#AletheaTech
Explore tagged Tumblr posts
Text
How to Optimize Your Digital Content for Engagement
Alethea Tech combines Generative AI and Blockchain to create innovative solutions, transforming digital content creation and providing secure, decentralized asset management for creators and businesses.
0 notes
Text
Which one is better: Wi-Fi 6 or Wi-Fi 7 ?
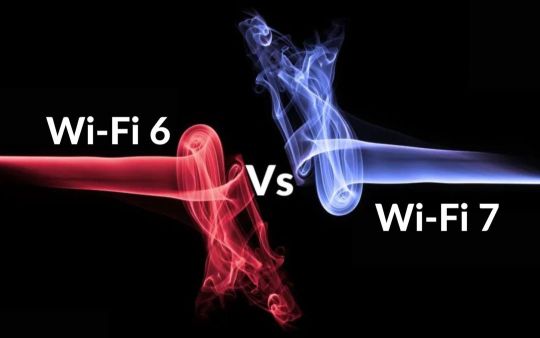
Wi-Fi 6 and Wi-Fi 7 are the latest generations of wireless networking technology. Both Wi-Fi 6 and Wi-Fi 7 promise faster speeds, improved performance, and greater capacity than previous generations of Wi-Fi. However, there are some key differences between the two technologies that are important to understand.
One of the primary distinctions between Wi-Fi 6 and Wi-Fi 7 is their maximum data transfer rates. Wi-Fi 6, also known as 802.11ax, can reach speeds of up to 9.6 Gbps, while Wi-Fi 7, also known as 802.11be, can reach speeds of up to 30 Gbps. This means that Wi-Fi 7 is significantly faster than Wi-Fi 6.
Another difference between Wi-Fi 6 and Wi-Fi 7 is their capacity. Wi-Fi 6 is designed to handle a greater number of devices on a single network, allowing more devices to connect and communicate at the same time.
Wi-Fi 6 also has improved security features, including support for WPA3, the latest standard for wireless network security. Wi-Fi 7 also has advanced security features to protect the network from unauthorized access and malicious attacks.
Wi-Fi 6 also has a feature called Orthogonal Frequency Division Multiple Access (OFDMA) which enables the router to divide a channel into smaller sub-channels. This allows multiple devices to share the same channel and communicate at the same time, improving network efficiency and reducing latency.
Recent updates in Wi-Fi 7
Wi-Fi 7 is not yet an official standard and it has not been released yet. The Wi-Fi Alliance, the organization that oversees the development of Wi-Fi standards, has not announced any official plans for Wi-Fi 7. Wi-Fi 6 (802.11ax) is the latest standard that has been announced and it is currently available for use.
Wi-Fi 7 is in its early development stages and it is expected to be released in the future. It’s worth mentioning that Wi-Fi 7 is also known as 802.11be, it is expected to be faster and more efficient than Wi-Fi 6, with speeds of up to 30 Gbps and the ability to support even more devices and handle more data traffic. Checking the official website of Wi-Fi Alliance for the latest announcements and updates on Wi-Fi 7 and other wireless networking technologies.
Conclusion:
Wi-Fi 7 is faster, more efficient, and more secure than Wi-Fi 6. With faster speeds, greater capacity, and improved security, it is the ideal choice for organizations and businesses that need to support a large number of devices and handle large amounts of data traffic. However, the availability and the price of the devices that support Wi-Fi 7 is currently limited and it may take some time for it to be widely adopted.
0 notes
Text
Alethea Tech is a powerful platform designed for creating and deploying intelligent AI characters. It uses natural language processing (NLP) and machine learning to create characters that can interact with users in a human-like manner.
0 notes
Text
Best data analytics solutions by Alethea tech
Discover how Alethea Tech empowers enterprises with cutting-edge machine learning and data analytics solutions, helping businesses harness data for innovation and competitive advantage.
0 notes
Text
Revolutionizing AI: Alethea Tech’s Decentralized Future"
Alethea Tech combines Generative AI with Blockchain to create decentralized AI solutions. By promoting collective ownership and governance, Alethea Tech aims to harness AI's potential for the benefit of humanity.
0 notes
Text
Alethea Tech Unveils the Intelligence Layer for Building Immersive AI Characters
Massive strides started happening in the world of artificial intelligence when Alethea Tech announced its latest creation: the Intelligence Layer, specifically designed for crafting interactive AI characters. It's a fresh framework that equips developers with the critical tools needed to craft dynamic, user-interactive digital assets that respond intelligently to users.
The Intelligence Layer is thus a key enabler in infusing AI characters with rich behavior, emotional responses, and personalized interactions. This evolution has now given creators new dimensions to come up with characters that are not only aesthetically pleasing but can also interact with the user on multifarious levels.
By focusing on the Intelligence Layer, developers are able to create AI characters that can adapt different situations, creating more immersive user experiences in gaming, education, and virtual environments. This transformative approach underlines Alethea's mission-to arm creators with technology that closes the gap between imagination and reality.
Living in a world where the demand for more interactive content is growing day by day, Alethea Tech's Intelligence Layer positions itself at the forefront of this evolution, offering unmatched capabilities to the developer in search of innovation and audience capture through intelligent immersive AI characters.
0 notes
Text
youtube
With a focus on decentralized ownership, Alethea Tech enables creators to engage with AI-generated content securely. Alethea AI provides the tools necessary for artists to maintain control over their intellectual property, ensuring transparency, protection, and fair participation in a collaborative creative ecosystem.
1 note
·
View note
Text
The Future of In-Flight Wi-Fi: How Scale Testing Can Ensure Smooth Deployment

In the 21st century, Wi-Fi has become an integral part of our daily routine needed in every sector of industry. Wi-Fi as a technology introduced in the early 2000s has grown with each passing day and is evolving rapidly. With initial speeds from 100kbps in the early 2000s Wi-Fi can generate a speed of Gbps in seconds from anywhere in the world. Any information on any topic about Wi-Fi is available just in our fingerprints from any look and corner of the world and has certainly made our life easier and faster. With Wi-Fi showing high signs of improvement in all industries, the connectivity inside the aircraft is still not as up to the mark. That is why many experts say that it is the market of the 21st century and has valued it at 5.5 billion dollar market in 2022 which is expected to increase by 15% in the next few years. Connecting to the internet from an aircraft for a decade was something we had only imagined, thanks to the evolving Wi-Fi technology we are now able to get good and better connections throughout the journey. Even though the time of journey and spacious seats are a concern, the Wi-Fi connectivity in the last few years has certainly improved. Many of the market leaders are investing in In-flight connectivity as there is a high increase in high-definition streaming, data-loaded applications and connected networks everywhere. In the past few years, many carriers have started to deploy inflight Wi-Fi to their fleets to make the overall flight experience better. Wi-Fi has been a major looked criterion for people whenever they are about to book a ticket and with this high increase in demand and need for Wi-Fi, it has become a must to install Wi-Fi in their planes but to deploy Wi-Fi in the aircraft is a lot of investment, time taking process which has to be done with a lot of testing to make sure to provide the best Wi-Fi to all the passengers. Before deployment, it is very necessary to test the performance and experience of the Wi-Fi and so testing at scale is a mandatory process to make sure that it caters to a large number of users at a single point in time. Therefore testing at scale ensures all the problems can be looked at and the best Wi-Fi with the best performance can be installed in the aircraft.
The deployment can be done as per the requirement after the large-scale testing to provide a high-quality connection to all the users without any interruption. At Alethea Communications Technologies, we test inflight Wi-Fi at scale before the deployment process to make sure that all the users get a seamless connection for a memorable flight journey.
To know more - Visit Us
0 notes
Text
Log Analysis using AI/ML for Broadband

Log Analysis using Artificial Intelligence/Machine Learning [AI/ML] for Broadband
Whenever you hear about “Log analysis”, we picture a developer, going through 1000s of lines of logs to figure out a problem. Does it always have to be like this? Our topic of discussion is what can Artificial Intelligence/Machine Learning [AI/ML]do to help us in Log analysis.
Need for Automated Log Analysis
In large-scale systems, the seemingly obvious way of log analysis is not so scalable. A broadband network managed by an operator like Comcast, having 100s of Wi-Fi Access Points and Routers/Switches and 4G/5G small cells, from multiple equipment providers, say Commscope, Aruba, or CISCO. Collection of logs at multiple nodes, there are GBs of data created every minute.
The possible issues are hidden, they may not be something as obvious as a crash. It may be a problem that occurred and went away and could not be detected, other than the fact that there were several complaints received by the Network Operators. These systems are developed by multiple developers (100(0)s), so it is difficult to be analyzed them by a single person. They pull out modules from various third parties and make extensive use of the open source. And then the parts of the systems are on continuous upgrade cycles. So there is a clearly established need for automated log analysis in large-scale networks through the use of smart log analysis techniques.
Mapping Log Analysis problem to Artificial Intelligence/Machine Learning [AI/ML] problem
Machine learning sees the problems in two ways:
supervised
unsupervised.
Supervised learning is applicable if we have a labeled data set i.e. input data, where we know the label (or value). With this data, we can train the model. After Training, the model can take the new input and predict the label (or value).
Unsupervised learning means we do not have labeled data sets. The model classifies data into different classes. When the new data arrives, it finds the correlation with the existing classes and puts it into one of those classes.
For log analysis, we are basically looking for anomalies in the log, something that is not normally expected. We may or may not have labeled data sets, and accordingly, we need to pick supervised or unsupervised learning.
Anomaly Detection algorithms. For supervised algorithms, we will have data sets, where each set is labeled as “normal” or “Anomaly”. For unsupervised algorithms, we need to configure the model for two classes only, “Normal” or “Anomaly”.
A combined approach is good for the broadband use case, where both can be used. For clear anomalous behaviour we can use supervised methods. And when creating an exhaustive labeled data set may not be possible, we can fall back to unsupervised.
These algorithms exist already and there are open-source implementations as well. (refer References)
Mapping Logs to Artificial Intelligence/Machine Learning [AI/ML] input
There are many ongoing online logs coming from various nodes. The only way to make a data set is to time-slice them, into smaller log snippets. Using each snippet we have to convert it into a data set.
Now the logs are distributed, coming from switches, routers, SysLogs and Pcaps, and Others. Do we need different models for each kind of log? No. The logs have to be given to a single Model as only then the correlation between different logs can be harnessed.
The logs are unstructured text, can we use (Natural Language Processing) Models to extract data sets from the logs. The answer is again “No”. For NLP models, the text is preprocessed to get features like the number of times a word is repeated, the different words followed by each other, and other features. There are pre-trained models which can do this and have been trained over the entire Wikipedia text! But these can not be used for logs, as logs have technical context and not the natural language.
Since logs have an underlying structure, we can view the log snippets as a series of predefined events. This way we can retain the information in each log. It also helps aggregate different kinds of logs, as we can consider the logs having different sets of events. The model will be trained by understanding based on events that are happening in a given time window and can then detect anomalies.
Constructing Artificial Intelligence/Machine Learning [AI/ML] Training data set from Logs
Artificial Intelligence/Machine Learning [AI/ML] works on vectors/matrices of numbers and additions and multiplications of these numbers. We can not feed these events directly to the model. They need to be converted into numbers. (Gradient Descent and Logistic Regression works with finding derivatives. Deep learning is Matrix multiplications and lots of it. Decision Trees or Random forests partition the data on numbers.)
For computer vision and image processing use cases, these numbers are the RGB value of each pixel in the image. For tabular data, the text is converted into numbers by assigning ordered or unordered series.
One option is to associate each event with an identifier number and give vectors of these identifiers to the model, along with a timestamp. However, synchronizing/aggregating this will be an issue as we will start getting these vectors from each node. Also one event may happen multiple times, in the snippet, so handling of these vectors will become complex.
So a better method is to collate vectors from each node for a given time slice and then go with the count of each kind of event in a master vector.
We explain the approach below in detail. The approach is derived from this popular paper, for more details please refer https://jiemingzhu.github.io/pub/slhe_issre2016.pdf)
1.Log Collection –
In broadband systems, we have multiple sources of logs (SysLog, Air captures, wired captures, Cloud Logs, Network element i.e. switches/Routers/Access Points logs). We need to first be able to gather logs from each of the sources.
We need to make an exhaustive list of all sources as
[S1, S2, S3.. Sn]
2.Event Definition – For each source, we need to come up with predefined event types. In the networking world, broadly event types in the logs, can be defined as follows
Protocol message
Errors/Alerts
Each Type is one event type
Layer
Management
Each Type is one event type
Control
Each Type is one event type
Data
Each Type is one Event type
State Change
Error Alerts
Each Type is one event type
Module
Each Critical Log Template is an event type
Each State Transitions is an event type
Errors/Alerts
Each type of Error/Alert is one event type each Leaf node corresponds to a different event
With this analysis, for each source, we come up with a list of events, as follows
[S1E1, S1E2, S1E3,.. S1Em,
S2E1, S2E2, S2E3,.. S2En,
… ,
SnE1, SnE2, SnE3,.. SnEp]
3.Log to Event conversion – Each line of the time series log will have a constant part and a variable part. The constant part is what we are interested in. Variable parts like IP addresses, source and destination are variable and need to be ignored. We need to parse logs for the constant parts, to check if the log has any event or not, and record only the event. Then the log snippet taken over a window of time will start looking like something like this for a source.
[T1, E2
T2, Nil
T3, E2
T4, E4]
4.Frequency transform – Invert the parsed log to find event frequency. Basically in a given time window how many times an event happened. So if the window goes from Time 1 to Time 4.
Going for multiple time slices it will look like this
The window can be fixed with timer intervals. These can be non-overlapping or sliding. Sliding windows can give better results, but maybe more computationally intensive.
For balancing computation load, it is advisable to do edge compute i.e. derive the Event Count Matrix separately from each source.
5.Event Frequency Matrix – Once the event count matrix is being fetched from each source, they should be all combined at a central place, before being fed to the ML world.
Highlighted Part is the final Matrix that is an input to the ML system. Each window is fed with a timestamp. So it becomes a time series input vector. Set of these vectors will make a data set. So finally now we have the data set for log analysis!
Resources
[1] AI/ML Theory Machine Learning by Stanford University
[2] Applied AI/ML Tutorial Deep Learning For Coders—36 hours of lessons for free
[3] Log Analysis AI/ML Research Paper Experience Report: System Log Analysis for Anomaly Detection
[4] LogPai/Loganaly (logpai/loglizer: A log analysis toolkit for automated anomaly detection [ISSRE’16])
[5] AICoE/LAD (AICoE/log-anomaly-detector: Log Anomaly Detection – Machine learning to detect abnormal events logs)
Request a Call
0 notes