#AlexHammer
Explore tagged Tumblr posts
Link
Alex Hammer is a famous author. He wrote 14 Books that are based on the laws and secrets of success.
1 note
·
View note
Text
Alex Hammer
Alex Hammer | Founder and CEO at Ecommerce ROI.
Creating your most successful you to win.
Check out Alex Hammer's book "The Laws and Secrets of Success" here: https://amzn.to/2IH1cre
1 note
·
View note
Video
Beyoncé Beyoncé Beyoncé Beyoncé Beyoncé Beyoncé Beyoncé Beyoncé Beyoncé Beyoncé Beyoncé Beyoncé Beyoncé Beyoncé Beyoncé Beyoncé Beyoncé Beyoncé Beyoncé Beyoncé Beyoncé Beyoncé Beyoncé Beyoncé Beyoncé Beyoncé Beyoncé Beyoncé Beyoncé Beyoncé Beyoncé Beyoncé Beyoncé Beyoncé Beyoncé Beyoncé Beyoncé Beyoncé Beyoncé Beyoncé Beyoncé Beyoncé Beyoncé Beyoncé Beyoncé Beyoncé Beyoncé Beyoncé Beyoncé Beyoncé Beyoncé Beyoncé Beyoncé Beyoncé Beyoncé Beyoncé Beyoncé Beyoncé Beyoncé Beyoncé Beyoncé Beyoncé Beyoncé Beyoncé Beyoncé Beyoncé Beyoncé Beyoncé Beyoncé Beyoncé Beyoncé Beyoncé Beyoncé Beyoncé Beyoncé Beyoncé Beyoncé Beyoncé Beyoncé Beyoncé Beyoncé Beyoncé Beyoncé Beyoncé Beyoncé Beyoncé Beyoncé Beyoncé Beyoncé Beyoncé Beyoncé Beyoncé Beyoncé Beyoncé Beyoncé Beyoncé Beyoncé Beyoncé Beyoncé Beyoncé Beyoncé Beyoncé Beyoncé Beyoncé Beyoncé Beyoncé Beyoncé Beyoncé Beyoncé Beyoncé Beyoncé Beyoncé Beyoncé Beyoncé Beyoncé Beyoncé Beyoncé Beyoncé Beyoncé Beyoncé Beyoncé Beyoncé Beyoncé Beyoncé Beyoncé Beyoncé Beyoncé Beyoncé Beyoncé Beyoncé Beyoncé Beyoncé Beyoncé Beyoncé Beyoncé Beyoncé Beyoncé Beyoncé Beyoncé Beyoncé Beyoncé Beyoncé Beyoncé Beyoncé Beyoncé Beyoncé Beyoncé Beyoncé Beyoncé Beyoncé Beyoncé Beyoncé Beyoncé Beyoncé Beyoncé Beyoncé Beyoncé Beyoncé Beyoncé Beyoncé Beyoncé Beyoncé Beyoncé Beyoncé Beyoncé Beyoncé Beyoncé Beyoncé Beyoncé Beyoncé Beyoncé Beyoncé Beyoncé Beyoncé Beyoncé Beyoncé Beyoncé Beyoncé Beyoncé Beyoncé Beyoncé Beyoncé Beyoncé Beyoncé Beyoncé Beyoncé Beyoncé Beyoncé Beyoncé Beyoncé Beyoncé Beyoncé Beyoncé Beyoncé Beyoncé Beyoncé Beyoncé Beyoncé Beyoncé Beyoncé Beyoncé Beyoncé Beyoncé Beyoncé
2 notes
·
View notes
Video
This is Beyoncé's video for a fantastic song called "Countdown" and just love everything about it.
0 notes
Video
vimeo
M.I.A. - "Bring The Noize" (OFFICIAL VIDEO)
#Vimeo#bringthenoise#mdotidotadot#mathangiarulpragasam#alexhammer#alexanderhammer#goodcompany#jonathanlia#musicvideo
1 note
·
View note
Video
vimeo
M.I.A. - "Bring The Noize" (OFFICIAL VIDEO)
#Vimeo#bringthenoise#mdotidotadot#mathangiarulpragasam#alexhammer#alexanderhammer#goodcompany#jonathanlia#musicvideo
1 note
·
View note
Text
bikini
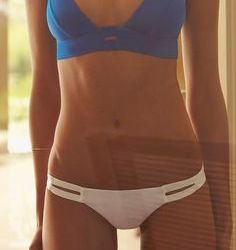
bikini Source/Repost=> https://www.pinterest.com/pin/819936675890026056/ ** Alex Hammer | Founder and CEO at Ecommerce ROI ** https://www.pinterest.com/creatingyourmostsuccessfulyout/
0 notes
Video
vimeo
This.
1 note
·
View note
Text
Getting started in Messenger Marketing – Chatbots Life
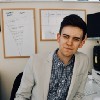
I’m regularly asked what Messenger marketing is, and exactly how it can benefit a business, so I’ve decided to write an article covering some of the main points, and showing you how you can get started today.
First of all, I want to point out that there are 1.3 billion FaceBook Messenger users, and not to mention millions more on FaceBook’s other messaging platforms.
And what’s most interesting to me is that those 1.3 billion users are having 7 billion conversations each day! That means there’s a hell of a lot of activity across FaceBook Messenger each day.
Platforms like FaceBook Messenger are the new trend for Social Media marketing, since they allow businesses to reach out to consumers on a large scale through advertisements, and can then interact with them on a more individual level. I believe that a well-designed Messenger chatbot can effectively do the work of an entire marketing department. Chatbot’s will allow you to provide instant responses to your customers questions, and alert real people when there’s a question that needs to be answered by a human.
Trending Articles on Chatbot Marketing:
1. How to Get to 1 Million Users for your Chatbot
2. How to Get Users for Free using a Viral Loop
3. How I grew JokeBot from 26k subscribers to 117k subscribers
4.Chatbot Conference in San Francisco
Why choose Messenger marketing?
When marketing a business or product, your aim should be to target your customers across the platforms that they are most active with. Messenger marketing will allow businesses to engage with customers and leads in a more conversational way than traditional email marketing. This is giving businesses an opportunity to develop personalised relationships with their customers.
Messenger Is Quickly Becoming A Mainstream Channel For Businesses!
When email first came out, email marketing was born, everyone was sending emails and this was a perfect opportunity for businesses to communicate with their customers. The same happened when phones became common, telemarketing came out. Today, people communicate across Messenger apps and the popularity with this channel keeps on growing, year by year!
How to use Messenger marketing
There are a number of ways in which you can use Messenger marketing, but I tend to break the different methods into the following:
Lead generation: Lead generation is one of the biggest reasons why many businesses are adopting Messenger marketing. Build out a marketing funnel which uses Messenger as a warm way to introduce your business to your target audience. For example, let’s say you’re a restaurant that wants to drive new people down to your business…
You could boost a FaceBook post promoting a special offer to new customers. You can then setup a Chatbot to automatically respond to people who leave a comment on the post with a specific keyword. You then run both Messenger ads and post engagement ads driving people to leave comments, or click into your Messenger to claim that offer. Each time this happens, the page can automatically respond with the offer.
You then have this user subscribed to your Messenger marketing list. This means you can keep running promotional messages to this user from your chosen bot platform, without having to pay for ads. It’s the same process as running an email campaign to your email subscribers.
Content delivery:
The majority of sites send new content to users through people who have opted in through email, but this is now something that you can do through Messenger. You can ask your users to optin through Messenger to be delivered new content through their inboxes.
Most bot platforms allow you to connect an RSS feed with your site, so for each time you post, it’s delivered into their inbox automatically.
You can also offer your users to search for specific content, by adding in some categories to your Chatbot. Tech Crunch has a great example for doing this.
One of the best features with content delivery is that you can personalise the content that you deliver to your users. For example, adding tags to your Messenger subscribers will allow you to fire more personalised/desired content.
Customer support:
As well as being able to provide your subscribers with instant customer support, there are many other ways of being able to support your customers with Messenger marketing.
One of my favourite ways is to achieve valuable feedback from your customers. If you’re running an event, broadcast messages to your attenders asking for feedback on how well the event went. This method is often used in eCommerce, as you can ask your customers to leave reviews on the products that they’ve purchased.
You’ll also find a much higher feedback response through Messenger rather than email!
Some interesting facts about Messenger marketing:
Messenger has a 4x higher open rate.
Messenger has an 8x-12.5x higher click-through rate.
Messenger Generates 1.6x more revenue.
Our latest client achieved 16K in table bookings
Our average Messenger campaign gets an open rate of 96%
We’ve helped a startup achieve 11,500 Messenger subscribers in a short period of time!
Start your first campaign today!
If you’ve got this far, then you may be considering a Messenger campaign. If that’s the case then I encourage you to continue with those thoughts! If you’re a little stuck, then here are some steps for launching your first Messenger marketing campaign:
Find a platform to build your Chatbot with. I’d recommend either ManyChat or ChatFuel. In these instructions I’ll talk about ManyChat. Create an account with them, and connect the bot to your page.
2. Create a post on FaceBook with a great offer. Think of something catchy that will instantly capture the attention of your audience. Tell them to comment a keyword which will enable them to claim their offer. The page will message those who comment with the relevant keyword. In this case I’ll use the keyword “interested”.
3. Go back to ManyChat, head over to the growth tools, click new growth tool and select the FaceBook comment tool.
4. Here’s where it may get tricky, I’ll write an in depth article about how to correctly set this up in a few days but, you basically want to select the post you have created with the offer in, and connect the comment tool. You want to setup the tool to only reply to users who have left a specific keyword in their comment. In this case, it’s for anyone who commented “interested”
You should see something that looks like this:
Make sure you’ve selected that checkbox below the “change post” button. Then click next.
5. Now create an opt in message. As your page is sending a message to someone who left a comment on your post, I always recommend putting something like:
“Hey Full Name! We’re messaging you because you left a comment on our recent post. Reply with “yes” to be sent the offer.”
When someone leaves a comment saying “yes” they become subscribed to your Messenger marketing list, because they’ve technically opted into your bot by leaving a reply.
6. When you reach the optin message section, you want to select “send only to users who reply with a specific keyword”. This is where you’ll enter the keyword “yes” in our case. You’ll then see a field which is your optin message.
Think of this as the response to the user, after they have replied with “yes”. For now, keep the default message in there, but remember what it’s called. You can then press save and activate.
Now whenever someone leaves a comment with “interested” and responds with “yes” the page will send across the offer, and also add the user to the pages Messenger subscriber list. Also, make sure that it’s set to active in the top left and not draft.
7. Now head to the flows tab. You should be able to see “optin messages”, click on this and select that optin message that you took note of before, when we setup the comment response. You need to click on this, and edit the flow.
Whatever text is inside of the flow will be what is sent to users who respond with “yes”. Once you’re finished with your message, click publish and now that response will be fired to the user once they have replied.
8. The very final step is to now boost that post you created earlier on. I’d recommend boosting it with the objective of post engagements, to drive the most comments for the budget that you have. Make sure you’re targeting the right people, and you’ll soon start seeing comments.
You’ve now created your first Messenger ad!
This can go really advanced, such as subscribing these users to a specific sequence, or adding tags to them so you know where the leads have come from. If you’re a restaurant then you could even fire up a second message asking when they’d like to book a table.. the possibilities are endless!
Source link
Source/Repost=> http://technewsdestination.com/getting-started-in-messenger-marketing-chatbots-life/ ** Alex Hammer | Founder and CEO at Ecommerce ROI ** http://technewsdestination.com
0 notes
Text
YouTube’s Upcoming AR Ads Let You Try On Makeup During Beauty Vlogs
Try on makeup directly from the YouTube app with AR Beauty Try-On.
Hot on the heels of their recent announcement regarding the introduction of 3D AR models to Google search results, the company revealed this week the arrival of AR Beauty Try-On, a new augmented reality-powered feature for the official YouTube app that allows users to try-on makeup as they watch their favorite beauty vloggers.
Those who have spent at least a couple hours on YouTube can tell you that beauty channels run by vloggers who provide reviews, tips, and inspirations for hair, fashion, and cosmetic, account for a wide portion of YouTube’s 2 billion monthly active users. In an effort to provide a more personalized and effective experience for viewers as well as brands looking to advertise over specific content, AR Beauty Try-On enables viewers to try-on real cosmetic products in real-time without even having to pause the video.
Image Credit: Google / YouTube
So say you’re watching a popular beauty channel famous for its in-depth reviews of the hottest lipstick brands. If the channel has AR Beauty Try-On activated, instead of seeing a standard banner ad as you would normally, you’d instead be offered a second window in which to try-on a realistic AR rendition of the product being reviewed in the video above.
In a quiet test run conducted earlier this year alongside several prominent Beauty brands, Google claims 30% of viewers activated the augmented advertisements, with a majority spending around 80 seconds trying on AR lipstick. The first company taking part in the campaign will be M·A·C Cosmetics, which will offer a series of AR options when AR Beauty Try-On launches later this summer.
Creators interested in participating can join up using Famebit, YouTube’s branded content platform.
Image Credit: Google / YouTube
Along with the announcement of AR Beauty Try-On, Google is also introducing Swirl, their first immersive display format designed specifically for mobile web via Display & Video 360 which allows users to interact with advertisements in a variety of new ways. To assist brands with creating Swirl-based interactive advertisements, Google is also introducing a new editor to Google’s 3D platform, Poly. This new addition offers developers more creative control over 3D objects, allowing them to change animation settings, customize backgrounds, and add realistic reflections.
You can head over to Google’s official blog to learn more about these new features.
!function(f,b,e,v,n,t,s){if(f.fbq)return;n=f.fbq=function(){n.callMethod? n.callMethod.apply(n,arguments):n.queue.push(arguments)};if(!f._fbq)f._fbq=n; n.push=n;n.loaded=!0;n.version='2.0';n.queue=[];t=b.createElement(e);t.async=!0; t.src=v;s=b.getElementsByTagName(e)[0];s.parentNode.insertBefore(t,s)}(window, document,'script','https://connect.facebook.net/en_US/fbevents.js'); fbq('init', '597165077146323'); // Insert your pixel ID here. fbq('track', 'PageView');(function(d, s, id) { var js, fjs = d.getElementsByTagName(s)[0]; if (d.getElementById(id)) return; js = d.createElement(s); js.id = id; js.src = "http://connect.facebook.net/en_US/sdk.js#xfbml=1&appId=715429028587329&version=v2.3"; fjs.parentNode.insertBefore(js, fjs); }(document, 'script', 'facebook-jssdk')); Source link
Source/Repost=> http://technewsdestination.com/youtubes-upcoming-ar-ads-let-you-try-on-makeup-during-beauty-vlogs/ ** Alex Hammer | Founder and CEO at Ecommerce ROI ** http://technewsdestination.com
0 notes
Text
One-Shot Learning with Siamese Networks, Contrastive Loss, and Triplet Loss for Face Recognition
One-shot learning is a classification task where one, or a few, examples are used to classify many new examples in the future.
This characterizes tasks seen in the field of face recognition, such as face identification and face verification, where people must be classified correctly with different facial expressions, lighting conditions, accessories, and hairstyles given one or a few template photos.
Modern face recognition systems approach the problem of one-shot learning via face recognition by learning a rich low-dimensional feature representation, called a face embedding, that can be calculated for faces easily and compared for verification and identification tasks.
Historically, embeddings were learned for one-shot learning problems using a Siamese network. The training of Siamese networks with comparative loss functions resulted in better performance, later leading to the triplet loss function used in the FaceNet system by Google that achieved then state-of-the-art results on benchmark face recognition tasks.
In this post, you will discover the challenge of one-shot learning in face recognition and how comparative and triplet loss functions can be used to learn high-quality face embeddings.
After reading this post, you will know:
One-shot learning are classification tasks where many predictions are required given one (or a few) examples of each class, and face recognition is an example of one-shot learning.
Siamese networks are an approach to addressing one-shot learning in which a learned feature vector for the known and candidate example are compared.
Contrastive loss and later triplet loss functions can be used to learn high-quality face embedding vectors that provide the basis for modern face recognition systems.
Let’s get started.
One-Shot Learning with Siamese Networks, Contrastive, and Triplet Loss for Face Recognition Photo by Heath Cajandig, some rights reserved.
Overview
This tutorial is divided into four parts; they are:
One-Shot Learning and Face Recognition
Siamese Network for One-Shot Learning
Contrastive Loss for Dimensionality Reduction
Triplet Loss for Learning Face Embeddings
One-Shot Learning and Face Recognition
Typically, classification involves fitting a model given many examples of each class, then using the fit model to make predictions on many examples of each class.
For example, we may have thousands of measurements of plants from three different species. A model can be fit on these examples, generalizing from the commonalities among the measurements for a given species and contrasting differences in the measurements across species. The result, hopefully, is a robust model that, given a new set of measurements in the future, can accurately predict the plant species.
One-shot learning is a classification task where one example (or a very small number of examples) is given for each class, that is used to prepare a model, that in turn must make predictions about many unknown examples in the future.
In the case of one-shot learning, a single exemplar of an object class is presented to the algorithm.
— Knowledge transfer in learning to recognize visual objects classes, 2006.
This is a relatively easy problem for humans. For example, a person may see a Ferrari sports car one time, and in the future, be able to recognize Ferraris in new situations, on the road, in movies, in books, and with different lighting and colors.
Humans learn new concepts with very little supervision – e.g. a child can generalize the concept of “giraffe” from a single picture in a book – yet our best deep learning systems need hundreds or thousands of examples.
— Matching Networks for One Shot Learning, 2017.
One-shot learning is related to but different from zero-shot learning.
This should be distinguished from zero-shot learning, in which the model cannot look at any examples from the target classes.
— Siamese Neural Networks for One-shot Image Recognition, 2015.
Face recognition tasks provide examples of one-shot learning.
Specifically, in the case of face identification, a model or system may only have one or a few examples of a given person’s face and must correctly identify the person from new photographs with changes to expression, hairstyle, lighting, accessories, and more.
In the case of face verification, a model or system may only have one example of a persons face on record and must correctly verify new photos of that person, perhaps each day.
As such, face recognition is a common example of one-shot learning.
Want Results with Deep Learning for Computer Vision?
Take my free 7-day email crash course now (with sample code).
Click to sign-up and also get a free PDF Ebook version of the course.
Download Your FREE Mini-Course
Siamese Network for One-Shot Learning
A network that has been popularized given its use for one-shot learning is the Siamese network.
A Siamese network is an architecture with two parallel neural networks, each taking a different input, and whose outputs are combined to provide some prediction.
It is a network designed for verification tasks, first proposed for signature verification by Jane Bromley et al. in the 2005 paper titled “Signature Verification using a Siamese Time Delay Neural Network.”
The algorithm is based on a novel, artificial neural network, called a “Siamese” neural network. This network consists of two identical sub-networks joined at their outputs.
— Signature Verification using a “Siamese” Time Delay Neural Network, 2005.
Two identical networks are used, one taking the known signature for the person, and another taking a candidate signature. The outputs of both networks are combined and scored to indicate whether the candidate signature is real or a forgery.
Verification consists of comparing an extracted feature vector with a stored feature vector for the signer. Signatures closer to this stored representation than a chosen threshold are accepted, all other signatures are rejected as forgeries.
— Signature Verification using a “Siamese” Time Delay Neural Network, 2005.
Example of a Siamese Network for Signature Verification. Taken from: Signature Verification using a “Siamese” Time Delay Neural Network.
Siamese networks were used more recently, where deep convolutional neural networks were used in parallel image inputs in a 2015 paper by Gregory Koch, et al. titled “Siamese Neural Networks for One-Shot Image Recognition.”
The deep CNNs are first trained to discriminate between examples of each class. The idea is to have the models learn feature vectors that are effective at extracting abstract features from the input images.
Example of Image Verification Used to Train a Siamese Network. Taken from: Siamese Neural Networks for One-Shot Image Recognition.
The models are then re-purposed for verification to predict whether new examples match a template for each class.
Specifically, each network produces a feature vector for an input image, which are then compared using the L1 distance and a sigmoid activation. The model was applied to benchmark handwritten character datasets used in computer vision.
Example of One-Shot Image Classification Used to Test a Siamese Network. Taken from: Siamese Neural Networks for One-Shot Image Recognition.
The Siamese Network is interesting for its approach to solving one-shot learning by learning feature representations (feature vectors) that are then compared for verification tasks.
An example of a face recognition system that was developed using a Siamese Network is DeepFace, described by Yaniv Taigman, et al. in the 2014 paper titled “DeepFace: Closing the Gap to Human-Level Performance in Face Verification.”
Their approach involved first training the model for face identification, then removing the classifier layer of the model and using the activations as a feature vector that were then calculated and compared for two different faces for face verification.
We have also tested an end-to-end metric learning approach, known as Siamese network: once learned, the face recognition network (without the top layer) is replicated twice (one for each input image) and the features are used to directly predict whether the two input images belong to the same person.
— DeepFace: Closing the Gap to Human-Level Performance in Face Verification, 2014.
Contrastive Loss for Dimensionality Reduction
Learning a vector representation of a complex input, like an image, is an example of dimensionality reduction.
Dimensionality reduction aims to translate high dimensional data to a low dimensional representation such that similar input objects are mapped to nearby points on a manifold.
— Dimensionality Reduction by Learning an Invariant Mapping, 2006.
The goal of effective dimensionality reduction is to learn a new lower dimensional representation that preserves the structure of the input such that distances between output vectors meaningfully capture the differences in the input. Yet, the vectors must capture the invariant features in the input.
The problem is to find a function that maps high dimensional input patterns to lower dimensional outputs, given neighborhood relationships between samples in input space.
— Dimensionality Reduction by Learning an Invariant Mapping, 2006.
Dimensionality reduction is the approach that Siamese networks use to address one-shot learning.
In their 2006 paper titled “Dimensionality Reduction by Learning an Invariant Mapping,” Raia Hadsell, et al. explore using a Siamese network for dimensionality reduction with convolutional neural networks with image data and propose training the models via contrastive loss.
Unlike other loss functions that may evaluate the performance of a model across all input examples in the training dataset, contrastive loss is calculated between pairs of inputs, such as between the two inputs provided to a Siamese network.
Pairs of examples are provided to the network, and the loss function penalizes the model differently based on whether the classes of the samples are the same or different. Specifically, if the classes are the same, the loss function encourages the models to output feature vectors that are more similar, whereas if the classes differ, the loss function encourages the models to output feature vectors that are less similar.
The contrastive loss requires face image pairs and then pulls together positive pairs and pushes apart negative pairs. […] However, the main problem with the contrastive loss is that the margin parameters are often difficult to choose.
— Deep Face Recognition: A Survey, 2018.
The loss function requires that a margin is selected that is used to determine the limit to which examples from different pairs are penalized. Choosing this margin requires careful consideration and is one downside of using the loss function.
Plot of Contrastive Loss Calculation for Similar (red) and Dissimilar (blue) Pairs. Taken From: Dimensionality reduction by learning an invariant mapping
Contrastive loss can be used to train a face recognition system, specifically for the task of face verification. Further, this can be achieved without the need for parallel models used in the Siamese network architecture by providing pairs of examples sequentially and saving the predicted feature vectors before calculating the loss and updating the model.
An example is the DeepID2 and subsequent systems (DeepID2+ and DeepID3) that used deep convolutional neural networks, but not a Siamese network architecture, and achieved then state-of-the-art results on benchmark face recognition datasets.
The verification signal directly regularize DeepID2 and can effectively reduce the intra-personal variations. Commonly used constraints include the L1/L2 norm and cosine similarity. We adopt the following loss function based on the L2 norm, which was originally proposed by Hadsell et al. for dimensionality reduction.
— Deep Learning Face Representation by Joint Identification-Verification, 2014.
Triplet Loss for Learning Face Embeddings
The idea of comparative loss can be further extended from two examples to three, called triplet loss.
Triplet loss was introduced by Florian Schroff, et al. from Google in their 2015 paper titled “FaceNet: A Unified Embedding for Face Recognition and Clustering.”
Rather than calculating loss based on two examples, triplet loss involves an anchor example and one positive or matching example (same class) and one negative or non-matching example (differing class).
The loss function penalizes the model such that the distance between the matching examples is reduced and the distance between the non-matching examples is increased.
It requires the face triplets, and then it minimizes the distance between an anchor and a positive sample of the same identity and maximizes the distance between the anchor and a negative sample of a different identity.
— Deep Face Recognition: A Survey, 2018.
Example of The Effect on Anchor, Positive, and Negative Both Before and After Applying Triplet Loss. Taken from: Facenet: A unified embedding for face recognition and clustering.
The result is a feature vector, referred to as a ‘face embedding,’ that has a meaningful Euclidean relationship, such that similar faces produce embeddings that have small distances (e.g. can be clustered) and different examples of the same face produce embeddings that are very small and allow verification and discrimination from other identities.
This approach is used as the basis behind the FaceNet system that achieved then state-of-the-art results on benchmark face recognition datasets.
In this paper we present a system, called FaceNet, that directly learns a mapping from face images to a compact Euclidean space where distances directly correspond to a measure of face similarity.
— Facenet: A unified embedding for face recognition and clustering, 2015.
The triplets that are used to train the model are carefully chosen.
Triplets that are easy, result in a small loss, and are not effective at updating the model. Instead, hard triplets are sought that encourage changes to the model and the predicted face embeddings.
Choosing which triplets to use turns out to be very important for achieving good performance and, inspired by curriculum learning, we present a novel online negative exemplar mining strategy which ensures consistently increasing difficulty of triplets as the network trains.
— Facenet: A unified embedding for face recognition and clustering, 2015.
Triplets are generated in an online manner, and so-called hard positive (matching) and hard negative (non-matching) cases are found and used in the estimate of the loss for the batch.
It is crucial to select hard triplets, that are active and can therefore contribute to improving the model.
— Facenet: A unified embedding for face recognition and clustering, 2015.
The approach of directly training face embeddings, such as via triplet loss, and using the embeddings as the basis for face identification and face verification models, such as FaceNet, is the basis for modern and state-of-the-art methods for face recognition.
… for models trained from scratch as well as pretrained ones, using a variant of the triplet loss to perform end-to-end deep metric learning outperforms most other published methods by a large margin.
— In Defense of the Triplet Loss for Person Re-Identification, 2017.
Further Reading
This section provides more resources on the topic if you are looking to go deeper.
Papers
Knowledge transfer in learning to recognize visual objects classes, 2006.
Matching Networks for One Shot Learning, 2017.
Siamese Neural Networks for One-shot Image Recognition, 2015.
Signature Verification using a “Siamese” Time Delay Neural Network, 2005.
DeepFace: Closing the Gap to Human-Level Performance in Face Verification, 2014.
Dimensionality Reduction by Learning an Invariant Mapping, 2006.
Deep Face Recognition: A Survey, 2018.
Deep Learning Face Representation by Joint Identification-Verification, 2014.
Facenet: A unified embedding for face recognition and clustering, 2015.
In Defense of the Triplet Loss for Person Re-Identification, 2017.
Articles
Summary
In this post, you discovered the challenge of one-shot learning in face recognition and how comparative and triplet loss functions can be used to learn high-quality face embeddings.
Specifically, you learned:
One-shot learning are classification tasks where many predictions are required given one (or a few) examples of each class, and face recognition is an example of one-shot learning.
Siamese networks are an approach to addressing one-shot learning in which a learned feature vector for the known and candidate example are compared.
Contrastive loss and later triplet loss functions can be used to learn high-quality face embedding vectors that provide the basis for modern face recognition systems.
Do you have any questions? Ask your questions in the comments below and I will do my best to answer.
Develop Deep Learning Models for Vision Today!
Develop Your Own Vision Models in Minutes
…with just a few lines of python code
Discover how in my new Ebook: Deep Learning for Computer Vision
It provides self-study tutorials on topics like: classification, object detection (yolo and rcnn), face recognition (vggface and facenet), data preparation and much more…
Finally Bring Deep Learning to your Vision Projects
Skip the Academics. Just Results.
Click to learn more.
Source link
Source/Repost=> http://technewsdestination.com/one-shot-learning-with-siamese-networks-contrastive-loss-and-triplet-loss-for-face-recognition/ ** Alex Hammer | Founder and CEO at Ecommerce ROI ** http://technewsdestination.com
0 notes
Text
Watch 20 Minutes of Asgard’s Wrath Gameplay – Road to VR
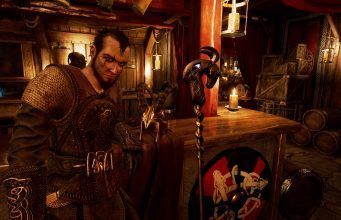
Sanzaru Games and Oculus Studios showed off a new demo for their upcoming melee adventure Asgard’s Wrath at this year’s E3. We got a chance to not only jump in to see more of the game’s questing, but also get a feel for the scope of the world ahead.
I had around 20 minutes in the new demo—only just enough to sample other important components of the game besides rote combat, which I saw a fair bit of in my first hands-on at GDC in March.
This time around I got to create chimeric buddies from the world’s beasts, solve light puzzles while dungeoning, and accumulate loot for crafting along the way. What most impressed me about the game though was its visual fidelity and rich environments, something I hope to explore more during the game’s purported 30+ hour duration.
If you’re short on viewing time, check out my article ‘Asgard’s Wrath Shows Titanic Ambition & Visual Finesse’ for the play-by-play and my impressions of the E3 demo.
Asgard’s Wrath is slated to arrive exclusively on Rift sometime in 2019.
youtube
Source link
Source/Repost=> http://technewsdestination.com/watch-20-minutes-of-asgards-wrath-gameplay-road-to-vr/ ** Alex Hammer | Founder and CEO at Ecommerce ROI ** http://technewsdestination.com
0 notes
Text
How to make a million dollars (with advice from actual millionaires)
‘How to make a million dollars’ has been plastered on book covers, typed millions of times in Google and is, generally speaking, one of the biggest goals for entrepreneurs, usually with a time window like 1 year, . Everyone loves to give money advice, and 9/10 times, it’s not good advice. Why? Whoever gave it to you could have the best intentions for you when they give it, but unless they’ve actually amassed that amount, it all comes being nothing more than second or thirdhand information at best.
That’s why I wanted to bring you expert insights from not one, but two women who have made over a million dollars in their respective businesses, and their advice likely flies in the face of everything else you’ve been told or read.
Click any link below or scroll down to read the entire post:
Meet our millionaires: Luisa Zhou & Shannon Badger
Luisa Zhou
Luisa had a great job with a healthy six-figure income, but she decided one day that she wanted more.
That’s when she started her first side hustle consulting people in digital advertising and now teaching aspiring entrepreneurs how to launch their own business the right way.
She’s since scaled her business and was able to earn a whopping $1.1 million in sales in less than 11 months. You can read about her journey here, on our sister site GrowthLab.
Shannon Badger
With three kids to take care of, Shannon knew she had to really learn the art of the grind in order to quit her job and take her freelancing seriously.
That’s how she was able to grow her freelance CPA consulting hustle into a full-fledged business (Badger and Badger CPA) that she runs with her husband. It’s helped earn her her first million dollar year in 2017.
“It was a huge mentality shift to how much can I ramp it up while still working full time in my other job,” she says. “There’s a quote by Dave Ramsey that goes: ‘Live like no one else, so you later can live like no one else.’ My husband and I were really disciplined when we got started, and it made the process less difficult.”
And now, these two millionaires are going to divulge their strategies on how they got to where they are — and how you can do it too.
How to make a million dollars
Remember, our two millionaires were able to earn their money through a powerful combination of two things:
Investing and saving
Earning more money through side hustles
Though you can actually make a million dollars on investing and saving alone, you can watch your net worth explode if you combine them both — which I suggest you do.
Part I: Investing and saving
Step 0: Get out of debt
The number one barrier preventing people from living a Rich Life is debt.
That’s why this is Step 0. Before you even think about investing, saving, or earning more money, you need to take steps to get out of credit card debt.
“A [freelancing business] is not the solution to your money problems,” Luisa says. “If you’re struggling to pay your bills or are in debt, the first thing you want to do is get a job that can help you. That’s the easiest way to really help you first.”
That’s why getting out of debt was also a priority for Shannon when she and her husband/business partner first got married.
“When my husband and I were first married, we were really disciplined about getting out of debt and saving,” Shannon says. “We paid off all of our debt out of college. We paid off all of our car debt. And now we’re paying off our house.”
Paying off your credit card debt is one of the most important investments you can make into your Rich Life. I’ve written extensively about this before, both on the blog and in my New York Times bestselling book.
If you’re in debt and want to learn more about my system, I highly suggest you read my article on how to get out of debt fast.
Step 1: Save money for when you need it most
By saving money, you give yourself the freedom to earn more money.
That’s why it’s important to set savings goals.
To find out how much you need in your emergency savings fund, you simply have to take into account three to six months worth of:
Utility bills (internet, water, electricity, etc)
Rent
Car/home payments
Food/groceries
Basically, any living expense that you have should be accounted for.
You should also start an account exclusively for your emergency savings fund. Most banks allow you to create a sub-savings account along with your normal savings account. (You can even name them too!) So create one for your emergency fund.
And you can start putting money into the account through my favorite system: Automating your personal finances.
The process only takes one to two hours at the most, but once you set it up, you don’t have to worry about it again.
AND it’ll save you thousands of dollars over your lifetime.
Here is a 12-minute video of me explaining the exact process I use below.
youtube
Step 2: Invest in your future
Investing your money is the best way to guarantee you become a millionaire. In fact, I promise you, if you follow the systems below you will eventually become rich.
Shannon knows that too.
“My husband and I have been putting 10 – 15 percent of our earnings into our retirement accounts for a while now,” she says. “We also have a 529 plan for each of our kids.”
When it comes to accounts for retirement, you have two options:
401k
Roth IRA
These are retirement accounts. That means you’ll be able to accrue gains with big tax advantages with one caveat: you promise to save and invest long term. That means you can buy and sell shares of almost anything as often as you want as long as you leave the money in your account until you get near retirement age.
Let’s take a look at each one.
401k: Free money from your employer
A 401k is a powerful retirement account offered to you by your employer. With each pay period, you put a portion of your pre-tax paycheck into the account. That means you’re able to invest more money into a 401k than you would a regular investment account.
But here’s the best part: Your company will match you 1:1 up to a certain percentage of your paycheck.
Say your company offers 3% matching. If your yearly salary is $150,000 and you invest 3% of your yearly salary (~$5,000) into your 401k, your company would match you that amount — doubling your investment.
Check out the graph below that illustrates this.
This is free money!!! If your company offers a match, you should ABSOLUTELY take part in their 401k plan.
For more on 401k’s, be sure to check out my article on how the account is the best way to grow your money.
But 401k’s are only one part of the equation when you want to start saving for retirement. The other account you should get is a Roth IRA. And ideally, you have both.
Roth IRA: The best long-term investment
A Roth IRA is simply the best deal I’ve found for long-term investing.
Remember how your 401k uses pre-tax dollars and you pay income tax when you take the money out at retirement? Well, a Roth IRA uses after-tax dollars to give you an even better deal.
With a Roth, you put in already taxed income into stocks, bonds, or index funds and pay no taxes when you withdraw it.
For example, if Roth IRAs had been around in 1970 and you’d invested $10,000 in Southwest Airlines, you’d only have had to pay taxes on the initial $10,000 income. When you withdrew the money 30 years later, you wouldn’t have had to pay any taxes on it.
Oh, and by the way, your $10,000 would have turned into $10 million.
That’s an exceptional example, but when saving for retirement your greatest advantage is time. You have time to weather the bumps in the market. And over years, those tax-free gains are an amazing deal.
How to open a Roth IRA account
To open up a Roth IRA, find a brokerage account. There are many out there, so I highly suggest shopping around and taking a look at the options out there.
Certain factors you want to consider when looking at brokers can be:
Minimum investment fees
Customer service
Investment options
Transaction fees
A few brokers I suggest, though, are Charles Schwab, Vanguard, and E*TRADE.
These brokers offer fantastic customer service and are well-known in the investment community for their great stock options.
Special note: Most brokers typically have minimum amounts for opening a Roth IRA, usually $3,000. Sometimes they’ll waive the minimums if you set up an automatic payment plan depositing, say, $100/month.
Also, it’s worth noting that there’s currently a yearly maximum investment of $6,000 to a Roth. (This amount changes often so be sure to check out the IRS contribution limits page to keep updated.)
Once your account is set up, your money will just be sitting there. You need to do things then:
First, set up an automatic payment plan so you’re automatically depositing money into your Roth.
Second, decide where to invest your Roth money; technically you can be in stocks, index funds, mutual funds, whatever. But I suggest investing your money in a low-cost, diversified portfolio that includes index funds such as the S&P 500. The S&P 500 averages a return of 10% and is managed with barely any fees.
For more, read my introductory articles on stocks and bonds to gain a better understanding of your options. I also created a video that’ll show you exactly how to choose a Roth IRA.
NOTE: After you invest in your retirement accounts, you can actually stop right there. After many years, your money will compound and earn you well into the millions if you continue investing.
Say you’re 25 years old and you decide to invest $500/month in a low-cost, diversified index fund. If you do that until you’re 60, how much money do you think you’d have?
Take a look:
$1,116,612.89.
However, if you want to be able to hit a million dollars sooner than that, there’s only one really good option: Earning more money through a hustle.
Part II: Earning more money
The reason earning money is one of my favorite ways to get to a Rich Life is simple:
There’s a limit to how much you can save, but no limit to how much you can earn.
If you’re willing to put in the work and develop a hustle that’ll scale and grow, you can earn as much money as you want. And while there are a lot of ways you can make more money, my favorite way is by starting a freelance hustle.
That’s what Shannon and Luisa did, and they’re going to show you how.
Step 3: Find a million dollar business idea (it’s easier than you think)
One very common misconception about starting your own freelance hustle is that you need to come up with the “perfect” idea to do it — when that couldn’t be further from the case.
“People think that they need a cool crazy idea like the next Facebook to make a significant amount of money,” Luisa says. “But what most people don’t realize is that they already have the skills to make a million dollars.”
I know it’s difficult to imagine that you might have profitable skills already — but you do. In fact, Shannon has a perfect solution to find out those skills: Look at what your friends ask you to help them out with. That’s how she got her start as a freelance CPA consultant.
From Shannon:
“I had a colleague who needed help sorting through her finances. She asked me to help her out, and she became my first client. Then I had another friend who started a law office and needed help, so I helped them out with all of their accounting. I’d meet with them to make sure that they were still compliant and help with their tax returns.
It just started with helping people as a hobby, but then my husband pointed out that I was getting clients without even trying. Eventually, I was able to start a freelancing business from it.”
That’s not the only way you can find a profitable freelancing idea either. There are actually 4 questions you can ask yourself right now to find an idea you can leverage for your hustle.
What do you already pay for? We already pay people to do a lot of different things. Can you turn one of those things into your own online business? Examples: Clean your home, walk your pet, cook you meals, etc.
What skills do you have? Now, what do you know — and know well? These are the skills you have that you’re great at — and people want to pay you to teach them. Examples: Fluency in a foreign language, programming knowledge, cooking skills, etc.
What do your friends say you’re great at? I love this question. Not only can it be a nice little ego boost — but it can also be incredibly revealing. Examples: Workout routines, relationship advice, great fashion sense, etc.
What do you do on a Saturday morning? What do you do on a Saturday morning before everyone else is awake? This can be incredibly revealing to what you’re passionate about and what you like to spend your time on. Examples: Browsing fashion websites, working on your car, reading fitness subreddits, etc.
Find an answer to those questions and I promise you you’ll find a great freelancing idea.
ACTION STEP: Find a profitable idea.
Spend about 10 – 20 minutes now writing down five answers for each of the four questions above. Once you’re done, congratulations — you now have 20 potential business ideas that you can grow into a flourishing side hustle.
For now, just choose one business idea. It’s okay, you can always change it later. For now, we’re going to just try one out and try to find a client with it.
Step 4: Find your first client
In order to start earning money, you need to find the people who will give you money for your ideas.
But the question is…how? Where do you find these people?
For Luisa, that meant going online and finding out where her clients lived.
“I spent a lot of time on Facebook groups talking with potential clients answering their questions about advertising,” Luisa says. “That’s when I found my first client.”
You can do the same thing.
Ask yourself:
Who is my client?
Where do they go when they want to look for a solution to their problems?
Where are they ALREADY looking for solutions to their problems?
How can you connect them to your service?
At this point, you’re also going to want to niche down your market in order to really tailor your services and draw in customers.
“Stay in your niche,” Shannon suggests. “We had a few instances where we veered from the niche and we paid for it dearly. It might feel cheesy to sit down and figure out what your target market is or what your goals are for the company, but you have to do that. All that legwork needs to be done upfront. It’s just practical.”
So think about who’s an example of a client who might want to buy your product.
A few questions to jumpstart your research:
How old are they?
Where do they live?
What are their interests?
How much do they make?
What books do they read?
Using this information, find out what your clients need by going to the places they go.
For example:
Want to pitch to moms that blog about children? Go to The Mom Blogs and start with the ones under “Popular Blogs.”
Looking for physical or massage therapists within 50 miles of your house? Yelp should get you started easily.
If you want to do large dog grooming and sitting, well there’s probably a local pet store or dog park near you where owners are all congregating just waiting for you to offer them a solution.
Here are a few suggestions of some other great sites freelancers can use to find business online:
Once you find a potential client, you’re going to want to reach out to them and pitch your services.
ACTION STEP: Find a client and email them (with scripts).
Find your client using the information, I’ve outlined above. Once you’ve done that, you can reach out to them using this handy script:
CLIENT’S NAME,
I saw your post on X and visited your website. I noticed that you’ve recently started using videos too.
I’ve been doing video editing for three years and I’d like to offer to help you edit your videos and get them optimized for the web.
That would make them look more professional and load faster, which is important for your readers. And you’d free up time that you could use to create new content.
We can discuss the details, of course, but first I wanted to see if this is something you might be interested in.
If so, would it be okay if I sent you a few ideas on how to help?
Best,
Ramit Sethi
A few takeaways:
There is zero fat in the pitch. Every word counts and is needed to help really sell the benefits of working with you.
Don’t mention payment. There’s nothing that will kill a potential client’s interest in you more than pushing prices on them before they’re ready.
Stress the benefits. This email shows the client why it would be in their best interest to buy from you in the third paragraph.
Once you get a client using this email, congrats! You just secured your first client — but it doesn’t end there. You need to actually do the work for them, and that means continually adding value.
Step 5: Invest again — but this time, in yourself
Investing takes many shapes. It’s not all stocks, bonds, and retirement accounts. Investing can also be in yourself — and it’s something you need to do if you want to earn a million dollars. Be continually curious.
From Luisa:
“I’ve always been big on investing [in myself]. Even in my previous businesses it’s been a lot of me putting in the cash into my business. I invested in my site. I invested in a copywriter to teach me how to make copy. I invested in someone to teach me how to make sales calls.
Thankfully, I knew what I didn’t know, so I invested heavily in those things. I’m not a big risk taker. But I do believe in calculated risk. After all, I am my best asset. My top priority is myself or my business.”
I love this. It hits on an idea that all IWT readers should embrace: Be continually curious.
Ask questions when you don’t understand something and don’t be afraid to seek out more information through books, courses, or schooling. It’s only then that you can hope to truly live your Rich Life.
That’s why my team and I have worked hard to create a guide to help you invest in yourself today: The Ultimate Guide to Making Money.
In it, I’ve included my best strategies to:
Create multiple income streams so you always have a consistent source of revenue.
Start your own business and escape the 9-to-5 for good.
Increase your income by thousands of dollars a year through side hustles like freelancing.
Download a FREE copy of the Ultimate Guide today by entering your name and email below — and start blowing up your net worth today.
Yes, send me the Ultimate Guide to Making Money
Source link
Source/Repost=> http://technewsdestination.com/how-to-make-a-million-dollars-with-advice-from-actual-millionaires/ ** Alex Hammer | Founder and CEO at Ecommerce ROI ** http://technewsdestination.com
0 notes
Text
Snapchat announces new augmented reality platform – Haptical
NEW EXPERIENCES
Garuda launches inflight virtual reality entertainment
Garuda Indonesia’s latest inflight entertainment option is the first VR system in Asia Pacific to have been certified with an international flight safety approval, according to a statement from the airline… (Jakarta Post)
Full-body virtual reality lets you feel the Amazon rainforest
In recent years, an increasing number of pop-ups and other location-based virtual reality arcades have allowed people to go beyond simply strapping on a headset by incorporating multi-sensory interactions like motion and scent… (ABC News)
Inside Muse and Microsoft’s virtual reality tour experience
Muse has partnered with Microsoft to offer fans a one-of-a-kind virtual reality experience during their Simulation Theory world tour, which kicked off earlier this year… (Billboard)
Source link
Source/Repost=> http://technewsdestination.com/snapchat-announces-new-augmented-reality-platform-haptical/ ** Alex Hammer | Founder and CEO at Ecommerce ROI ** http://technewsdestination.com
0 notes
Text
Create an iOS app that uses built-in and custom classifiers – IBM Developer
Summary
In this developer code pattern, use IBM Watson
Visual Recognition to showcase various built-in and custom classifiers on IBM Cloud using an iOS app built using Swift. A user can open the app on an iOS-based mobile phone and choose the different classifiers (faces, explicit, food, etc.) they want to use, including custom classifiers. The Watson Visual Recognition service on IBM Cloud classifies and provides the app with the classification results.
Description
The app in this code pattern has support for the following features of Watson Visual Recognition:
General — Watson Visual Recognition’s default classification returns the confidence of an image from thousands of classes.
Food — A classifier intended for images of food items.
Explicit — Returns percentage of confidence of whether an image is inappropriate for general use.
Custom classifier(s) — Gives the user the ability to create his own classifier.
Flow
Clone the repo.
Install dependencies with Carthage.
Set up Watson Visual Recognition credentials.
Run the app with Xcode.
Instructions
Ready to check it out? See the all detailed info on GitHub.
Components Watson Visual Recognition
Source link
Source/Repost=> http://technewsdestination.com/create-an-ios-app-that-uses-built-in-and-custom-classifiers-ibm-developer/ ** Alex Hammer | Founder and CEO at Ecommerce ROI ** http://technewsdestination.com
0 notes
Text
The AI Elephant in the Call Center – Chatbots Life
Deep logic #1: How to add expertise to chatbots
Image by goeshand(Zcool) from 4-designer.com
This is the article #1 in a series about the challenges of adding deep logic (expertise) to product support chatbots. I work for eXvisory.ai, an Oxford UK startup that provides visual dev tools to scalably and maintainably add deep logic to chatbots. To demo these tools we’ve build a chatbot that troubleshoots problems with mobile phones or tablets. Instead of chatting with a scripted human in a call center users chat with a scripted AI.
Lots of companies say they do that — how is eXvisory different?
eXvisory is a tad unfashionable. It’s AI in a tweed jacket. It doesn’t use machine learning (gasp). Machine learning AI is great for matching single questions to single answers (given lots of high quality Q&A training data) but not so hot for conversational or back-and-forth Q&A. It’s not a problem with machine learning algorithms — it’s just much harder (impossible?) to obtain enough high quality conversational Q&A training data. eXvisory uses deep logic networks, an Internet reboot of rule-based expert systems. It also asks the questions. Natural language query interfaces are fun, but customers quickly tire of them when they are not helpful.
But the biggest difference is that eXvisory is taking aim at the big grey AI elephant in the call center (metaphorically speaking).
Support scripts
The state-of-the-art for post-sales product support, especially for companies selling high volumes with low margins, is out-sourced call centers staffed with low paid humans slavishly following scripted diagnostic procedures they don’t appear to understand. The consumer support experience is excruciating — a dire combination of tedious scripts and inexpert staff.
Top Articles on How Businesses are using Bots:
1. WhatsApp for Business: A Beginners Guide
2. Revenue models for bots and chatbots
3. Chatbots: What Happened?
4. Chatbot Conference in San Francisco
It’s a catch-22 situation. High volumes and low margins means there’s not enough money to pay for product support experts, so it’s outsourced to low paid, high utilization call centers where inexpert staff are expected to support multiple products. The staff know nothing about the products so they blindly follow scripts. The scripts are linear and tedious because the staff can only cope with simple scripts and it’s HARDER THAN IT LOOKS to develop scripts capable of diagnosing a useful number of problems with complex products without asking an irritating number of irrelevant questions.
That’s the AI elephant in the call center — support scripts. If they are straight-forward to create and actually useful, why haven’t they already been automated by AIs? After all, the support staff are not allowed to deviate from their scripts, so they are already behaving like robots … or AIs.
Because consumers prefer to talk to a real person?
Right. They might prefer talking to an expert human, but talking to an inexpert human is even more irritating than talking to an inexpert AI, because you feel trapped on an unhelpful call or chat, unwilling to be impolite by leaving to make a cup of tea or walk the dog. Chatting with an expert AI in your own time and at your own pace sounds heavenly by comparison.
Machine learning is not the only AI
Most current product support AI projects are riding the wave of interest in machine learning AI by adding natural language search interfaces to existing but ineffective knowledge bases. Training machine learning AIs on low quality knowledge bases generated by inexpert support staff is flawed. Machine learning is amazing but it’s not magic. If you look at successful machine learning projects they have lots of high quality training data (e.g. expert medical diagnoses of retinal scans) or can generate lots of their own training data (e.g. by playing billions of games with themselves).
From scripts to support AIs
eXvisory has developed a deep logic AI framework that enables companies to turn their support scripts and knowledge bases into convenient, self-paced support chatbots. Our development tools enable senior support engineers to stop training low paid humans and start training AIs instead.
Our goal is the same as all support AI projects — to improve the customer support experience (better outcomes, more convenience) while dramatically reducing support costs (fewer and briefer customer contacts, handed over from useful initial AI sessions) but our approach is quite different!
Build your own deep logic chatbot
To be notified of more deep logic articles follow me on my Medium profile page. Or say hello at [email protected] if you’d like access to our dev documentation, online tutorials, a web demo — or your own free dev instance to build your own deep logic chatbot. If you enjoyed this article please recommend it to others by clapping madly (below) and sharing or linking to it. You can also leave feedback below.
Thanks!
Don’t forget to give us your
!
Source link
Source/Repost=> http://technewsdestination.com/the-ai-elephant-in-the-call-center-chatbots-life/ ** Alex Hammer | Founder and CEO at Ecommerce ROI ** http://technewsdestination.com
0 notes