integration/continuous deployment (CI/CD). Their tasks include:
Building and managing CI/CD pipelines.
Automating infrastructure provisioning.
Enhancing application performance in cloud environments.
4. Cloud Security Specialist
As cybersecurity becomes increasingly critical, cloud security specialists protect cloud environments from threats. Their responsibilities include:
Implementing robust security practices.
Identifying and addressing vulnerabilities.
Managing access controls and identity systems.
5. Data Engineer
Data engineers use cloud-based tools to process and analyze large datasets. They rely on cloud platforms for storage, computation, and machine learning tasks. Key responsibilities include:
Developing data pipelines.
Managing cloud data warehouses like Google BigQuery or Amazon Redshift.
Ensuring data quality and availability.
Essential Skills for Cloud Computing Careers
Excelling in a cloud computing role requires a blend of technical expertise and soft skills. Here are the key skills to focus on:
Technical Skills
Familiarity with Cloud Platforms
Proficiency in AWS, Azure, or GCP is a must, as each platform offers unique tools and services tailored to business needs.
Programming Knowledge
Languages like Python, Java, and Go are widely used for automating tasks and building cloud-native applications.
Networking Fundamentals
Understanding concepts like DNS, load balancing, and firewalls is crucial for managing cloud environments.
Containerization and Orchestration
Tools like Docker and Kubernetes are essential for deploying and managing containerized applications.
Cloud Security
Familiarity with encryption, identity management, and other security best practices is vital for safeguarding cloud resources.
Soft Skills
Problem-Solving
Cloud professionals must identify and resolve technical challenges effectively.
Team Collaboration
Strong communication and teamwork skills are essential for working across departments.
Adaptability
Keeping up with rapidly evolving cloud technologies is a critical skill for long-term success.
Steps to Launch Your Cloud Computing Career
If you’re ready to embark on a career in cloud computing, follow these steps:
1. Understand the Basics
Start by learning the foundational concepts of cloud computing, such as virtualization, cloud deployment models, and service types (IaaS, PaaS, SaaS).
2. Get Hands-On Experience
Create free accounts on platforms like AWS, Azure, or Google Cloud to practice setting up virtual machines, deploying applications, and configuring storage.
3. Earn Certifications
Certifications demonstrate your expertise and enhance your resume. Popular certifications include:
AWS Certified Solutions Architect
Microsoft Azure Fundamentals
Google Cloud Professional Cloud Architect
4. Join a Training Program
Structured training programs can accelerate your learning and provide real-world experience. The Boston Institute of Analytics offers tailored Cloud Computing Course to prepare you for a successful career in cloud computing.
Why Choose the Boston Institute of Analytics for Cloud Computing And DevOps Course?
The Boston Institute of Analytics (BIA) is a globally renowned institution that specializes in training for emerging technologies. Their Cloud Computing Course is designed to equip learners with the skills needed to excel in cloud computing roles.
Highlights of the Cloud Training Institute
Comprehensive Curriculum
Covers critical topics like cloud architecture, DevOps, security, and data analytics.
Hands-On Practice
Offers practical projects and lab exercises to build real-world expertise.
Expert-Led Training
Learn from seasoned professionals with extensive experience in cloud computing and DevOps.
Certification Support
Helps you prepare for globally recognized certifications, enhancing your career prospects.
Career Services
Provides resume assistance, interview preparation, and job placement assistance to help you succeed.
Why Cloud Computing Careers Are Booming
The demand for cloud computing professionals is growing rapidly due to:
Digital Transformation
Organizations are adopting cloud technologies to improve agility and reduce costs.
Remote Work Trends
Cloud solutions enable seamless collaboration and remote access to resources.
Technological Innovations
Emerging technologies like AI, ML, and IoT heavily depend on cloud infrastructure.
Increased Focus on Security
As more businesses migrate to the cloud, the need for robust security measures continues to rise.
Final Thoughts
A career in cloud computing offers the chance to work with cutting-edge technologies and drive impactful projects. Whether your interests lie in engineering, architecture, DevOps, or data analytics, there’s a role for you in this dynamic field.
To get started, consider enrolling in the Boston Institute of Analytics' Cloud Computing Course. With their industry-aligned curriculum, hands-on projects, and expert mentorship, you’ll gain the skills and confidence needed to excel in the competitive world of cloud computing.
0 notes
Text
Best DBT Course in Hyderabad | Data Build Tool Training
What is DBT, and Why is it Used in Data Engineering?
DBT, short for Data Build Tool, is an open-source command-line tool that allows data analysts and engineers to transform data in their warehouses using SQL. Unlike traditional ETL (Extract, Transform, Load) processes, which manage data transformations separately, DBT focuses solely on the Transform step and operates directly within the data warehouse.
DBT enables users to define models (SQL queries) that describe how raw data should be cleaned, joined, or transformed into analytics-ready datasets. It executes these models efficiently, tracks dependencies between them, and manages the transformation process within the data warehouse. DBT Training
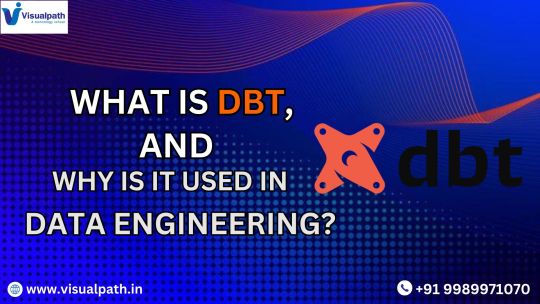
Key Features of DBT
SQL-Centric: DBT is built around SQL, making it accessible to data professionals who already have SQL expertise. No need for learning complex programming languages.
Version Control: DBT integrates seamlessly with version control systems like Git, allowing teams to collaborate effectively while maintaining an organized history of changes.
Testing and Validation: DBT provides built-in testing capabilities, enabling users to validate their data models with ease. Custom tests can also be defined to ensure data accuracy.
Documentation: With dbt, users can automatically generate documentation for their data models, providing transparency and fostering collaboration across teams.
Modularity: DBT encourages the use of modular SQL code, allowing users to break down complex transformations into manageable components that can be reused. DBT Classes Online
Why is DBT Used in Data Engineering?
DBT has become a critical tool in data engineering for several reasons:
1. Simplifies Data Transformation
Traditionally, the Transform step in ETL processes required specialized tools or complex scripts. DBT simplifies this by empowering data teams to write SQL-based transformations that run directly within their data warehouses. This eliminates the need for external tools and reduces complexity.
2. Works with Modern Data Warehouses
DBT is designed to integrate seamlessly with modern cloud-based data warehouses such as Snowflake, BigQuery, Redshift, and Databricks. By operating directly in the warehouse, it leverages the power and scalability of these platforms, ensuring fast and efficient transformations. DBT Certification Training Online
3. Encourages Collaboration and Transparency
With its integration with Git, dbt promotes collaboration among teams. Multiple team members can work on the same project, track changes, and ensure version control. The autogenerated documentation further enhances transparency by providing a clear view of the data pipeline.
4. Supports CI/CD Pipelines
DBT enables teams to adopt Continuous Integration/Continuous Deployment (CI/CD) workflows for data transformations. This ensures that changes to models are tested and validated before being deployed, reducing the risk of errors in production.
5. Focus on Analytics Engineering
DBT shifts the focus from traditional ETL to ELT (Extract, Load, Transform). With raw data already loaded into the warehouse, dbt allows teams to spend more time analyzing data rather than managing complex pipelines.
Real-World Use Cases
Data Cleaning and Enrichment: DBT is used to clean raw data, apply business logic, and create enriched datasets for analysis.
Building Data Models: Companies rely on dbt to create reusable, analytics-ready models that power dashboards and reports. DBT Online Training
Tracking Data Lineage: With its ability to visualize dependencies, dbt helps track the flow of data, ensuring transparency and accountability.
Conclusion
DBT has revolutionized the way data teams approach data transformations. By empowering analysts and engineers to use SQL for transformations, promoting collaboration, and leveraging the scalability of modern data warehouses, dbt has become a cornerstone of modern data engineering. Whether you are cleaning data, building data models, or ensuring data quality, dbt offers a robust and efficient solution that aligns with the needs of today’s data-driven organizations.
Visualpath is the Best Software Online Training Institute in Hyderabad. Avail complete Data Build Tool worldwide. You will get the best course at an affordable cost.
Attend Free Demo
Call on - +91-9989971070.
Visit: https://www.visualpath.in/online-data-build-tool-training.html
WhatsApp: https://www.whatsapp.com/catalog/919989971070/
Visit Blog: https://databuildtool1.blogspot.com/
#DBT Training#DBT Online Training#DBT Classes Online#DBT Training Courses#Best Online DBT Courses#DBT Certification Training Online#Data Build Tool Training in Hyderabad#Best DBT Course in Hyderabad#Data Build Tool Training in Ameerpet
0 notes
Text
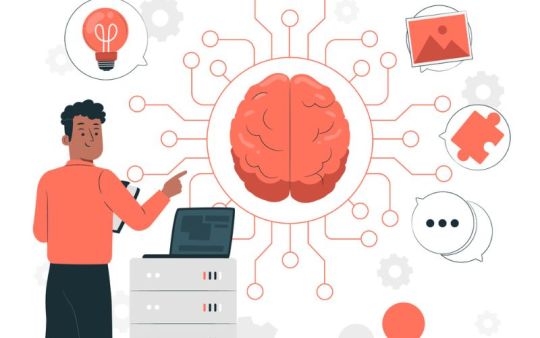
Global Decision Intelligence Market Industry Trends, Growth Insights, and Forecasts for 2032
Global decision intelligence market is projected to witness a CAGR of 17.51% during the forecast period 2025-2032, growing from USD 13.59 billion in 2024 to USD 49.41 billion in 2032.
The growth in the decision intelligence market is primarily driven by several technological advances and changing business needs, such as advanced generative AI models like GPT-4, which redefined the scope of natural language processing with its release in 2023. They are used to create coherent and contextually relevant text, which has been observed as a critical advancement in content creation applications and customer service functions. Their capacity to comprehend and create similar human responses has the potential to provide enhanced user experience through AI-based chatbots and virtual assistants. Furthermore, AI systems with multi-modal machine learning can process and integrate data from multiple sources, including text, images, and audio, to improve context and decision-making ability. For example, combining visual and audio data leads to better object recognition, which drives the decision intelligence market through important applications like virtual assistants and customer insights. Furthermore, TinyML is running machine learning algorithms locally at the edge of devices, which reduces latency and bandwidth usage as it processes data locally. It has appeared to increase efficiency for the users and even improve user privacy, mainly in the IoT landscape.
According to Oracle Corporation, 74% of businesses in 2023 observed that the number of daily decisions they make has risen tenfold in the past three years with the increasing complexity of decision-making. Hence, the growing need for effective decision-intelligence solutions to help people and businesses navigate their decision-making processes is further propelling the decision intelligence market.
For example, in May 2023, MDS Global Limited launched MDS DecisionAI, an AI-powered marketing decision intelligence platform that assists mobile network operators, mobile virtual network operators, and fixed-line operators in reducing churn and optimizing revenue strategies.
Demand For Data-Driven Decision-Making Promoting Growth in the Decision Intelligence Market
The growing need to make data-driven decisions is a key factor accelerating the decision intelligence market. Businesses, specifically in finance, healthcare, and retail, are gradually leaning on data to shape their strategic decisions. Thus, sophisticated decision intelligence tools with advanced technologies such as AI and machine learning are increasingly needed to analyze large datasets, actionable insights for improved operational efficiency, and new ways to engage customers. Organizations are putting in investments in strong data infrastructures and analytics capabilities to make effective use of data. Moreover, regulatory requirements are now coming with the essence of responsible data governance, compelling companies to use data-centric approaches. Integrating decision intelligence with emerging technologies, such as IoT and edge computing, helps organizations process data in real-time. Therefore, organizations can anticipate market trends and optimize their decision-making processes. Hence, the decision intelligence market is growing rapidly, as data is essential for shaping strategic business outcomes.
For example, in August 2024, Google Cloud LLC announced over 350 new data analytics innovations designed to enhance artificial intelligence use cases. It includes key updates such as Gemini integration in BigQuery, enhancements to Cloud Spanner, and real-time data-sharing capabilities.
Integration Of IoT and Automation Fueling the Decision Intelligence Market
Integrating IoT and automation significantly enhances the decision intelligence market by enabling real-time data collection and analysis. IoT devices generate vast amounts of data, which streamline the decision-making process across various industries when combined with automation. This synergy allows organizations to quickly respond to changing conditions and optimize operations. Businesses can gain insights into customer behavior, operational efficiency, and market trends, facilitating informed decision-making by leveraging IoT data. Automation further enhances this process by reducing manual intervention, minimizing errors, and ensuring consistency in decision outcomes. The demand for decision-intelligence solutions that can analyze and interpret this data in real-time is growing as organizations are increasingly adopting IoT technologies. This trend is particularly evident in manufacturing, logistics, and retail sectors, where timely insights are crucial for maintaining competitive advantage. Consequently, the decision intelligence market is expected to experience substantial growth, driven by the need for integrated solutions that harness the power of IoT and automation to enhance business agility and responsiveness.
For example, in September 2024, ManageEngine, a division of Zoho Corporation, upgraded Analytics Plus to version 6.0, introducing “Spotlight,” an AI-driven recommendations engine that enhances decision-making by identifying IT inefficiencies and reducing insight time.
Government Initiatives Fostering Revenue in the Decision Intelligence Market
Government initiatives such as the United States Executive Order on Safe, Secure, and Trustworthy AI, the United Kingdom AI Safety Institute, and the European AI Act are highly contributing to revenue in the decision intelligence market. These initiatives create a conducive environment for the development and adoption of decision intelligence solutions by establishing ethical guidelines and regulatory frameworks. They encourage businesses to invest in AI technologies that comply with safety and ethical standards, thereby driving demand for innovative decision-making tools. Moreover, the focus on transparency and risk mitigation enhances trust in AI systems, leading to increased adoption across sectors such as defense, telecommunications, and healthcare. As organizations seek to align with these regulations, the market for decision intelligence solutions expands, resulting in substantial revenue growth and the emergence of new business opportunities.
For instance, in October 2024, the United States National Security Memorandum on AI reinforced the commitment to leveraging artificial intelligence for national security objectives. It highlights the necessity of responsible AI deployment to enhance decision intelligence while safeguarding civil liberties and promoting democratic integrity.
Banking, Financial Services, and Insurance Leading in Decision Intelligence Market
Banking, Financial Services, and Insurance segment are leading the decision intelligence market with the deployment of advanced analytics and artificial intelligence to enable operational efficiency and customer experience. BFSI firms capture a considerable market share utilizing data-driven insights for risk management, fraud detection, and regulatory compliance. Big data analytics allows these institutions to process vast datasets, giving them valuable insights that inform their strategic decisions. Cloud computing infrastructure also supports scalable and cost-effective data management, which enables real-time analytics. Moreover, personalized services focus on tailoring financial products according to the needs of individual customers, ensuring loyalty and satisfaction. The use of machine learning algorithms for integration enhances predictive capabilities, ensuring proactive decision-making in complex financial environments.
North America Dominates Decision Intelligence Market Share
North America is leading the decision intelligence market owing to the few key contributions from major participants. These contributions include fostering cultures of innovation that emphasize new analytics and data-driven thinking. The region also has a highly skilled workforce for artificial intelligence and machine learning, which enhances the work on sophisticated decision intelligence tools and solution development. Moreover, the application of big data analytics and cloud computing enables organizations to effectively utilize vast amounts of data. This capability ensures real-time insights and agile decision-making, which are quite important in the fast-paced business environment.
Additionally, a supportive regulatory framework encourages investment in technology and innovation, facilitating the growth of decision intelligence applications across various sectors. The emphasis on digital transformation initiatives across industries further drives the adoption of decision intelligence, ensuring that organizations remain competitive and responsive to market changes.
For instance, in September 2024, Crimson Phoenix, LLC secured funding from the United States Army Medical Research and Development Command (USAMRDC) to develop advanced artificial intelligence algorithms, enhancing casualty care documentation in Tactical Combat Casualty Care (TCCC) environments.
Download Free Sample Report
Future Market Scenario (2025 – 2032F)
Organizations may increasingly adopt AI-driven autonomous systems that analyze data and make real-time decisions, especially in the finance and logistics sectors, thereby increasing efficiency.
The integration of decision intelligence with IoT data streams could allow the company to make well-informed decisions based on real-time insights from devices, enabling the improvement of predictive maintenance and customer engagement through connected IoT devices.
Growing concerns over data privacy may lead to the creation of ethical guidelines for AI deployment, where companies can demonstrate transparency and accountability to build trust.
The demand for customized decision intelligence platforms in various industries may increase, as companies can tailor algorithms based on specific needs.
Report Scope
“Decision Intelligence Market Assessment, Opportunities and Forecast, 2018-2032F”, is a comprehensive report by Markets and Data, providing in-depth analysis and qualitative and quantitative assessment of the current state of global decision intelligence market, industry dynamics, and challenges. The report includes market size, segmental shares, growth trends, opportunities, and forecast between 2025 and 2032. Additionally, the report profiles the leading players in the industry, mentioning their respective market share, business models, competitive intelligence, etc.
Click here for full report- https://www.marketsandata.com/industry-reports/decision-intelligence-market
Latest reports-
Contact
Mr. Vivek Gupta 5741 Cleveland street, Suite 120, VA beach, VA, USA 23462 Tel: +1 (757) 343–3258 Email: [email protected]
0 notes
Text
Google Colab vs. Google Data Studio: A Comprehensive Comparison
Google provides a suite of tools to address the diverse needs of data analysis, collaboration, and visualization. Among these, Google Colab (Google Colab) and Google Data Studio (datastudio.google.com) are two standout platforms. While both are robust, they cater to different functionalities and audiences. This article compares their unique features and use cases to help you determine which tool best suits your needs.
1. Purpose and Features
Google Colab
Purpose: Google Colab is a cloud-based coding platform for Python programming, primarily used for data analysis, machine learning, and computational tasks. It is akin to an online Jupyter Notebook.
Features:Write and execute Python code interactively.Pre-installed libraries like TensorFlow, NumPy, and Pandas.Access to GPUs and TPUs for high-performance computation.Real-time collaboration on shared notebooks.
Ideal For:Building and testing machine learning models.Exploring large datasets programmatically.Teaching and learning Python-based data science.
Like That:
FREE Instagram Private Profile Viewer Without Following
Private Instagram Viewer
This Is Link Style
https://colab.research.google.com/drive/1jL_ythMr1Ejk2c3pyvlno1EO1BBOtK_Z
https://colab.research.google.com/drive/1e9AxOP_ELN4SYSLhJW8b8KXFcM5-CavY
Google Data Studio
Purpose: Google Data Studio is a business intelligence tool that turns raw data into dynamic, visually appealing dashboards and reports.
Features:Seamlessly integrate with data sources such as Google Analytics, BigQuery, and Sheets.Create interactive, customizable reports and dashboards.Enable real-time updates for shared insights.
Ideal For:Visualizing marketing and business performance data.Crafting presentations for decision-making.Tracking KPIs and performance metrics efficiently.
Like That:
Free Instagram private account viewer
How to see private Instagram profiles
Private Instagram Viewer Apps
Recover hacked Instagram account
Recover hacked Snapchat account
Use Cases:
https://datastudio.google.com/embed/s/hqgxnNMpaBA
https://datastudio.google.com/embed/s/g8oLu_-1sNQ
2. Target Users
Google Colab
Targeted at data scientists, researchers, and developers proficient in Python.
Requires programming expertise for tasks such as algorithm development and data modeling.
Google Data Studio
Designed for business analysts, marketers, and decision-makers without coding knowledge.
Simplifies data interpretation through easy-to-use visual tools.
3. Data Access and Processing
Google Colab
Allows direct data manipulation using Python scripts.
Supports integrations with Google Drive, APIs, databases, and other sources.
Offers unparalleled flexibility for custom computations and workflows.
Google Data Studio
Focused on visualizing structured data from external sources like SQL databases or CSV files.
Limited in data transformation capabilities compared to coding tools like Colab.
4. Collaboration Capabilities
Google Colab
Enables simultaneous editing and execution of code in a shared notebook environment.
Perfect for team projects involving programming and analysis.
Google Data Studio
Supports collaborative creation and sharing of dashboards and reports.
Real-time updates ensure everyone is on the same page.
5. Performance and Scalability
Google Colab
Free tier provides basic compute resources, including limited GPU and TPU access.
Colab Pro plans offer enhanced runtimes and resource allocation for intensive tasks.
Google Data Studio
Scales efficiently for real-time data visualization.
Performance depends on the complexity of the report and connected data sources.
6. Cost and Accessibility
Google Colab
Free tier includes essential features for most users.
Paid Pro plans add advanced compute options for heavy workloads.
Google Data Studio
Free to use for creating reports and dashboards.
Some integrations, like BigQuery, may incur additional costs based on usage.
Google Colab vs. Google Data Studio: Understanding Their Differences
Understanding the Key Differences Between Google Colab and Google Data Studio
Conclusion
Both Google Colab and Google Data Studio are invaluable tools, but they serve different purposes. Google Colab is tailored for programmers and data scientists needing a flexible coding environment for analysis and machine learning. Conversely, Google Data Studio excels in creating visually engaging reports for business insights. Depending on your workflow, you might find value in using both—leveraging Colab for data preparation and analysis, and Data Studio for presenting insights to stakeholders.
0 notes
Text
Google Cloud (GCP): Revolutionizing Cloud Computing
In the rapidly evolving world of technology, Google Cloud (GCP) stands out as one of the most powerful and versatile cloud platforms. Businesses, developers, and learners are flocking to GCP to leverage its tools for building, deploying, and managing applications and services on a global scale. If you’re curious about what makes GCP a game-changer, read on as we explore its features, benefits, and why it’s becoming the go-to choice for cloud enthusiasts.
What is Google Cloud (GCP)?
Google Cloud (GCP) is a suite of cloud computing services offered by Google. It provides infrastructure as a service (IaaS), platform as a service (PaaS), and software as a service (SaaS) solutions. Businesses use GCP for hosting, data storage, machine learning, analytics, and app development, among other purposes.
With GCP, you get access to Google's world-class infrastructure, which powers its own services like Search, YouTube, and Gmail.
Why Choose Google Cloud (GCP)?
1. Cost-Effective Solutions
One of the biggest draws of Google Cloud (GCP) is its competitive pricing. Unlike traditional IT infrastructure that requires heavy upfront costs, GCP allows you to pay only for what you use. The pay-as-you-go model ensures businesses of all sizes can afford top-notch technology.
2. Scalability
With GCP, scaling up your business infrastructure is seamless. Whether you’re a startup experiencing rapid growth or a large enterprise needing more resources during peak times, GCP's scalable services ensure you always have enough capacity.
3. Security and Compliance
Google Cloud (GCP) offers advanced security features, including encryption, threat detection, and compliance certifications, making it one of the safest platforms for sensitive data.
4. Global Reach
With data centers in multiple regions, GCP offers high availability and low latency to ensure your applications perform optimally, no matter where your users are located.
Key Features of Google Cloud (GCP)
1. Compute Engine
Google Compute Engine is the backbone of GCP's infrastructure services. It provides virtual machines (VMs) with customizable configurations to match your needs.
2. Google Kubernetes Engine (GKE)
For businesses working with containers, GKE simplifies containerized application management, ensuring seamless deployment, scaling, and operation.
3. BigQuery
BigQuery is a fully managed data warehouse solution that enables businesses to analyze massive datasets in real time. It’s particularly useful for data-driven decision-making.
4. Cloud Storage
Google Cloud Storage offers highly reliable and durable solutions for data storage. Whether you’re storing personal files or enterprise-level data, GCP’s storage options can handle it all.
5. AI and Machine Learning Tools
With tools like Vertex AI and pre-trained ML models, Google Cloud (GCP) empowers businesses to integrate artificial intelligence into their processes with ease.
Top Use Cases for Google Cloud (GCP)
1. Website Hosting
Google Cloud Hosting is a popular choice for businesses looking to build scalable, secure, and fast-loading websites.
2. App Development
Developers use Google Cloud (GCP) for seamless app development and deployment. The platform supports multiple programming languages, including Python, Java, and Node.js.
3. Data Analytics
GCP’s data analytics tools make it easy to collect, process, and analyze large datasets, giving businesses actionable insights.
4. E-commerce
Many e-commerce businesses trust GCP to handle traffic spikes during sales and manage their databases efficiently.
Advantages of Learning Google Cloud (GCP)
For students and professionals, learning Google Cloud (GCP) offers numerous benefits:
1. High-Demand Skillset
With businesses migrating to the cloud, professionals skilled in GCP are in high demand.
2. Certification Opportunities
GCP certifications, such as the Google Cloud Associate Engineer and Google Cloud Professional Architect, add significant value to your resume.
3. Career Advancement
With knowledge of GCP, you can unlock roles in cloud engineering, data analytics, DevOps, and more.
GCP vs. Competitors: Why It Stands Out
While platforms like AWS and Microsoft Azure are well-established, Google Cloud (GCP) has carved its niche through unique offerings:
Superior Networking: Google’s private global fiber network ensures faster data transfers.
Advanced AI Tools: With Google’s leadership in AI, GCP provides unparalleled machine learning tools.
Simplified Billing: GCP's transparent and straightforward pricing appeals to many businesses.
How to Start with Google Cloud (GCP)?
1. Explore Free Resources
Google offers a free tier to help users get started with its services. This includes credits for popular tools like Compute Engine and BigQuery.
2. Enroll in GCP Courses
Platforms like Udemy provide in-depth Google Cloud (GCP) courses tailored for beginners and advanced learners.
3. Work on Projects
Hands-on experience is key to mastering GCP. Create projects, set up VMs, or analyze datasets to build confidence.
Future of Google Cloud (GCP)
The future of Google Cloud (GCP) looks incredibly promising. With continued investment in AI, edge computing, and multi-cloud solutions, GCP is well-positioned to lead the next wave of digital transformation.
Conclusion
In today’s cloud-centric world, Google Cloud (GCP) offers unmatched opportunities for businesses and individuals alike. Its robust features, affordability, and global reach make it a powerful choice for building and scaling applications. Whether you’re a business owner, developer, or student, learning and using Google Cloud (GCP) can transform your digital experience and career trajectory
0 notes
Text
Data engineering
The Backbone of Modern Analytics: Data Engineering in Practice
In an increasingly data-driven world, organizations are constantly leveraging the power of analytics to gain competitive advantages, enhance decision-making, and uncover valuable insights. However, the value of data is only realized when it is structured, clean, and accessible — this is where data engineering comes into play. As the foundational discipline underpinning data science, machine learning, and business intelligence, data engineering is the unsung hero of modern analytics.
In this comprehensive blog, we’ll explore the landscape of data engineering: its definition, components, tools, challenges, and best practices, as well as its pivotal role in today’s digital economy.
What is Data Engineering?
Data engineering refers to the process of designing, building, and maintaining systems and architectures that allow large-scale data to be collected, stored, and analyzed. Data engineers focus on transforming raw, unstructured, or semi-structured data into structured formats that are usable for analysis and business.
Think of data engineering as constructing the "plumbing" of data systems: building pipelines to extract data from various sources, ensuring data quality, transforming it into a usable state, and loading it into systems where analysts and data scientists can access it easily.
The Core Components of Data Engineering
1. Data Collection and Ingestion
Data engineers start by collecting data from various sources like databases, APIs, files, IoT devices, and other third-party systems. Data ingestion is the term given for this process. The incorporation of different systems forms the basis of data ingestion with consistent and efficient importation into centralized repositories.
2. Data Storage
Once consumed, data has to be stored in systems that are scalable and accessible. Data engineers will decide whether to use conventional relational databases, distributed systems such as Hadoop, or cloud-based storage solutions, such as Amazon S3 or Google Cloud Storage. Depending on the volume, velocity, and variety of the data, the choice is made Raw data is rarely usable in its raw form. Data transformation involves cleaning, enriching, and reformatting the data to make it analysis-ready. This process is encapsulated in the ETL (Extract, Transform, Load) or ELT (Extract, Load, Transform) pipelines.
4. Data Pipelines
At the heart of data engineering are data pipelines that automate the movement of data between systems. These can be designed to handle either real-time (streaming) or batch data, based on the use case.
5. Data Quality and Governance
To obtain reliable analytics, the data must be correct and consistent. Data engineers put in validation and deduplication processes and ensure standardization with proper adherence to data governance standards such as GDPR or CCPA.
6. Data Security
Data is a very important business resource, and safeguarding it must be a data engineer's core responsibility. They therefore use encryption, access controls, and other security measures over sensitive information.
Common Tools in Data Engineering
Data engineering has seen lots of change in recent history, with numerous tools having emerged to tackle various themes in the discipline. Following are some of the leading tools:
1. Data Ingestion Tools
Apache Kafka: A distributed event streaming platform ideal for real-time ingestion.
Apache Nifi: Simplifies the movement of data between systems.
Fivetran and Stitch: Cloud-based tools for ETL pipelines.
2. Data Storage Solutions
Relational Databases: MySQL, PostgreSQL, and Microsoft SQL Server.
Distributed Systems: Apache HDFS, Amazon S3, and Google BigQuery.
NoSQL Databases: MongoDB, Cassandra, and DynamoDB.
3. Data Processing Frameworks
Apache Spark: A unified analytics engine for large-scale data processing.
Apache Flink: Focused on stream processing.
Google Dataflow: A cloud-based service for batch and streaming data processing.
4. Orchestration Tools
Apache Airflow: Widely used for scheduling and managing workflows.
Prefect: A more recent alternative to Airflow, with a focus on flexibility.
Dagster: A platform for orchestrating complex data pipelines.
5. Cloud Ecosystems
AWS: Redshift, Glue, and EMR
Google Cloud: BigQuery, Dataflow, and Pub/Sub
Microsoft Azure: Synapse Analytics and Data Factory
The Role of Data Engineers in the Data Ecosystem
Data engineers play a very important role in the larger data ecosystem by working with other data professionals, including data scientists, analysts, and software engineers. Responsibilities include:
Enablement of Data Scientists: Ensuring that high-quality, well-organized data is available for modeling and machine learning tasks.
Enablement of Business Intelligence: Creating data models and warehouses that power dashboards and reports.
Scalability and Performance: Optimize systems for growing datasets with efficient delivery of real-time insights.
Building Resilient Architectures: Ensuring fault tolerance, disaster recovery, and scalability in data systems.
Challenges in Data Engineering
Data engineering is a challenge in its own right While data engineering is quite important, it's by no means without its problems:
1. Managing Data Volume, Velocity, and Variety
The exponential growth of data creates challenges in storage, processing, and integration. Engineers must design systems that scale seamlessly.
2. Data Quality Issues
Handling incomplete, inconsistent, or redundant data requires meticulous validation and cleansing processes.
3. Real-Time Processing
Real-time analytics demands low-latency systems, which can be difficult to design and maintain.
**4. Keeping Up with Technology
The pace of innovation in data engineering tools and frameworks requires continuous learning and adaptation.
5. Security and Compliance
Data security breaches and ever-changing regulations add complexity to building compliant and secure pipelines.
Best Practices in Data Engineering
To address these challenges, data engineers adhere to best practices that ensure reliable and efficient data pipelines:
Scalability Design: Use distributed systems and cloud-native solutions to manage large datasets.
Automation of Repetitive Tasks: Use tools like Airflow and Prefect for workflow automation.
Data Quality: Implement validation checks and error-handling mechanisms.
DevOps Principles: Use CI/CD pipelines for deploying and testing data infrastructure.
Document Everything: Maintain comprehensive documentation for pipelines, transformations, and schemas.
Collaborate Across Teams: Work with analysts and data scientists to get what they need and make it actionable.
The Future of Data Engineering
As the amount of data continues to explode, data engineering will only grow in importance. Some of the key trends that will shape the future are:
1. The Rise of DataOps
DataOps applies DevOps-like principles toward automation, collaboration, and process improvement in data workflows.
2. Serverless Data Engineering
Cloud providers increasingly offer serverless solutions, and engineers can focus on data rather than infrastructure.
3. Real-Time Data Pipelines
As IoT, edge computing, and event-driven architectures become more prominent, real-time processing is no longer the exception but the rule.
4. AI in Data Engineering
Machine learning is being incorporated into data engineering workflows to automate tasks like anomaly detection and schema mapping.
5. Unified Platforms Databricks and Snowflake, among others, are becoming unified platforms to simplify data engineering and analytics.
Why Data Engineering Matters
Companies that put strong data engineering into their practice reap big advantages:
Faster Time-to-Insights: Clean, accessible data facilitates quicker and more reliable decisions.
Stronger Data-Driven Culture: Well-structured data systems enable each member of the team to leverage data.
Cost Savings: Efficient pipelines reduce storage and processing costs.
Innovation Enablement: High-quality data fuels cutting-edge innovations in AI and machine learning.
Conclusion
Data engineering is the backbone of the modern data-driven world. It enables the organization to unlock the full potential of data by building the infrastructure that transforms raw data into actionable insights. The field certainly poses significant challenges, but strong data engineering practices bring great rewards, from enhanced analytics to transformative business outcomes.
As data continues to grow in scale and complexity, the role of data engineers will become even more crucial. Whether you’re an aspiring professional, a business leader, or a tech enthusiast, understanding the principles and practices of data engineering is key to thriving in today’s digital economy.
for more information visit our website
https:// researchpro.online/upcoming
0 notes
Text
Powering Innovation with Brillio and Google Cloud: Unleashing the Potential of AI and ML
In today’s rapidly evolving digital landscape, businesses face growing pressure to innovate, optimize processes, and deliver exceptional customer experiences. Artificial Intelligence (AI) and Machine Learning (ML) have emerged as game-changing technologies, driving transformative solutions across industries. At the forefront of this revolution is Brillio, leveraging its strategic partnership with Google Cloud to offer cutting-edge AI and ML solutions.
This blog dives into how Brillio’s expertise in collaboration with Google Cloud Platform (GCP) empowers businesses to unlock the true potential of GCP machine learning and GCP ML services.
Transforming Businesses with AI and ML
The potential of AI and ML goes far beyond automation. These technologies enable businesses to uncover insights, predict future trends, and enhance decision-making. However, implementing AI and ML can be complex, requiring the right tools, infrastructure, and expertise. This is where Brillio and its partnership with Google Cloud come into play.
Brillio specializes in designing customized AI and ML solutions that align with unique business needs. By leveraging the powerful capabilities of GCP machine learning, Brillio helps organizations tap into the full spectrum of possibilities offered by Google’s advanced cloud services.
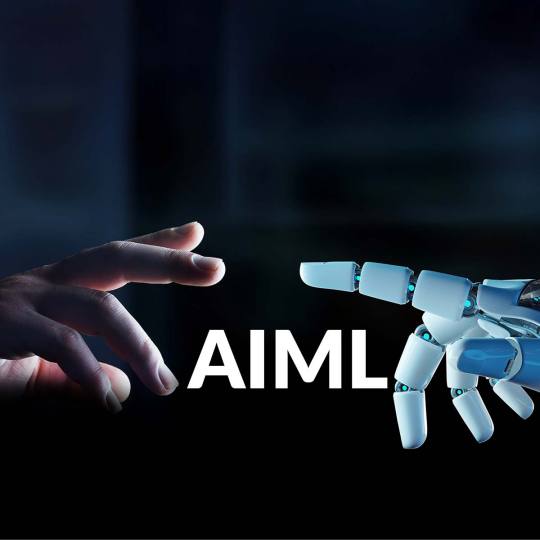
Why Google Cloud?
Google Cloud Platform is a leader in cloud computing, particularly in the AI and ML space. Its ecosystem of products and services is designed to support businesses in building scalable, secure, and innovative solutions. Let’s explore some of the key benefits of GCP ML services:
Pre-built Models for Faster Implementation: GCP offers pre-trained ML models like Vision AI and Translation AI, which can be deployed quickly for common use cases. Brillio ensures these tools are seamlessly integrated into your workflows to save time and resources.
Scalability and Performance: With GCP’s managed services like Vertex AI, businesses can train and deploy ML models efficiently, even at scale. Brillio’s expertise ensures optimal performance and cost-effectiveness for businesses of all sizes.
Data-Driven Insights: Leveraging BigQuery ML, GCP allows businesses to apply ML models directly within their data warehouses. This simplifies data analysis and speeds up decision-making processes. Brillio helps organizations make the most of these capabilities.
Secure Infrastructure: Google Cloud prioritizes data security and compliance, making it a trusted platform for industries like healthcare, finance, and retail. Brillio ensures that businesses adopt these services while maintaining the highest standards of security.
Brillio’s Approach to AI and ML on GCP
Brillio combines its domain expertise with GCP’s advanced technologies to create impactful AI and ML solutions. Here’s how Brillio drives success for its clients:
Customized Solutions: Brillio focuses on understanding a company’s unique challenges and tailors AI/ML implementations to solve them effectively.
Agile Delivery: By using an agile methodology, Brillio ensures quick deployment and iterative improvements to deliver value faster.
Seamless Integration: With a strong focus on user-centric design, Brillio ensures that AI and ML models are easily integrated into existing systems and processes.
Continuous Support: The journey doesn’t end with deployment. Brillio offers ongoing support to optimize performance and adapt to changing business needs.
Real-World Impact
Brillio’s partnership with Google Cloud has enabled countless organizations to achieve remarkable outcomes:
Retail Transformation: By leveraging GCP machine learning, Brillio helped a leading retailer implement personalized product recommendations, boosting sales and enhancing customer experience.
Predictive Analytics in Healthcare: Brillio empowered a healthcare provider with predictive models built using GCP ML services, enabling better patient outcomes through early intervention.
Supply Chain Optimization: A manufacturing client streamlined its supply chain with AI-driven demand forecasting, significantly reducing operational costs.
The Future of AI and ML with Brillio and GCP
As technology continues to advance, the potential applications of AI and ML will only grow. Brillio and Google Cloud remain committed to driving innovation and delivering transformative solutions for businesses worldwide.
Whether it’s predictive analytics, natural language processing, or advanced data analysis, Brillio ensures that companies harness the best of GCP machine learning and GCP ML services to stay ahead in a competitive market.
Conclusion
Brillio’s partnership with Google Cloud represents a powerful combination of expertise and innovation. By leveraging GCP machine learning and GCP ML services, businesses can unlock new possibilities, improve operational efficiency, and drive growth.
Are you ready to take your business to the next level with AI and ML? Partner with Brillio and Google Cloud today and transform your vision into reality.
Through strategic solutions and a relentless focus on customer success, Brillio and Google Cloud are paving the way for a smarter, more connected future.
#gcp machine learning#gcp cloud machine learning#machine learning gcp#machine learning in gcp#gcp ml services
0 notes
Text
Explain secure data sharing and use cases for cross-organization collaboration.
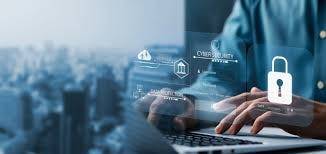
Secure Data Sharing and Use Cases for Cross-Organization Collaboration In today’s data-driven world, organizations frequently need to share data securely across departments, partners, vendors, and regulatory bodies.
Secure data sharing ensures that sensitive data is protected while enabling seamless collaboration, analytics, and decision-making.
🔐 What Is Secure Data Sharing?
Secure data sharing refers to controlled access to data across different organizations or business units without compromising security, privacy, or compliance.
Modern cloud platforms offer mechanisms that allow governed, permission-based sharing without physically moving or duplicating data.
Key Features of Secure Data Sharing:
✅ Access Control — Fine-grained permissions control who can access data.
✅ Encryption & Masking — Protects sensitive data in transit and at rest.
✅ Audit Logging — Tracks who accessed what data and when.
✅ Federated Identity Management — Ensures only authorized users access shared data.
✅ Multi-Cloud & Hybrid Support — Enables secure sharing across different cloud providers. 🛠 Technologies for Secure Data Sharing Various platforms offer secure data-sharing features, including:
1️⃣ Azure Synapse Analytics & Azure Data Share Azure Data Share enables organizations to share data without duplication. Synapse Analytics allows cross-team collaboration while enforcing access policies.
2️⃣ AWS Data Exchange & Lake Formation AWS Lake Formation provides fine-grained access control for shared data lakes. AWS Data Exchange allows businesses to subscribe to third-party data securely.
3️⃣ Snowflake Secure Data Sharing Enables real-time, zero-copy data sharing across organizations without moving data. Supports row- and column-level security for precise control.
4️⃣ Google BigQuery Data Sharing Uses authorized views and datasets for multi-tenant collaboration. 📌 Use Cases for Cross-Organization Collaboration
1️⃣ Enterprise Data Sharing Across Departments
📊 Use Case: A large multinational company wants to share HR, finance, and marketing data across different departments while maintaining strict access controls.
✅ Solution: Using Snowflake Secure Data Sharing or Azure Data Share, teams can access only the data they are authorized to see, eliminating the need for redundant data copies.
2️⃣ Partner & Vendor Collaboration
🔗 Use Case: A retail company wants to share real-time sales data with suppliers for demand forecasting.
✅ Solution: AWS Lake Formation enables secure data lake sharing, allowing suppliers to access live sales trends while maintaining customer privacy through data masking.
3️⃣ Data Monetization & Marketplace Models 💰 Use Case: A financial institution wants to sell anonymized transaction data to third-party analytics firms.
✅ Solution: AWS Data Exchange or Snowflake enables governed, controlled data sharing with external consumers without exposing personal details.
4️⃣ Healthcare & Research Collaboration
🏥 Use Case: Hospitals and pharmaceutical companies collaborate on medical research while complying with HIPAA & GDPR regulations.
✅ Solution: Federated data sharing models allow hospitals to run analytics on combined datasets without sharing raw patient records.
5️⃣ Regulatory & Compliance Reporting
📜 Use Case: Banks must share financial transaction data with regulators without violating privacy laws.
✅ Solution: Secure APIs with token-based authentication provide regulators with controlled access while maintaining an audit trail.
🔐 Best Practices for Secure Data Sharing
🔹 Role-Based Access Control (RBAC): Assign access rights based on user roles.
🔹 Data Masking & Anonymization: Protect sensitive PII data before sharing.
🔹 Encryption (TLS & AES-256): Encrypt data in transit and at rest.
🔹 Federated Authentication (OAuth, SAML): Ensure secure identity verification.
🔹 Zero Trust Architecture: Implement least privilege access policies.
🚀 Conclusion
Secure data sharing is crucial for modern enterprises looking to collaborate efficiently while protecting data privacy. Technologies like Azure Data Share, Snowflake, and AWS Lake Formation enable organizations to share data securely across departments, partners, and regulators.
By following best practices and using the right platforms, businesses can unlock secure, compliant, and scalable data collaboration.
0 notes
Text
How Does CAI Differ from CDI in Informatica Cloud?
Informatica Cloud is a powerful platform that offers various integration services to help businesses manage and process data efficiently. Two of its core components—Cloud Application Integration (CAI) and Cloud Data Integration (CDI)—serve distinct but complementary purposes. While both are essential for a seamless data ecosystem, they address different integration needs. This article explores the key differences between CAI and CDI, their use cases, and how they contribute to a robust data management strategy. Informatica Training Online
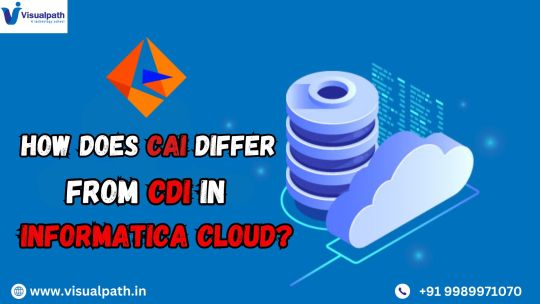
What is Cloud Application Integration (CAI)?
Cloud Application Integration (CAI) is designed to enable real-time, event-driven integration between applications. It facilitates communication between different enterprise applications, APIs, and services, ensuring seamless workflow automation and business process orchestration. CAI primarily focuses on low-latency and API-driven integration to connect diverse applications across cloud and on-premises environments.
Key Features of CAI: Informatica IICS Training
Real-Time Data Processing: Enables instant data exchange between systems without batch processing delays.
API Management: Supports REST and SOAP-based web services to facilitate API-based interactions.
Event-Driven Architecture: Triggers workflows based on system events, such as new data entries or user actions.
Process Automation: Helps in automating business processes through orchestration of multiple applications.
Low-Code Development: Provides a drag-and-drop interface to design and deploy integrations without extensive coding.
Common Use Cases of CAI:
Synchronizing customer data between CRM (Salesforce) and ERP (SAP).
Automating order processing between e-commerce platforms and inventory management systems.
Enabling chatbots and digital assistants to interact with backend databases in real time.
Creating API gateways for seamless communication between cloud and on-premises applications.
What is Cloud Data Integration (CDI)?
Cloud Data Integration (CDI), on the other hand, is focused on batch-oriented and ETL-based data integration. It enables organizations to extract, transform, and load (ETL) large volumes of data from various sources into a centralized system such as a data warehouse, data lake, or business intelligence platform.
Key Features of CDI: Informatica Cloud Training
Batch Data Processing: Handles large datasets and processes them in scheduled batches.
ETL & ELT Capabilities: Transforms and loads data efficiently using Extract-Transform-Load (ETL) or Extract-Load-Transform (ELT) approaches.
Data Quality and Governance: Ensures data integrity, cleansing, and validation before loading into the target system.
Connectivity with Multiple Data Sources: Integrates with relational databases, cloud storage, big data platforms, and enterprise applications.
Scalability and Performance Optimization: Designed to handle large-scale data operations efficiently.
Common Use Cases of CDI:
Migrating legacy data from on-premises databases to cloud-based data warehouses (e.g., Snowflake, AWS Redshift, Google BigQuery).
Consolidating customer records from multiple sources for analytics and reporting.
Performing scheduled data synchronization between transactional databases and data lakes.
Extracting insights by integrating data from IoT devices into a centralized repository.
CAI vs. CDI: Key Differences
CAI is primarily designed for real-time application connectivity and event-driven workflows, making it suitable for businesses that require instant data exchange. It focuses on API-driven interactions and process automation, ensuring seamless communication between enterprise applications. On the other hand, CDI is focused on batch-oriented data movement and transformation, enabling organizations to manage large-scale data processing efficiently.
While CAI is ideal for integrating cloud applications, automating workflows, and enabling real-time decision-making, CDI is better suited for ETL/ELT operations, data warehousing, and analytics. The choice between CAI and CDI depends on whether a business needs instant data transactions or structured data transformations for reporting and analysis. IICS Online Training
Which One Should You Use?
Use CAI when your primary need is real-time application connectivity, process automation, and API-based data exchange.
Use CDI when you require batch processing, large-scale data movement, and structured data transformation for analytics.
Use both if your organization needs a hybrid approach, where real-time data interactions (CAI) are combined with large-scale data transformations (CDI).
Conclusion
Both CAI and CDI play crucial roles in modern cloud-based integration strategies. While CAI enables seamless real-time application interactions, CDI ensures efficient data transformation and movement for analytics and reporting. Understanding their differences and choosing the right tool based on business needs can significantly improve data agility, process automation, and decision-making capabilities within an organization.
For More Information about Informatica Cloud Online Training
Contact Call/WhatsApp: +91-9989971070
Visit: https://www.visualpath.in/informatica-cloud-training-in-hyderabad.html
Visit Blog: https://visualpathblogs.com/category/informatica-cloud/
WhatsApp: https://www.whatsapp.com/catalog/919989971070/
#Informatica Training in Hyderabad#IICS Training in Hyderabad#IICS Online Training#Informatica Cloud Training#Informatica Cloud Online Training#Informatica IICS Training#Informatica Training Online#Informatica Cloud Training in Chennai#Informatica Cloud Training In Bangalore#Best Informatica Cloud Training in India#Informatica Cloud Training Institute#Informatica Cloud Training in Ameerpet
0 notes
Text
Dataplex Automatic Discovery & Cataloging For Cloud Storage
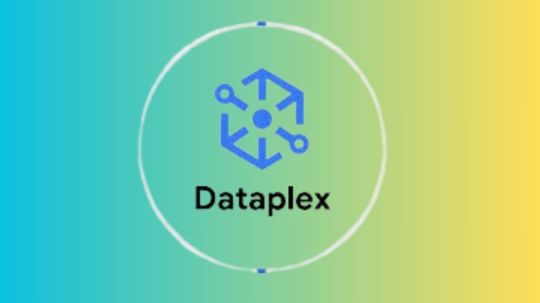
Cloud storage data is made accessible for analytics and governance with Dataplex Automatic Discovery.
In a data-driven and AI-driven world, organizations must manage growing amounts of structured and unstructured data. A lot of enterprise data is unused or unreported, called “dark data.” This expansion makes it harder to find relevant data at the correct time. Indeed, a startling 66% of businesses say that at least half of their data fits into this category.
Google Cloud is announcing today that Dataplex, a component of BigQuery’s unified platform for intelligent data to AI governance, will automatically discover and catalog data from Google Cloud Storage to address this difficulty. This potent potential enables organizations to:
Find useful data assets stored in Cloud Storage automatically, encompassing both structured and unstructured material, including files, documents, PDFs, photos, and more.
When data changes, you can maintain schema definitions current with integrated compatibility checks and partition detection to harvest and catalog metadata for your found assets.
With auto-created BigLake, external, or object tables, you can enable analytics for data science and AI use cases at scale without having to duplicate data or build table definitions by hand.
How Dataplex automatic discovery and cataloging works
The following actions are carried out by Dataplex Automatic Discovery and cataloging process:
With the help of the BigQuery Studio UI, CLI, or gcloud, users may customize the discovery scan, which finds and categorizes data assets in your Cloud Storage bucket containing up to millions of files.
Extraction of metadata: From the identified assets, pertinent metadata is taken out, such as partition details and schema definitions.
Database and table creation in BigQuery: BigQuery automatically creates a new dataset with multiple BigLake, external, or object tables (for unstructured data) with precise, current table definitions. These tables will be updated for planned scans as the data in the cloud storage bucket changes.
Preparation for analytics and artificial intelligence: BigQuery and open-source engines like Spark, Hive, and Pig can be used to analyze, process, and conduct data science and AI use cases using the published dataset and tables.
Integration with the Dataplex catalog: Every BigLake table is linked into the Dataplex catalog, which facilitates easy access and search.
Dataplex automatic discovery and cataloging Principal advantages
Organizations can benefit from Dataplex automatic discovery and cataloging capability in many ways:
Increased data visibility: Get a comprehensive grasp of your data and AI resources throughout Google Cloud, doing away with uncertainty and cutting down on the amount of effort spent looking for pertinent information.
Decreased human work: By allowing Dataplex to scan the bucket and generate several BigLake tables that match your data in Cloud Storage, you can reduce the labor and effort required to build table definitions by hand.
Accelerated AI and analytics: Incorporate the found data into your AI and analytics processes to gain insightful knowledge and make well-informed decisions.
Streamlined data access: While preserving the necessary security and control mechanisms, give authorized users simple access to the data they require.
Please refer to Understand your Cloud Storage footprint with AI-powered queries and insights if you are a storage administrator interested in managing your cloud storage and learning more about your whole storage estate.
Realize the potential of your data
Dataplex’s automated finding and cataloging is a big step toward assisting businesses in realizing the full value of their data. Dataplex gives you the confidence to make data-driven decisions by removing the difficulties posed by dark data and offering an extensive, searchable catalog of your Cloud Storage assets.
FAQs
What is “dark data,” and why does it pose a challenge for organizations?
Data that is unused or undetected in an organization’s systems is referred to as “dark data.” It presents a problem since it might impede well-informed decision-making and represents lost chances for insights.
How does Dataplex address the issue of dark data within Google Cloud Storage?
By automatically locating and cataloguing data assets in Google Cloud Storage, Dataplex tackles dark data and makes them transparent and available for analysis.
Read more on Govindhtech.com
#Dataplex#DataplexAutomatic#CloudStorage#AI#cloudcomputing#BigQuery#BigLaketable#News#Technews#Technology#Technologynews#Technologytrends#govindhtech
0 notes
Text
Is SQL Necessary for Cloud Computing?
As cloud computing continues to reshape the tech industry, many professionals and newcomers are curious about the specific skills they need to thrive in this field. A frequent question that arises is: "Is SQL necessary for cloud computing?" The answer largely depends on the role you’re pursuing, but SQL remains a highly valuable skill that can greatly enhance your effectiveness in many cloud-related tasks. Let’s dive deeper to understand the connection between SQL and cloud computing.
What Exactly is SQL?
SQL, or Structured Query Language, is a programming language designed for managing and interacting with relational databases. It enables users to:
Query data: Extract specific information from a database.
Update records: Modify existing data.
Insert data: Add new entries into a database.
Delete data: Remove unnecessary or outdated records.
SQL is widely adopted across industries, forming the foundation of countless applications that rely on data storage and retrieval.
A Quick Overview of Cloud Computing
Cloud computing refers to the on-demand delivery of computing resources—including servers, storage, databases, networking, software, and analytics—over the internet. It offers flexibility, scalability, and cost savings, making it an essential part of modern IT infrastructures.
Leading cloud platforms such as Amazon Web Services (AWS), Microsoft Azure, and Google Cloud Platform (GCP) provide robust database services that often rely on SQL. With data being central to cloud computing, understanding SQL can be a significant advantage.
Why SQL Matters in Cloud Computing
SQL plays a crucial role in several key areas of cloud computing, including:
1. Database Management
Many cloud providers offer managed database services, such as:
Amazon RDS (Relational Database Service)
Azure SQL Database
Google Cloud SQL
These services are built on relational database systems like MySQL, PostgreSQL, and SQL Server, all of which use SQL as their primary query language. Professionals working with these databases need SQL skills to:
Design and manage database structures.
Migrate data between systems.
Optimize database queries for performance.
2. Data Analytics and Big Data
Cloud computing often supports large-scale data analytics, and SQL is indispensable in this domain. Tools like Google BigQuery, Amazon Redshift, and Azure Synapse Analytics leverage SQL for querying and analyzing vast datasets. SQL simplifies data manipulation, making it easier to uncover insights and trends.
3. Cloud Application Development
Cloud-based applications often depend on databases for data storage and retrieval. Developers working on these applications use SQL to:
Interact with back-end databases.
Design efficient data models.
Ensure seamless data handling within applications.
4. Serverless Computing
Serverless platforms, such as AWS Lambda and Azure Functions, frequently integrate with databases. SQL is often used to query and manage these databases, enabling smooth serverless workflows.
5. DevOps and Automation
In DevOps workflows, SQL is used for tasks like database configuration management, automating deployments, and monitoring database performance. For instance, tools like Terraform and Ansible can integrate with SQL databases to streamline cloud resource management.
When SQL Might Not Be Essential
While SQL is incredibly useful, it’s not always a strict requirement for every cloud computing role. For example:
NoSQL Databases: Many cloud platforms support NoSQL databases, such as MongoDB, DynamoDB, and Cassandra, which do not use SQL.
Networking and Security Roles: Professionals focusing on areas like cloud networking or security may not use SQL extensively.
Low-code/No-code Tools: Platforms like AWS Honeycode and Google AppSheet enable users to build applications without writing SQL queries.
Even in these cases, having a basic understanding of SQL can provide added flexibility and open up more opportunities.
Advantages of Learning SQL for Cloud Computing
1. Broad Applicability
SQL is a universal language used across various relational database systems. Learning SQL equips you to work with a wide range of databases, whether on-premises or in the cloud.
2. Enhanced Career Prospects
SQL is consistently ranked among the most in-demand skills in the tech industry. Cloud computing professionals with SQL expertise are often preferred for roles involving data management and analysis.
3. Improved Problem-Solving
SQL enables you to query and analyze data effectively, which is crucial for troubleshooting and decision-making in cloud environments.
4. Stronger Collaboration
Having SQL knowledge allows you to work more effectively with data analysts, developers, and other team members who rely on databases.
How the Boston Institute of Analytics Can Help
The Boston Institute of Analytics (BIA) is a premier institution offering specialized training in Cloud Computing and DevOps. Their programs are designed to help students acquire the skills needed to excel in these fields, including SQL and its applications in cloud computing.
Comprehensive Learning Modules
BIA’s courses cover:
The fundamentals of SQL and advanced querying techniques.
Hands-on experience with cloud database services like Amazon RDS and Google Cloud SQL.
Practical training in data analytics tools like BigQuery and Redshift.
Integration of SQL into DevOps workflows.
Industry-Centric Training
BIA collaborates with industry experts to ensure its curriculum reflects the latest trends and practices. Students work on real-world projects and case studies, building a strong portfolio to showcase their skills.
Career Support and Certification
BIA offers globally recognized certifications that validate your expertise in Cloud Computing and SQL. Additionally, they provide career support services, including resume building, interview preparation, and job placement assistance.
Final Thoughts
So, is SQL necessary for cloud computing? While it’s not mandatory for every role, SQL is a critical skill for working with cloud databases, data analytics, and application development. It empowers professionals to manage data effectively, derive insights, and collaborate seamlessly in cloud environments.
If you’re aiming to build or advance your career in cloud computing, learning SQL is a worthwhile investment. The Boston Institute of Analytics offers comprehensive training programs to help you master SQL and other essential cloud computing skills. With their guidance, you’ll be well-prepared to excel in the ever-evolving tech landscape.
0 notes
Text
Integrating SAP ERP Data into Google BigQuery: Methods and Considerations
Introduction As organizations increasingly rely on cloud-based analytics, integrating enterprise data from SAP ERP systems like SAP ECC and SAP S/4HANA into Google Cloud Platform's (GCP) BigQuery is crucial. This integration enables advanced analytics, real-time insights, and improved decision-making. There are several methods to achieve this data ingestion, each with its own advantages and considerations. This POV explores four primary options: BigQuery Connector for SAP, Cloud Data Fusion integrations for SAP, exporting data through SAP Data Services, and replicating data using SAP Data Services and SAP LT Replication Server. image 1) BigQuery Connector for SAP 1.1 Overview The BigQuery Connector for SAP is a native integration tool designed to streamline the data transfer process from SAP systems to BigQuery. It facilitates direct connections, ensuring secure and efficient data pipelines. 1.2 Advantages - Seamless Integration: Native support ensures compatibility and ease of use. - Performance: Optimized for high throughput and low latency, enhancing data transfer efficiency. - Security: Leverages Google Cloud's security protocols, ensuring data protection during transit. 1.3 Considerations - Complexity: Initial setup might require expertise in both SAP and Google Cloud environments. - Cost: Potentially higher costs due to licensing and data transfer fees. 1.4 Use Cases - Real-time analytics where low latency is critical. - Organizations with existing investments in Google Cloud and BigQuery. 2) Cloud Data Fusion Integrations for SAP 2.1 Overview Cloud Data Fusion is a fully managed, cloud-native data integration service that supports building and managing ETL/ELT data pipelines. It includes various pre-built connectors for SAP data sources. Plugins and Their Details - SAP Ariba Batch Source - Source Systems: SAP Ariba - Capabilities: Extracts procurement data in batch mode. - Limitations: Requires API access and permissions; subject to API rate limits. - SAP BW Open Hub Batch Source - Source Systems: SAP Business Warehouse (BW) - Capabilities: Extracts data from SAP BW Open Hub destinations. - Limitations: Dependent on SAP BW Open Hub scheduling; complex configuration. - SAP OData - Source Systems: SAP ECC, SAP S/4HANA (via OData services) - Capabilities: Connects to SAP OData services for data extraction. - Limitations: Performance depends on OData service response times; requires optimized configuration. - SAP ODP (Operational Data Provisioning) - Source Systems: SAP ECC, SAP S/4HANA - Capabilities: Extracts data using the ODP framework for a consistent interface. - Limitations: Initial setup and configuration complexity. - SAP SLT Replication - Source Systems: SAP ECC, SAP S/4HANA - Capabilities: Real-time data replication to Google Cloud Storage (GCS). - Process: Data is first loaded into GCS, then into BigQuery. - Limitations: Requires SAP SLT setup; potential latency from GCS staging. - SAP SuccessFactors Batch Source - Source Systems: SAP SuccessFactors - Capabilities: Extracts HR and talent management data in batch mode. - Limitations: API rate limits; not suitable for real-time data needs. - SAP Table Batch Source - Source Systems: SAP ECC, SAP S/4HANA - Capabilities: Direct batch extraction from SAP tables. - Limitations: Requires table access authorization; batch processing latency. 2.2 Advantages - Low-code Interface: Simplifies ETL pipeline creation with a visual interface. - Scalability: Managed service scales with data needs. - Flexibility: Supports various data formats and integration scenarios. 2.3 Considerations - Learning Curve: Requires some learning to fully leverage features. - Google Cloud Dependency: Best suited for environments heavily using Google Cloud. 2.4 Use Cases - Complex ETL/ELT processes. - Organizations seeking a managed service to reduce operational overhead. 3) Export Data from SAP Systems to Google BigQuery through SAP Data Services 3.1 Overview SAP Data Services provides comprehensive data integration, transformation, and quality features. It can export data from SAP systems and load it into BigQuery. 3.2 Advantages - Comprehensive ETL Capabilities: Robust data transformation and cleansing features. - Integration: Seamlessly integrates with various SAP and non-SAP data sources. - Data Quality: Ensures high data quality through built-in validation and cleansing processes. 3.3 Considerations - Complexity: Requires skilled resources to develop and maintain data pipelines. - Cost: Additional licensing costs for SAP Data Services. 3.4 Use Cases - Complex data transformation needs. - Organizations with existing SAP Data Services infrastructure. 4) Replicating Data from SAP Applications to BigQuery through SAP Data Services and SAP SLT Replication Server 4.1 Overview Combines SAP Data Services with SAP LT Replication Server to provide real-time data replication using the ODP framework. 4.2 Detailed Process - SAP LT Replication Server with ODP Framework - Source Systems: SAP ECC, SAP S/4HANA. - Capabilities: Utilizes ODP framework for real-time data extraction and replication. - Initial Load and Real-Time Changes: Captures an initial data snapshot and subsequent changes in real-time. - Replication to ODP: Data is replicated to an ODP-enabled target. - Loading Data into Google Cloud Storage (GCS) - Data Transfer: Replicated data is staged in GCS. - Storage Management: GCS serves as an intermediary storage layer. - SAP Data Services - Extracting Data from GCS: Pulls data from GCS for further processing. - Transforming Data: Applies necessary transformations and data quality checks. - Loading into BigQuery: Final step involves loading processed data into BigQuery. 4.3 Advantages - Real-Time Data Availability: Ensures data in BigQuery is current. - Robust ETL Capabilities: Extensive features of SAP Data Services ensure high data quality. - Scalability: Utilizes Google Cloud’s scalable infrastructure. 4.4 Considerations - Complex Setup: Requires detailed configuration of SLT, ODP, and Data Services. - Resource Intensive: High resource consumption due to real-time replication and processing. - Cost: Potentially high costs for licensing and resource usage. 4.5 Use Cases - Real-time data analytics and reporting. - Scenarios requiring continuous data updates in BigQuery. Conclusion Each method for ingesting data from SAP ERP systems to GCP/BigQuery offers unique strengths and is suitable for different use cases. The BigQuery Connector for SAP is ideal for seamless, low-latency integration, while Cloud Data Fusion provides a scalable, managed solution for complex ETL needs with its various plugins. Exporting data via SAP Data Services is robust for comprehensive data transformation, and combining it with SAP LT Replication Server provides a powerful option for real-time data replication. Organizations should assess their specific requirements, existing infrastructure, and strategic goals to select the most suitable option for their data integration needs. Read the full article
0 notes