Don't wanna be here? Send us removal request.
Text
Tableau course in Banglore offered by NearLearn
This training program offers a thorough understanding of the Tableau platform and its tools, enabling participants to effectively gather, collaborate, and manage data for improved organizational performance. Practical sessions and hands-on exercises will provide a comprehensive understanding of how to transform raw data into actionable insights that drive business success. The course covers topics such as Tableau's features, data visualization techniques, data preparation, cleaning, blending and joining, advanced analytics, sharing, collaboration, and administration of Tableau Server and Online. Upon completion of this program, participants will possess the necessary skills and knowledge to maximize Tableau's potential, making them valuable assets to any organization.
To learn more and enrol in this course, visit the following link: https://nearlearn.com/tableau-online-training-india
0 notes
Text
"Kickstart Your Career as a Full-Stack Java Developer with NearLearn's Expert Guidance in Bangalore"
Looking to become a full-stack Java developer in Bangalore? Enrol in NearLearn's Java full-stack career course for expert guidance and practical experience. Our comprehensive curriculum will prepare you for the professional world and help you achieve your dream job. Contact us today to start your journey.
WEB: https://nearlearn.com/courses/java-full-stack-online-training
0 notes
Text
Tableau Training - In-depth Knowledge of the Tableau Platform
This course provides a comprehensive understanding of Tableau tools and their usage, empowering you to collect, collaborate, and manage data effectively for better organizational performance. Through practical sessions and hands-on exercises, you'll learn how to turn raw data into actionable insights that drive business success.
Topics covered in this course include:
Introduction to Tableau and its features
Data visualization techniques
Data preparation and cleaning
Data blending and joining
Advanced analytics using Tableau
Sharing and collaboration with Tableau
Tableau Server and Online administration
By the end of this course, you'll have the skills and knowledge to leverage Tableau's full potential, making you a valuable asset to any organization.
To learn more and enrol in this course, visit the following link:https://nearlearn.com/courses/tableau-online-training
0 notes
Text
"Kickstart Your Career as a Full-Stack Java Developer with NearLearn's Expert Guidance in Bangalore"
Looking to become a full-stack Java developer in Bangalore? Enrol in NearLearn's Java full-stack career course for expert guidance and practical experience. Our comprehensive curriculum will prepare you for the professional world and help you achieve your dream job. Contact us today to start your journey.
WEB: https://nearlearn.com/courses/java-full-stack-online-training
0 notes
Text
Your Ultimate Solution for Data Visualization
If you are looking for the best Tableau course in Bangalore, look no further than NearLearn. NearLearn is a leading provider of high-quality online and classroom-based courses in data science, machine learning, artificial intelligence, and more. With their Tableau course, you can gain the skills and knowledge you need to create powerful data visualisations that help you make better decisions and achieve better business outcomes.
0 notes
Text
What is the main advantage of Tableau?

Tableau is a powerful and intuitive data visualization tool that has gained immense popularity in recent years. It is used by businesses and organizations of all sizes to analyze and interpret complex data in a visually appealing and easy-to-understand format. One of the main advantages of Tableau is its ability to help users quickly and easily transform raw data into actionable insights.
In this article, we will explore the main advantage of Tableau and how it can benefit your organization.
One of the most significant advantages of Tableau is its user-friendly interface. It offers a drag-and-drop functionality that allows users to quickly and easily create custom data visualizations without any coding knowledge. This ease of use makes it an ideal choice for businesses and organizations that require frequent data analysis and reporting.
Tableau also offers a wide range of visualization options that can be tailored to meet the specific needs of your organization. From charts and graphs to maps and dashboards, Tableau provides users with the flexibility to create custom visualizations that are both informative and engaging. This level of customization ensures that the data is presented in a way that is easy to understand and provides insights that are relevant to your business.
Another significant advantage of Tableau is its ability to connect to a wide range of data sources. Whether your data is stored in spreadsheets, databases, or cloud-based platforms, Tableau can quickly and easily connect to it. This ability to connect to multiple data sources makes it an ideal tool for businesses that rely on data from different departments or systems.
Tableau also offers advanced analytics capabilities that allow users to drill down into their data to identify trends and patterns. This ability to analyze data at a granular level provides users with deeper insights into their business operations and helps them make more informed decisions.
Tableau's data visualization capabilities also make it an ideal tool for collaboration. With Tableau, users can easily share data visualizations with their colleagues, allowing them to collaborate and make decisions based on real-time data. This level of collaboration ensures that everyone is on the same page and working towards a common goal.
In addition to its user-friendly interface and data visualization capabilities, Tableau is also known for its speed and performance. It uses a proprietary data engine that allows users to quickly analyze and visualize large datasets. This speed and performance make it an ideal tool for businesses that require real-time data analysis and reporting.
Tableau's speed and performance are also enhanced by its ability to handle complex data structures. Whether your data is structured, semi-structured, or unstructured, Tableau can quickly and easily handle it. This ability to handle complex data structures ensures that businesses can make informed decisions based on all available data, not just a subset of it.
Tableau is also known for its ability to integrate with other business intelligence tools. It can be easily integrated with tools such as Microsoft Excel, Salesforce, and Google Analytics, allowing businesses to leverage the power of their existing data infrastructure. This level of integration ensures that businesses can get the most out of their existing data investments.
Read More: Join the best tableau course training provided by NearLearn.
In conclusion, Tableau offers a wide range of advantages that make it an ideal tool for businesses and organizations of all sizes. Its user-friendly interface, data visualization capabilities, and advanced analytics capabilities make it easy to transform raw data into actionable insights. Additionally, its ability to connect to multiple data sources, handle complex data structures, and integrate with other business intelligence tools make it an essential tool for any organization that relies on data to make informed decisions.
0 notes
Text
What makes Tableau unique?

Tableau is a powerful data visualization tool that is widely used by businesses and organizations of all sizes to analyze and interpret complex data. It is known for its user-friendly interface, flexibility, and advanced analytics capabilities. In this article, we will explore what makes Tableau unique and how it can benefit your organization.
User-friendly interface:
Tableau's user-friendly interface is one of its most significant strengths. It offers a drag-and-drop functionality that allows users to create custom data visualizations without any coding knowledge. This ease of use makes it an ideal choice for businesses and organizations that require frequent data analysis and reporting. Additionally, Tableau offers a wide range of visualization options that can be tailored to meet the specific needs of your organization.
Advanced analytics capabilities:
Tableau's advanced analytics capabilities allow users to analyze data at a granular level. It provides users with the ability to drill down into their data to identify trends and patterns. This level of analysis provides users with deeper insights into their business operations and helps them make more informed decisions.
Flexibility:
Tableau is a highly flexible tool that can be customized to meet the specific needs of your organization. It offers a wide range of visualization options, including charts, graphs, maps, and dashboards, that can be tailored to meet the specific needs of your business. Additionally, Tableau can connect to a wide range of data sources, including spreadsheets, databases, and cloud-based platforms.
Real-time data analysis:
Tableau's speed and performance make it an ideal tool for businesses that require real-time data analysis and reporting. It uses a proprietary data engine that allows users to quickly analyze and visualize large datasets. This speed and performance make it an essential tool for businesses that require real-time insights into their operations.
Collaboration:
Tableau's collaboration capabilities allow users to easily share data visualizations with their colleagues. This level of collaboration ensures that everyone is on the same page and working towards a common goal. Additionally, Tableau's collaboration capabilities allow users to collaborate in real time, ensuring that decisions are made based on the most up-to-date data.
Integration with other business intelligence tools:
Tableau can be easily integrated with other business intelligence tools, such as Microsoft Excel, Salesforce, and Google Analytics. This level of integration ensures that businesses can get the most out of their existing data investments. Additionally, Tableau's ability to integrate with other business intelligence tools allows businesses to leverage the power of their existing data infrastructure.
Read More: Join the best Tableau training course Offered By NearLearn.
In conclusion, Tableau is a unique data visualization tool that offers a wide range of advantages that make it an essential tool for businesses and organizations of all sizes. Its user-friendly interface, flexibility, and advanced analytics capabilities make it easy to transform raw data into actionable insights. Additionally, its real-time data analysis capabilities, collaboration capabilities, and integration with other business intelligence tools make it an essential tool for any organization that relies on data to make informed decisions.
#Tableau#data visualization tool#unique#advantages#advanced analytics#advanced analytics capabilities
0 notes
Text
Java Full Stack vs MERN Stack: Which One Pays Better?

In today's fast-paced world of software development, choosing the right technology stack is crucial for your career growth. Java Full Stack and MERN Stack are two of the most popular technology stacks for web development. Both offer unique features and benefits that can enhance your skills and boost your salary. In this article, we will compare the salary trends of Java Full Stack and MERN Stack developers and help you decide which one pays better.
Java Full Stack
Java Full Stack refers to the combination of Java-based technologies used for building web applications. It includes Java Enterprise Edition (Java EE), Spring Framework, and front-end technologies such as HTML, CSS, and JavaScript. Java Full Stack developers are responsible for designing, developing, and maintaining web applications using these technologies.
According to PayScale, the average salary for a Java Full Stack developer in the United States is $87,218 per year. However, the salary can range from $59,000 to $126,000 depending on the company, location, and experience level.
MERN Stack
MERN Stack stands for MongoDB, ExpressJS, ReactJS, and NodeJS. It is a popular technology stack for building web applications that include a No SQL database, server-side JavaScript, and a front-end library. MERN Stack developers are responsible for designing, developing, and maintaining web applications using these technologies.
According to Indeed, the average salary for a MERN Stack developer in the United States is $120,529 per year. However, the salary can range from $75,000 to $190,000 depending on the company, location, and experience level.
Comparison
When comparing the salaries of Java Full Stack and MERN Stack developers, it's important to consider the demand for these skills in the market. According to LinkedIn, Java Full Stack is among the top 10 in-demand skills for 2021. On the other hand, MERN Stack is also in high demand, especially in the tech hub cities such as San Francisco, Seattle, and New York.
Another factor to consider is the experience level. Both Java Full Stack and MERN Stack require different levels of expertise, and salaries vary accordingly. Junior Java Full Stack developers can earn an average of $60,000 per year, while senior Java Full Stack developers can earn up to $136,000 per year. Similarly, junior MERN Stack developers can earn an average of $70,000 per year, while senior MERN Stack developers can earn up to $200,000 per year.
Read More: Join India's best Machine Learning & AI training Online.
conclusion, both Java Full Stack and MERN Stack are lucrative career options for web developers. However, the salary trends suggest that MERN Stack developers have a higher earning potential than Java Full Stack developers. That being said, choosing a technology stack solely based on salary is not recommended. It's important to consider your skills, interests, and job market demand before making a decision.
0 notes
Text
Tableau Training - In-depth Knowledge of the Tableau Platform
This course provides a comprehensive understanding of Tableau tools and their usage, empowering you to collect, collaborate, and manage data effectively for better organizational performance. Through practical sessions and hands-on exercises, you'll learn how to turn raw data into actionable insights that drive business success.
Topics covered in this course include:
Introduction to Tableau and its features
Data visualization techniques
Data preparation and cleaning
Data blending and joining
Advanced analytics using Tableau
Sharing and collaboration with Tableau
Tableau Server and Online administration
By the end of this course, you'll have the skills and knowledge to leverage Tableau's full potential, making you a valuable asset to any organization.
To learn more and enroll in this course, visit the following link:https://nearlearn.com/courses/tableau-online-training
0 notes
Text
Java full stack route in Bangalore with the aid of NearLearn
Java full stack route
Becoming a full-stack Java developer through enrolling in NearLearn java full-stack profession course. And with the NearLearn right guidance, you can obtain your dream job. NearLearn provides the most promising curriculum to provide a direct method to the expert world with complete know-how of Java full stack.
WEB: https://nearlearn.com/courses/java-full-stack-online-training
Email: [email protected]
Enquiry: +91-80-41700110
ADD: No: 61,1st Floor, 7th Main, 12th Cross Rd, BTM 2nd Stage, Bengaluru, Karnataka 560076
Facebook page: https://www.facebook.com/NearLearnGlobal/
Instagram: https://www.instagram.com/near_learn/?hl=en
Linkedin: https://in.linkedin.com/company/nearandlearn
Twitter: https://twitter.com/nearlearn/status/1253662336390324224
in Bangalore with the aid of NearLearn
0 notes
Text
Java full stack route in Bangalore with the aid of NearLearn
Becoming a full-stack Java developer through enrolling in NearLearn java full-stack profession course. And with the NearLearn right guidance, you can obtain your dream job. NearLearn provides the most promising curriculum to provide a direct method to the expert world with complete know-how of Java full stack.
WEB: https://nearlearn.com/courses/java-full-stack-online-training
Email: [email protected]
Enquiry: +91-80-41700110
ADD: No: 61,1st Floor, 7th Main, 12th Cross Rd, BTM 2nd Stage, Bengaluru, Karnataka 560076
Facebook page: https://www.facebook.com/NearLearnGlobal/
Instagram: https://www.instagram.com/near_learn/?hl=en
Linkedin: https://in.linkedin.com/company/nearandlearn
Twitter: https://twitter.com/nearlearn/status/1253662336390324224
0 notes
Text
2023 GUIDE: ON HOW TO START JAVA FULL STACK CAREER

Starting a career in Java full-stack development can be a rewarding and challenging experience. The demand for skilled full-stack developers is constantly growing and the technology is widely used in a variety of industries. In this guide, we will discuss the steps you need to take to start a successful career in Java full-stack development.
Learn the fundamentals of Java programming: Before you dive into full-stack development, it's important to have a strong understanding of Java programming. You can start by learning basic Java concepts like data types, control structures, and object-oriented programming.
Gain experience with front-end development: Full-stack developers need to be proficient in front-end development as well as back-end development. You can start by learning HTML, CSS, and JavaScript.
Familiarize yourself with Java frameworks: There are several popular Java frameworks, such as Spring and Hibernate, that are widely used in full-stack development. Familiarizing yourself with these frameworks will give you a strong foundation for your full-stack career.
Learn database management: Full-stack developers need to be able to work with databases, so it's important to learn SQL and database management concepts.
Build projects: The best way to gain experience and improve your skills is by building projects. You can start by building simple projects like a to-do list application, and then move on to more complex projects.
Keep learning: The technology and best practices in full-stack development are constantly changing, so it's important to stay up to date by reading blogs, attending meetups, and participating in online communities.
Get certified: A certification in Java full-stack development can help validate your skills and show potential employers that you have a strong understanding of the technology.
Read More: Where to learn Java full-stack and why it can benefit your career.
Starting a career in Java full-stack development requires dedication and hard work, but with the right education and experience, you can become a successful full-stack developer. By following these steps, you'll be well on your way to a fulfilling and lucrative career in the field.
Can I learn full stack in 1 year?
Yes, it is possible to learn full-stack development in one year, but it depends on your previous experience, learning style, and dedication. Full stack development involves learning front-end technologies like HTML, CSS, and JavaScript, as well as back-end technologies like Java, databases, and server-side programming.
If you are new to programming and have a full-time job or other responsibilities, it may take you longer than a year to become a full-stack developer. However, if you are able to dedicate a significant amount of time to learning and practising, it is possible to make significant progress in a year.
The key to successfully learning full-stack development in one year is to stay focused and consistently learn and practice. Enrol in online courses, attend workshops and events, build projects, and get involved in the developer community to continually improve your skills.
Ultimately, the pace of your learning will depend on your individual circumstances and goals. But with dedication and hard work, learning full stack development in one year is definitely achievable.
Is Java developer a good career in 2023?
Yes, Java development is a good career in 2023. Java is a widely used programming language and has been around for over two decades, making it one of the most stable and in-demand programming languages in the industry.
In 2023, the demand for Java developers is expected to remain high, particularly in industries such as finance, healthcare, and e-commerce, which rely on Java for building complex and secure applications. Java developers with experience in developing scalable and secure applications will continue to be in high demand in the coming years.
In addition, Java is an object-oriented programming language that is easy to learn and is known for its versatility, making it a good choice for developers who want to build a diverse range of applications.
Read More: Python Vs JavaScript: Which One Is Better?
In conclusion, Java development is a good career choice in 2023 due to its stability, versatility, and high demand in the industry. Whether you are just starting out in your career or looking for a change, Java development offers many opportunities for growth and advancement.
0 notes
Text
2023 Guide: on how to start a career in ml

Starting a career in Machine Learning (ML) can be a lucrative and rewarding experience. With the rise of technology, businesses and organizations are constantly looking for experts who can help them harness the power of ML. In this article, we will guide you on how to start your career in ML.
Gain a strong foundation in mathematics and computer science: ML is built on a foundation of mathematics, statistics, and computer science. It's important to have a good understanding of linear algebra, calculus, and probability.
Learn programming languages: ML is usually done in Python or R, so it's essential to learn one or both of these programming languages.
Get hands-on experience: The best way to learn ML is to work on projects. Participate in online ML challenges and hackathons, or build your own projects.
Get familiar with ML frameworks: ML frameworks such as TensorFlow, PyTorch, and Caffe make it easier to build ML models. Get comfortable with at least one of these frameworks.
Stay updated with the latest developments: ML is a fast-evolving field, so it's important to stay updated with the latest developments and trends. Follow ML blogs, attend conferences and workshops, and participate in online forums.
Build a strong portfolio: A strong portfolio is essential for landing a job in ML. Showcase your ML projects and the problems you solved with ML.
Network with ML experts: ML is a field where networking is key. Attend ML meetups, conferences, and workshops, and connect with other ML experts.
Read More: Machine Learning Demanding & Diverse Career Path & Salary in India: Why 2023 is the Game Changer for Machine Learning Engineer?
How many months does it take to learn ML?
The amount of time it takes to learn Machine Learning (ML) varies depending on several factors such as prior experience, learning style, and the amount of time and effort you are willing to invest. On average, it can take anywhere from 6 months to a year of dedicated learning to become proficient in ML.
For someone who already has a strong foundation in mathematics, statistics, and computer science, and is familiar with programming, it might take less time. However, for someone who is new to these fields, it may take longer.
It's also important to note that ML is a constantly evolving field and learning is a continuous process. Even after you become proficient in ML, you will need to continuously update your knowledge and skills to stay current in the field.
In summary, the amount of time it takes to learn ML is not set in stone and can vary widely, but with dedicated effort and a willingness to continuously learn and grow, it is possible to become proficient in ML in 6 months to a year.
Is it easy to get a job in ML?
Getting a job in Machine Learning (ML) can be competitive, but it is not necessarily difficult. The demand for ML professionals is high due to the increasing use of ML in various industries. However, the job market is also becoming more competitive, with many qualified candidates applying for the same positions.
To increase your chances of getting a job in ML, it's important to have a solid understanding of the fundamental concepts of ML, as well as hands-on experience with building ML models. Additionally, having a strong portfolio of projects and a network of connections in the field can be valuable.
Overall, while it may not be easy to get a job in ML, it is achievable with the right skills, experience, and preparation.
How do freshers get ML jobs?
For freshers, getting a job in Machine Learning (ML) can be a challenge, but it's not impossible. Here are some steps you can take to increase your chances of getting an ML job:
Build a strong foundation in mathematics, statistics, and computer science: ML is built on a foundation of mathematics, statistics, and computer science. Make sure you have a good understanding of linear algebra, calculus, and probability.
Learn programming languages: ML is usually done in Python or R, so it's essential to learn one or both of these programming languages.
Get hands-on experience: The best way to learn ML is to work on projects. Participate in online ML challenges and hackathons, or build your own projects.
Get familiar with ML frameworks: ML frameworks such as TensorFlow, PyTorch, and Caffe make it easier to build ML models. Get comfortable with at least one of these frameworks.
Stay updated with the latest developments: ML is a fast-evolving field, so it's important to stay updated with the latest developments and trends. Follow ML blogs, attend conferences and workshops, and participate in online forums.
Build a strong portfolio: A strong portfolio is essential for landing a job in ML. Showcase your ML projects and the problems you solved with ML.
Network with ML experts: ML is a field where networking is key. Attend ML meetups, conferences, and workshops, and connect with other ML experts.
Apply for entry-level positions: Many companies offer entry-level positions for freshers. Look for roles such as ML engineer, data scientist, or ML analyst.
Apply for internships: Internships are a great way to gain hands-on experience and build your ML skills. Look for internships in companies that use ML.
Read More: Top 10 Highly Recommended Machine Learning Software!
In conclusion, starting a career in ML requires a combination of technical skills and hands-on experience. By following these steps, you will be well on your way to a successful ML career.
0 notes
Text
How to become a java expert in 2023

Java is widely used for developing enterprise applications, web applications, and mobile apps. It is also the primary language for developing Android apps. One of the most notable features of Java is that it uses a "write once, run anywhere" (WORA) approach, meaning that once a program is written and compiled, it can be run on any platform that has a Java Virtual Machine (JVM) installed. Becoming a Java expert in 2023 will require a combination of education and practical experience. Here are some steps you can take to achieve this goal:
Learn the fundamentals of the Java programming language by studying its syntax, data types, and control structures.
Learn the basics of object-oriented programming (OOP) concepts like classes, objects, inheritance, and polymorphism.
Learn the standard libraries and frameworks that are commonly used in Java development, such as the Spring framework and Hibernate.
Get hands-on experience by building Java-based applications and experimenting with different tools and techniques.
Stay up-to-date with the latest developments in the Java ecosystem by following blogs, and podcasts, and attending conferences.
Join online communities and forums where Java developers share their knowledge and experience.
Keep practising, learn from your mistakes and try to work on projects that are challenging and outside your comfort zone.
Consider getting certified as a Java Developer if you want to add more credibility to your skills.
Is Java in demand in 2023?
Java is one of the most popular programming languages and has been in demand for many years. It is widely used for developing enterprise applications, web applications, and mobile apps. Java is also the primary language for developing Android apps. As of 2023, it is still in demand and is expected to continue to be a widely used programming language.
However, it's important to note that technology is constantly evolving and new programming languages and frameworks are emerging. It's always good to stay informed about current trends in the industry and to diversify your skill set.
Read More: Join the Best Machine Learning & AI Courses.
HOW LONG DOES IT TAKE TO BECOME JAVA EXPERT?
The length of time it takes to become an expert in Java depends on several factors, including your prior experience with programming, your learning style, and the amount of time you can dedicate to studying and practising.
On average, it can take several months to a year or more to become proficient in Java, and several more years of experience to become an expert.
To become proficient in Java, you should first learn the basics of the language, and then continue to practice and build projects. It's also important to stay current with updates and new features in the language, as well as industry best practices.
It's important to note that becoming an expert in Java, or in any field, takes time and dedication. It's not only about the time you spend learning but also about the time you spend applying your knowledge and gaining real-world experience.
0 notes
Text
HOW TO BECOME A MACHINE LEARNING PRO IN 2023

To become a machine learning professional, you should start by gaining a solid understanding of the fundamental concepts and mathematical foundations of the field. This can be done through taking online courses, reading books and research papers, and practising with hands-on projects. Some key areas to focus on include:
Linear algebra and calculus
Probability and statistics
Programming
Specific machine learning algorithms
Deep learning
Read More: Become a Machine Learning Expert in 2023.
Once you have a strong foundation in these areas, you should continue to build your skills by working on projects and participating in machine learning competitions. It's also important to stay current with the latest advancements and research in the field.
Linear algebra and calculus
Linear algebra and calculus are both used extensively in machine learning. Linear algebra provides the mathematical foundation for many of the algorithms used in machine learning, such as matrix operations and eigendecompositions. Calculus is used for optimisation, which is a key component in many machine learning algorithms. For example, gradient descent, which is used to train many types of neural networks, relies heavily on calculus to adjust the model's parameters in order to minimize the error. Additionally, Calculus also helps in understanding the behaviour of functions and their local minima/maxima, which is useful in understanding the optimization techniques used in ML. Overall, Linear Algebra and Calculus are essential tools for understanding and implementing many machine learning algorithms.
Probability and statistics
Probability and statistics are fundamental concepts that are used extensively in machine learning. They are used to model and analyze data, make predictions, and evaluate the performance of machine learning models.
Probability is used to represent the uncertainty in data, which is often modelled using probability distributions. This is important for understanding and modelling the relationships between variables in the data, and for making predictions.
Statistics are used to summarize and describe the data, and to make inferences about the underlying population from a sample of data. This is important for understanding the characteristics of the data, and for selecting appropriate models and algorithms for the task at hand.
Probability and statistics are used for feature selection, feature engineering, and model selection. They also play a key role in evaluating and comparing the performance of different machine learning models. For example, hypothesis testing, p-value, Bayesian inference, Maximum Likelihood Estimation, etc are all statistical concepts used in ML.
Overall, probability and statistics are essential tools for understanding and working with data in machine learning, and for developing and evaluating machine learning models.
3. Programming
Programming is an essential tool for implementing machine learning algorithms and building machine learning systems. It allows data scientists and engineers to translate mathematical models and algorithms into working code that can be run on a computer.
In machine learning, programming is used to:
Collect, clean and prepare the data for modelling.
Implement and test different machine learning algorithms and models.
Train and fine-tune models using large datasets.
Evaluate the performance of models using metrics like accuracy and error.
Deploy machine learning models in production environments, such as web applications or mobile apps.
Popular programming languages used in machine learning include Python, R, Matlab, Java and C++. These languages have a wide range of libraries and frameworks that make it easy to implement machine learning algorithms, such as TensorFlow, scikit-learn, and Keras.
Overall, programming is a critical skill for anyone working in machine learning, as it allows them to implement and test the models they develop, and to build systems that can be used in real-world applications.
4. how do Specific machine learning algorithms help in learning machine learning?
Different machine learning algorithms have different strengths and weaknesses and are suited for different types of tasks and datasets. Some common examples include:
Linear regression and logistic regression are simple and easy to understand and are often used for basic prediction tasks.
Decision trees and random forests are powerful for classification and regression tasks and can handle non-linear relationships and missing data.
Support vector machines (SVMs) are effective for high-dimensional and non-linearly separable data.
Neural networks and deep learning are extremely powerful and flexible and are used for a wide range of tasks including image and speech recognition, natural language processing and more.
k-nearest neighbours is a simple algorithm that is used for classification and regression tasks.
Gradient Boosting Machine (GBM) is used for both classification and regression tasks and is a powerful algorithm for handling imbalanced and non-linearly separable data.
There are many other algorithms such as Naive Bayes, K-means, etc which are used for specific tasks.
In summary, different machine learning algorithms are well suited for different types of datasets and tasks, and choosing the right algorithm for a specific problem can make a big difference in the performance of a machine learning model.
Read More: Gain the best machine Learning Knowledge by NearLearn Blogs.
5. Deep learning
Deep learning is a subset of machine learning that uses artificial neural networks with multiple layers to learn and make predictions or decisions. These neural networks are inspired by the structure and function of the human brain and are used for tasks such as image and speech recognition, natural language processing, and decision-making. Deep learning algorithms can automatically learn features from large amounts of data, making them particularly useful for tasks where traditional rule-based approaches are not feasible.
0 notes
Text
Java full stack route in Bangalore with the aid of NearLearn

Becoming a full-stack Java developer through enrolling in NearLearn java full-stack profession course. And with the NearLearn right guidance, you can obtain your dream job. NearLearn provides the most promising curriculum to provide a direct method to the expert world with complete know-how of Java full stack.
WEB: https://nearlearn.com/courses/java-full-stack-online-training
Email: [email protected]
Enquiry: +91-80-41700110
ADD: No: 61,1st Floor, 7th Main, 12th Cross Rd, BTM 2nd Stage, Bengaluru, Karnataka 560076
Facebook page: https://www.facebook.com/NearLearnGlobal/
Instagram: https://www.instagram.com/near_learn/?hl=en
Linkedin: https://in.linkedin.com/company/nearandlearnTwitter: https://twitter.com/nearlearn/status/1253662336390324224
0 notes
Text
MACHINE LEARNING IN FINANCE
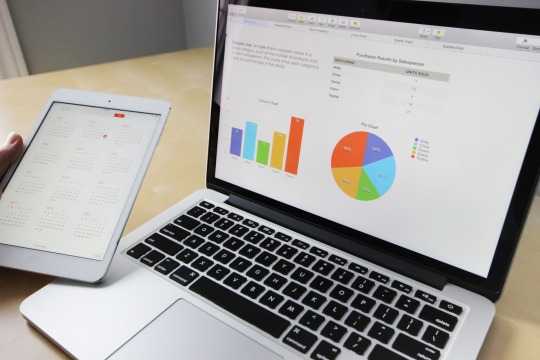
What is Machine Learning (in Finance)?
A form of artificial intelligence known as machine learning enables software systems to automatically learn from experience and get better over time without being explicitly designed. Machine learning techniques can be applied to the financial industry to evaluate financial data and provide forecasts or suggestions. A machine learning model, for instance, could be taught to spot fraudulent credit card transactions or estimate the likelihood that a borrower will default on a loan. Additionally, trading decisions can be made using machine learning algorithms to find trends in the financial markets.
Machine learning is widely used in finance for a variety of tasks, including:
Fraud detection: Algorithms trained in machine learning can spot patterns and irregularities in financial transactions that can point to fraud. In comparison to more conventional techniques, this can assist financial institutions in detecting fraudulent behaviour more quickly and accurately.
Credit risk assessment: Machine learning can be used to foretell a borrower's propensity for loan default. Financial organisations may be able to better manage credit risk and make better lending decisions as a result.
Trading algorithms can be created using machine learning to make judgements about buying and selling financial assets based on a variety of market data. Compared to manually entering deals, this can assist traders with doing so more swiftly and accurately.
The performance of a financial manager's investment portfolios can be optimised with the aid of machine learning. To do this, trends in historical market data can be found and used to forecast the state of the market in the future.
Customer segmentation: By analysing customer data and classifying customers into distinct categories based on their financial behaviours, machine learning algorithms can be utilised by financial institutions to create more successful targeted marketing campaigns.
Automating the process of adhering to financial regulations is possible using machine learning. This can shorten the time and expense of compliance while assisting financial institutions in ensuring that they are in compliance with regulations.
These are only a handful of the numerous applications of machine learning in finance. Machine learning in finance is a rapidly developing topic, and new applications are being created all the time.
Should finance professionals learn machine learning?
Learning machine learning can help financial professionals make better predictions and judgements and acquire insights from massive amounts of data. Machine learning can be used, for instance, to forecast stock prices, spot fraud, or find patterns in financial data that might guide investment choices. Furthermore, as machine learning in finance gains popularity, having an understanding of the topic may increase a professional's value in the job market.
Read More: Join java full stack and machine learning course provided by NearLearn.
Machine learning is one tool that may be used to evaluate financial data, but it is crucial to remember that it may not be the ideal option in every situation. Finance experts should also be well-versed in the underlying financial theories and models, as well as the constraints of the data they use. Furthermore, it's crucial to be able to explain and convey to stakeholders the findings of any analysis or models.
As a result, while understanding machine learning as a finance professional may be advantageous, it's crucial to remember that it should not be employed as a stand-alone solution but rather as a component of a larger set of skills and knowledge in the field of finance.
0 notes