Don't wanna be here? Send us removal request.
Text
#nlp libraries#natural language processing libraries#python libraries#nodejs nlp libraries#python and libraries#javascript nlp libraries#best nlp libraries for nodejs#nlp libraries for java script#best nlp libraries for javascript#nlp libraries for nodejs and javascript#nltk library#python library#pattern library#python best gui library#python library re#python library requests#python library list#python library pandas#python best plotting library
0 notes
Text
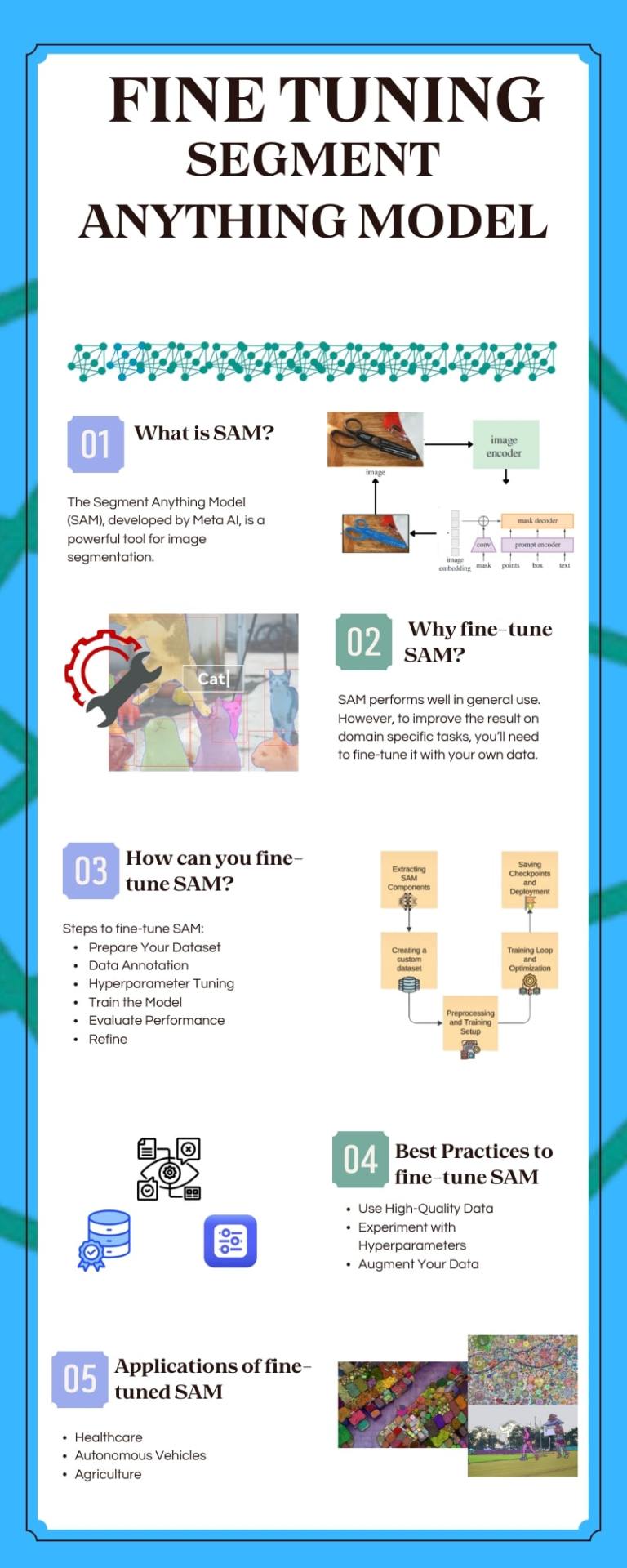
Unlock the full potential of fine tuning Segment Anything Model (SAM) to enhance its performance for specific tasks. Learn about dataset preparation, hyperparameter tuning, and real-world applications across industries. Dive deeper at https://www.labellerr.com/blog/fine-tuning-sam/
1 note
·
View note