#siemens digital twin case study
Explore tagged Tumblr posts
Text
Title: Laser Technology in PCD Tool Manufacturing: Key Innovations, Industry Leaders, and Future Trends
1. Overview of Laser Applications in PCD Tool Machining 1.1: Why Lasers Dominate PCD Tool Manufacturing Polycrystalline diamond (PCD), with its extreme hardness (7,000–8,000 HV) and wear resistance, is critical for precision cutting tools, semiconductor packaging, and automotive components. However, traditional mechanical methods struggle with PCD’s hardness, leading to rapid tool wear and low accuracy. Laser technology has emerged as a game-changer, offering:
Non-contact processing: Eliminates tool wear. Ultra-fine heat-affected zones (HAZ <5 μm): Preserves material integrity. Complex geometry shaping: Enables intricate tool designs.
2: Core Laser Technologies & Challenges 2.1Ultrafast Lasers (Femtosecond/Picosecond)
Femtosecond lasers (e.g., Coherent Monaco Series): Pulse width <500 fs: Achieves nanoscale precision for micro-tools (<0.1 mm edges). Zero thermal damage: Ideal for edge preparation of micro-drills. Picosecond lasers (e.g., Trumpf TruMicro 5000): 5–10 ps pulses: Balances speed and accuracy for separating PCD composites (diamond + carbide). 2.2 Water-Guided Laser (Laser MicroJet)
Synova LCS 150: Combines laser beams with high-pressure water jets to reduce thermal stress, achieving surface roughness Ra <0.2 μm (critical for aerospace tools).
2.3 Multi-Axis Laser Systems
DMG MORI Lasertec 65 Shape: Integrates 5-axis motion and real-time monitoring for spiral grooves and 3D chip breakers in a single setup. Key Challenges:
Thermal management: PCD’s high thermal conductivity (2,000 W/m·K) demands precise energy control. Interfacial bonding: Thermal expansion mismatch between diamond and carbide causes delamination.
3: Top 5 Companies Driving Innovation 3.1 Ewag AG (Switzerland)
Flagship Product: Laser Line Ultra Series Tech Highlights: Femtosecond lasers + adaptive optics: ±1 μm edge control for micro-tools (0.05–3 mm diameter). AI-powered optimization: 30% faster processing via machine learning. Case Study: Supplies Apple with iPhone PCB micro-drills (500,000 holes per edge).
3.2. COBORN (UK)
Flagship Product: ORBIT 360° Laser System Tech Highlights: Ring-shaped beam: Minimizes edge chipping (taper angle <0.5°). Multi-wavelength compatibility: Adapts to PCD grain sizes (2–30 μm). Clients: Volkswagen, Bosch (crankshaft machining tools).
3.3 Synova (Switzerland)
Flagship Product: Laser MicroJet Hybrid Tech Highlights: Water-cooled laser: HAZ reduced to 2 μm; Ra 0.1 μm surface finish. 20:1 aspect ratio: Deep-hole drilling for fuel injectors. Partnership: Sandvik wind turbine gearbox tools (40% cost reduction).
3.4 Coherent (USA)
Flagship Product: HighLight FL4000 Femtosecond Laser Tech Highlights: 400 W average power: Cuts 3 mm PCD sheets in 5 minutes. Beam shaping: Square/ring profiles for complex edges. Application: Tesla 4680 battery casing tools (99.5% yield).
3.5 TKD CO.,LTD (China)
Flagship Product: TKD-300F/TKD-023F Tech Highlights: Ultra-precision optics: 0.06 mm line width at 3 m/s. Fiber laser system: Maintenance-free, high-accuracy cutting. Markets: PCD tools, ceramic machining (e.g., TSMC 3nm wafer dicing).
4: Future Trends & Challenges (2025–2030) 4.1. Hybrid Manufacturing Techniques
Laser + ECM/ultrasonic: GF Machining Solutions’ hybrid systems address micro-cracks at PCD-carbide interfaces.
4.2. Smart Manufacturing
Digital twins: Siemens NX reduces trial costs by 60% via full-process simulation.
4.3. Sustainability Demands
EU Green Tool Directive: Mandates carbon footprint <5 kg CO₂/kg, driving renewable energy adoption. Key Challenges:
High costs: Femtosecond systems exceed $2 million, limiting SME access. Lack of standards: No global certification for laser-based PCD processes.
5: Conclusion: Precision, Power, and Sustainability Laser technology is redefining PCD tool manufacturing:
Precision pioneers (Ewag, Synova) focus on submicron accuracy. Industrial giants (Coherent, DMG) leverage high-power, smart systems. Niche experts (TKD, COBORN) dominate sector-specific applications. With the EV and semiconductor boom, the laser-processed PCD tool market is projected to grow at 18% CAGR, reaching $2.5 billion by 2030. Companies must prioritize precision, efficiency, and eco-design to stay competitive.
1 note
·
View note
Text
Smart Factories: The Next Leap in Manufacturing
As the manufacturing sector evolves, the rise of smart factories marks a transformative leap into the future. Powered by the Internet of Things (IoT), predictive maintenance, and advanced robotics, these intelligent production environments are redefining efficiency, innovation, and scalability across industries worldwide. In this blog, we’ll explore how these technologies drive change, examine global trends, and highlight real-world case studies showcasing their profound impact.
The Essence of Smart Factories
A smart factory is more than an automated facility; it’s an ecosystem where physical production processes are seamlessly integrated with digital technologies. Leveraging interconnected devices, data analytics, and intelligent automation, smart factories enhance operational efficiency while minimizing downtime and waste. They embody the principles of Industry 4.0, propelling manufacturing into a digitally optimized future.
Key pillars of smart factories include:
IoT-enabled Devices: Real-time monitoring and communication across machines and systems.
Predictive Maintenance: Anticipating failures before they occur, reducing costly disruptions.
Robotics and AI: Enhancing precision, speed, and adaptability in production lines.
Digital Twins: Virtual simulations of physical processes to optimize design and operation.
The Role of IoT in Manufacturing
The Internet of Things (IoT) is the backbone of smart factories, enabling devices, sensors, and systems to exchange data in real-time. This connectivity drives unprecedented transparency, allowing manufacturers to monitor operations and make data-driven decisions.
Global Trends in IoT Manufacturing
Edge Computing: Processing data closer to where it’s generated reduces latency, enabling faster responses in production environments.
5G Connectivity: Ultra-fast, low-latency networks are critical for real-time communication between IoT devices.
Smart Supply Chains: IoT enhances supply chain visibility, tracking goods from raw materials to finished products.
Case Study: Siemens Amberg Electronics Plant
At Siemens’ Amberg plant in Germany, IoT plays a pivotal role. The factory achieves a production efficiency of over 99% by connecting 1,000 IoT devices across its production line. These devices analyze data to detect anomalies, optimize workflows, and maintain product quality.
Predictive Maintenance: The Game-Changer
Traditional maintenance models often involve reactive or scheduled maintenance, which can lead to unnecessary costs or unexpected downtime. Predictive maintenance, driven by IoT and AI, changes the game by identifying potential equipment failures before they happen.
How Predictive Maintenance Works
Data Collection: Sensors on machines gather data on temperature, vibration, and other parameters.
Analysis: AI algorithms detect patterns indicating wear or failure.
Actionable Insights: Operators receive alerts to perform maintenance at the optimal time.
Benefits of Predictive Maintenance
Reduced Downtime: Machines are serviced only when needed, ensuring maximum uptime.
Cost Efficiency: Minimizes unnecessary maintenance and avoids expensive repairs.
Extended Equipment Lifespan: Prevents excessive wear and tear.
Case Study: General Motors (GM)
GM employs predictive maintenance across its assembly plants using IoT sensors and analytics platforms. This approach has resulted in a 50% reduction in unexpected downtime, saving millions annually while improving production efficiency.
Advanced Robotics: The Workforce of the Future
Robotics has been integral to manufacturing for decades, but today’s smart robots are powered by AI, making them more versatile and capable than ever. These robots not only execute repetitive tasks with precision but also adapt to complex environments.
Trends in Robotics
Collaborative Robots (Cobots): Designed to work alongside humans, cobots enhance productivity without replacing human workers.
AI-powered Robots: Machine learning enables robots to learn and improve over time, adapting to new tasks.
Autonomous Mobile Robots (AMRs): These robots navigate factory floors independently, transporting materials efficiently.
Case Study: Tesla Gigafactories
Tesla’s Gigafactories exemplify cutting-edge robotics. Robots perform tasks such as welding, assembly, and material handling, ensuring consistency and speed in electric vehicle production. The integration of AI enables these robots to adjust operations dynamically, reducing errors and waste.
Digital Twins: Bridging the Physical and Digital Worlds
A digital twin is a virtual replica of a physical system, enabling manufacturers to simulate, monitor, and optimize operations. This technology provides invaluable insights, from design to production, enhancing both speed and accuracy.
Applications of Digital Twins
Product Design: Simulate prototypes to refine designs without costly physical trials.
Process Optimization: Test and adjust manufacturing workflows digitally.
Performance Monitoring: Track real-time performance and predict potential issues.
Case Study: Boeing
Boeing uses digital twins to streamline aircraft production. By simulating assembly processes, the company reduces errors and shortens production cycles, ensuring faster delivery without compromising quality.
Global Trends Shaping Smart Factories
1. Sustainability
Smart factories prioritize energy efficiency and waste reduction. By analyzing energy usage patterns, manufacturers can minimize their environmental footprint. Example: Schneider Electric’s smart factory in India reduced energy consumption by 10% and water usage by 20% using IoT and analytics.
2. Resilience
The COVID-19 pandemic highlighted the need for resilient supply chains. Smart factories enhance agility, enabling manufacturers to adapt quickly to disruptions. Example: Procter & Gamble leveraged IoT and AI to maintain production during the pandemic, ensuring consistent supply despite fluctuating demand.
3. Workforce Evolution
Automation does not eliminate the need for human workers; instead, it shifts their roles toward higher-value tasks like strategy and oversight. Upskilling programs are critical to prepare the workforce for this transition. Example: Foxconn’s “automation-first” strategy includes extensive training programs to reskill employees for new roles alongside robots.
Challenges and the Path Forward
While smart factories offer immense potential, they also face challenges:
Cybersecurity: Increased connectivity poses risks of cyberattacks. Robust security protocols are essential.
High Initial Costs: The adoption of advanced technologies requires significant investment.
Skill Gaps: The transition to smart manufacturing demands a skilled workforce comfortable with digital tools.
Future Outlook
Despite these challenges, the momentum toward smart factories is unstoppable. By 2030, the global smart manufacturing market is projected to reach $514 billion, driven by advances in AI, IoT, and robotics. Governments and private sectors must collaborate to address barriers, ensuring equitable access to these transformative technologies.
Conclusion: A New Era of Manufacturing
Smart factories represent the pinnacle of manufacturing innovation, combining IoT, predictive maintenance, robotics, and digital twins to revolutionize the industry. From enhanced efficiency to greater sustainability, the benefits are undeniable. As companies like Siemens, GM, and Tesla lead the way, the future of manufacturing promises to be smarter, faster, and more resilient.
The next leap in manufacturing is already here. Embracing smart factory technology isn’t just an option — it’s an imperative for companies aiming to stay competitive in an increasingly dynamic global market.
So, is your business ready to step into the future of manufacturing?
#Tech4bizsolutions #SmartFactories #Industry40 #ManufacturingInnovation#DigitalTransformation #IoTInManufacturing #AIInManufacturing #PredictiveMaintenance #IndustrialAutomatio #RoboticsInManufacturing #SmartManufacturing #FactoryOfTheFuture#AdvancedManufacturing #TechInIndustry #AutomationRevolution #SustainableManufacturing#DigitalTwins #SupplyChainInnovation #FutureOfManufacturing #IndustrialIoT #ManufacturingTechnology
0 notes
Text
21 ways medical digital twins will transform health care
Where does your enterprise stand on the AI adoption curve? Take our AI survey to find out.
The health care industry is starting to adopt digital twins to improve personalized medicine, health care organization performance, and new medicines and devices. Although simulations have been around for some time, today’s medical digital twins represent an important new take. These digital twins can create useful models based on information from wearable devices, omics, and patient records to connect the dots across processes that span patients, doctors, and health care organizations, as well as drug and device manufacturers.
It is still early days, but the field of digital twins is expanding quickly based on advances in real-time data feeds, machine learning, and AR/VR. As a result, digital twins could dramatically shift how we diagnose and treat patients, and help realign incentives for improving health. Some proponents liken the current state of digital twins to where the human genome project was 20 years ago, and it may require a similar large-scale effort to take shape fully. A team of Swedish researchers recently wrote, “Given the importance of the medical problem, the potential of digital twins merits concerted research efforts on a scale similar to those involved in the HGP.”
While such a “moon shot” effort may not be immediately underway, there are many indicators that digital twins are gaining traction in medicine. Presented here are 21 ways digital twins are starting to shape health care today, broken roughly into personalized medicine, improving health care organizations, and drug and medical devices and development. In fact, many types of digital twins span multiple use cases and even categories; it is these cross-domain use-cases that form a major strength of digital twins.
Personalized medicine
Digital twins show tremendous promise in making it easier to customize medical treatments to individuals based on their unique genetic makeup, anatomy, behavior, and other factors. As a result, researchers are starting to call on the medical community to collaborate on scaling digital twins from one-off projects to mass personalization platforms on par with today’s advanced customer data platforms.
1. Virtual organs
Several vendors have all been working on virtual hearts that can be customized to individual patients and updated to understand the progression of diseases over time or understand the response to new drugs, treatments, or surgical interventions. Philip HeartModel simulates a virtual heart, starting with the company’s ultrasound equipment. Siemens Healthineers has been working on a digital twin of the heart to improve drug treatment and simulate cardiac catheter interventions. European startup FEops has already received regulatory approval and commercialized the FEops Heartguide platform. It combines a patient-specific replica of the heart with AI-enabled anatomical analysis to improve the study and treatment of structural heart diseases.
Dassault launched its Living Heart Project in 2014 to crowdsource a virtual twin of the human heart. The project has evolved as an open source collaboration among medical researchers, surgeons, medical device manufacturers, and drug companies. Meanwhile, the company’s Living Brain project is guiding epilepsy treatment and tracking the progression of neurodegenerative diseases. The company has organized similar efforts for lungs, knees, eyes, and other systems.
“This is a missing scientific foundation for digital health able to power technologies such as AI and VR and usher in a new era of innovation,” Dassault senior director of virtual human modeling Steve Levine told VentureBeat. He added that this “could have an even greater impact on society than what we have seen in telecommunications.”
2. Genomic medicine
Swedish researchers have been mapping mice RNA into a digital twin that can help predict the effect of different types and doses of arthritis drugs. The goal is to personalize human diagnosis and treatment using RNA. The researchers observed that medication does not work about 40% to 70% of the time. Similar techniques are also mapping the characteristics of human T-cells that play a crucial role in immune defense. These maps can help diagnose many common diseases earlier when they are more effective and cheaper to treat.
3. Personalized health information
The pandemic has helped fuel the growth of digital health services that help people assess and address simple medical conditions using AI. For example, Babylon Health‘s Healthcheck App captures health data into digital twins. It works with manually entered data such as health histories, a mood tracker, symptom tracker, and automatic capture from fitness devices and wearables like the Apple Watch. The digital twin can provide basic front-line information or help guide priorities and interactions with doctors to address more severe or persistent conditions.
4. Customize drug treatment
The Empa research center in Switzerland is working on digital twins to optimize drug dosage for people afflicted by chronic pain. Characteristics such as age and lifestyle help customize the digital twin to help predict the effects of pain medications. In addition, patient reports about the effectiveness of different dosages calibrate digital twin accuracy.
5. Scanning the whole body
Most approaches to digital twins build on existing equipment to capture the appropriate data, while Q Bio’s new Gemini Digital Twin platform starts with a whole-body scan. The company claims to capture a whole-body scan in 15 minutes without radiation or breath holds, using advanced computational physics models that are more precise than conventional MRI for many diagnoses. The company has received over $80 million from Andreessen Horowitz, Kaiser Foundation Hospitals, and others. Q Bio is also developing integrations to improve these models using data from genetics, chemistry, anatomy, lifestyle, and medical history.
6. Planning surgery
A Boston hospital has been working with Dassault’s digital heart to improve surgical procedure planning and assess the outcomes afterward. The digital twins also help them to generate the shape of a cuff between the heart and arteries.
Sim&Cure’s Sim&Size is a digital twin to help brain surgeons treat aneurysms using simulations to improve patient safety. Aneurysms are enlarged blood vessels that can result in clots or strokes. These digital twins can improve the ability to plan and execute less invasive surgery using catheters to install unique implants. Data from individual patients helps customize simulations that run on an embedded simulation package from Ansys. Preliminary results have dramatically reduced the need for follow-up surgery.
Improving health care organizations
Digital twins also show promise in improving the way health care organizations deliver care. Gartner coined the term digital twin of the organizations to describe this process of modeling how an organization operates to improve underlying processes.
In most industries, this can start by using process mining to discover variations in business processes. New health care-specific tools can complement these techniques.
7. Improving caregiver experience
Digital twins can also help caregivers capture and find information shared across physicians and multiple specialists. John Snow Labs CTO David Talby said, “We’re generating more data than ever before, and no one has time to sort through it all.” For example, if a person sees their regular primary care physician, they will have a baseline understanding of the patient, their medical history, and medications. If the same patient sees a specialist, they may be asked many of the same repetitive questions.
A digital twin can model the patient and then use technologies like NLP to understand all of the data and cut through the noise to summarize what’s going on. This saves time and improves the accuracy of capturing and presenting information like specific medications, health conditions, and more details that providers need to know in context to make clinical decisions.
8. Driving efficiency
The GE Healthcare Command Center is a major initiative to virtualize hospitals and test the impact of various decisions on changes in overall organizational performance. Involved are modules for evaluating changes in operational strategy, capacities, staffing, and care delivery models to objectively determine which actions to take. For example, they have developed modules to estimate the impact of bed configurations on care levels, optimize surgical schedules, improve facility design, and optimize staff levels. This allows managers to test various ideas without having to run a pilot. Dozens of organizations are already using this platform, GE said.
9. Shrinking critical treatment window
Siemens Healthineers has been working with the Medical University of South Carolina to improve the hospital’s daily routine through workflow analysis, system redesign, and process improvement methodologies. For example, they are working to reduce the time to treat stroke patients. This is important since early treatment is critical but requires the coordination of several processes to perform smoothly.
10. Value-based health care
The rising cost of health care has many nations exploring new incentive models to better align new drugs, interventions, and treatments with outcomes. Value-based health care is one approach that is growing in popularity. The basic idea is that participants, like drug companies, will only get compensation proportionate to their impact on the outcomes. This will require the development of new types of relationships across multiple players in the health delivery systems. Digital twins could provide the enabling infrastructure for organizing the details for crafting these new types of arrangements.
11. Supply chain resilience
The pandemic illustrated how brittle modern supply chains could be. Health care organizations immediately faced shortages of essential personal protection equipment owing to shutdowns and restrictions from countries like China. Digital twins of a supply chain can help health care organizations model their supply chain relationships to understand better how to plan around new events, shutdowns, or shortages. This can boost planning and negotiations with government officials in a pinch, as was the case in the recent pandemic. A recent Accenture survey found that 87% of health care executives say digital twins are becoming essential to their organization’s ability to collaborate in strategic ecosystem partnerships.
12. Faster hospital construction
Digital twins could also help streamline construction of medical facilities required to keep up with rapid changes, such as were seen in the pandemic. Atlas Construction developed a digital twin platform to help organize all the details for health care construction. The project was inspired long before the pandemic when Atlas founder Paul Teschner saw how hard it was to get new facilities built in remote areas of the world. The platform helps organize design, procurement, and construction processes. It is built on top of the Oracle Cloud platform and Primavera Unifier asset lifecycle management service.
13. Streamlining call center interactions
Digital twins can make it easier for customer service agents to understand and communicate with patients. For example, a large insurance provider used a TigerGraph graph database to integrate data from over 200 sources to create a full longitudinal health history of every member. “This level of detail paints a clear picture of the members current and historical medical situation,” said TigerGraph health care industry practice lead Andrew Anderson.
A holistic view of all diagnosis claims prescriptions, refills, follow-up visits, and outstanding claims reduced call handling time by 10%, TigerGraph claimed, resulting in over $100 million in estimated savings. Also, shorter but more relevant conversations between the agents and members have increased Net Promoter Score and lowered churn.
Drug and medical device development
There are many ways that digital twins can improve the design, development, testing, and monitoring of new medical devices and drugs. The U.S. FDA has launched a significant program to drive the adoption of various types of digital approaches. Regulators in the U.S. and Europe are also identifying frameworks for including modeling and simulation as sources of evidence in new drug and device approvals.
14. Software-as-a-medical device
The FDA is creating the regulatory framework to allow companies to certify and sell software-as-a-medical device. The core idea is to generate a patient-specific digital twin from different data sources, including lab tests, ultrasound, imaging devices, and genetic tests. In addition, digital twins can also help optimize the software in medical devices such as pacemakers, automated insulin pumps, and novel brain treatments.
15. Classifying drug risks
Pharmaceutical researchers are using digital twins to explore the heart risks of various drugs. This could help improve drug safety of individual drugs and drug combinations more cost-effectively than through manual testing. They have built a basic model for 23 drugs. Extending this model could help reduce the estimated $2.5 billion required to design, test, get approved, and launch new drugs.
16. Simulating new production lines
Siemens worked with several vaccine manufacturers to design and test various vaccine production line configurations. New mRNA vaccines are fragile and must be precisely combined using microfluidic production lines that precisely combine nanoscale-sized particles. Digital twins allowed them to design and validate the manufacturing devices, scale these processes, and accelerate its launch from 1 year down to 5 months.
17. Improve device uptime
Philips has launched a predictive maintenance program that collates data from over 15,000 medical imaging devices. The company is hoping that digital twins could improve uptime and help their engineers customize new equipment for the needs of different customers. In addition, it is hoping to apply similar principles across all of its medical equipment.
18. Post-market surveillance
Regulators are beginning to increase the emphasis for device makers to monitor the results of their equipment after-sales as part of a process called post-market surveillance. This requires either staffing expensive specialists to maintain the equipment or embedding digital twins capabilities into the equipment. For example, Sysmex worked with PTC to incorporate performance testing into its blood analyzer to receive a waiver from these new requirements, PTC CTO Steve Dertien told VentureBeat. This opened the market for smaller clinical settings closer to patients, which can speed diagnosis.
19. Simulating human variability
Skeletons and atlases commonly depict the perfect human. However, real-life humans typically have some minor variations in their muscles or bones that mostly go unnoticed. As a result, medical device makers struggle with how common anatomical variations among people may affect the fit and performance of their equipment. Virtonomy has developed a library of common variations to help medical equipment makers test conduct studies on how these variations may affect the performance and safety of new devices. In this case, they simulate the characteristics representing common variations in a given population rather than individuals.
20. Digital twin of a lab
Modern drug development often requires testing out thousands or millions of possibilities in a highly controlled environment. A digital twin of the lab can help to automate these facilities. It can also help to prioritize tests in response to discoveries. Digital twins could also improve the reproducibility of experiments across labs and personnel in the same lab. In this quest, Artificial recently closed $21.5 million in series A funding from Microsoft and others to develop lab automation software. The company is betting that unified data models and platforms could help them jump to the front of the $10 billion lab automation market.
21. Improving drug delivery
Researchers at Oklahoma State have been working with Ansys to develop a digital twin to improve drug delivery using models of simulated lungs as part of the Virtual Human System project. They found that only about 20% of many drugs reached their target. The digital twins allowed them to redesign the drug’s particle size and composition characteristics to improve delivery efficiency to 90%.
VentureBeat
VentureBeat's mission is to be a digital town square for technical decision-makers to gain knowledge about transformative technology and transact. Our site delivers essential information on data technologies and strategies to guide you as you lead your organizations. We invite you to become a member of our community, to access:
up-to-date information on the subjects of interest to you
our newsletters
gated thought-leader content and discounted access to our prized events, such as Transform 2021: Learn More
networking features, and more
Become a member
0 notes
Text
21 ways medical digital twins will transform health care
New Post has been published on https://tattlepress.com/health/21-ways-medical-digital-twins-will-transform-health-care/
21 ways medical digital twins will transform health care

Where does your enterprise stand on the AI adoption curve? Take our AI survey to find out.
The health care industry is starting to adopt digital twins to improve personalized medicine, health care organization performance, and new medicines and devices. Although simulations have been around for some time, today’s medical digital twins represent an important new take. These digital twins can create useful models based on information from wearable devices, omics, and patient records to connect the dots across processes that span patients, doctors, and health care organizations, as well as drug and device manufacturers.
It is still early days, but the field of digital twins is expanding quickly based on advances in real-time data feeds, machine learning, and AR/VR. As a result, digital twins could dramatically shift how we diagnose and treat patients, and help realign incentives for improving health. Some proponents liken the current state of digital twins to where the human genome project was 20 years ago, and it may require a similar large-scale effort to take shape fully. A team of Swedish researchers recently wrote, “Given the importance of the medical problem, the potential of digital twins merits concerted research efforts on a scale similar to those involved in the HGP.”
While such a “moon shot” effort may not be immediately underway, there are many indicators that digital twins are gaining traction in medicine. Presented here are 21 ways digital twins are starting to shape health care today, broken roughly into personalized medicine, improving health care organizations, and drug and medical devices and development. In fact, many types of digital twins span multiple use cases and even categories; it is these cross-domain use-cases that form a major strength of digital twins.
Personalized medicine
Digital twins show tremendous promise in making it easier to customize medical treatments to individuals based on their unique genetic makeup, anatomy, behavior, and other factors. As a result, researchers are starting to call on the medical community to collaborate on scaling digital twins from one-off projects to mass personalization platforms on par with today’s advanced customer data platforms.
1. Virtual organs
Several vendors have all been working on virtual hearts that can be customized to individual patients and updated to understand the progression of diseases over time or understand the response to new drugs, treatments, or surgical interventions. Philip HeartModel simulates a virtual heart, starting with the company’s ultrasound equipment. Siemens Healthineers has been working on a digital twin of the heart to improve drug treatment and simulate cardiac catheter interventions. European startup FEops has already received regulatory approval and commercialized the FEops Heartguide platform. It combines a patient-specific replica of the heart with AI-enabled anatomical analysis to improve the study and treatment of structural heart diseases.
Dassault launched its Living Heart Project in 2014 to crowdsource a virtual twin of the human heart. The project has evolved as an open source collaboration among medical researchers, surgeons, medical device manufacturers, and drug companies. Meanwhile, the company’s Living Brain project is guiding epilepsy treatment and tracking the progression of neurodegenerative diseases. The company has organized similar efforts for lungs, knees, eyes, and other systems.
“This is a missing scientific foundation for digital health able to power technologies such as AI and VR and usher in a new era of innovation,” Dassault senior director of virtual human modeling Steve Levine told VentureBeat. He added that this “could have an even greater impact on society than what we have seen in telecommunications.”
2. Genomic medicine
Swedish researchers have been mapping mice RNA into a digital twin that can help predict the effect of different types and doses of arthritis drugs. The goal is to personalize human diagnosis and treatment using RNA. The researchers observed that medication does not work about 40% to 70% of the time. Similar techniques are also mapping the characteristics of human T-cells that play a crucial role in immune defense. These maps can help diagnose many common diseases earlier when they are more effective and cheaper to treat.
3. Personalized health information
The pandemic has helped fuel the growth of digital health services that help people assess and address simple medical conditions using AI. For example, Babylon Health‘s Healthcheck App captures health data into digital twins. It works with manually entered data such as health histories, a mood tracker, symptom tracker, and automatic capture from fitness devices and wearables like the Apple Watch. The digital twin can provide basic front-line information or help guide priorities and interactions with doctors to address more severe or persistent conditions.
4. Customize drug treatment
The Empa research center in Switzerland is working on digital twins to optimize drug dosage for people afflicted by chronic pain. Characteristics such as age and lifestyle help customize the digital twin to help predict the effects of pain medications. In addition, patient reports about the effectiveness of different dosages calibrate digital twin accuracy.
5. Scanning the whole body
Most approaches to digital twins build on existing equipment to capture the appropriate data, while Q Bio’s new Gemini Digital Twin platform starts with a whole-body scan. The company claims to capture a whole-body scan in 15 minutes without radiation or breath holds, using advanced computational physics models that are more precise than conventional MRI for many diagnoses. The company has received over $80 million from Andreessen Horowitz, Kaiser Foundation Hospitals, and others. Q Bio is also developing integrations to improve these models using data from genetics, chemistry, anatomy, lifestyle, and medical history.
6. Planning surgery
A Boston hospital has been working with Dassault’s digital heart to improve surgical procedure planning and assess the outcomes afterward. The digital twins also help them to generate the shape of a cuff between the heart and arteries.
Sim&Cure’s Sim&Size is a digital twin to help brain surgeons treat aneurysms using simulations to improve patient safety. Aneurysms are enlarged blood vessels that can result in clots or strokes. These digital twins can improve the ability to plan and execute less invasive surgery using catheters to install unique implants. Data from individual patients helps customize simulations that run on an embedded simulation package from Ansys. Preliminary results have dramatically reduced the need for follow-up surgery.
Improving health care organizations
Digital twins also show promise in improving the way health care organizations deliver care. Gartner coined the term digital twin of the organizations to describe this process of modeling how an organization operates to improve underlying processes.
In most industries, this can start by using process mining to discover variations in business processes. New health care-specific tools can complement these techniques.
7. Improving caregiver experience
Digital twins can also help caregivers capture and find information shared across physicians and multiple specialists. John Snow Labs CTO David Talby said, “We’re generating more data than ever before, and no one has time to sort through it all.” For example, if a person sees their regular primary care physician, they will have a baseline understanding of the patient, their medical history, and medications. If the same patient sees a specialist, they may be asked many of the same repetitive questions.
A digital twin can model the patient and then use technologies like NLP to understand all of the data and cut through the noise to summarize what’s going on. This saves time and improves the accuracy of capturing and presenting information like specific medications, health conditions, and more details that providers need to know in context to make clinical decisions.
8. Driving efficiency
The GE Healthcare Command Center is a major initiative to virtualize hospitals and test the impact of various decisions on changes in overall organizational performance. Involved are modules for evaluating changes in operational strategy, capacities, staffing, and care delivery models to objectively determine which actions to take. For example, they have developed modules to estimate the impact of bed configurations on care levels, optimize surgical schedules, improve facility design, and optimize staff levels. This allows managers to test various ideas without having to run a pilot. Dozens of organizations are already using this platform, GE said.
9. Shrinking critical treatment window
Siemens Healthineers has been working with the Medical University of South Carolina to improve the hospital’s daily routine through workflow analysis, system redesign, and process improvement methodologies. For example, they are working to reduce the time to treat stroke patients. This is important since early treatment is critical but requires the coordination of several processes to perform smoothly.
10. Value-based health care
The rising cost of health care has many nations exploring new incentive models to better align new drugs, interventions, and treatments with outcomes. Value-based health care is one approach that is growing in popularity. The basic idea is that participants, like drug companies, will only get compensation proportionate to their impact on the outcomes. This will require the development of new types of relationships across multiple players in the health delivery systems. Digital twins could provide the enabling infrastructure for organizing the details for crafting these new types of arrangements.
11. Supply chain resilience
The pandemic illustrated how brittle modern supply chains could be. Health care organizations immediately faced shortages of essential personal protection equipment owing to shutdowns and restrictions from countries like China. Digital twins of a supply chain can help health care organizations model their supply chain relationships to understand better how to plan around new events, shutdowns, or shortages. This can boost planning and negotiations with government officials in a pinch, as was the case in the recent pandemic. A recent Accenture survey found that 87% of health care executives say digital twins are becoming essential to their organization’s ability to collaborate in strategic ecosystem partnerships.
12. Faster hospital construction
Digital twins could also help streamline construction of medical facilities required to keep up with rapid changes, such as were seen in the pandemic. Atlas Construction developed a digital twin platform to help organize all the details for health care construction. The project was inspired long before the pandemic when Atlas founder Paul Teschner saw how hard it was to get new facilities built in remote areas of the world. The platform helps organize design, procurement, and construction processes. It is built on top of the Oracle Cloud platform and Primavera Unifier asset lifecycle management service.
13. Streamlining call center interactions
Digital twins can make it easier for customer service agents to understand and communicate with patients. For example, a large insurance provider used a TigerGraph graph database to integrate data from over 200 sources to create a full longitudinal health history of every member. “This level of detail paints a clear picture of the members current and historical medical situation,” said TigerGraph health care industry practice lead Andrew Anderson.
A holistic view of all diagnosis claims prescriptions, refills, follow-up visits, and outstanding claims reduced call handling time by 10%, TigerGraph claimed, resulting in over $100 million in estimated savings. Also, shorter but more relevant conversations between the agents and members have increased Net Promoter Score and lowered churn.
Drug and medical device development
There are many ways that digital twins can improve the design, development, testing, and monitoring of new medical devices and drugs. The U.S. FDA has launched a significant program to drive the adoption of various types of digital approaches. Regulators in the U.S. and Europe are also identifying frameworks for including modeling and simulation as sources of evidence in new drug and device approvals.
14. Software-as-a-medical device
The FDA is creating the regulatory framework to allow companies to certify and sell software-as-a-medical device. The core idea is to generate a patient-specific digital twin from different data sources, including lab tests, ultrasound, imaging devices, and genetic tests. In addition, digital twins can also help optimize the software in medical devices such as pacemakers, automated insulin pumps, and novel brain treatments.
15. Classifying drug risks
Pharmaceutical researchers are using digital twins to explore the heart risks of various drugs. This could help improve drug safety of individual drugs and drug combinations more cost-effectively than through manual testing. They have built a basic model for 23 drugs. Extending this model could help reduce the estimated $2.5 billion required to design, test, get approved, and launch new drugs.
16. Simulating new production lines
Siemens worked with several vaccine manufacturers to design and test various vaccine production line configurations. New mRNA vaccines are fragile and must be precisely combined using microfluidic production lines that precisely combine nanoscale-sized particles. Digital twins allowed them to design and validate the manufacturing devices, scale these processes, and accelerate its launch from 1 year down to 5 months.
17. Improve device uptime
Philips has launched a predictive maintenance program that collates data from over 15,000 medical imaging devices. The company is hoping that digital twins could improve uptime and help their engineers customize new equipment for the needs of different customers. In addition, it is hoping to apply similar principles across all of its medical equipment.
18. Post-market surveillance
Regulators are beginning to increase the emphasis for device makers to monitor the results of their equipment after-sales as part of a process called post-market surveillance. This requires either staffing expensive specialists to maintain the equipment or embedding digital twins capabilities into the equipment. For example, Sysmex worked with PTC to incorporate performance testing into its blood analyzer to receive a waiver from these new requirements, PTC CTO Steve Dertien told VentureBeat. This opened the market for smaller clinical settings closer to patients, which can speed diagnosis.
19. Simulating human variability
Skeletons and atlases commonly depict the perfect human. However, real-life humans typically have some minor variations in their muscles or bones that mostly go unnoticed. As a result, medical device makers struggle with how common anatomical variations among people may affect the fit and performance of their equipment. Virtonomy has developed a library of common variations to help medical equipment makers test conduct studies on how these variations may affect the performance and safety of new devices. In this case, they simulate the characteristics representing common variations in a given population rather than individuals.
20. Digital twin of a lab
Modern drug development often requires testing out thousands or millions of possibilities in a highly controlled environment. A digital twin of the lab can help to automate these facilities. It can also help to prioritize tests in response to discoveries. Digital twins could also improve the reproducibility of experiments across labs and personnel in the same lab. In this quest, Artificial recently closed $21.5 million in series A funding from Microsoft and others to develop lab automation software. The company is betting that unified data models and platforms could help them jump to the front of the $10 billion lab automation market.
21. Improving drug delivery
Researchers at Oklahoma State have been working with Ansys to develop a digital twin to improve drug delivery using models of simulated lungs as part of the Virtual Human System project. They found that only about 20% of many drugs reached their target. The digital twins allowed them to redesign the drug’s particle size and composition characteristics to improve delivery efficiency to 90%.
VentureBeat
VentureBeat’s mission is to be a digital town square for technical decision-makers to gain knowledge about transformative technology and transact.
Our site delivers essential information on data technologies and strategies to guide you as you lead your organizations. We invite you to become a member of our community, to access:
up-to-date information on the subjects of interest to you
our newsletters
gated thought-leader content and discounted access to our prized events, such as Transform 2021: Learn More
networking features, and more
Become a member
Source link
0 notes
Link
The report provides a detailed assessment of the “Global Electrical Digital Twin Market”. This includes enabling technologies, key trends, market drivers, challenges, competition, standardization, regulatory landscape, deployment models, market size, operator case studies, opportunities, future roadmaps, value chains, ecosystem player profiles, and strategies included. The report also presents a SWOT analysis and forecast for Electrical Digital Twin investments from 2020 to 2026.
0 notes
Text
The Benefits Of Robotic Palletizing
For a Free Demonstration using your actual product, fill out the form and a QComp representative click here will get back to you to schedule an appointment. After assessing and designing your end-of-line layout, Fuji staff will match you up with the right integrator to ensure that you have someone you can trust installing your system.
In these instances, determine whether the porosity of the corrugated board has changed. Another fact to be aware of is that high acceleration does not work well with vacuum tools. Dense array tools have their own compressed-air generator for greater air flow. Find out what the world’s most innovative companies are doing to improve productivity in their plants and distribution centers.
If you evaluated all of the speculative articles on artificial intelligence in the last decade, you could conclude that that we are on the verge of building a robot that is self-aware and can think just like a human. But there is a new threat that is striking fear into the heart of working people.
Gains associated to purchasing robotic palletizer system are often related to lower labor costs but also linked to increased productivity. The KR 1000 titan stacks the heaviest loads with utmost speed and maximum reach. When it comes to heavy loads, the KR 700 PA comes into its own – and stacks higher than any other robot. The KR 40 PA stacks europallets faster than any other robot – up to a height of 1.8 meters.
HMI/controller – The HMI (human/machine interface) allows for product to be changed over easily and provides system information and alerts. Non specialized integrated robotic palletizer components utilized standard industry manufacturers, such as Hytrol and ABB . A means to identify bags using a non-contact UV ink application, detection and verification system, thereby uniquely identifying bags according to originating filling station.
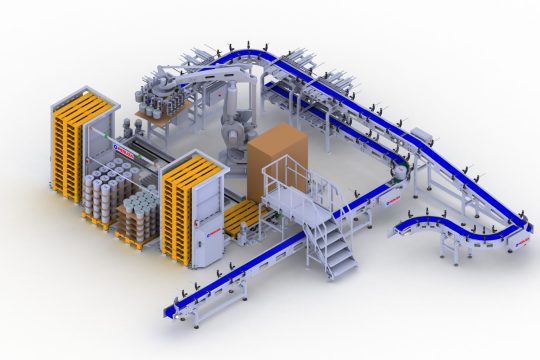
A Leader In The Robot Palletizing And Packaging Industries
Frequent cleaning of corrugated dust off the vacuum cups is part of routine maintenance. Occasionally, even with clean vacuum heads and a vacuum that is operating perfectly, cases simply fail to lift or detach from the tool.
Multi-Conveyor recently built three mild-steel constructed conveyors that passive off transfer from narrow to wide side leading to facilitate multiple side case printing. Sign up for our newsletter to receive the latest in packaging tips, case studies and more. Working with an experienced integrator will help you make informed choices and specify the equipment best suited for your application. Look for an integrator that’s certified by the robotic palletizer association . This independent, nonprofit organization awards the title of RIA Certified Robot Integrator only to the most accomplished organizations who demonstrate a high level of skill and experience.
And while there is certainly nothing wrong with manual labor, there are few people who would choose meeting a quota of stacked boxes during their workday over more meaningful tasks. Our team consults with our customers from the initial design specifications through final design, fabrication, programming and final testing.
Over millions of years, the human brain grew in size and complexity until it developed conscious thought and self-awareness. The author assumes that to really achieve artificial intelligence that has self- awareness will require designing a computer that has most of the features and capabilities of the human brain.
— Some tools can cause indentations in shrink-wrap or leave marks on your packaging, which may be unacceptable for items intended for display. — The tooling you choose can significantly affect your throughput capabilities. Start by determining an acceptable range of cases per minute, which will help you make informed decisions when evaluating tooling options.
Integrating an existing industrial communication standard with an up-and-coming method often creates headaches. But several industry experts see an easy path to integrating IO-Link and Single Pair Ethernet. Companies want to rely less on skilled labor and more on AI and robotic palletizer intelligence, but currently only 1% of all manufacturers are utilizing this technology in their operations. The Industrial Internet Consortium releases a white paper that provides direction on the use of blockchain applications for Industrial Internet of Things applications. Predictive analytics, digital twinning, and augmented and virtual reality technologies are all finding uses in manufacturing operations – from line installation and maintenance to process training.
Applications That Favor Robotics
Customer consultation and feedback are critical to creating a high-performance solution that our customers can count on. With this wide range of payload capacity, it’s your choice to pick single-case or an entire layer for stacking and breaking down pallet loads.
The human brain is a living, wet analogue of networks that can perform massively parallel processes at the same time and operates in agreement with biological laws. There is no programming, and the brain has the ability to change from one moment to the next, constantly forming new synapses. The human brain also includes areas we call the subconscious and conscious mind, which are absolutely essential in reaching consciousness or sentience.
Sign Up Stay current with the latest industry trends and news about our innovations. Robotic Palletizing – A cost effective robotic palletizer solution that improves profitability. Hamer-Fischbein has the equipment to meet all your needs with the innovation, durability, and quality you have come to expect from Hamer-Fischbein.
This article shows that for the brain to evolve to self-aware status requires developing an unconscious mind, using emotions, having modulated neurons and content addressable memory, and combining processing with memory. The only model that we know that has evolved to self-awareness and consciousness is the human brain.
It is the possibility that artificial intelligence will progress to the point that machines will become sentient and replace people in all working environments. This idea has been popularized in movies like the Terminator, when scientists created a computer chip that made machines conscious and self-aware. Tesla founder Elon Musk and physicist Stephen Hawking both warned that machines will eventually start programming themselves, and trigger the collapse of civilization. Red Devil hoped to reduce injuries and labor costs, while improving the efficiency of its lines. The APT MOD-PAL-PAIL can handle up to 10 ppm depending upon the product weight and size.
Fuji has been optimizing end-of-line operations since the 1940's and our engineers know how to choose the right equipment and put them in the best layout for your facility. When you work with Fuji, you receive all the benefits of the Fuji Advantage. Our team will work with you from design to install, and support your operation for the life of the system. Material Transfer & Storage completed an expansion that doubles its assembly space and enables additional processes to be brought in-house.
Servo motion control is the main driver in gantry systems and MESH is an expert in Rockwell and Siemens servo systems. Additionally, we have developed HMI screens that are intuitive and flexible. Delkor Palletizer offers seamless integration, simplified operation, and ease of installation to any Delkor end-of-line packaging system. This approach could ensure greater safety for both two- and four-legged soldiers. Don’t be too quick to use vacuum tooling; clamping may offer better performance and flexibility.
There is no one place which stores this information; it is stored all over the brain from the pre-frontal cortex, to the thalamus, and many other different parts of the brain. The unconscious mind does not reason or think; it simply stores all of the information needed by the conscious mind for the thinking process. But to simulate the typical 3.5 pound human brain would require matching the brain’s billions of interactions between cell types, neurotransmitters, neuromodulators, axonal branches and dendritic spines. Because the brain is nonlinear, and because it has so much more capacity than any computer, it functions completely different from a digital computer.
Consciousness is a holistic phenomenon occurring simultaneously in the entire brain. This may be feelings, experiences, memories, or facts that the brain views as related to the problem. Just how the brain can access the right neurons to gather the relevant information for the conscious mind to think is still unknown. It is not like a computer hard drive because it records everything we have smelled, touched, tasted, or heard including perceptions, memories, feelings, reflections, thoughts, hope since birth.
Increase Efficiency With Pearson Robotic Palletizers
Our engineered gripper also handles both slip sheets and pallet placement. Form, fit, and function are met with the design and selection of materials for each application. As organizations continue to see the value in industrial analytics, making sense of the sheer amount of data produced can be a difficult task. Finding the right product and developing a proper workflow is important to get long-term use out of the system.
Unlike the digital computer, in the brain, processing and memory are performed by the same components. To solve a problem or find and answer, the digital computer processes information from memory using CPUs, and then writes the results of that processing back to memory. All conscious thinking processes begin in the subconscious mind and are outside human awareness.
Ocura Robotic Palletizer Solutions - Courtesy of ESS Technologies - When floor space and interaction with employees is high on your requirement list, FANUC's CR Collaborative Robot Series is the best choice. With payloads ranging from 15 to 35kg and the ability to stop immediately upon contact, a collaborative solution eliminates the need for expensive and space-consuming safety guarding. Fork EOATs require the most space to operate, which may or may not be a problem, depending on the plant space available. Due to their weight and size, these tools may require a heftier robot than either vacuum or clamp EOATs, adding to cost.
0 notes
Text
Applying AI to Process Manufacturing
What do Rolls Royce, Siemens, and General Motors have in common? All of them use Artificial Intelligence to increase production efficiency and reduce costs. According to recent studies, the AI market will reach a fascinating $190.61 billion by 2025, which only proves that more companies will adopt this technology and use data for their benefit.
Here are some of the ways you can apply AI in manufacturing to achieve greater production speed and better user experience:
Predictive Maintenance
One of the biggest concerns for any manufacturer is downtime, faulty machinery or unexpected issues. Reactive maintenance or solving the failure after it occurs costs a lot of money.
That’s why predictive maintenance is a smart and productive implementation of AI in manufacturing.
By using machine learning, the AI’s algorithm can leverage and analyze any current and previous data about the machine, and provide useful insights about unplanned failures.
This way, Artificial Intelligence anticipates the possible downtime and creates an opportunity for an engineer to come up with a better and smarter decision in the manufacturing process.
If you want to read more on this topic check out our blog post – Big Data Analytics for Manufacturing – Getting Started with Predictive Maintenance.
Quality Control
With the rise of technology, manufacturers feel the pressure to provide a product or machine with a skyrocketing quality. Applying AI in manufacturing is one way to maintain steady quality, especially in the production phase.
An effective way to do this is by using computer vision. Even though the production process can be managed by a team of qualified and experienced engineers, some product flaws are too small to be noticed by the human eye. If you set up cameras to your AI machine, the machine can catch even the smallest flaws or defects that may occur, take a picture, and send it to an expert to evaluate it.
Nowadays, this entire process can be automated without an expert’s evaluation, and the system can notice the flaw, and alert the engineer to fix it immediately.
Want more?
Subscribe to receive articles on topics of your interest, straight to your inbox.
Success!
First Name
Last Name
Email
Subscribe
Digital Twins
Another productive use of AI in manufacturing is by building an accurate virtual representation of a product or a machine. This process includes using a combination of data, different AI tools, and CAD to develop a virtual representation of a real-world data.
In simpler words, you are creating a digital twin of the physical characteristics of a real object.
All the data AI collects is connected to a cloud-based service which is afterward analyzed and processed. Developing digital twins is extremely important to design engineers.
AI enables them to monitor and analyze the performance of the object, the change in quality with the smallest design changes, and all this before the product is developed.
Not only that a lot of time and resources are saved by applying AI in manufacturing, but also the overall positive user experience increases since the number of critical mistakes are cut down entirely.
With Syntelli and our solution in AI and machine learning, your company can experience all these benefits too. Contact us to learn more.
SIMILAR POSTS
Python & R vs. SPSS & SAS
Although computer programming has improved exponentially in the past several decades, not all programming languages are capable of processing the data used for machine learning. Big data has grown significantly, and the resulting data sets are both larger and more...
read more
Cloud Migration – Is it too late to adopt?
“Action is the foundational key to all success.” – Pablo Picasso No technology gets adopted overnight. On-premise environment provides full ownership, maintenance, responsibility. You pay for physical machines and virtualizations to meet your demands. Lately, such...
read more
The Power of Big Data and AI in the Fight Against COVID-19
The emergence of a novel coronavirus in December 2019 has had the medical community on edge. Public health officials around the world have faced an uphill battle in treating current cases and preventing the further spread of the virus. The 2002 SARS outbreak, and...
read more
The post Applying AI to Process Manufacturing appeared first on Syntelli Solutions Inc..
https://www.syntelli.com/applying-ai-to-process-manufacturing
0 notes
Text
Digitalisation: helping business thrive and survive
Success stories of businesses using technology to advance were at the forefront of Siemens’ Digitalize 2019 conference. The event showcased discussions on cybersecurity, smart infrastructure, scalability in manufacturing, Australia’s energy transition, future of work in the industry and opportunities presented by Industry 4.0. At the core of these discussions was digitalisation; particularly its role within organisations of all sizes — from large entities such as Powerlink Queensland and Deloitte; start-ups such as Brogan’s Way distillery; and SMEs such as Sage Automation and HeliMods.
Energy transition
The digitalised world is demanding change in the energy sector, perhaps more so than any other. The centralisation of the industry has brought new demands that have seen previous innovations rendered outdated much faster than anticipated.
Powerlink Queensland Executive General Manager Kevin Kehl discussed the challenges his company was facing and boiled it down to the ‘three Ds’: the decarbonisation of power generation; the decentralisation of customers and storage; and digitalisation itself, which, if capitalised on, could facilitate and enhance the other two.
Kehl cited the importance of predicting customer behaviour as critical to shaping the energy transition. He explained that Powerlink Queensland conducts a household energy survey every two years, with the local distribution companies in Queensland, to discover what customers are thinking in order to help predict behaviours and expectations.
“I think, irrespective of any policy objectives, consumers will largely lead the change as they have,” Kehl said. “Indeed, that’s going to be the biggest driver of the change for us.”
Customers are investing more capital in rooftop solar and increased storage, making transmission infrastructure the glue that holds together the system. Australia is predicted to be the most distributed grid worldwide in the next decade and Kehl emphasised that this will require a much more dynamic and information-intensive approach.
Smart infrastructure
Urbanisation is occurring across the world, with approximately 55% of the global population currently living in urban areas. Predictions suggest that by 2050, this will rise to 68%. Australia is no exception. Melbourne, for example, is predicted to have 7.3 million people by 2050, up from the current 4.6 million who live there now.
It’s clear that new approaches need to be adopted, such as the building of smart infrastructure, which is key to sustainability. According to Siemens Global Head of Urban Development Martin Powell, combining infrastructure with data analytics will provide countless opportunities to counter these problems.
“Using weather data analytics to gather readings on air quality could allow cities to create low-emission zones,” Powell said. “We could introduce ‘no school on bad air days’ or penalise people who drive in the affected areas.”
Other innovations are based on the idea of the digital twin, allowing for ideas and concepts to be worked through digitally to find any problems before any money is spent. This would also allow for different cities facing similar problems to share data and make adjustments according to their own unique challenges.
“We are applying machine learning so that we can reduce the amounts of maintenance that we need across our cities,” Powell added.
Scalable manufacturing in Industry 4.0
Industry 4.0 and automation is being increasingly adopted by small and medium manufacturers, debunking the myth that it’s only of value and affordable to big companies.
Hon. Cameron Dick, Queensland State Minister for State Development, Manufacturing, Infrastructure and Planning, commended the state’s manufacturers for being “well advanced on their Industry 4.0 journey”, calling it a showcase of the diversity in the sector.
Case study: Kaiju Brewery
Kaiju Brewery co-owner Callum Reeves explained that his brewery exemplifies the scalability of modern automation technology.
Reeves’ business was growing at a rate of 100% per annum but faced the issue of losing consistency with expansion. Technology came to his rescue.
“One of the biggest things is maintaining the quality that people expect of your brand as you scale,” Reeves said.
Reeves’ passion for technology and futureproofing is what encouraged him to begin using Deacam’s Fermecraft technology combined with Siemens’ MindSphere software to monitor the brewing process and ensure each brew remained uniform.
The investment in upgrading automation and digital technology has prepared Kaiju for the next few years of manufacturing.
Case study: HeliMods
Queensland-based SME HeliMods utilised digital twin technology to track and change product designs to make a real, practical difference to its helicopter operators.
HeliMods CEO Will Shrapnel said, “You wouldn’t set up a workshop with physical assets and leave them lying about the place. It’s no different in the digital world. If you’re going to invest in digital technologies, you[’ve] got to understand how those assets are going to be used, re-used, stored and how you’re going to develop them over time. It’s critically important,” he said.
Cybersecurity
Unlike most industries in the current climate, there is less of a requirement to transition from an old model, but a need to grow the sector to meet the demands of the digital world.
AustCyber CEO Michelle Price said that, “Digitalisation is both an opportunity and a risk, but you must be on top of your game and vigilant.
“There are enormous opportunities available to us when we embrace cybersecurity and recognise that it is an investment in our future.”
Australia is claimed to be the number one per capita ransomware target in the world and is in the top 10 nations for email phishing attacks. Australia also has a $3 trillion superannuation system that, according to Price, “makes every single corner of every single populated space in Australia a high-value target”.
Price challenged businesses to take stock of their own security and ask themselves if they are “cyber prepared or cyber scared?”
Futureproofing the workforce
It’s important to recognise that the future of work will require an adjustment to the way in which employees are continuously trained and upskilled, as well as changes in the higher education sector to ensure that students are being prepared sufficiently for the workforce.
Professor Bronwyn Fox, Director of the Manufacturing Futures Research Institute at Swinburne University of Technology, was involved in a panel on the subject and discussed the work Swinburne is doing in partnership with Siemens and Ai Group to facilitate this education process.
“We’ve learnt a lot from our partners in Germany and we’ve learnt a lot from the Austrian Industry 4.0 network, which has allowed us to really translate that to the Australian environment,” Fox said.
“We can create six cyber-physical labs that are an immersive environment for industry leaders and newcomers to come and understand the concepts of digitalisation.”
Collaboration from industry leaders, tertiary education institutions, unions and government agencies is crucial for the future of employment.
Ultimately, the consistent message across all speakers and discussions at the conference was that everybody needs to be able to adapt and cooperate so that the Australian market can thrive.
Image courtesy of Siemens Australia.
source http://sustainabilitymatters.net.au/content/sustainability/article/digitalisation-helping-business-thrive-and-survive-732543160
from WordPress https://davidkent.home.blog/2019/08/29/digitalisation-helping-business-thrive-and-survive/
0 notes
Text
Investing Big in RPA is Not a Fool’s Game | Sherpas in Blue Shirts
The news of another big round of funding for UiPath, US$225 million series C, and a valuation of US$3 billion created a lot of excitement and amazement in the market. It followed on from Automation Anywhere’s whopping series A funding round of US$250 million in July, which valued the company at US$1.8 billion, and which surpassed UiPath’s earlier series B funding of US$153 million and a valuation of US$1 billion in Q1 2018.
These valuations are phenomenal. In UiPath’s case, the rise from US$1 billion to US$3 billion in less than six months is, I believe, unprecedented. You might think that investors are living on a different planet than us ordinary folks, and that this kind of valuation is plain wrong. I beg to differ.
Investing in the Future of RPA
My case rests on the rapid increase in market adoption and the huge investments that vendors are making in their platforms. As much has already been said about the fast rate of enterprise adoption, there’s no need for me to repeat it again here. Jumping to the second part of my case: RPA today is not the RPA that launched this market three to four years ago. The original developments lacked many of the features that we see today, e.g., computer vision to pick objects on the screen and robust control panels. Similarly, tomorrow’s RPA will be superior to today’s.
As someone who assesses RPA technology on an annual basis, I see a fast rate of product development, not just year on year, but in some cases quarter by quarter.
Everest Group’s “RPA Virtuous Circle” highlights the continuous cycle of developments in the market.
Much has been said of organizations struggling to scale their deployments. I completely agree with this, and for a while I’ve been asking vendors to do something about this issue. I am delighted to see that they have been listening and are investing in features for scaling. These include enhanced robot run time control and management features including intelligent control systems for dynamic workload balancing, auto-scaling, and even identifying processes for further automation. Another major stream of development is turning RPA platforms into the glue that holds together business process management systems (BPMS), different varieties of machine learning, and narrow artificial intelligence. These will ultimately be integrated and will combine seamlessly to provide end-to-end process automation.
While vendors do their bit for scale, organizations should also examine their deployment models for RPA and take a more programmatic approach. Automation is going to be a serious competitive differentiator, and a programmatic approach would significantly speed up organizations’ adoption and realization of desired outcomes. Everest Group’s RPA Pinnacle study highlights some of the approaches that organizations have taken to achieve excellence in RPA.
Of course, these enormous investments in RPA do carry some risks. There is the possibility of tech giants bringing their own RPA solutions to market, in turn pushing out the current RPA vendors. But that wouldn’t be easy to do, as the existing vendors have gained a lot of hard to emulate know how in the past few years. And any one of the existing RPA vendors could be acquired in a major acquisition, but then the investors would get the handsome returns they anticipated…just in a different way.
Taking the Manufacturing Model to Business Processes
Another reason for my optimism about the recent investments in RPA and vendor valuations is that I recently got a glimpse into the future of business automation by looking at manufacturing. On a visit to Siemens Digital, I saw how the concept of digital twin and simulation of manufacturing processes is helping speed up production times and efficiency, even in manual/human processes.
For years, corporate global services functions have attempted to copy manufacturing principles, e.g., adopting Lean and Six Sigma methodologies. Today, they have moved on to automation, which manufacturing adopted decades ago. Having started on automation of global services, enterprises are not going to turn back. They will continue to follow manufacturing’s lead.
Leading organizations are already giving their processes version numbers with supporting documentation, having taken each step through a rigorous Lean Six Sigma methodology. On the automation front, while the focus has been primarily on tactical needs, it will increasingly move to outcomes and the finished “product,” as in manufacturing.
We will see enterprises develop digital twins of their processes or robots, and run complex functions end-to-end in virtual reality before committing to the final model for deployment in the real world. Future versions of RPA will have to support these requirements, and that is where some of the millions of funding will be spent; on product development and advanced features.
Today’s RPA products are paving the way for a far bigger change in automation of global services than we have seen to date. They are the building blocks of the platforms of the future for an inevitable automation journey that every organization will have to take sooner or later. That is why the current group of vendors are so attractive to investors. They are betting not just on today’s growing revenues, but what is to come.
The post Investing Big in RPA is Not a Fool’s Game | Sherpas in Blue Shirts appeared first on Everest Group.
from pesonivt2a https://www.everestgrp.com/2018-09-investing-big-rpa-not-fools-game-sherpas-blue-shirts-46859.html/ via http://www.rssmix.com/
0 notes
Text
Medtech firms get personal with digital twins
New Post has been published on http://newsintoday.info/2018/09/02/medtech-firms-get-personal-with-digital-twins-2/
Medtech firms get personal with digital twins
HEIDELBERG, Germany (Reuters) – Armed with a mouse and computer screen instead of a scalpel and operating theater, cardiologist Benjamin Meder carefully places the electrodes of a pacemaker in a beating, digital heart.
Using this “digital twin” that mimics the electrical and physical properties of the cells in patient 7497’s heart, Meder runs simulations to see if the pacemaker can keep the congestive heart failure sufferer alive – before he has inserted a knife.
The digital heart twin developed by Siemens Healthineers is one example of how medical device makers are using artificial intelligence (AI) to help doctors make more precise diagnoses as medicine enters an increasingly personalized age.
The challenge for Siemens Healthineers and rivals such as Philips and GE Healthcare is to keep an edge over tech giants from Alphabet’s Google to Alibaba that hope to use big data to grab a slice of healthcare spending.
With healthcare budgets under increasing pressure, AI tools such as the digital heart twin could save tens of thousands of dollars by predicting outcomes and avoiding unnecessary surgery.
A shortage of doctors in countries such as China is also spurring demand for new AI tools to analyze medical images and the race is on to commercialize products that could shake up healthcare systems around the world.
While AI has been used in medical technology for decades, the availability of vast amounts data, lower computing costs and more sophisticated algorithms mean revenues from AI tools are expected to soar to $6.7 billion by 2021 from $811 million in 2015, according to a study by research firm Frost & Sullivan ww2.frost.com.
The size of the global medical imaging analytics software market is also expected to jump to $4.3 billion by 2025 from $2.4 billion in 2016, said data portal Statista www.statista.com.
“What started as an evolution is accelerating towards more of a revolution,” said Thomas Rudolph who leads McKinsey & Company’s www.mckinsey.com pharma and medical technology practice in Germany.
‘GPS OF HEALTHCARE’
For Siemens Healthineers and its traditional rivals, making the transition from being mainly hardware companies to medical software pioneers is seen as crucial in a field becoming increasingly crowded with new entrants.
Google has developed a raft of AI tools, including algorithms that can analyze medical images to diagnose eye disease, or sift through digital records to predict the likelihood of death.
Alibaba, meanwhile, hopes to use its cloud and data systems to tackle a shortage of medical specialists in China. It is working on AI-assisted diagnosis tools to help analyze images such as CT scans and MRIs.Siemens Healthineers, which was spun off from German parent Siemens in March, has outpaced the market in recent quarters with sales of medical imaging equipment thanks to a slew of new products.
But analysts say the German firm, Dutch company Philips and GE Healthcare, a subsidiary of General Electric, will all come under pressure to prove they can save healthcare systems money as spending becomes more linked to patient outcomes and as hospitals rely on bulk purchasing to push for discounts.
Siemens Healthineers has a long history in the industry. It made the first industrially manufactured X-ray machines in 1896 and is now the world’s biggest maker of medical imaging equipment.
Now, Chief Executive Bernd Montag’s ambition is to transform it into the “GPS of healthcare” – a company that harnesses its data to sell intelligent services, as well as letting smaller tech firms develop Apps feeding off its database.
As it adapts, Siemens Healthineers has invested heavily in IT. It employs some 2,900 software engineers and has over 600 patents and patent applications in machine learning.
It is not alone. Philips says about 60 percent of its research and development (R&D) staff and spending is focused on software and data science. The company said it employs thousands of software engineers, without being specific.
MEDICAL REVOLUTION
Experts say the success of AI in medical technology will hinge on access to reliable data, not only to create models for diagnosis but also to predict how effective treatments will be for a specific patient in the days and years to come.
“Imagine that in the future, we have a patient with all their organ functions, all their cellular functions, and we are able to simulate this complexity,” said Meder, a cardiologist at Heidelberg University Hospital here in Germany who is testing Siemens Healthineers’ digital heart software.
A three-dimensional printout of a human heart is seen at the Heidelberg University Hospital (Universitaetsklinikum Heidelberg) in Heidelberg, Germany, August 14, 2018. REUTERS/Ralph Orlowski
“We would be able to predict weeks or months in advance which patients will get ill, how a particular patient will react to a certain therapy, which patients will benefit the most. That could revolutionize medicine.”
To this end, Siemens Healthineers has built up a vast database of more than 250 million annotated images, reports and operational data on which to train its new algorithms.
In the example of the digital twin, the AI system was trained to weave together data about the electrical and physical properties and the structure of a heart into a 3D image.
One of the main challenges was hiding the complexity and creating an interface that is easy to use, said Tommaso Mansi, a senior R&D director at Siemens Healthineers who developed the software.
To test the technology, Meder’s team created 100 digital heart twins of patients being treated for heart failure in a six-year trial. The computer makes predictions based on the digital twin and they are then compared with actual outcomes.
His team hopes to finish evaluating the predictions by the end of 2018. If the results are promising, the system will be tested in a larger, multi-center trial as the next step to getting the software approved by regulators for commercial use.
Siemens Healthineers declined to say when the technology might eventually be used by clinics or give details on how its digital heart, or models of other organs it is developing such as the lungs and liver, could be monetized.
IN DATA WE TRUST
Both GE and Philips are also working on versions of digital heart twins while non-traditional players have been active too.
Drawing on its experience of making digital twins to test bridges and machinery, French software firm Dassault Systemes launched the first commercial “Living Heart” model in May 2015, though it is only currently available for research.
Philips sells AI-enabled heart models that can, for example, turn 2D ultrasound images into data that helps doctors diagnose problems, or automatically analyze scans to help surgeons plan operations.
Its vision, like Siemens Healthineers, is to add more complexity to its existing heart models by pulling together scans, ECGs and medical records to create a model that can predict how a heart will respond to therapy in real life.
For now, such software is still in the early stages of development and companies will have to work with regulators to thrash out how predictive models can be approved before doctors are willing to trust a diagnosis generated by a machine.
Access to high-quality data with enough variation will be crucial, as will be the ability to interpret that data and turn it into something medical professionals can use, say experts.
In particular, models will have to be trained on rare cases as they get closer to perfection, said Vivek Bhatt, chief technology officer at GE Healthcare’s clinical care solutions division.
“It’s going to be extremely critical to have an ongoing process for getting more data, getting the right kind of data and getting data with those unique cases,” he said.
The established medtech players say their long-running relationships with hospitals and research institutes and vast networks of installed machines will give them an edge over new tech entrants.
Siemens Healthineers, GE Healthcare and Philips say their databases are fed with a mixture of publicly-available data, data from clinical trials or from collaborations with hospitals – as well as some data from customers. All the data is made anonymous and only used with patients’ consent, they say.
Still, some campaigners and academics worry about patients’ data being used primarily by companies as a commercial tool.
Boris Bogdan, managing director at Accenture’s www.accenture.com life science practice in Switzerland, believes the ownership of data is a gray zone that could lead to a patient backlash if companies start making fortunes from it.
“When Facebook started nobody really cared who owned the information,” he said.
“Now that people understand that Facebook earns tremendous money with their data, questions like data privacy, data usage and data monetization are becoming more visible.”
Slideshow (16 Images)
(This version of the story has been corrected to fix typo in headline.)
Reporting by Caroline Copley; editing by David Clarke
Our Standards:The Thomson Reuters Trust Principles.
Source link
0 notes
Text
Medtech firms get personal with digital twins
New Post has been published on http://resellerwebhostingproviders.com/medtech-firms-get-personal-with-digital-twins/
Medtech firms get personal with digital twins
HEIDELBERG, Germany (Reuters) – Armed with a mouse and computer screen instead of a scalpel and operating theater, cardiologist Benjamin Meder carefully places the electrodes of a pacemaker in a beating, digital heart.
A three-dimensional printout of a human heart is seen at the Heidelberg University Hospital (Universitaetsklinikum Heidelberg) in Heidelberg, Germany, August 14, 2018. REUTERS/Ralph Orlowski
Using this “digital twin” that mimics the electrical and physical properties of the cells in patient 7497’s heart, Meder runs simulations to see if the pacemaker can keep the congestive heart failure sufferer alive – before he has inserted a knife.
The digital heart twin developed by Siemens Healthineers is one example of how medical device makers are using artificial intelligence (AI) to help doctors make more precise diagnoses as medicine enters an increasingly personalized age.
The challenge for Siemens Healthineers and rivals such as Philips and GE Healthcare is to keep an edge over tech giants from Alphabet’s Google to Alibaba that hope to use big data to grab a slice of healthcare spending.
With healthcare budgets under increasing pressure, AI tools such as the digital heart twin could save tens of thousands of dollars by predicting outcomes and avoiding unnecessary surgery.
A shortage of doctors in countries such as China is also spurring demand for new AI tools to analyze medical images and the race is on to commercialize products that could shake up healthcare systems around the world.
While AI has been used in medical technology for decades, the availability of vast amounts data, lower computing costs and more sophisticated algorithms mean revenues from AI tools are expected to soar to $6.7 billion by 2021 from $811 million in 2015, according to a study by research firm Frost & Sullivan ww2.frost.com.
The size of the global medical imaging analytics software market is also expected to jump to $4.3 billion by 2025 from $2.4 billion in 2016, said data portal Statista www.statista.com.
“What started as an evolution is accelerating towards more of a revolution,” said Thomas Rudolph who leads McKinsey & Company’s www.mckinsey.com pharma and medical technology practice in Germany.
‘GPS OF HEALTHCARE’
For Siemens Healthineers and its traditional rivals, making the transition from being mainly hardware companies to medical software pioneers is seen as crucial in a field becoming increasingly crowded with new entrants.
Google has developed a raft of AI tools, including algorithms that can analyze medical images to diagnose eye disease, or sift through digital records to predict the likelihood of death.
Alibaba, meanwhile, hopes to use its cloud and data systems to tackle a shortage of medical specialists in China. It is working on AI-assisted diagnosis tools to help analyze images such as CT scans and MRIs.Siemens Healthineers, which was spun off from German parent Siemens in March, has outpaced the market in recent quarters with sales of medical imaging equipment thanks to a slew of new products.
But analysts say the German firm, Dutch company Philips and GE Healthcare, a subsidiary of General Electric, will all come under pressure to prove they can save healthcare systems money as spending becomes more linked to patient outcomes and as hospitals rely on bulk purchasing to push for discounts.
A general view of the Klaus-Tschira-Institute for Integrative Computational Cardiology, department of the Heidelberg University Hospital (Universitaetsklinikum Heidelberg), in Heidelberg, Germany, August 14, 2018. REUTERS/Ralph Orlowski
Siemens Healthineers has a long history in the industry. It made the first industrially manufactured X-ray machines in 1896 and is now the world’s biggest maker of medical imaging equipment.
Now, Chief Executive Bernd Montag’s ambition is to transform it into the “GPS of healthcare” – a company that harnesses its data to sell intelligent services, as well as letting smaller tech firms develop Apps feeding off its database.
As it adapts, Siemens Healthineers has invested heavily in IT. It employs some 2,900 software engineers and has over 600 patents and patent applications in machine learning.
It is not alone. Philips says about 60 percent of its research and development (R&D) staff and spending is focused on software and data science. The company said it employs thousands of software engineers, without being specific.
MEDICAL REVOLUTION
Experts say the success of AI in medical technology will hinge on access to reliable data, not only to create models for diagnosis but also to predict how effective treatments will be for a specific patient in the days and years to come.
“Imagine that in the future, we have a patient with all their organ functions, all their cellular functions, and we are able to simulate this complexity,” said Meder, a cardiologist at Heidelberg University Hospital here in Germany who is testing Siemens Healthineers’ digital heart software.
“We would be able to predict weeks or months in advance which patients will get ill, how a particular patient will react to a certain therapy, which patients will benefit the most. That could revolutionize medicine.”
To this end, Siemens Healthineers has built up a vast database of more than 250 million annotated images, reports and operational data on which to train its new algorithms.
In the example of the digital twin, the AI system was trained to weave together data about the electrical and physical properties and the structure of a heart into a 3D image.
One of the main challenges was hiding the complexity and creating an interface that is easy to use, said Tommaso Mansi, a senior R&D director at Siemens Healthineers who developed the software.
To test the technology, Meder’s team created 100 digital heart twins of patients being treated for heart failure in a six-year trial. The computer makes predictions based on the digital twin and they are then compared with actual outcomes.
His team hopes to finish evaluating the predictions by the end of 2018. If the results are promising, the system will be tested in a larger, multi-center trial as the next step to getting the software approved by regulators for commercial use.
Siemens Healthineers declined to say when the technology might eventually be used by clinics or give details on how its digital heart, or models of other organs it is developing such as the lungs and liver, could be monetized.
Slideshow (15 Images)
IN DATA WE TRUST
Both GE and Philips are also working on versions of digital heart twins while non-traditional players have been active too.
Drawing on its experience of making digital twins to test bridges and machinery, French software firm Dassault Systemes launched the first commercial “Living Heart” model in May 2015, though it is only currently available for research.
Philips sells AI-enabled heart models that can, for example, turn 2D ultrasound images into data that helps doctors diagnose problems, or automatically analyze scans to help surgeons plan operations.
Its vision, like Siemens Healthineers, is to add more complexity to its existing heart models by pulling together scans, ECGs and medical records to create a model that can predict how a heart will respond to therapy in real life.
For now, such software is still in the early stages of development and companies will have to work with regulators to thrash out how predictive models can be approved before doctors are willing to trust a diagnosis generated by a machine.
Access to high-quality data with enough variation will be crucial, as will be the ability to interpret that data and turn it into something medical professionals can use, say experts.
In particular, models will have to be trained on rare cases as they get closer to perfection, said Vivek Bhatt, chief technology officer at GE Healthcare’s clinical care solutions division.
“It’s going to be extremely critical to have an ongoing process for getting more data, getting the right kind of data and getting data with those unique cases,” he said.
The established medtech players say their long-running relationships with hospitals and research institutes and vast networks of installed machines will give them an edge over new tech entrants.
Siemens Healthineers, GE Healthcare and Philips say their databases are fed with a mixture of publicly-available data, data from clinical trials or from collaborations with hospitals – as well as some data from customers. All the data is made anonymous and only used with patients’ consent, they say.
Still, some campaigners and academics worry about patients’ data being used primarily by companies as a commercial tool.
Boris Bogdan, managing director at Accenture’s www.accenture.com life science practice in Switzerland, believes the ownership of data is a gray zone that could lead to a patient backlash if companies start making fortunes from it.
“When Facebook started nobody really cared who owned the information,” he said.
“Now that people understand that Facebook earns tremendous money with their data, questions like data privacy, data usage and data monetization are becoming more visible.”
(This version of the story has been corrected to fix typo in headline.)
Reporting by Caroline Copley; editing by David Clarke
Our Standards:The Thomson Reuters Trust Principles.
0 notes
Text
Medtech firms get personal with digital twins
New Post has been published on http://resellerwebhostingproviders.com/medtech-firms-get-personal-with-digital-twins/
Medtech firms get personal with digital twins
HEIDELBERG, Germany (Reuters) – Armed with a mouse and computer screen instead of a scalpel and operating theater, cardiologist Benjamin Meder carefully places the electrodes of a pacemaker in a beating, digital heart.
A three-dimensional printout of a human heart is seen at the Heidelberg University Hospital (Universitaetsklinikum Heidelberg) in Heidelberg, Germany, August 14, 2018. REUTERS/Ralph Orlowski
Using this “digital twin” that mimics the electrical and physical properties of the cells in patient 7497’s heart, Meder runs simulations to see if the pacemaker can keep the congestive heart failure sufferer alive – before he has inserted a knife.
The digital heart twin developed by Siemens Healthineers is one example of how medical device makers are using artificial intelligence (AI) to help doctors make more precise diagnoses as medicine enters an increasingly personalized age.
The challenge for Siemens Healthineers and rivals such as Philips and GE Healthcare is to keep an edge over tech giants from Alphabet’s Google to Alibaba that hope to use big data to grab a slice of healthcare spending.
With healthcare budgets under increasing pressure, AI tools such as the digital heart twin could save tens of thousands of dollars by predicting outcomes and avoiding unnecessary surgery.
A shortage of doctors in countries such as China is also spurring demand for new AI tools to analyze medical images and the race is on to commercialize products that could shake up healthcare systems around the world.
While AI has been used in medical technology for decades, the availability of vast amounts data, lower computing costs and more sophisticated algorithms mean revenues from AI tools are expected to soar to $6.7 billion by 2021 from $811 million in 2015, according to a study by research firm Frost & Sullivan ww2.frost.com.
The size of the global medical imaging analytics software market is also expected to jump to $4.3 billion by 2025 from $2.4 billion in 2016, said data portal Statista www.statista.com.
“What started as an evolution is accelerating towards more of a revolution,” said Thomas Rudolph who leads McKinsey & Company’s www.mckinsey.com pharma and medical technology practice in Germany.
‘GPS OF HEALTHCARE’
For Siemens Healthineers and its traditional rivals, making the transition from being mainly hardware companies to medical software pioneers is seen as crucial in a field becoming increasingly crowded with new entrants.
Google has developed a raft of AI tools, including algorithms that can analyze medical images to diagnose eye disease, or sift through digital records to predict the likelihood of death.
Alibaba, meanwhile, hopes to use its cloud and data systems to tackle a shortage of medical specialists in China. It is working on AI-assisted diagnosis tools to help analyze images such as CT scans and MRIs.Siemens Healthineers, which was spun off from German parent Siemens in March, has outpaced the market in recent quarters with sales of medical imaging equipment thanks to a slew of new products.
But analysts say the German firm, Dutch company Philips and GE Healthcare, a subsidiary of General Electric, will all come under pressure to prove they can save healthcare systems money as spending becomes more linked to patient outcomes and as hospitals rely on bulk purchasing to push for discounts.
A general view of the Klaus-Tschira-Institute for Integrative Computational Cardiology, department of the Heidelberg University Hospital (Universitaetsklinikum Heidelberg), in Heidelberg, Germany, August 14, 2018. REUTERS/Ralph Orlowski
Siemens Healthineers has a long history in the industry. It made the first industrially manufactured X-ray machines in 1896 and is now the world’s biggest maker of medical imaging equipment.
Now, Chief Executive Bernd Montag’s ambition is to transform it into the “GPS of healthcare” – a company that harnesses its data to sell intelligent services, as well as letting smaller tech firms develop Apps feeding off its database.
As it adapts, Siemens Healthineers has invested heavily in IT. It employs some 2,900 software engineers and has over 600 patents and patent applications in machine learning.
It is not alone. Philips says about 60 percent of its research and development (R&D) staff and spending is focused on software and data science. The company said it employs thousands of software engineers, without being specific.
MEDICAL REVOLUTION
Experts say the success of AI in medical technology will hinge on access to reliable data, not only to create models for diagnosis but also to predict how effective treatments will be for a specific patient in the days and years to come.
“Imagine that in the future, we have a patient with all their organ functions, all their cellular functions, and we are able to simulate this complexity,” said Meder, a cardiologist at Heidelberg University Hospital here in Germany who is testing Siemens Healthineers’ digital heart software.
“We would be able to predict weeks or months in advance which patients will get ill, how a particular patient will react to a certain therapy, which patients will benefit the most. That could revolutionize medicine.”
To this end, Siemens Healthineers has built up a vast database of more than 250 million annotated images, reports and operational data on which to train its new algorithms.
In the example of the digital twin, the AI system was trained to weave together data about the electrical and physical properties and the structure of a heart into a 3D image.
One of the main challenges was hiding the complexity and creating an interface that is easy to use, said Tommaso Mansi, a senior R&D director at Siemens Healthineers who developed the software.
To test the technology, Meder’s team created 100 digital heart twins of patients being treated for heart failure in a six-year trial. The computer makes predictions based on the digital twin and they are then compared with actual outcomes.
His team hopes to finish evaluating the predictions by the end of 2018. If the results are promising, the system will be tested in a larger, multi-center trial as the next step to getting the software approved by regulators for commercial use.
Siemens Healthineers declined to say when the technology might eventually be used by clinics or give details on how its digital heart, or models of other organs it is developing such as the lungs and liver, could be monetized.
Slideshow (15 Images)
IN DATA WE TRUST
Both GE and Philips are also working on versions of digital heart twins while non-traditional players have been active too.
Drawing on its experience of making digital twins to test bridges and machinery, French software firm Dassault Systemes launched the first commercial “Living Heart” model in May 2015, though it is only currently available for research.
Philips sells AI-enabled heart models that can, for example, turn 2D ultrasound images into data that helps doctors diagnose problems, or automatically analyze scans to help surgeons plan operations.
Its vision, like Siemens Healthineers, is to add more complexity to its existing heart models by pulling together scans, ECGs and medical records to create a model that can predict how a heart will respond to therapy in real life.
For now, such software is still in the early stages of development and companies will have to work with regulators to thrash out how predictive models can be approved before doctors are willing to trust a diagnosis generated by a machine.
Access to high-quality data with enough variation will be crucial, as will be the ability to interpret that data and turn it into something medical professionals can use, say experts.
In particular, models will have to be trained on rare cases as they get closer to perfection, said Vivek Bhatt, chief technology officer at GE Healthcare’s clinical care solutions division.
“It’s going to be extremely critical to have an ongoing process for getting more data, getting the right kind of data and getting data with those unique cases,” he said.
The established medtech players say their long-running relationships with hospitals and research institutes and vast networks of installed machines will give them an edge over new tech entrants.
Siemens Healthineers, GE Healthcare and Philips say their databases are fed with a mixture of publicly-available data, data from clinical trials or from collaborations with hospitals – as well as some data from customers. All the data is made anonymous and only used with patients’ consent, they say.
Still, some campaigners and academics worry about patients’ data being used primarily by companies as a commercial tool.
Boris Bogdan, managing director at Accenture’s www.accenture.com life science practice in Switzerland, believes the ownership of data is a gray zone that could lead to a patient backlash if companies start making fortunes from it.
“When Facebook started nobody really cared who owned the information,” he said.
“Now that people understand that Facebook earns tremendous money with their data, questions like data privacy, data usage and data monetization are becoming more visible.”
(This version of the story has been corrected to fix typo in headline.)
Reporting by Caroline Copley; editing by David Clarke
Our Standards:The Thomson Reuters Trust Principles.
0 notes
Text
Medtech firms gets personal with digital twins
New Post has been published on https://rwamztech.com/medtech-firms-gets-personal-with-digital-twins/
Medtech firms gets personal with digital twins
HEIDELBERG, Germany (Reuters) – Armed with a mouse and computer screen instead of a scalpel and operating theater, cardiologist Benjamin Meder carefully places the electrodes of a pacemaker in a beating, digital heart.
A three-dimensional printout of a human heart is seen at the Heidelberg University Hospital (Universitaetsklinikum Heidelberg) in Heidelberg, Germany, August 14, 2018. REUTERS/Ralph Orlowski
Using this “digital twin” that mimics the electrical and physical properties of the cells in patient 7497’s heart, Meder runs simulations to see if the pacemaker can keep the congestive heart failure sufferer alive – before he has inserted a knife.
The digital heart twin developed by Siemens Healthineers (SHLG.DE) is one example of how medical device makers are using artificial intelligence (AI) to help doctors make more precise diagnoses as medicine enters an increasingly personalized age.
The challenge for Siemens Healthineers and rivals such as Philips PHL.AS and GE Healthcare is to keep an edge over tech giants from Alphabet’s (GOOGL.O) Google to Alibaba (BABA.N) that hope to use big data to grab a slice of healthcare spending.
With healthcare budgets under increasing pressure, AI tools such as the digital heart twin could save tens of thousands of dollars by predicting outcomes and avoiding unnecessary surgery.
A shortage of doctors in countries such as China is also spurring demand for new AI tools to analyze medical images and the race is on to commercialize products that could shake up healthcare systems around the world.
While AI has been used in medical technology for decades, the availability of vast amounts data, lower computing costs and more sophisticated algorithms mean revenues from AI tools are expected to soar to $6.7 billion by 2021 from $811 million in 2015, according to a study by research firm Frost & Sullivan ww2.frost.com.
The size of the global medical imaging analytics software market is also expected to jump to $4.3 billion by 2025 from $2.4 billion in 2016, said data portal Statista www.statista.com.
“What started as an evolution is accelerating toward more of a revolution,” said Thomas Rudolph who leads McKinsey & Company’s www.mckinsey.com pharma and medical technology practice in Germany.
‘GPS OF HEALTHCARE’
For Siemens Healthineers and its traditional rivals, making the transition from being mainly hardware companies to medical software pioneers is seen as crucial in a field becoming increasingly crowded with new entrants.
Google has developed a raft of AI tools, including algorithms that can analyze medical images to diagnose eye disease, or sift through digital records to predict the likelihood of death.
Alibaba, meanwhile, hopes to use its cloud and data systems to tackle a shortage of medical specialists in China. It is working on AI-assisted diagnosis tools to help analyze images such as CT scans and MRIs.Siemens Healthineers, which was spun off from German parent Siemens (SIEGn.DE) in March, has outpaced the market in recent quarters with sales of medical imaging equipment thanks to a slew of new products.
But analysts say the German firm, Dutch company Philips and GE Healthcare, a subsidiary of General Electric (GE.N), will all come under pressure to prove they can save healthcare systems money as spending becomes more linked to patient outcomes and as hospitals rely on bulk purchasing to push for discounts.
Slideshow (16 Images)
Siemens Healthineers has a long history in the industry. It made the first industrially manufactured X-ray machines in 1896 and is now the world’s biggest maker of medical imaging equipment.
Now, Chief Executive Bernd Montag’s ambition is to transform it into the “GPS of healthcare” – a company that harnesses its data to sell intelligent services, as well as letting smaller tech firms develop Apps feeding off its database.
As it adapts, Siemens Healthineers has invested heavily in IT. It employs some 2,900 software engineers and has over 600 patents and patent applications in machine learning.
It is not alone. Philips says about 60 percent of its research and development (R&D) staff and spending is focused on software and data science. The company said it employs thousands of software engineers, without being specific.
MEDICAL REVOLUTION
Experts say the success of AI in medical technology will hinge on access to reliable data, not only to create models for diagnosis but also to predict how effective treatments will be for a specific patient in the days and years to come.
“Imagine that in the future, we have a patient with all their organ functions, all their cellular functions, and we are able to simulate this complexity,” said Meder, a cardiologist at Heidelberg University Hospital here in Germany who is testing Siemens Healthineers’ digital heart software.
“We would be able to predict weeks or months in advance which patients will get ill, how a particular patient will react to a certain therapy, which patients will benefit the most. That could revolutionize medicine.”
To this end, Siemens Healthineers has built up a vast database of more than 250 million annotated images, reports and operational data on which to train its new algorithms.
In the example of the digital twin, the AI system was trained to weave together data about the electrical and physical properties and the structure of a heart into a 3D image.
One of the main challenges was hiding the complexity and creating an interface that is easy to use, said Tommaso Mansi, a senior R&D director at Siemens Healthineers who developed the software.
To test the technology, Meder’s team created 100 digital heart twins of patients being treated for heart failure in a six-year trial. The computer makes predictions based on the digital twin and they are then compared with actual outcomes.
His team hopes to finish evaluating the predictions by the end of 2018. If the results are promising, the system will be tested in a larger, multi-center trial as the next step to getting the software approved by regulators for commercial use.
Siemens Healthineers declined to say when the technology might eventually be used by clinics or give details on how its digital heart, or models of other organs it is developing such as the lungs and liver, could be monetized.
IN DATA WE TRUST
Both GE and Philips are also working on versions of digital heart twins while non-traditional players have been active too.
Drawing on its experience of making digital twins to test bridges and machinery, French software firm Dassault Systemes (DAST.PA) launched the first commercial “Living Heart” model in May 2015, though it is only currently available for research.
Philips sells AI-enabled heart models that can, for example, turn 2D ultrasound images into data that helps doctors diagnose problems, or automatically analyze scans to help surgeons plan operations.
Its vision, like Siemens Healthineers, is to add more complexity to its existing heart models by pulling together scans, ECGs and medical records to create a model that can predict how a heart will respond to therapy in real life.
For now, such software is still in the early stages of development and companies will have to work with regulators to thrash out how predictive models can be approved before doctors are willing to trust a diagnosis generated by a machine.
Access to high-quality data with enough variation will be crucial, as will be the ability to interpret that data and turn it into something medical professionals can use, say experts.
In particular, models will have to be trained on rare cases as they get closer to perfection, said Vivek Bhatt, chief technology officer at GE Healthcare’s clinical care solutions division.
“It’s going to be extremely critical to have an ongoing process for getting more data, getting the right kind of data and getting data with those unique cases,” he said.
The established medtech players say their long-running relationships with hospitals and research institutes and vast networks of installed machines will give them an edge over new tech entrants.
Siemens Healthineers, GE Healthcare and Philips say their databases are fed with a mixture of publicly-available data, data from clinical trials or from collaborations with hospitals – as well as some data from customers. All the data is made anonymous and only used with patients’ consent, they say.
Still, some campaigners and academics worry about patients’ data being used primarily by companies as a commercial tool.
Boris Bogdan, managing director at Accenture’s www.accenture.com life science practice in Switzerland, believes the ownership of data is a grey zone that could lead to a patient backlash if companies start making fortunes from it.
“When Facebook started nobody really cared who owned the information,” he said.
“Now that people understand that Facebook earns tremendous money with their data, questions like data privacy, data usage and data monetization are becoming more visible.”
($1 = 0.8841 euros)
Reporting by Caroline Copley; editing by David Clarke
Our Standards:The Thomson Reuters Trust Principles.
0 notes
Text
Medtech firms gets personal with digital twins
New Post has been published on https://rwamztech.com/medtech-firms-gets-personal-with-digital-twins/
Medtech firms gets personal with digital twins
HEIDELBERG, Germany (Reuters) – Armed with a mouse and computer screen instead of a scalpel and operating theater, cardiologist Benjamin Meder carefully places the electrodes of a pacemaker in a beating, digital heart.
A three-dimensional printout of a human heart is seen at the Heidelberg University Hospital (Universitaetsklinikum Heidelberg) in Heidelberg, Germany, August 14, 2018. REUTERS/Ralph Orlowski
Using this “digital twin” that mimics the electrical and physical properties of the cells in patient 7497’s heart, Meder runs simulations to see if the pacemaker can keep the congestive heart failure sufferer alive – before he has inserted a knife.
The digital heart twin developed by Siemens Healthineers (SHLG.DE) is one example of how medical device makers are using artificial intelligence (AI) to help doctors make more precise diagnoses as medicine enters an increasingly personalized age.
The challenge for Siemens Healthineers and rivals such as Philips PHL.AS and GE Healthcare is to keep an edge over tech giants from Alphabet’s (GOOGL.O) Google to Alibaba (BABA.N) that hope to use big data to grab a slice of healthcare spending.
With healthcare budgets under increasing pressure, AI tools such as the digital heart twin could save tens of thousands of dollars by predicting outcomes and avoiding unnecessary surgery.
A shortage of doctors in countries such as China is also spurring demand for new AI tools to analyze medical images and the race is on to commercialize products that could shake up healthcare systems around the world.
While AI has been used in medical technology for decades, the availability of vast amounts data, lower computing costs and more sophisticated algorithms mean revenues from AI tools are expected to soar to $6.7 billion by 2021 from $811 million in 2015, according to a study by research firm Frost & Sullivan ww2.frost.com.
The size of the global medical imaging analytics software market is also expected to jump to $4.3 billion by 2025 from $2.4 billion in 2016, said data portal Statista www.statista.com.
“What started as an evolution is accelerating toward more of a revolution,” said Thomas Rudolph who leads McKinsey & Company’s www.mckinsey.com pharma and medical technology practice in Germany.
‘GPS OF HEALTHCARE’
For Siemens Healthineers and its traditional rivals, making the transition from being mainly hardware companies to medical software pioneers is seen as crucial in a field becoming increasingly crowded with new entrants.
Google has developed a raft of AI tools, including algorithms that can analyze medical images to diagnose eye disease, or sift through digital records to predict the likelihood of death.
Alibaba, meanwhile, hopes to use its cloud and data systems to tackle a shortage of medical specialists in China. It is working on AI-assisted diagnosis tools to help analyze images such as CT scans and MRIs.Siemens Healthineers, which was spun off from German parent Siemens (SIEGn.DE) in March, has outpaced the market in recent quarters with sales of medical imaging equipment thanks to a slew of new products.
But analysts say the German firm, Dutch company Philips and GE Healthcare, a subsidiary of General Electric (GE.N), will all come under pressure to prove they can save healthcare systems money as spending becomes more linked to patient outcomes and as hospitals rely on bulk purchasing to push for discounts.
Slideshow (16 Images)
Siemens Healthineers has a long history in the industry. It made the first industrially manufactured X-ray machines in 1896 and is now the world’s biggest maker of medical imaging equipment.
Now, Chief Executive Bernd Montag’s ambition is to transform it into the “GPS of healthcare” – a company that harnesses its data to sell intelligent services, as well as letting smaller tech firms develop Apps feeding off its database.
As it adapts, Siemens Healthineers has invested heavily in IT. It employs some 2,900 software engineers and has over 600 patents and patent applications in machine learning.
It is not alone. Philips says about 60 percent of its research and development (R&D) staff and spending is focused on software and data science. The company said it employs thousands of software engineers, without being specific.
MEDICAL REVOLUTION
Experts say the success of AI in medical technology will hinge on access to reliable data, not only to create models for diagnosis but also to predict how effective treatments will be for a specific patient in the days and years to come.
“Imagine that in the future, we have a patient with all their organ functions, all their cellular functions, and we are able to simulate this complexity,” said Meder, a cardiologist at Heidelberg University Hospital here in Germany who is testing Siemens Healthineers’ digital heart software.
“We would be able to predict weeks or months in advance which patients will get ill, how a particular patient will react to a certain therapy, which patients will benefit the most. That could revolutionize medicine.”
To this end, Siemens Healthineers has built up a vast database of more than 250 million annotated images, reports and operational data on which to train its new algorithms.
In the example of the digital twin, the AI system was trained to weave together data about the electrical and physical properties and the structure of a heart into a 3D image.
One of the main challenges was hiding the complexity and creating an interface that is easy to use, said Tommaso Mansi, a senior R&D director at Siemens Healthineers who developed the software.
To test the technology, Meder’s team created 100 digital heart twins of patients being treated for heart failure in a six-year trial. The computer makes predictions based on the digital twin and they are then compared with actual outcomes.
His team hopes to finish evaluating the predictions by the end of 2018. If the results are promising, the system will be tested in a larger, multi-center trial as the next step to getting the software approved by regulators for commercial use.
Siemens Healthineers declined to say when the technology might eventually be used by clinics or give details on how its digital heart, or models of other organs it is developing such as the lungs and liver, could be monetized.
IN DATA WE TRUST
Both GE and Philips are also working on versions of digital heart twins while non-traditional players have been active too.
Drawing on its experience of making digital twins to test bridges and machinery, French software firm Dassault Systemes (DAST.PA) launched the first commercial “Living Heart” model in May 2015, though it is only currently available for research.
Philips sells AI-enabled heart models that can, for example, turn 2D ultrasound images into data that helps doctors diagnose problems, or automatically analyze scans to help surgeons plan operations.
Its vision, like Siemens Healthineers, is to add more complexity to its existing heart models by pulling together scans, ECGs and medical records to create a model that can predict how a heart will respond to therapy in real life.
For now, such software is still in the early stages of development and companies will have to work with regulators to thrash out how predictive models can be approved before doctors are willing to trust a diagnosis generated by a machine.
Access to high-quality data with enough variation will be crucial, as will be the ability to interpret that data and turn it into something medical professionals can use, say experts.
In particular, models will have to be trained on rare cases as they get closer to perfection, said Vivek Bhatt, chief technology officer at GE Healthcare’s clinical care solutions division.
“It’s going to be extremely critical to have an ongoing process for getting more data, getting the right kind of data and getting data with those unique cases,” he said.
The established medtech players say their long-running relationships with hospitals and research institutes and vast networks of installed machines will give them an edge over new tech entrants.
Siemens Healthineers, GE Healthcare and Philips say their databases are fed with a mixture of publicly-available data, data from clinical trials or from collaborations with hospitals – as well as some data from customers. All the data is made anonymous and only used with patients’ consent, they say.
Still, some campaigners and academics worry about patients’ data being used primarily by companies as a commercial tool.
Boris Bogdan, managing director at Accenture’s www.accenture.com life science practice in Switzerland, believes the ownership of data is a grey zone that could lead to a patient backlash if companies start making fortunes from it.
“When Facebook started nobody really cared who owned the information,” he said.
“Now that people understand that Facebook earns tremendous money with their data, questions like data privacy, data usage and data monetization are becoming more visible.”
($1 = 0.8841 euros)
Reporting by Caroline Copley; editing by David Clarke
Our Standards:The Thomson Reuters Trust Principles.
0 notes
Text
32 B2B Content Marketing Case Studies for 2018
One of the great honors of working in the marketing agency world is seeing your work recognized. For me, an even greater honor is seeing the work of our clients and my team recognized and that’s exactly what happened at the 2018 Killer Content Awards.
This year the award in question went to our client Cherwell Software. Thanks to amazing work by Alison Munn and the Cherwell Software team (pictured above), as well as our team at TopRank Marketing, their integrated influencer content program drove 22% of all new sales pipeline revenue in 2017.
But this post isn’t about just one B2B content marketing story. It’s about 32 stories from an impressive collection of B2B brands. These award winners are case studies for content marketing that we can all learn from. A BIG THANKS goes to the team at B2B Marketing Exchange for sharing raw case study data and both Anne Leuman and Lane Ellis from my team at TopRank Marketing for their collaboration on word-smithing the content and capturing the images of this post.
Check out the case studies below covering a range of categories including:
Measurable ROI, Nurture Campaign
Multi-Touch Campaign
Account-Based Marketing Campaign
Sales Enablement Campaign
Buyer-Focused Content, Bundled Content
Influencer Content
Interactive Content
Short-Form Content
Video Content
Research-Based Content
Agency Partnership
Social Amplification
32 B2B Marketing Case Studies Featuring Killer Content and Performance Results
#1 – Ciox Health Project: Ciox Health partnered with Content4Demand to uncover new growth opportunities with target audiences (e.g. law firms). After creating detailed personas, they developed highly tailored content messaging for all stages of the buyer’s journey. The final campaign featured an infographic, interactive quizzes, interactive listicles, checklists, Q&A sessions, and mixed media video.
Results:
Reached 1,884 potential prospects
42.8% open rate
14.5% CTR
#2 – Equifax Project: Equifax developed a multi-touch campaign consisting of more than seven touch points, including emails, social posts, blogs, webinars, and promotional emails. Quarterly webinars were the centerpiece of the campaign, allowing Equifax to capitalize on existing economic trends and CreditTrends reporting that were relevant to their target audience.
Results:
Increased webinar registrations by over 200%
Nearly doubled webinar attendees
#3 – The Kount Project: The Kount team, a provider of award-winning anti-fraud technology, created the Fraud360 worldwide tours, regular webinars, and video ads, which were designed to provide market-specific content and tailored insights that focused on specific trends and industries.
Results:
Average of 450 registered webinar attendees per session
Thousands of views on video ads
Reached thousands of professionals in target regions, including Asia, Australia, and EMEA
#4 – Xactly Project: In order to prove its knowledge of buyer pain points and the effectiveness of its solutions, Xactly rolled out the Power of X campaign. Using customer testimonials and product demos, Xactly strived to nurture existing relationships and drive demand through an integrated, buyer-focused campaign across all segments, featuring a landing page hub, social promotion, direct mail, customer videos, and webinars.
Results: 280 leads generated
#5 – SAP Ariba Project: SAP Ariba wanted to create a complete lifecycle nurture program for each of its targeted personas: Procurement, Supply Chain, Finance, and IT. Working with DemandGen, SAP Ariba mapped all 80 emails appropriately and used non-promotional language to emphasize their “thought leadership” content.
Results: 454% higher open rate
#6 – ADP Project: To identify potential buyers and convert readers into sales opportunities, ADP developed a flagship Research Nurture Program. The program leverages website analytics, marketing automation, and scoring to identify key buyer personas, customize content, and send nurture emails for longer-term engagement.
Results:
Generated thousands of influenced sales opportunities Millions of dollars forecasted in total opportunity pipeline
#7 – Bottomline Technologies Project: Bottomline Technologies breathed new life into its quarterly awareness email campaigns by introducing themes that aligned with pop culture events. By making subtle tweaks, the company was also able to create relevant messaging for different lines of business (e.g. strategic finance, controller, accounts payable), including infographics, white papers, and checklists.
Results:
1,000 infographic downloads within 24 hours
62% of downloads were net-new contacts
#8 – Veracode Project: Veracode created the Application Security Program Journey multi-touch campaign to drive awareness and generate demand for application security. The integrated, multi-touch campaign consisted of various content mapped against the buyer’s journey, as well as multiple inbound and outbound promotional tactics.
Results:
4,000 inquiries
479 opportunities
241 wins
#9 – Optum Project: To promote the launch of its new brand, OptumIQ, Optum created Data In Focus, an event to attract decision makers and influencers in person and via a livestream. Over a six-week period leading up to the event, the company unveiled key event details via an integrated campaign utilizing email, paid and organic social, digital advertising, retargeting ads, direct mail, and more.
Results:
5,022 external registrations
Exceeded registration-to-attendee conversion rate goal by 33%
13.6 million impressions
886 marketing contacts
#10 – Broadridge Project: With a sales cycle that can be quite lengthy, Broadridge sought to create a campaign that would steadily educate target buyers — finance executives and operations/IT leaders — on their value proposition. The full-funnel campaign included interactive infographics, eBooks, executive briefs, and Q&A’s that addressed buyer pain points. Broadridge paired this campaign with an internal guide to educate sales on the campaign goals, individual assets, and follow-up conversation starters to ensure quality interactions with buyers.
Results:
2,133 MQLs
6,995 content downloads
#11 – Grant Thornton Project: The Growth and Future of Industry campaign from Grant Thornton was created to help business leaders understand ways to accelerate growth and manage disruption. With over 60 pieces of content and an extensive social media campaign, it is the single biggest research program and thought leadership campaign the company has ever undertaken. Grant Thornton also leveraged paid media — a first for the company — to improve campaign reach and visibility among clients and prospects.
Results:
Exceeded reach goal by 4x
Exceeded conversion rate goal by 7.5x
Industry-specific reach and conversion goals were also exceeded
#12 – OpenText Project: The OpenText Digital Disruption thought leadership campaign was launched to engage enterprise executives in a fun and engaging way as they strive to understand and embrace digital disruption. The campaign used a re-designed microsite to house a variety of assets with a fun superpower theme, allowing visitors to easily consume content — even binge it all in a single sitting.
Results:
9:12 average session duration
Increased social traffic to the microsite by 1,062%
#13 – Cherwell Software Project: Cherwell Software partnered with TopRank Marketing to develop a comprehensive influencer program for the IT service management industry. A 24-page eBook called IT Service Management 2020, kicked off the campaign, featuring influencer opinions about the future of the ITSM industry. To generate pre-launch interest, Cherwell produced and promoted several blog posts, an infographic, and co-hosted a webinar with the influencers.
Results:
100% share rate with influencers
240% greater download rate than the average gated asset
29% increase in web traffic to Cherwell.com from social
Leads from the campaign contributed to 22% of the revenue pipeline for 2017
#14 – Paycom Project: Paycom collaborated with best-selling author, keynote speaker and futurist Jacob Morgan on a series of content to give HR professionals a closer look into why employee engagement scores are at an all-time low despite increased employer investment. The campaign featured a two-part podcast, a webinar, and a series of thought leadership blog articles — all featuring Morgan.
Results: 255 live attendees, 30 of which signed up for a Paycom consultation 1,172 podcast downloads 494 podcast page views 1,410 blog post page views
#15 – Blackbaud Project: To differentiate the company’s two fundraising solutions, Blackbaud launched their Choose Your Solution campaign. The campaign featured an interactive quiz to help arts and cultural organizations identify the right fundraising solution based on their needs, and to help qualify leads faster and bypass repetitive introductory questions asked by sales reps.
Results:
36 influenced opportunities that resulted in $34,000 in pipeline
42% MQL-to-opportunity conversion rate
#16 – Uberflip Project: Uberflip created an interactive marketing maturity assessment and companion eBook that asked marketers to take a hard look and identify where they stand in their marketing path. The assessment enabled Uberflip to provide their sales team with better MQLs and gain more information about existing accounts.
Results:
907,843 impressions and 1,297 clicks on social media in just three months
38% question completion rate
64% average lead submission rate
#17 – Siemens PLM Software Project: To educate customers and prospects on digital twins and digital threads, Siemens PLM Software created a thought leadership initiative. This initiative included creating a series of blog posts answering common buyer questions on digital twins and threads.
Results:
3,800 page views across articles
Ranking No. 2 on google for “value of the digital twin” and No. 14 for “digital twin technology”
#18 – CAS Project: In order to help scientists and research leaders at research and development organizations define important problems and highlight the opportunities additional time could give them, CAS, a division of the American Chemical Society, developed the Where Does Your Time Go? infographic.
Results:
Generated 489 leads
20,400 views
#19 – Oracle Project: Oracle developed The Modern Finance Leader blog series to establish itself as a leader in the world of finance. The blog targets finance executives across North America, EMEA, and APAC and provides content designed to educate and inform the audience on the latest trends and topics in finance.
Results:
330 posts published
90,000 unique visits
500,000 page views
63% increase in web traffic quarter over quarter
#20 – Bottomline Technologies Project: Bottomline Technologies partnered Content4Demand to develop an interactive eBook designed to showcase how three organizations — from manufacturing, healthcare, and property management industries — used their Paymode-X network to elevate efficiency and improve their bottom line.
Results:
54.3% email open rate, 39.8% CTR, 73.4% click-to-open rate
362 downloads through content syndication
4 MQLs, 2 SAOs, and $3.2 million in associated pipeline
#21 – Anthem Blue Cross and Blue Shield Project: Anthem Blue Cross and Blue Shield collaborated with Skyword to revamp an existing piece of content, titled: The Benefits Guide. In response to new audience needs, Anthem pivoted the asset away from the Affordable Care Act (ACA) focus and replaced it with a newsroom that conveyed news and decisions relevant for plan holders.
Results:
103% increase in page views and 102% increase in search views from Q2 to Q3 in 2017
798,000 total page views from 2016 to 2017
#22 – SAP Project: SAP launched its #LifeAt video campaign to highlight their many innovators, game-changers, and true entrepreneurs, but SAP also sought to humanize the brand for its target audience. The SAP team partnered with the video marketing agency Aftermarq to produce video stories of SAP SMB clients of varying lengths.
Results:
4.5 million impressions
31% view-through rate for 5:00 videos
21% view-through rate for 1:00 videos
#23 – LinkedIn Project: LinkedIn’s Live with Marketers campaign is a live talk show by marketers for marketers, designed to resolve pain points around top-of-mind topics such as marketing attribution, ROI optimization, and driving business impact on social media.
Results:
12,000 registrants
5,000 live attendees
Increased projected revenue from deals closed through this series versus traditional webcasts
#24 – MATRIXX Software Project: MATRIXX Software designed its 150 Points of Opportunity campaign to differentiate their content from that of their competitors, while also showcasing how their product delivers value to customers. The campaign featured a 44-page eBook and five standalone videos.
Results:
77% return rate to the MATRIXX website
43% increase in average session duration
25% growth in C-suite interaction and target account engagement rate
#25 – Tempur Sealy Hospitality Project: Tempur Sealy Hospitality was looking for a way to present their high-quality mattresses to B2B buyers in the hospitality industry without having to lug around a physical sample. The company worked with The Mx Group to create an interactive mattress cutaway tool that allowed sales reps to digitally present and sell various mattresses to hospitality customers online and at industry trade shows.
Results: Achieved a 90% adoption rate with the sales force
#26 – LookBookHQ Project: In an effort to re-engage lost opportunities and give the sales team more prospects that were more likely to convert, LookBookHQ created their Caveman campaign. The campaign consisted of an interactive digital experience built on the LookBookHQ platform, a direct mail component, and follow-up email outreach from sales.
Results:
Booked 300 meetings
Generated more than 50 new opportunities
Saw a 56% overall conversion rate, up 27% from the previous year
#27 – ChannelAdvisor Project: ChannelAdvisor decided to create two unique ABM campaigns that targeted strategic accounts via direct mail. The two campaigns provided over 250 prospects with pre-loaded Amazon devices, featuring ChannelAdvisor skills and apps that educated prospects on e-commerce strategies that were relevant to them.
Results:
Achieved an ROI of 130%
39% of generated opportunities were net-new
#28 – Trapeze Group Project: Trapeze Group kicked off an ABM pilot with the objective to identify top accounts with which to deepen engagements and create personalized one-to-one messaging and campaigns — ultimately influencing closed-won opportunities. The ABM pilot has since been rolled out to 60 target accounts.
Results:
111% increase in session duration
100% response rate to the direct mail component
#29 – Harland Clarke Project: To drive awareness for the company’s new product, GRC Spotlight, Harland Clarke created the “Keeping Up with Kevin” campaign. The star and subject matter expert for the campaign, Kevin Malicki, participated in video blogs that were shared over social media — primarily LinkedIn — to help deliver tips and real-world scenarios in the GRC space.
Results:
33,000 LinkedIn impressions
Increased Malicki’s LinkedIn connections by 22%
Increased Malicki’s LinkedIn profile views by 110%
#30 – Ipswitch Project: Ipswitch created the “Defrag This” podcast and blog to help provide a trusted knowledge base for IT professionals that offers audience-centric content via social channels.
Results:
Nearly 200% growth in blog subscribers
174% increase in monthly visitors to the blog
133% increase in organic traffic to the blog
#31 – Radius Project: Radius’ Revenue Ops campaign was designed to help educate prospects in marketing and sales operations on how their role in B2B business is evolving — from simple execution to providing data and insights to help drive revenue. The campaign was fueled by an eBook that Radius co-created with companies such as Heinz Marketing, Engagio, Forrester, and more.
Results:
500 eBook downloads in the first two days
Engaged more than 300 top-tier accounts
Influenced more than $5 million in pipeline
#32 – Emma Project: Emma wanted to learn what makes today’s marketers tick, as well as promote collaboration and learning within the community. The company surveyed roughly 200 marketers and interviewed more than 25 industry experts to gauge the goals, concerns, and pressures facing marketers, then compiled the data into its first Email Marketing Industry Report.
Results:
Over 41,000 unique views
Contributed to 37% of the company’s content downloads
Top Takeaways for B2B Content Marketers
Themes of success from this collection of B2B content marketing examples include: data informed personas, personalization, interactive content, integrated content, thought leadership and influence. Of particular note was the use of live video by LinkedIn Marketing Solutions.
As interactive content has become a more common feature in award-winning B2B content in 2018, I think video will take that spot in 2019.
There’s a lot to learn from with award winning content marketing programs and I congratulate all the B2B brands that brought him Finny’s this year. The awards give recognition to great work and they also give us a look inside what’s really working in the industry.
Have you noticed a B2B content marketing campaign this year that was remarkable? If so, please share in the comments why you thought it was special.
Gain a competitive advantage by subscribing to the TopRank® Online Marketing Newsletter.
© Online Marketing Blog – TopRank®, 2018. | 32 B2B Content Marketing Case Studies for 2018 | https://ift.tt/faSbAI
The post 32 B2B Content Marketing Case Studies for 2018 appeared first on Online Marketing Blog – TopRank®.
http://www.toprankblog.com/
The post 32 B2B Content Marketing Case Studies for 2018 appeared first on Unix Commerce.
from WordPress https://ift.tt/2I2TDXp via IFTTT
0 notes
Text
32 B2B Content Marketing Case Studies for 2018
One of the great honors of working in the marketing agency world is seeing your work recognized. For me, an even greater honor is seeing the work of our clients and my team recognized and that’s exactly what happened at the 2018 Killer Content Awards.
This year the award in question went to our client Cherwell Software. Thanks to amazing work by Alison Munn and the Cherwell Software team (pictured above), as well as our team at TopRank Marketing, their integrated influencer content program drove 22% of all new sales pipeline revenue in 2017.
But this post isn’t about just one B2B content marketing story. It’s about 32 stories from an impressive collection of B2B brands. These award winners are case studies for content marketing that we can all learn from. A BIG THANKS goes to the team at B2B Marketing Exchange for sharing raw case study data and both Anne Leuman and Lane Ellis from my team at TopRank Marketing for their collaboration on word-smithing the content and capturing the images of this post.
Check out the case studies below covering a range of categories including:
Measurable ROI, Nurture Campaign
Multi-Touch Campaign
Account-Based Marketing Campaign
Sales Enablement Campaign
Buyer-Focused Content, Bundled Content
Influencer Content
Interactive Content
Short-Form Content
Video Content
Research-Based Content
Agency Partnership
Social Amplification
32 B2B Marketing Case Studies Featuring Killer Content and Performance Results
#1 – Ciox Health Project: Ciox Health partnered with Content4Demand to uncover new growth opportunities with target audiences (e.g. law firms). After creating detailed personas, they developed highly tailored content messaging for all stages of the buyer’s journey. The final campaign featured an infographic, interactive quizzes, interactive listicles, checklists, Q&A sessions, and mixed media video.
Results:
Reached 1,884 potential prospects
42.8% open rate
14.5% CTR
#2 – Equifax Project: Equifax developed a multi-touch campaign consisting of more than seven touch points, including emails, social posts, blogs, webinars, and promotional emails. Quarterly webinars were the centerpiece of the campaign, allowing Equifax to capitalize on existing economic trends and CreditTrends reporting that were relevant to their target audience.
Results:
Increased webinar registrations by over 200%
Nearly doubled webinar attendees
#3 – The Kount Project: The Kount team, a provider of award-winning anti-fraud technology, created the Fraud360 worldwide tours, regular webinars, and video ads, which were designed to provide market-specific content and tailored insights that focused on specific trends and industries.
Results:
Average of 450 registered webinar attendees per session
Thousands of views on video ads
Reached thousands of professionals in target regions, including Asia, Australia, and EMEA
#4 – Xactly Project: In order to prove its knowledge of buyer pain points and the effectiveness of its solutions, Xactly rolled out the Power of X campaign. Using customer testimonials and product demos, Xactly strived to nurture existing relationships and drive demand through an integrated, buyer-focused campaign across all segments, featuring a landing page hub, social promotion, direct mail, customer videos, and webinars.
Results: 280 leads generated
#5 – SAP Ariba Project: SAP Ariba wanted to create a complete lifecycle nurture program for each of its targeted personas: Procurement, Supply Chain, Finance, and IT. Working with DemandGen, SAP Ariba mapped all 80 emails appropriately and used non-promotional language to emphasize their “thought leadership” content.
Results: 454% higher open rate
#6 – ADP Project: To identify potential buyers and convert readers into sales opportunities, ADP developed a flagship Research Nurture Program. The program leverages website analytics, marketing automation, and scoring to identify key buyer personas, customize content, and send nurture emails for longer-term engagement.
Results:
Generated thousands of influenced sales opportunities Millions of dollars forecasted in total opportunity pipeline
#7 – Bottomline Technologies Project: Bottomline Technologies breathed new life into its quarterly awareness email campaigns by introducing themes that aligned with pop culture events. By making subtle tweaks, the company was also able to create relevant messaging for different lines of business (e.g. strategic finance, controller, accounts payable), including infographics, white papers, and checklists.
Results:
1,000 infographic downloads within 24 hours
62% of downloads were net-new contacts
#8 – Veracode Project: Veracode created the Application Security Program Journey multi-touch campaign to drive awareness and generate demand for application security. The integrated, multi-touch campaign consisted of various content mapped against the buyer’s journey, as well as multiple inbound and outbound promotional tactics.
Results:
4,000 inquiries
479 opportunities
241 wins
#9 – Optum Project: To promote the launch of its new brand, OptumIQ, Optum created Data In Focus, an event to attract decision makers and influencers in person and via a livestream. Over a six-week period leading up to the event, the company unveiled key event details via an integrated campaign utilizing email, paid and organic social, digital advertising, retargeting ads, direct mail, and more.
Results:
5,022 external registrations
Exceeded registration-to-attendee conversion rate goal by 33%
13.6 million impressions
886 marketing contacts
#10 – Broadridge Project: With a sales cycle that can be quite lengthy, Broadridge sought to create a campaign that would steadily educate target buyers — finance executives and operations/IT leaders — on their value proposition. The full-funnel campaign included interactive infographics, eBooks, executive briefs, and Q&A’s that addressed buyer pain points. Broadridge paired this campaign with an internal guide to educate sales on the campaign goals, individual assets, and follow-up conversation starters to ensure quality interactions with buyers.
Results:
2,133 MQLs
6,995 content downloads
#11 – Grant Thornton Project: The Growth and Future of Industry campaign from Grant Thornton was created to help business leaders understand ways to accelerate growth and manage disruption. With over 60 pieces of content and an extensive social media campaign, it is the single biggest research program and thought leadership campaign the company has ever undertaken. Grant Thornton also leveraged paid media — a first for the company — to improve campaign reach and visibility among clients and prospects.
Results:
Exceeded reach goal by 4x
Exceeded conversion rate goal by 7.5x
Industry-specific reach and conversion goals were also exceeded
#12 – OpenText Project: The OpenText Digital Disruption thought leadership campaign was launched to engage enterprise executives in a fun and engaging way as they strive to understand and embrace digital disruption. The campaign used a re-designed microsite to house a variety of assets with a fun superpower theme, allowing visitors to easily consume content — even binge it all in a single sitting.
Results:
9:12 average session duration
Increased social traffic to the microsite by 1,062%
#13 – Cherwell Software Project: Cherwell Software partnered with TopRank Marketing to develop a comprehensive influencer program for the IT service management industry. A 24-page eBook called IT Service Management 2020, kicked off the campaign, featuring influencer opinions about the future of the ITSM industry. To generate pre-launch interest, Cherwell produced and promoted several blog posts, an infographic, and co-hosted a webinar with the influencers.
Results:
100% share rate with influencers
240% greater download rate than the average gated asset
29% increase in web traffic to Cherwell.com from social
Leads from the campaign contributed to 22% of the revenue pipeline for 2017
#14 – Paycom Project: Paycom collaborated with best-selling author, keynote speaker and futurist Jacob Morgan on a series of content to give HR professionals a closer look into why employee engagement scores are at an all-time low despite increased employer investment. The campaign featured a two-part podcast, a webinar, and a series of thought leadership blog articles — all featuring Morgan.
Results: 255 live attendees, 30 of which signed up for a Paycom consultation 1,172 podcast downloads 494 podcast page views 1,410 blog post page views
#15 – Blackbaud Project: To differentiate the company’s two fundraising solutions, Blackbaud launched their Choose Your Solution campaign. The campaign featured an interactive quiz to help arts and cultural organizations identify the right fundraising solution based on their needs, and to help qualify leads faster and bypass repetitive introductory questions asked by sales reps.
Results:
36 influenced opportunities that resulted in $34,000 in pipeline
42% MQL-to-opportunity conversion rate
#16 – Uberflip Project: Uberflip created an interactive marketing maturity assessment and companion eBook that asked marketers to take a hard look and identify where they stand in their marketing path. The assessment enabled Uberflip to provide their sales team with better MQLs and gain more information about existing accounts.
Results:
907,843 impressions and 1,297 clicks on social media in just three months
38% question completion rate
64% average lead submission rate
#17 – Siemens PLM Software Project: To educate customers and prospects on digital twins and digital threads, Siemens PLM Software created a thought leadership initiative. This initiative included creating a series of blog posts answering common buyer questions on digital twins and threads.
Results:
3,800 page views across articles
Ranking No. 2 on google for “value of the digital twin” and No. 14 for “digital twin technology”
#18 – CAS Project: In order to help scientists and research leaders at research and development organizations define important problems and highlight the opportunities additional time could give them, CAS, a division of the American Chemical Society, developed the Where Does Your Time Go? infographic.
Results:
Generated 489 leads
20,400 views
#19 – Oracle Project: Oracle developed The Modern Finance Leader blog series to establish itself as a leader in the world of finance. The blog targets finance executives across North America, EMEA, and APAC and provides content designed to educate and inform the audience on the latest trends and topics in finance.
Results:
330 posts published
90,000 unique visits
500,000 page views
63% increase in web traffic quarter over quarter
#20 – Bottomline Technologies Project: Bottomline Technologies partnered Content4Demand to develop an interactive eBook designed to showcase how three organizations — from manufacturing, healthcare, and property management industries — used their Paymode-X network to elevate efficiency and improve their bottom line.
Results:
54.3% email open rate, 39.8% CTR, 73.4% click-to-open rate
362 downloads through content syndication
4 MQLs, 2 SAOs, and $3.2 million in associated pipeline
#21 – Anthem Blue Cross and Blue Shield Project: Anthem Blue Cross and Blue Shield collaborated with Skyword to revamp an existing piece of content, titled: The Benefits Guide. In response to new audience needs, Anthem pivoted the asset away from the Affordable Care Act (ACA) focus and replaced it with a newsroom that conveyed news and decisions relevant for plan holders.
Results:
103% increase in page views and 102% increase in search views from Q2 to Q3 in 2017
798,000 total page views from 2016 to 2017
#22 – SAP Project: SAP launched its #LifeAt video campaign to highlight their many innovators, game-changers, and true entrepreneurs, but SAP also sought to humanize the brand for its target audience. The SAP team partnered with the video marketing agency Aftermarq to produce video stories of SAP SMB clients of varying lengths.
Results:
4.5 million impressions
31% view-through rate for 5:00 videos
21% view-through rate for 1:00 videos
#23 – LinkedIn Project: LinkedIn’s Live with Marketers campaign is a live talk show by marketers for marketers, designed to resolve pain points around top-of-mind topics such as marketing attribution, ROI optimization, and driving business impact on social media.
Results:
12,000 registrants
5,000 live attendees
Increased projected revenue from deals closed through this series versus traditional webcasts
#24 – MATRIXX Software Project: MATRIXX Software designed its 150 Points of Opportunity campaign to differentiate their content from that of their competitors, while also showcasing how their product delivers value to customers. The campaign featured a 44-page eBook and five standalone videos.
Results:
77% return rate to the MATRIXX website
43% increase in average session duration
25% growth in C-suite interaction and target account engagement rate
#25 – Tempur Sealy Hospitality Project: Tempur Sealy Hospitality was looking for a way to present their high-quality mattresses to B2B buyers in the hospitality industry without having to lug around a physical sample. The company worked with The Mx Group to create an interactive mattress cutaway tool that allowed sales reps to digitally present and sell various mattresses to hospitality customers online and at industry trade shows.
Results: Achieved a 90% adoption rate with the sales force
#26 – LookBookHQ Project: In an effort to re-engage lost opportunities and give the sales team more prospects that were more likely to convert, LookBookHQ created their Caveman campaign. The campaign consisted of an interactive digital experience built on the LookBookHQ platform, a direct mail component, and follow-up email outreach from sales.
Results:
Booked 300 meetings
Generated more than 50 new opportunities
Saw a 56% overall conversion rate, up 27% from the previous year
#27 – ChannelAdvisor Project: ChannelAdvisor decided to create two unique ABM campaigns that targeted strategic accounts via direct mail. The two campaigns provided over 250 prospects with pre-loaded Amazon devices, featuring ChannelAdvisor skills and apps that educated prospects on e-commerce strategies that were relevant to them.
Results:
Achieved an ROI of 130%
39% of generated opportunities were net-new
#28 – Trapeze Group Project: Trapeze Group kicked off an ABM pilot with the objective to identify top accounts with which to deepen engagements and create personalized one-to-one messaging and campaigns — ultimately influencing closed-won opportunities. The ABM pilot has since been rolled out to 60 target accounts.
Results:
111% increase in session duration
100% response rate to the direct mail component
#29 – Harland Clarke Project: To drive awareness for the company’s new product, GRC Spotlight, Harland Clarke created the “Keeping Up with Kevin” campaign. The star and subject matter expert for the campaign, Kevin Malicki, participated in video blogs that were shared over social media — primarily LinkedIn — to help deliver tips and real-world scenarios in the GRC space.
Results:
33,000 LinkedIn impressions
Increased Malicki’s LinkedIn connections by 22%
Increased Malicki’s LinkedIn profile views by 110%
#30 – Ipswitch Project: Ipswitch created the “Defrag This” podcast and blog to help provide a trusted knowledge base for IT professionals that offers audience-centric content via social channels.
Results:
Nearly 200% growth in blog subscribers
174% increase in monthly visitors to the blog
133% increase in organic traffic to the blog
#31 – Radius Project: Radius’ Revenue Ops campaign was designed to help educate prospects in marketing and sales operations on how their role in B2B business is evolving — from simple execution to providing data and insights to help drive revenue. The campaign was fueled by an eBook that Radius co-created with companies such as Heinz Marketing, Engagio, Forrester, and more.
Results:
500 eBook downloads in the first two days
Engaged more than 300 top-tier accounts
Influenced more than $5 million in pipeline
#32 – Emma Project: Emma wanted to learn what makes today’s marketers tick, as well as promote collaboration and learning within the community. The company surveyed roughly 200 marketers and interviewed more than 25 industry experts to gauge the goals, concerns, and pressures facing marketers, then compiled the data into its first Email Marketing Industry Report.
Results:
Over 41,000 unique views
Contributed to 37% of the company’s content downloads
Top Takeaways for B2B Content Marketers
Themes of success from this collection of B2B content marketing examples include: data informed personas, personalization, interactive content, integrated content, thought leadership and influence. Of particular note was the use of live video by LinkedIn Marketing Solutions.
As interactive content has become a more common feature in award-winning B2B content in 2018, I think video will take that spot in 2019.
There’s a lot to learn from with award winning content marketing programs and I congratulate all the B2B brands that brought him Finny’s this year. The awards give recognition to great work and they also give us a look inside what’s really working in the industry.
Have you noticed a B2B content marketing campaign this year that was remarkable? If so, please share in the comments why you thought it was special.
Gain a competitive advantage by subscribing to the TopRank® Online Marketing Newsletter.
© Online Marketing Blog - TopRank®, 2018. | 32 B2B Content Marketing Case Studies for 2018 | http://www.toprankblog.com
The post 32 B2B Content Marketing Case Studies for 2018 appeared first on Online Marketing Blog - TopRank®.
from The SEO Advantages http://www.toprankblog.com/2018/05/b2b-content-marketing-case-studies-2018/
0 notes